Construction and validation of a prognostic model based on oxidative stress-related genes in non-small cell lung cancer (NSCLC): predicting patient outcomes and therapy responses
Highlight box
Key findings
• This study identified 74 prognostic oxidative stress (OS)-related differentially expressed genes in non-small cell lung cancer (NSCLC), and developed a predictive risk-score model with high accuracy. The model was validated as an independent prognostic indicator for NSCLC.
• We also created a nomogram that integrates the risk score and tumor node metastasis (TNM) stage to predict patient survival.
• This study showed that patients in the high-risk group exhibited an immunosuppressive microenvironment and responded better to chemotherapy compared to those in the low-risk group.
What is known, and what is new?
• The role of OS in NSCLC has been widely recognized; however, its prognostic remains unclear until now.
• This study established a novel risk-score model that quantifies the prognostic impact of OS-genes in NSCLC.
What is the implication, and what should change now?
• The model has significant clinical implications for the stratification and personalized treatment of NSCLC patients.
• It advocates for a paradigm shift towards applying the risk score to guide therapeutic decisions, aiming to enhance patient outcomes through targeted interventions.
Introduction
Lung cancer has a high incidence and is the most common cause of cancer-related deaths worldwide (1). Based on pathological classification, lung cancer is classified into two main types: small cell lung cancer (SCLC) and non-small cell lung cancer (NSCLC). About 85% of lung cancers are classified as NSCLC (2). While early-stage NSCLC can be treated successfully by surgery, most patients are diagnosed at an advanced stage, where the 5-year survival rate is only 18.6% (3). Currently, drug resistance is the primary issue affecting the therapeutic efficacy of NSCLC. Like traditional chemotherapy, targeted drugs and immune checkpoint inhibitors (ICIs) also lead to drug resistance. Due to the heterogeneity of the tumor and the complexity of the tumor microenvironment, ICIs are highly effective only in patients with programmed cell death ligand 1 (PD-L1) >50% (about 30% of the total) (4). Therefore, there is an urgent need for new pharmacodynamic strategies to guide the treatment of NSCLC patients.
The pathogenesis of lung cancer is believed to be associated with the interaction between environmental risk factors and individual genetic susceptibility (5). Smoking and air pollution are recognized as major environmental risk factors for lung cancer (6-8). Tobacco smoke and polluted air are rich in carcinogens and oxidants, such as polycyclic aromatic hydrocarbons (PAHs). Oxidative stress (OS) caused by PAHs may be a key factor in the development of lung cancer (9). In addition, adiponectin is thought to contribute to the pathogenesis of lung cancer by inducing lipid peroxidation and altering the oxidation state (10). This finding provides new insights into the pathophysiological mechanism of NSCLC (11). Research has also demonstrated that applying reactive oxygen species (ROS) inducer to gefitinib- and erlotinib-resistant H1975 cell lines increases ROS levels and induces apoptosis in these cells (12). An excess of reactive oxygen free radicals disrupts the balance between oxidation and antioxidation leading to metabolic dysfunction and resulting in ROS accumulation and genetic damage (13). These genetic lesions can eventually trigger tumor development (14,15). Regulating ROS is emerging as a novel adjuvant anti-cancer strategy, offering hope for patients with drug-resistant NSCLC (16,17). A comprehensive understanding of the molecular mechanisms of redox homeostasis will provide crucial guidance for the rational treatment of tumors (18-20).
ROS is closely associated with both the innate and adaptive immune responses and plays a significant role in the development of various cancers. It can induce the immunogenic death of tumor cells, enhance the antigen-presenting capabilities of dendritic cells (DCs), and influence the proliferation, differentiation, and immune response of T cells. Additionally, ROS regulates the phenotype and function of other immune cells, such as tumor-associated macrophages (TAMs). Consequently, it is closely linked to drug sensitivity and the development of immune resistance (21,22). It has been shown that high expression of OS- and anoikis-related genes is associated with poor prognosis in men and lung NSCLC patients, and that M0 macrophages and the phosphatidylinositol 3-kinase (PI3K)/protein kinase B (Akt) pathway play a key role in OS-related apoptosis in NSCLC (23). However, there are still few studies on the prognostic value of ROS-related genes in NSCLC. In addition, the relationship between the expression of ROS-related genes and drug treatment responses remains unclear.
