Lower respiratory tract microbiome is associated with checkpoint inhibitor pneumonitis in lung cancer patients
Highlight box
Key findings
• The relative abundance of the Proteobacteria phylum (P<0.001) and Firmicutes phylum (P=0.01) was significantly higher in the checkpoint inhibitor pneumonitis (CIP) group than the non-CIP group.
What is known, and what is new?
• The gut microbiome is associated with the occurrence and severity of immune-related adverse events in cancer patients undergoing immunotherapy.
• The lower respiratory tract (LRT) microbiome is associated with CIP in lung cancer patients.
What is the implication, and what should change now?
• The relative abundance of the Proteobacteria and Firmicutes phyla in the LRT may hold potential as a biomarker for CIP in lung cancer patients receiving immune checkpoint inhibitors. Studies with more patients are needed to confirm these results.
Introduction
The advent of immune checkpoint inhibitors (ICIs) revolutionized the treatment landscape of lung cancer, providing significant survival benefits by harnessing the body’s immune system (1,2). However, in addition to their remarkable efficacy, ICIs also have a distinct spectrum of immune-related adverse events (irAEs), among which checkpoint inhibitor pneumonitis (CIP) stands out as a significant concern (3-5). CIP, which is characterized by inflammation of the lung parenchyma secondary to immune activation, poses a formidable challenge in the management of lung cancer patients undergoing immunotherapy (6-10).
The human microbiome is associated with the development and progression of various diseases (11-15). Studies have shown that the gut microbiota is also associated with irAEs in cancer patients receiving immunotherapy (16-18). For instance, in patients with hepatobiliary cancers, a higher relative abundance of Prevotellamassilia timonensis in the gut microbiota was found to be correlated with more severe immunotherapy-related colitis (16). Similarly, in patients with melanoma, a higher abundance of Bacteroides intestinalis was linked to severe irAEs (17). Additionally, in non-small-cell lung cancer (NSCLC) patients, Bacteroides dorei was found to be enriched in those experiencing ICI-related skin toxicity (18).
The predominant phyla in healthy lungs are Bacteroidetes and Firmicutes (19). The disruption of the lung microbiome is associated with various lung diseases such as asthma, chronic obstructive pulmonary disease, idiopathic pulmonary fibrosis, and lung cancer (20-29). Moreover, the lung microbiome is believed to be associated with the prognosis of lung cancer patients receiving immunotherapy (30,31). However, the relationship between the lung microbiome and irAEs, particularly CIP, remains unclear. This relationship is crucial to understand the onset of irAEs from the microbial perspective and for the early prediction of such events.
Hence, our study sought to address this gap by analyzing of the lower respiratory tract (LRT) microbiome in lung cancer patients undergoing immunotherapy and experiencing CIP using metagenomic next-generation sequencing (mNGS) (32). Our analysis shed light on the microbial differences between CIP and non-CIP patients, providing insights into the association between the LRT microbiome and the occurrence of CIP in the context of lung cancer immunotherapy. We present this article in accordance with the STROBE reporting checklist (available at https://tlcr.amegroups.com/article/view/10.21037/tlcr-24-853/rc).
Methods
Patients
This retrospective study included a total of 52 patients diagnosed with lung cancer who underwent immunotherapy at Peking Union Medical College Hospital between October 1, 2020, and July 1, 2024. All patients had undergone mNGS analysis of LRT specimens, specifically bronchoalveolar lavage fluid (BALF). The patients were allocated to the CIP and non-CIP groups based on their final diagnosis determined via multidisciplinary discussion.
A diagnosis of CIP was considered if all three of the following criteria were met: (I) the patient had received treatment with ICIs; (II) imaging studies revealed new lung shadows; and (III) other potential causes such as lung infection, lung tumor progression, interstitial lung disease, pulmonary vasculitis, pulmonary embolism, and pulmonary edema were excluded.
mNGS
BALF specifically refers to BALF obtained through bronchoalveolar lavage (BAL) procedures. All BALF samples were collected via BAL procedures at sites of suspected pneumonia identified through imaging. To ensure sample quality, we defined a qualified BALF sample as one obtained by instilling 100 mL of sterile saline solution and recovering at least 35 mL of fluid.
Samples were then transported to the laboratory under cold-chain conditions to ensure rapid and secure delivery. Subsequently, next-generation sequencing (NGS) analysis and bioinformatics services were provided by KingMed Diagnostics (Tianjin, China). After sample homogenization, nucleic acids were extracted using the 2005-01 Nucleic Acid Extraction and Purification Kit (Genseq, Shanghai, China), and the extracted concentrations were quantified with a Qubit 4.0 fluorometer (Thermo Scientific, Waltham, MA, USA). For library preparation, 5 ng of extracted DNA was used with the 2102 Sequencing Reaction Preparation Universal Kit (Genseq), with adaptor primers added during the process. Library quality was assessed again using the Qubit 4.0 fluorometer before sequencing, which was performed on the MGISEQ-200 platform (MGI, Shenzhen, China) with a single-end 50 bp read length.
The reference pathogen database included all species cataloged in sources such as the Manual of Clinical Microbiology, Clinical Microbiology Diagnosis and Interpretation, and the NCBI RefSeq Genome Database (https://www.ncbi.nlm.nih.gov/refseq/). Initial sequencing results were filtered to exclude species with <1% genome coverage and >2× depth or any species deemed background contamination by comparison to the negative control, using historical fluctuation data. For bacteria, viruses, and parasites, the top 10 organisms by read count were reported and interpreted alongside clinical data to identify likely pathogens.
Statistical analysis
We conducted the microbial diversity analysis using the R package vegan (version 2.6-4). The alpha-diversity analysis included calculations of abundance-based coverage estimator (ACE), Chao1, Simpson, Shannon, and observed species indexes. For the beta-diversity analysis, we used the Bray-Curtis distance metric and a principal coordinates analysis. The Anosim analysis was employed to determine if there were statistically significant differences in the microbial distribution between the CIP and non-CIP groups. Additionally, we performed a linear discriminant analysis (LDA) effect size (LEFse) analysis to identify any microbes that differed significantly between the CIP and non-CIP patients using R software (version 4.1.1; R Foundation for Statistical Computing, Vienna, Austria).
The categorical variables were compared using either the χ2 test or Fisher’s exact test, while the continuous variables were compared using either the independent t-test or Mann-Whitney U test. All the statistical analyses were two-tailed, and a P value <0.05 was considered statistically significant.
Ethical approval
This study was conducted in accordance with the Declaration of Helsinki (as revised in 2013) and was approved by the Ethics Committee of Peking Union Medical College Hospital (approval number: I-24PJ1079). The patients provided their written informed consent to participate in this study.
Results
Baseline characteristics
A total of 52 patients were included in the study. Among these patients, 33 were confirmed to have CIP, while 19 were classified as non-CIP (of whom, nine had infections, seven had disease progression, two had radiation-related pneumonitis, and one had targeted therapy-related pneumonitis) (Figure 1). In the CIP group, 69.7% of the patients were male and 48.5% had squamous cell carcinoma. Previous smokers accounted for 66.7% of the patients, and 33.3% of the patients had a family history of tumors. Additionally, 78.8% of the patients in the CIP group received programmed cell death protein 1 (PD-1) therapy. There were no differences between the CIP and non-CIP groups in terms of clinical characteristics such as age, gender, pathology, smoking history, family history of tumors, and ICI type (Table 1).
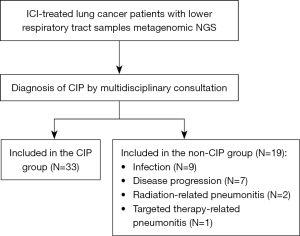
Table 1
Items | All patients (n=52) | Non-CIP (n=19) | CIP (n=33) | P value |
---|---|---|---|---|
Age (years) | 0.15 | |||
≤65 | 26 (50.0) | 7 (36.8) | 19 (57.6) | |
>65 | 26 (50.0) | 12 (63.2) | 14 (42.4) | |
Gender | 0.76 | |||
Female | 15 (28.8) | 5 (26.3) | 10 (30.3) | |