Over the years, several prognosis prediction models have been developed for NSCLC. These models incorporate various predictors such as clinical factors [age, gender, tumor node metastasis (TNM) stage], genetic mutations (epidermal growth factor receptor, Kirsten rat sarcoma viral oncogene homolog), and biomarkers (carcinoembryonic antigen, cytokeratin 19 fragment) (24-26). However, each of these models has its limitations. Clinical factor-based models may not fully capture the biological complexity of the disease. Genetic mutation-based models are limited by the fact that not all patients have detectable mutations, and biomarker-based models may lack specificity or sensitivity. For example, while some models using traditional biomarkers have shown promise, they often fail to accurately predict prognosis in heterogeneous patient populations.
To optimize therapy or develop new treatment strategies, the present study identified ROS-related genes, classified them using cluster analysis, and established and validated a risk-score model based on these genes. The expression of ROS genes in NSCLC was verified through immunohistochemistry (IHC), Western blot, and quantitative real-time polymerase chain reaction (qRT-PCR). In addition, the relationship between ROS and the immune microenvironment was analyzed by multiplex immunofluorescence (mIF). The sensitivity of chemotherapy drugs was tested using organoids. Our results are expected to be valuable for assessing prognosis and tailoring individual treatment plans for NSCLC patients. We present this article in accordance with the TRIPOD reporting checklist (available at https://tlcr.amegroups.com/article/view/10.21037/tlcr-24-888/rc).
Methods
Data source
The study was conducted in accordance with the Declaration of Helsinki (as revised in 2013). This study was approved by the Ethics Committee of The First Affiliated Hospital of Shandong First Medical University (No. YXLL-KY-081). Informed consent was obtained from all the patients. Six NSCLC patients who did not receive neoadjuvant therapy were recruited from the First Affiliated Hospital of Shandong First Medical University for this study. All patients underwent lobectomy or sublobar resection, and samples of tumor and adjacent normal tissue were collected. The gene expression profiles and clinical features of patients with NSCLC were retrieved directly from The Cancer Genome Atlas (TCGA) database (https://portal.gdc.cancer.gov/). GSE72094 was obtained from the Gene Expression Omnibus (GEO) database (https://www.ncbi.nlm.nih.gov/geo/) to verify the prognostic signature. ROS-related genes, referred to as OS-genes, were identified based on their involvement in the biological process of response to ROS, as annotated in the Gene Ontology (GO) database under the term (GO: 0000302). A total of 214 OS-genes were used for the downstream analysis.
Identification and functional analysis of OS-related differentially expressed genes (OS-DEGs) in NSCLC
The Differently Expressed Genes (DEGs) between 1,027 NSCLC and 108 adjacent control samples were identified by the “DESeq2” R package (v4.2.1; http://www.R-project.org) using a |log2 fold change|>1 and an adjusted P value <0.05 as the criteria. The OS-DEGs were obtained by overlapping the DEGs with the 214 OS-genes. The ClusterProfiler R package was used to identify the GO terms (https://www.geneontology.org/) and the Kyoto Encyclopedia of Genes and Genomes (KEGG) pathways (https://www.genome.jp/kegg/) that were significantly enriched among the OS-DEGs, applying a stringent criterion of an adjusted P value <0.05.
Identification of robust prognostic OS-DEGs in NSCLC
A total of 1,030 patients with complete expression profiles and survival information from TCGA-NSCLC cohort formed the training set. In the initial phase, we employed the training dataset to conduct a univariate Cox regression analysis to identify OS-DEGs significantly associated with survival outcomes, using a P value <0.05 as the criterion. Thereafter, the OS-DEGs identified by the univariate Cox regression analysis were further screened by the least absolute shrinkage and selection operator (LASSO) algorithm using the “glmnet” R package.
Construction and verification of the risk-score model in NSCLC
Based on the expression levels of the prognostic OS-DEGs and their corresponding coefficients, the risk score of each patient in the training set was calculated using the formula:
where “Coef” denotes the regression coefficients attributed to each gene, and “Exp” refers to the normalized expression levels of the signature genes. Patients in the training set were stratified into high- and low-risk groups based on the median risk-score value. The survival rates of these groups were compared by Kaplan-Meier analysis.