Male | 37 (71.2) | 14 (73.7) | 23 (69.7) | |
ECOG PS | 0.89 | |||
0–1 | 28 (53.8) | 10 (52.6) | 18 (54.5) | |
≥2 | 24 (46.2) | 9 (47.4) | 15 (45.5) | |
Treatment lines | 0.41 | |||
First line | 43 (82.7) | 14 (73.7) | 29 (87.9) | |
Second line | 5 (9.6) | 3 (15.8) | 2 (6.1) | |
Third line | 4 (7.7) | 2 (10.5) | 2 (6.1) | |
TNM staging | 0.76 | |||
III | 15 (28.8) | 5 (26.3) | 10 (30.3) | |
IV | 37 (71.2) | 14 (73.7) | 23 (69.7) | |
Pathology | 0.13 | |||
Adenocarcinoma | 22 (42.3) | 10 (52.6) | 12 (36.4) | |
Squamous cell carcinoma | 20 (38.5) | 4 (21.1) | 16 (48.5) | |
SCLC | 6 (11.5) | 2 (10.5) | 4 (12.1) | |
Others | 4 (7.7) | 3 (15.8) | 1 (3.0) | |
Smoking history | 0.60 | |||
No | 16 (30.8) | 5 (26.3) | 11 (33.3) | |
Yes | 36 (69.2) | 14 (73.7) | 22 (66.7) | |
Family history of tumor | 0.17 | |||
No | 38 (73.1) | 16 (84.2) | 22 (66.7) | |
Yes | 14 (26.9) | 3 (15.8) | 11 (33.3) | |
Type of ICI | 0.76 | |||
PD-1 inhibitor | 43 (82.7) | 17 (89.5) | 26 (78.8) | |
Pembrolizumab | 27 (51.9) | 11 (57.9) | 16 (48.5) | |
Tislelizumab | 11 (21.2) | 5 (26.3) | 6 (18.2) | |
Camrelizumab | 3 (5.8) | 1 (5.3) | 2 (6.1) | |
Sintilimab | 2 (3.8) | 0 (0.0) | 2 (6.1) | |
PD-L1 inhibitor | 9 (17.3) | 2 (10.5) | 7 (21.2) | |
Grading of CIP | NA | NA | NA | |
1–2 | 18 (54.5) | |||
3–4 | 15 (45.5) | |||
Use of glucocorticoids | NA | NA | NA | |
No | 4 (12.1) | |||
Yes | 29 (87.9) | |||
Use of IVIG | NA | NA | NA | |
No | 27 (81.8) | |||
Yes | 6 (18.2) | |||
Use of IL-6 inhibitors | NA | NA | NA | |
No | 27 (81.8) | |||
Yes | 6 (18.2) | |||
Use of JAK inhibitors | NA | NA | NA | |
No | 31 (93.9) | |||
Yes | 2 (6.1) |
Data are presented as n (%). CIP, checkpoint inhibitor pneumonitis; ECOG PS, eastern cooperative oncology group performance status; TNM, tumor-node-metastasis; SCLC, small-cell lung cancer; ICI, immune checkpoint inhibitor; PD-1, programmed cell death protein 1; PD-L1, programmed death-ligand 1; NA, not applicable; IVIG, intravenous immunoglobulin; IL-6, interleukin-6; JAK, Janus kinase.
Besides, the radiologic patterns observed in 33 CIP patients were as follows: organizing pneumonia (OP) in 16 patients (48.5%), nonspecific interstitial pneumonia (NSIP) in 13 patients (39.4%), hypersensitivity pneumonitis (HP) in 3 patients (9.1%), and acute interstitial pneumonia (AIP) in 1 patient (3.0%). In the cohort of 33 CIP patients, 45.5% were classified as having severity grades 3–4. A total of 87.9% of CIP patients received glucocorticoids therapy, 18.2% received intravenous immunoglobulin (IVIG), 18.2% received interleukin-6 (IL-6) inhibitors, and 6.1% received Janus kinase (JAK) inhibitors.
Microbial diversity between the CIP and non-CIP groups
The alpha-diversity analysis showed that there were no significant differences in the ACE (P=0.33), Chao1 (P=0.96), Simpson (P=0.30), Shannon (P=0.61), and observed species indexes (P=0.98) between the CIP and non-CIP groups (Figure 2). The beta-diversity analysis also showed that there was no statistically significant difference in the microbial distribution between the two groups as determined by the Anosim test (P=0.58).
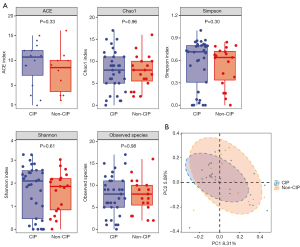
Microbial composition in the CIP and non-CIP groups
In the CIP group, the dominant phyla were Firmicutes (41.7%), Acinetobacter (18.2%), Proteobacteria (16.3%), Bacteroidetes (12.1%), and Ascomycota (3.4%). The dominant genera included Streptococcus (17.8%), Prevotella (9.8%), Veillonella (6.4%), Rothia (5.7%), Actinomyces (5.3%), Haemophilus (5.3%), Neisseria (4.9%), Staphylococcus (4.9%), Gemella (3.8%), and Corynebacterium (3.4%). In the non-CIP group, the dominant phyla were Firmicutes (38.2%), Acinetobacter (18.4%), Proteobacteria (17.8%), Bacteroidetes (11.2%), and Ascomycota (5.3%). In the non-CIP group, the dominant genera were Streptococcus (19.1%), Prevotella (10.5%), Rothia (7.9%), Veillonella (7.2%), Haemophilus (7.2%), Actinomyces (6.6%), Neisseria (5.3%), Corynebacterium (3.3%), Staphylococcus (2.6%), and Candida (2.6%). The distribution of the dominant phyla and genera in both groups is presented in Figure 3.