To assess the predictive accuracy of the risk-score model, the receiver operating characteristic (ROC) curves were generated with the “survivalROC” package in R. The predictive accuracy of the model is considered good if the area under the curves (AUC) is greater than 0.6. Additionally, to confirm the robustness of the model, the same set of analyses was applied to the GSE72094 validation cohort.
Construction of the nomogram to predict the prognosis of NSCLC
To identify the independent prognostic factors for NSCLC patients, a combination of clinical attributes, including age and TNM stage, along with the risk score, were analyzed using both univariate and multivariate Cox regression. The factors identified as independent prognostic determinants were subsequently used to develop a nomogram for predicting the 1-, 3-, and 5-year survival rates of the NSCLC patients. The accuracy of the nomogram was evaluated using calibration curves.
Exploration of the mechanisms underlying ROS-related NSCLC
To investigate the mechanisms underlying the prognostic impact of OS-DEGs on NSCLC, a multifaceted analysis was conducted. First, we performed a comparative analysis of the risk scores across various patient subgroups categorized by tumor stage (T1 to T4, indicating size and/or local extent of the primary tumor), node stage (N0/N1 versus N2/N3, reflecting the absence or presence and extent of regional lymph node involvement), metastasis stage (M0 versus M1, denoting the presence or absence of distant metastasis), and gender. This was done using the Wilcoxon test to assess the association between the risk score and NSCLC progression. Second, a gene set variation analysis (GSVA) of KEGG gene sets from the Molecular Signatures Database (MSigDB; https://www.gsea-msigdb.org/gsea/msigdb/) was conducted to identify the KEGG pathways significantly enriched in patients with low- versus high-risk scores, focusing on those with an adjusted P value of <0.05. Third, the ESTIMATE algorithm was employed to calculate the immune and stromal scores for each patient. Subsequently, the relationships between these scores and the individual risk scores were examined. Fourth, immune checkpoint data from the Tumor-Immune System Interactions and Drug Bank (TISIDB) database (http://cis.hku.hk/TISIDB/) was leveraged to discern variations between the two risk groups using the Wilcoxon test. Fifth, to extend our understanding of the effect of immunotherapy across different patient groups, the immune phenotype scores (IPSs) of the NSCLC patients from TCGA dataset were examined on The Cancer Imaging Archive (TCIA) platform (https://dev.cancerimagingarchive.net/). Finally, a comparative analysis of the tumor mutational burden (TMB) between the high- and low-risk groups was performed. Additionally, the mutation frequencies of the genes between these groups were scrutinized using mutation data from TCGA. The top 20 most frequently mutated genes were depicted in a waterfall plot, and genes with significant differences in mutation frequencies between the groups were identified.
RNA preparation and qRT-PCR
Total RNA was extracted from the tumor and adjacent normal tissues from 20 sets of samples using TRIzol reagent (Invitrogen, MA, USA). Isolated RNA was used for the reverse transcription reaction with HiScript III RT SuperMix for qRT-PCR (Vazyme, R323-01, Jiangsu, China). The relative RNA levels of the 11 prognostic OS-DEGs were detected using the indicated primers (Table S1). All the experiments were performed in triplicate. PCR product specificity was confirmed using the comparative cycle threshold (Ct), method and GAPDH was used as an internal control.
Organoid culture and drug sensitivity test
Twenty sets of NSCLC tissues were initially washed in a cold washing buffer containing 2% penicillin/streptomycin (Gibco, GrandIsland, USA) in advanced Dulbecco’s Modified Eagle Medium (DMEM)/F12 (Invitrogen) for 5 minutes. The tissues were then minced into fragments smaller than 1 mm3. Subsequently, the samples were digested with a digestion buffer containing 500 U/mL collagenase IV (Sigma-Aldrich, USA), 1.5 mg/mL collagenase II (Solarbio, Beijing, China), 20 mg/mL hyaluronidase (Solarbio), 0.1 mg/mL Dispase type II (Sigma-Aldrich), 10 mM ROCK inhibitor Y-27,632 (Sigma-Aldrich), and 1% fetal bovine serum in DMEM (Lonza) at 37 ℃ on a rocking platform for 30 minutes. Single cells were isolated by filtering the tissue-cell mixture through 100- and 70-µm filters.