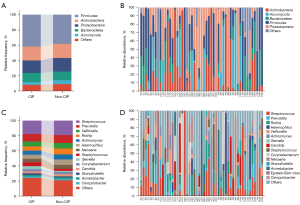
Subsequently, we conducted a detailed analysis of the top 50 species in the CIP and non-CIP groups (Figure 4). Among the top 50 species in both groups, 29 species were detected in common, including 24 bacteria, two fungi (Candida albicans and Candida parapsilosis), and three viruses [cytomegalovirus (CMV), Epstein-Barr virus (EBV), and human betaherpesvirus]. Further, 21 species were exclusively found in the CIP group, comprising 20 bacteria and one virus (human herpesvirus 1). Additionally, 21 species were exclusive to the non-CIP group, including 18 bacteria, two fungi (Pneumocystosis jiroveci and Aspergillus fumigatus), and one virus (human betaherpesvirus 6B).
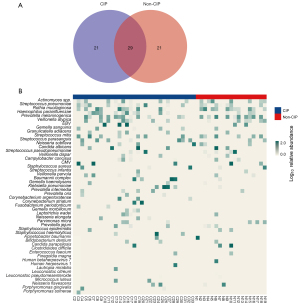
At the bacterial level, the most frequently detected species in the CIP group were Streptococcus pneumoniae (42.4%), Rothia mucilaginosa (42.4%), Actinomyces spp. (42.4%), Haemophilus parainfluenzae (39.4%), and Prevotella melaninogenica (36.4%). Similarly, in the non-CIP group, the dominant bacterial species included Rothia mucilaginosa (57.9%), Prevotella melaninogenica (52.6%), Actinomyces spp. (52.6%), Streptococcus pneumoniae (42.1%), and Veillonella atypica (36.8%). In both the CIP and non-CIP groups, the most commonly encountered fungi were Candida albicans and Candida parapsilosis. The most frequently detected viruses were EBV and CMV.
Microbial differences between the CIP and non-CIP groups
We further analyzed the differences in the relative abundance of the top five phyla between the CIP and non-CIP groups (Figure 5A). Notably, the relative abundance of the Proteobacteria phylum (P<0.001) and Firmicutes phylum (P=0.01) was significantly higher in the CIP group than the non-CIP group. However, there were no significant differences in the relative abundance of the Actinobacteria, Ascomycota, and Bacteroidetes phyla between the two groups. To further explore potential microbiome differences across radiologic patterns within CIP cohort, we compared the microbial composition between the two most prevalent patterns, OP and NSIP. Our analysis showed no significant differences in the relative abundance of various phyla between these two groups (Figure S1).
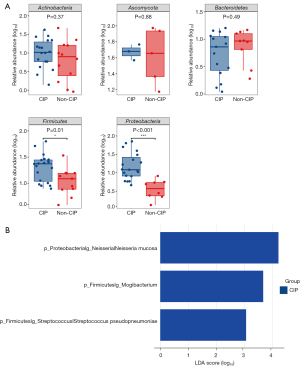
A LEFse analysis was conducted to further evaluate the differences between the CIP and non-CIP groups (Figure 5B). The level of Neisseria mucosa, which belongs to the Proteobacteria phylum, was significantly higher in the CIP group (P=0.02). The Proteobacteria phylum had the most substantial effect on the differences observed between the CIP and non-CIP groups with an LDA score of 4.2 for Neisseria mucosa. Additionally, the Mogibacterium genus and Streptococcus pseudopneumoniae, which are part of the Firmicutes phylum, were also significantly more abundant in the CIP group than the non-CIP group (P=0.02 and P=0.047, respectively). The Firmicutes phylum contributed to the differences between the groups, with LDA scores of 3.6 for Mogibacterium and 3.1 for Streptococcus pseudopneumoniae.