The cell suspension was adjusted to [1–2] ×104 cells per well. Next, it was re-suspended in pre-cooled DMEM/F12 medium to reach the desired volume. Once mixed, 40 µL of the resulting cell-matrix mixture was added to each well of a 96-well plate. The seeded plate was incubated for 30 minutes, to allow the matrix gel to solidify completely. Subsequently, 500 µL of pre-warmed organoid culture medium was carefully added to each well by slowly pouring it along the walls of the 24-well plate. After 3 days, the formation of organoids was observed under a microscope. Organoids with a diameter greater than 50 µm were considered successfully formed and were collected for further experimentation.
Upon successful culturing, the medium was removed and replaced with 100 µL of drug-containing medium. This medium included either carboplatin (2.0 µg/mL; S47020, Yuanye Biotechnology Co., Ltd., Shanghai, China), paclitaxel (1.0 µg/mL; B21695, Yuanye Biotechnology Co., Ltd.), pemetrexed disodium (3.0 µg/mL; B27986, Yuanye Biotechnology Co., Ltd.), or combinations of carboplatin with paclitaxel or pemetrexed disodium. Optical images were captured on day 4 post-drug treatment, and organoid viability was assessed using the calcein acetoxymethyl ester (AM) and propidium iodide (PI) cell viability assay (40747ES76, Yeasen Biotechnology, Shanghai, China) following the manufacturer’s instructions. The organoids were stained with PI, which marks dead cells in red, and calcein, which marks live cells in green.
IHC and mIF analyses
Twenty sets of NSCLC tumor samples and their adjacent normal lung tissues were preserved by fixation in a 10% neutral-buffered formalin solution for an extended period. Subsequently, these tissues were embedded in paraffin blocks. For IHC analysis, thin sections cut from the paraffin blocks underwent antigen-retrieval using microwave energy to enhance protein staining on the tissue sections. The antibodies against human lactate dehydrogenase A (LDHA) (19987-1-AP), protein tyrosine phosphatase receptor type N (PTPRN) (10584-1-AP), ADA (13328-1-AP), and SESN3 (11431-2-AP) were purchased from Proteintech (Wuhan, China). The antibodies against human transient receptor potential cation channel subfamily A (TRPA1) (TA382859) and GPR37 (TA324840) was obtained from OriGene Technologies (Rockville USA). The antibodies against SIRPA (ab260039), CDK1 (ab133327), ECT2 (ab236502), COL1A1 (ab138492), and BTK (ab208937) were purchased from Abcam Biotechnology. In accordance with the manufacturer’s instructions, the sections were treated with the Envision + DAB kit (Dako, Glostrup, Denmark). As previously described (27), a semi-quantitative method was used to measure the staining intensity and the proportion of positive cells. For each tumor, 10 visual fields were observed at ×400 magnification, and 100 tumor cells in each field were counted. The positive cells were scored as follows: <5% (0 points); 5–25% (1 point); greater than 25% but less than or equal to 50% (2 points); greater than 50% but less than or equal to 75% (3 points); and >75% (4 points). The staining intensity was scored as follows: no staining (0 points); light yellow (1 point), yellow-brown (2 points); and dark brown (3 points). The product of the positive cell score and staining intensity score was used as the final score of expression, which was further graded as follows: (−), 0–1; (+), 2–3; (++), 4–6; and (+++), 8–12.