Discussion
In this study, we conducted an exploratory analysis of the LRT microbiome among CIP and non-CIP patients using mNGS, delineated the microbial composition, and compared differences between the CIP and non-CIP groups. Our findings revealed a notable distinction in the relative abundance of the Proteobacteria and Firmicutes phyla, both of which were significantly higher in the CIP group than the non-CIP group.
The role of microbiota in the development and prognosis of tumors, as well as the occurrence of adverse reactions in cancer patients undergoing immunotherapy, has been an area of increasing interest. However, much of the previous research in this field has predominantly focused on the gut microbiota (13,33,34). In recent years, attention has shifted toward investigating the relationship between the lung microbiota and lung cancer (22-24). For instance, Cheng et al. conducted a study in which they analyzed BALF samples from 32 lung cancer patients and 22 individuals with benign lung diseases using 16S ribosomal RNA amplicon sequencing. They observed a significant enrichment of six genera (i.e., TM7-3, Capnocytophaga, Sediminibacterium, Gemmiger, Blautia, and Oscillospira) in the BALF of lung cancer patients compared to those with benign lung diseases (35). Similarly, Kim et al. reported significant differences in the alpha- and beta-diversity of the BALF microbiota between lung cancer patients and those with benign lung diseases. Notably, the presence of unclassified_SAR202_clade, which belongs to the phylum Chloroflexi, was a prominent microbial difference between the two groups (24). These findings collectively suggested that lung cancer is associated with dysbiosis of the local microbiota, characterized by shifts in bacterial composition and alterations in diversity.
Further, the lung microbiome played a pivotal role in the prognosis of lung cancer patients. A study indicated that the preoperative LRT microbiome composition differed significantly between patients with postoperative recurrence and those without, suggesting a potential association between the preoperative LRT microbiome composition and early recurrence of NSCLC (36). Additionally, patients with higher diversity and abundance of the microbiome in lung tumor-distal normal tissue exhibited shorter disease-free survival and lower recurrence-free survival rates (37). For lung cancer patients receiving immunotherapy, the respiratory microbiota also played a role in predicting treatment efficacy. Chu et al. suggested that patients with pre-existing enrichment of Fusobacterium in the airway had a poorer response to immunotherapy (30). Jang et al. found that lung cancer patients with enrichment of Haemophilus influenzae and Neisseria perflava in BALF had a worse response to immunotherapy (31).
Mechanistically, the lung microbiome regulates both innate and adaptive immunity (25,38,39). Commensal bacteria in the upper respiratory tract were shown to defend against influenza virus infection in mice by polarizing M2 macrophages and secreting anti-inflammatory mediators such as interleukin-10 (IL-10) and transforming growth factor-β (38). The enrichment of the oropharyngeal microbiota in the lungs, such as Veillonella and Prevotella, was shown to be associated with an inflammatory phenotype, characterized by an increase in T helper 17 cell lymphocytes, the upregulation of inflammatory cytokines, and the decreased expression of Toll-like receptor 4 in alveolar macrophages (40). Thus, the lung microbiome is involved in the development and prognosis of lung cancer by shaping the tumor microenvironment and modulating the activity of tumor-infiltrating immune cells.
However, research on the relationship between respiratory microbiota and irAEs in lung cancer patients is limited. Our study revealed a significant increase in the relative abundance of the Proteobacteria and Firmicutes phyla in the LRT samples of the CIP group compared to pneumonia caused by other etiologies. Research has shown that an elevated abundance of Proteobacteria in the gut microbiome is associated with a poor prognosis in patients with solid tumors (41). Additionally, Liu et al. found that individuals with increased levels of Proteobacteria in the gut are more likely to experience serious adverse effects from immunotherapy, which is consistent with our findings (42).
In terms of the Firmicutes phylum, previous studies have consistently shown a favorable correlation between the Firmicutes phylum in the gut and the improved prognosis of cancer patients receiving immunotherapy (16,43,44). However, research on the relationship between the Firmicutes phylum and irAEs has yielded conflicting results. Mao et al. analyzed the mNGS results of fecal samples from 65 patients with advanced hepatobiliary carcinoma receiving PD-1 therapy and found enrichment of the Firmicutes phylum in patients with mild diarrhea, which suggests that Firmicutes phylum may exert a protective effect against immunotherapy-related toxicity (16). Similarly, Hakozaki et al. prospectively collected fecal samples from patients with advanced NSCLC undergoing immunotherapy and observed that patients with enrichment of the Firmicutes phylum had lower grades of irAEs (43). Conversely, Chaput et al. reported an increased incidence of ipilimumab-induced colonitis in patients with enriched Firmicutes phylum among those receiving ipilimumab treatment for metastatic melanoma (44). Further studies are warranted to examine the relationship between the Firmicutes phylum and irAEs, along with their underlying mechanisms.