In this study, the three-color staining method was employed for mIF. Briefly, antigen retrieval was performed after paraffin section dewaxing. The section was immersed in a 3% hydrogen peroxide solution and subsequently incubated at room temperature, shielded from light for 25 minutes, a process designed to block the activity of endogenous peroxidases. Blockage was carried out; 10% rabbit serum was used if the primary antibody was derived from a goat, and for all other sources, 3% bovine serum albumin was used. The section then underwent further incubation for 30 min. Post blockage, the first primary antibody was introduced. The section was positioned in a humid chamber and incubated overnight at 4 ℃. A HRP-labelled secondary antibody corresponding to the primary antibody was subsequently added, followed by incubation for 50 min at room temperature. T Tyramide signal amplification was appropriately applied, and the sections were incubated at room temperature in the dark for 10 min. The tissue section was then transferred to a retrieval box containing an antigen-retrieval buffer and subjected to microwave heating. The type of retrieval solution used was consistent with the initial retrieval. The heating was set to medium for 8 min, followed by an 8-min pause, and then 7 min at medium-low heat. This process was repeated for the application of both the second primary antibody and the corresponding secondary antibody.
Following the addition of the third primary antibody, the section was returned to the humid chamber for overnight incubation at 4 ℃. A fluorescent secondary antibody corresponding to the primary antibody was added and incubated at room temperature for 50 min, avoiding exposure to light. For nuclear counterstaining, the sections were treated with a 4',6-diamidino-2-phenylindole (DAPI) staining solution and incubated for 10 minutes at room temperature in darkness. Spontaneous fluorescence quenching agent B was applied for 5 min, followed by rinsing under running water for 10 min. Subsequently, a fluorescence quenching cover slip solution was used to cover the slide.
Image acquisition was conducted using an upright fluorescence microscope (Nikon Eclipse C1, Tokyo, Japan) and a scanner (Pannoramic MIDI, 3DHISTECH, Budapest, Hungary). For DAPI staining, the excitation wavelength was set between 330 and 380 nm, and the emission wavelength was set at 420 nm, revealing a blue nuclear channel. For the 488 dyes, the excitation wavelength was set between 465 and 495 nm, and the emission wavelength was set between 515 and 555 nm, revealing a green positive channel. For CY3, the excitation wavelength was set between 510 and 560 nm, and the emission wavelength was set at 590 nm, revealing a red positive channel. For CY5, the excitation wavelength was set between 608 and 648 nm, and the emission wavelength was set between 672–712 nm, revealing a pink positive channel. The relevant markers were as follows: CD68 (GB113150, 1:500, Servicebio, Wuhan, China), CD20 (GB14030, 1:300, Servicebio), FAP (ab218164, 1:200, Abcam), CD8 (GB12068, 1:500, Servicebio), CD4 (GB113500, 1:200, Servicebio), CD11c (GB11059, 1:250, Servicebio), CD163 (GB113152, 1:500, Servicebio), CD86 (ab239075, 1:2,000, Abcam), and PD-L1 (ab213524, 1:500, Cambridge, UK).
Statistical methods
The DEGs were identified using the Wilcoxon test. Univariate Cox regression analysis focusing on overall survival was conducted to identify the OS-DEGs that were significantly correlated with the survival outcomes. Kaplan-Meier survival curves were drawn to visualize and statistically compare the survival distributions of the two distinct groups using the log-rank test. The Wilcoxon test was employed to assess the statistically significant differences between these groups. The relationship between the risk score, calculated by the prognostic model, and both stromal and immune scores was examined using Spearman correlation analysis. An unpaired t-test was used to determine the significance of differences between groups. The threshold for statistical significance was set at a P value <0.05.
Results
The associated DEGs are implicated in NSCLC
In the comparison between NSCLC and control samples, a total of 9,611 DEGs were identified. Of these, 6,854 were upregulated and 2,757 were downregulated (Figure 1A and table available at https://cdn.amegroups.cn/static/public/tlcr-24-888-1.xls). The expression levels of the top 15 upregulated and top 15 downregulated genes are shown in a heatmap (Figure 1B). After overlapping the DEGs with the OS-genes, 74 genes were identified as OS-DEGs (Figure 1C and Table S2). The functional analysis showed that the OS-DEGs were mainly enriched in 957 GO terms and 207 KEGG pathways relevant to ROS (Figure 2A,2B), including the response to ROS and chemical carcinogenesis ROS pathway. Finally, the protein-protein interactions (PPIs) of the candidate genes were explored using the Search Tool for the Retrieval of Interacting Genes/Proteins (STRING) database (https://cn.string-db.org/) (Figure S1).