This study provided preliminary insights into the associations between the LRT microbiome and CIP in lung cancer patients receiving immunotherapy. As it was not feasible to collect BAL samples from patients without respiratory symptoms, all patients included in this study had suspected pneumonitis and underwent BAL, which meant our findings might not apply as a predictive biomarker for all ICIs-treated patients. However, microbiome analysis might hold clinical significance in differentiating CIP from other causes of pneumonitis in ICIs-treated patients. This could support clinicians in making more informed decisions regarding the etiology of pneumonitis in such contexts.
Our study has several limitations. First, the relatively small sample size may limit the generalizability of our findings to a broader population. A larger, prospective multicenter study involving diverse patient groups would be valuable to confirm these results. Second, while some patients received antibiotics prior to sample collection, we did not separate patients based on antibiotic treatment status. This information could have provided more insight into how antibiotic usage may influence microbial composition (45). Finally, our study lacked paired BALF samples from the same patients before and after treatment, which restricted our ability to assess microbial changes associated with the onset of CIP over time. A future study design including matched pre- and post-treatment samples from each patient would enable a more thorough analysis of treatment-induced microbiome changes.
Conclusions
The relative abundance of the Proteobacteria and Firmicutes phyla was significantly higher in the CIP group than the non-CIP group, which suggested that the relative abundance of the Proteobacteria and Firmicutes phyla in the LRT may hold potential as a biomarker for CIP in lung cancer patients receiving ICIs.
Acknowledgments
Funding: This work was supported by funding from
Footnote
Reporting Checklist: The authors have completed the STROBE reporting checklist. Available at https://tlcr.amegroups.com/article/view/10.21037/tlcr-24-853/rc
Data Sharing Statement: Available at https://tlcr.amegroups.com/article/view/10.21037/tlcr-24-853/dss
Peer Review File: Available at https://tlcr.amegroups.com/article/view/10.21037/tlcr-24-853/prf
Conflicts of Interest: All authors have completed the ICMJE uniform disclosure form (available at https://tlcr.amegroups.com/article/view/10.21037/tlcr-24-853/coif). The authors have no conflicts of interest to declare.
Ethical Statement: The authors are accountable for all aspects of the work in ensuring that questions related to the accuracy or integrity of any part of the work are appropriately investigated and resolved. This study was conducted in accordance with the Declaration of Helsinki (as revised in 2013) and was approved by the Ethics Committee of Peking Union Medical College Hospital (approval number: I-24PJ1079). The patients provided their written informed consent to participate in this study.
Open Access Statement: This is an Open Access article distributed in accordance with the Creative Commons Attribution-NonCommercial-NoDerivs 4.0 International License (CC BY-NC-ND 4.0), which permits the non-commercial replication and distribution of the article with the strict proviso that no changes or edits are made and the original work is properly cited (including links to both the formal publication through the relevant DOI and the license). See: https://creativecommons.org/licenses/by-nc-nd/4.0/.
References
- Lahiri A, Maji A, Potdar PD, et al. Lung cancer immunotherapy: progress, pitfalls, and promises. Mol Cancer 2023;22:40. [Crossref] [PubMed]
- Herbst RS, Morgensztern D, Boshoff C. The biology and management of non-small cell lung cancer. Nature 2018;553:446-54. [Crossref] [PubMed]
- Shankar B, Zhang J, Naqash AR, et al. Multisystem Immune-Related Adverse Events Associated With Immune Checkpoint Inhibitors for Treatment of Non-Small Cell Lung Cancer. JAMA Oncol 2020;6:1952-6. [Crossref] [PubMed]
- Friedman CF, Proverbs-Singh TA, Postow MA. Treatment of the Immune-Related Adverse Effects of Immune Checkpoint Inhibitors: A Review. JAMA Oncol 2016;2:1346-53. [Crossref] [PubMed]
- Puzanov I, Diab A, Abdallah K, et al. Managing toxicities associated with immune checkpoint inhibitors: consensus recommendations from the Society for Immunotherapy of Cancer (SITC) Toxicity Management Working Group. J Immunother Cancer 2017;5:95. [Crossref] [PubMed]
- Guo X, Chen S, Wang X, et al. Immune-related pulmonary toxicities of checkpoint inhibitors in non-small cell lung cancer: Diagnosis, mechanism, and treatment strategies. Front Immunol 2023;14:1138483. [Crossref] [PubMed]
- Atchley WT, Alvarez C, Saxena-Beem S, et al. Immune Checkpoint Inhibitor-Related Pneumonitis in Lung Cancer: Real-World Incidence, Risk Factors, and Management Practices Across Six Health Care Centers in North Carolina. Chest 2021;160:731-42. [Crossref] [PubMed]
- Das S, Johnson DB. Immune-related adverse events and anti-tumor efficacy of immune checkpoint inhibitors. J Immunother Cancer 2019;7:306. [Crossref] [PubMed]
- Gomatou G, Tzilas V, Kotteas E, et al. Immune Checkpoint Inhibitor-Related Pneumonitis. Respiration 2020;99:932-42. [Crossref] [PubMed]
- Frost N, Unger K, Blum TG, et al. Management, risk factors and prognostic impact of checkpoint-inhibitor pneumonitis (CIP) in lung cancer - A multicenter observational analysis. Lung Cancer 2023;179:107184. [Crossref] [PubMed]
- Dohlman AB, Klug J, Mesko M, et al. A pan-cancer mycobiome analysis reveals fungal involvement in gastrointestinal and lung tumors. Cell 2022;185:3807-3822.e12. [Crossref] [PubMed]
- Pathak JL, Yan Y, Zhang Q, et al. The role of oral microbiome in respiratory health and diseases. Respir Med 2021;185:106475. [Crossref] [PubMed]
- Pitt JM, Vétizou M, Waldschmitt N, et al. Fine-Tuning Cancer Immunotherapy: Optimizing the Gut Microbiome. Cancer Res 2016;76:4602-7. [Crossref] [PubMed]
- Davenport ER, Sanders JG, Song SJ, et al. The human microbiome in evolution. BMC Biol 2017;15:127. [Crossref] [PubMed]
- Rajagopala SV, Vashee S, Oldfield LM, et al. The Human Microbiome and Cancer. Cancer Prev Res (Phila) 2017;10:226-34. [Crossref] [PubMed]
- Mao J, Wang D, Long J, et al. Gut microbiome is associated with the clinical response to anti-PD-1 based immunotherapy in hepatobiliary cancers. J Immunother Cancer 2021;9:e003334. [Crossref] [PubMed]
- Andrews MC, Duong CPM, Gopalakrishnan V, et al. Gut microbiota signatures are associated with toxicity to combined CTLA-4 and PD-1 blockade. Nat Med 2021;27:1432-41. [Crossref] [PubMed]
- Zhang F, Ferrero M, Dong N, et al. Analysis of the Gut Microbiota: An Emerging Source of Biomarkers for Immune Checkpoint Blockade Therapy in Non-Small Cell Lung Cancer. Cancers (Basel) 2021;13:2514. [Crossref] [PubMed]
- Dickson RP, Erb-Downward JR, Martinez FJ, et al. The Microbiome and the Respiratory Tract. Annu Rev Physiol 2016;78:481-504. [Crossref] [PubMed]
- Ren L, Zhang R, Rao J, et al. Transcriptionally Active Lung Microbiome and Its Association with Bacterial Biomass and Host Inflammatory Status. mSystems 2018;3:e00199-18. [Crossref] [PubMed]
- Quintero-Puerta T, Lira-Lucio JA, Falfán-Valencia R, et al. Lung microbiome alterations in patients with anti-Jo1 antisynthetase syndrome and interstitial lung disease. Front Cell Infect Microbiol 2023;13:1321315. [Crossref] [PubMed]
- Li R, Li J, Zhou X. Lung microbiome: new insights into the pathogenesis of respiratory diseases. Signal Transduct Target Ther 2024;9:19. [Crossref] [PubMed]
- Liu HX, Tao LL, Zhang J, et al. Difference of lower airway microbiome in bilateral protected specimen brush between lung cancer patients with unilateral lobar masses and control subjects. Int J Cancer 2018;142:769-78. [Crossref] [PubMed]
- Kim G, Park C, Yoon YK, et al. Prediction of lung cancer using novel biomarkers based on microbiome profiling of bronchoalveolar lavage fluid. Sci Rep 2024;14:1691. [Crossref] [PubMed]
- Paudel KR, Dharwal V, Patel VK, et al. Role of Lung Microbiome in Innate Immune Response Associated With Chronic Lung Diseases. Front Med (Lausanne) 2020;7:554. [Crossref] [PubMed]
- Huffnagle GB, Dickson RP, Lukacs NW. The respiratory tract microbiome and lung inflammation: a two-way street. Mucosal Immunol 2017;10:299-306. [Crossref] [PubMed]
- Goto T. Airway Microbiota as a Modulator of Lung Cancer. Int J Mol Sci 2020;21:3044. [Crossref] [PubMed]
- Yan X, Yang M, Liu J, et al. Discovery and validation of potential bacterial biomarkers for lung cancer. Am J Cancer Res 2015;5:3111-22.