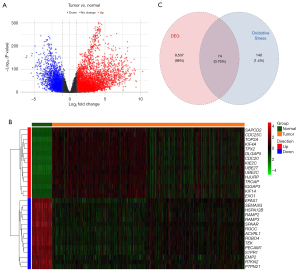
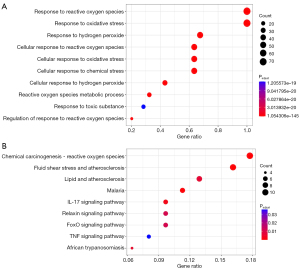
The construction and validation of an OS gene-related risk-score model for NSCLC
Subsequently, the prognostic significance of the 74 OS-DEGs in NSCLC was assessed by univariate Cox and LASSO regression analyses. The univariate Cox regression analysis showed that LDHA, PTPRN, TRPA1, GPR37, SIRPA, CDK1, ECT2, ADA, COL1A1, SESN3, and BTK were significantly correlated with the survival of the NSCLC patients (Figure 3A). To establish a more robust prognostic signature, the 11 OS-DEGs were input into the LASSO algorithm, and LDHA, PTPRN, TRPA1, GPR37, SIRPA, CDK1, ECT2, ADA, COL1A1, SESN3, and BTK were identified as prognostic OS-DEGs (Figure 3B,3C). Next, the risk score of each NSCLC patient in the training set was calculated using the following formula:
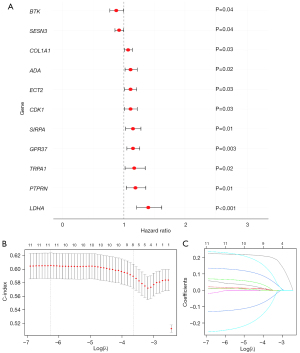
Next, the expression levels of the 11 genes that comprised the risk score were visualized (Figure S2A), and the correlation between the expression levels of the 11 model genes was analyzed using the Spearman correlation coefficients (Figure S2B).
Based on the median risk score value, the NSCLC patients in the TCGA training set were categorized into high- and low-risk groups (Figure 4A). An increase in the risk score was correlated with a higher number of fatalities (Figure 4B). The high-risk group exhibited poorer survival rates than the low-risk group (Figure 4C). The area under the curves (AUCs) for the 1-, 3-, and 5-year predictions were 0.661, 0.648, and 0.634, respectively (Figure 4D). The risk-score model was also validated using the GSE72094 dataset, and similar findings were observed (Figure 5A-5C). In the validation set, the AUCs were 0.643, 0.648, and 0.662 for the 1-, 3-, and 5-year predictions, respectively (Figure 5D), further confirming the model’s reliability in predicting the survival of NSCLC patients.
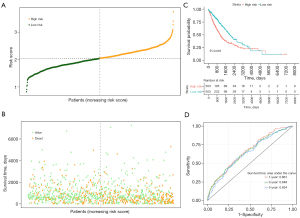
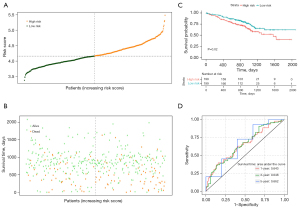
Development of an OS gene-related nomogram in NSCLC
We performed univariate and multivariate analyses and found that the risk score and TNM stage were independent prognostic factors in NSCLC (Figure 6A,6B). Using the identified independent prognostic factors, a predictive nomogram was developed to estimate the 1-, 3-, and 5-year survival rates of NSCLC patients (Figure 6C). The calibration curves showed that the predicted probabilities of the nomogram for overall survival closely matched the actual survival rates (Figure 6D-6F), showing the accuracy of the nomogram.
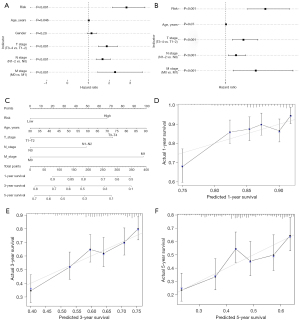
Prognostic OS-genes may influence the progression of NSCLC by regulating tumor cell proliferation and invasion
To further examine the influence of the prognostic OS-genes, a comparative analysis of the risk scores was conducted across groups categorized by T stage, N stage, M stage, and gender was conducted. Notably, the results showed that the risk scores increased as NSCLC advanced. Specifically, we found that patients in the T4 stage, N2 stage, and male patients had the highest risk scores (Figure 7A-7C). This observation suggests that the prognostic OS-genes significantly contribute to the severity and lymphatic spread of NSCLC, potentially affecting patient survival.