- Liang W, Yang Y, Gong S, et al. Airway dysbiosis accelerates lung function decline in chronic obstructive pulmonary disease. Cell Host Microbe 2023;31:1054-1070.e9. [Crossref] [PubMed]
- Chu S, Cheng Z, Yin Z, et al. Airway Fusobacterium is Associated with Poor Response to Immunotherapy in Lung Cancer. Onco Targets Ther 2022;15:201-13. [Crossref] [PubMed]
- Jang HJ, Choi JY, Kim K, et al. Relationship of the lung microbiome with PD-L1 expression and immunotherapy response in lung cancer. Respir Res 2021;22:322. [Crossref] [PubMed]
- Diao Z, Han D, Zhang R, et al. Metagenomics next-generation sequencing tests take the stage in the diagnosis of lower respiratory tract infections. J Adv Res 2022;38:201-12. [Crossref] [PubMed]
- Davar D, Dzutsev AK, McCulloch JA, et al. Fecal microbiota transplant overcomes resistance to anti-PD-1 therapy in melanoma patients. Science 2021;371:595-602. [Crossref] [PubMed]
- Newsome RC, Gharaibeh RZ, Pierce CM, et al. Interaction of bacterial genera associated with therapeutic response to immune checkpoint PD-1 blockade in a United States cohort. Genome Med 2022;14:35. [Crossref] [PubMed]
- Cheng C, Wang Z, Wang J, et al. Characterization of the lung microbiome and exploration of potential bacterial biomarkers for lung cancer. Transl Lung Cancer Res 2020;9:693-704. [Crossref] [PubMed]
- Patnaik SK, Cortes EG, Kannisto ED, et al. Lower airway bacterial microbiome may influence recurrence after resection of early-stage non-small cell lung cancer. J Thorac Cardiovasc Surg 2021;161:419-429.e16. [Crossref] [PubMed]
- Peters BA, Hayes RB, Goparaju C, et al. The Microbiome in Lung Cancer Tissue and Recurrence-Free Survival. Cancer Epidemiol Biomarkers Prev 2019;28:731-40. [Crossref] [PubMed]
- Iwasaki A, Medzhitov R. Control of adaptive immunity by the innate immune system. Nat Immunol 2015;16:343-53. [Crossref] [PubMed]
- Dickson RP, Erb-Downward JR, Falkowski NR, et al. The Lung Microbiota of Healthy Mice Are Highly Variable, Cluster by Environment, and Reflect Variation in Baseline Lung Innate Immunity. Am J Respir Crit Care Med 2018;198:497-508. [Crossref] [PubMed]
- Segal LN, Clemente JC, Tsay JC, et al. Enrichment of the lung microbiome with oral taxa is associated with lung inflammation of a Th17 phenotype. Nat Microbiol 2016;1:16031. [Crossref] [PubMed]
- Huang C, Li M, Liu B, et al. Relating Gut Microbiome and Its Modulating Factors to Immunotherapy in Solid Tumors: A Systematic Review. Front Oncol 2021;11:642110. [Crossref] [PubMed]
- Liu W, Ma F, Sun B, et al. Intestinal Microbiome Associated With Immune-Related Adverse Events for Patients Treated With Anti-PD-1 Inhibitors, a Real-World Study. Front Immunol 2021;12:756872. [Crossref] [PubMed]
- Hakozaki T, Richard C, Elkrief A, et al. The Gut Microbiome Associates with Immune Checkpoint Inhibition Outcomes in Patients with Advanced Non-Small Cell Lung Cancer. Cancer Immunol Res 2020;8:1243-50. [Crossref] [PubMed]
- Chaput N, Lepage P, Coutzac C, et al. Baseline gut microbiota predicts clinical response and colitis in metastatic melanoma patients treated with ipilimumab. Ann Oncol 2017;28:1368-79. [Crossref] [PubMed]
- Schwartz DJ, Langdon AE, Dantas G. Understanding the impact of antibiotic perturbation on the human microbiome. Genome Med 2020;12:82. [Crossref] [PubMed]
(English Language Editor: L. Huleatt)