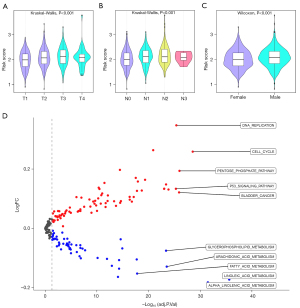
Additionally, a GSVA was employed to explore the underlying molecular mechanisms. This analysis revealed significant enrichment of specific KEGG pathways within the high-risk group, particularly those associated with cell-cycle regulation, DNA replication, the pentose phosphate pathway, and the P53 signaling pathway (Figure 7D). These pathways are crucial for tumor cell proliferation. These findings suggest that the prognostic OS-genes may influence the growth and progression of NSCLC by promoting tumor cell proliferation.
The GO biological processes (BPs), cellular components (CCs), and molecular functions (MFs) were also examined by GSVA. The results indicated significant enrichment of the glucose catabolic process (Figure S2C), cell cleavage furrow, and cell division site (Figure S2D) in the high-risk group. In contrast, the cellular response to hydroperoxide (Figure S2C) and oxidoreductase activity (Figure S2E) were significantly enriched in the low-risk group. These findings suggest that the prognostic OS-genes may affect cell division and proliferation, and that the ROS-related pathways are notably activated in the low-risk group.
Prognostic OS-genes exert significant effects on the chemotherapy outcomes in NSCLC
We successfully constructed NSCLC organoids and assessed the effects of carboplatin, paclitaxel, pemetrexed disodium, and their combinations on these models. The results indicated significant difference in the efficacy of paclitaxel and pemetrexed disodium between the low- and high-risk groups. However, carboplatin demonstrated superior anti-tumor activity in the low-risk group compared to the high-risk group. Notably, the combination of carboplatin and paclitaxel exhibited a higher tumor inhibition rate in the low-risk group. Furthermore, the combination of carboplatin and pemetrexed disodium showed the most potent anti-tumor effect in the low-risk group in our study (Figure 8 and Figure S3).
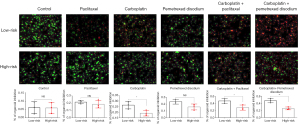
Prognostic OS-genes exerts significant effects on the immune microenvironment, immune checkpoint genes, and immunotherapy of NSCLC
A growing body of research shows the pivotal role of the immune microenvironment in the prognosis of NSCLC. Thus, we investigated the potential of OS-genes to influence the immune microenvironment in NSCLC patients (21,28). Our analysis revealed a strong significant correlation between the risk score and the immune score (P=6.68×10−5, Figure 9A). Building on this, we conducted a comparative assessment of immune cell infiltration between the low- and high-risk groups of patients. Using the CIBERSORT method (https://cibersortx.stanford.edu/), we identified variations in the levels of immune cell infiltration, including naïve B cells, resting DCs, M0 macrophages, M1 macrophages, and resting mast cells. Significant differences were observed (P<0.05, Figure 9B and Figure S4), suggesting that the OS-genes might influence the immune landscape in NSCLC and potentially affect patient outcomes.
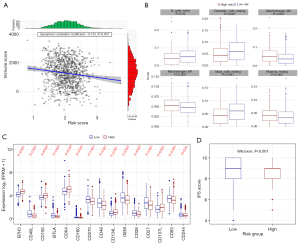
We then selected 34 key ICI genes for further research, and found that the expression of 15 ICI genes differed significantly between the low- and high-risk groups (Figure 9C and Table S3). We detected the expression of 11 prognostic genes in the NSCLC samples using qRT-PCR (Figure S5). To determine whether there is a difference in immunotherapy sensitivity between NSLCLC patients in the low-risk and high-risk groups, we calculated the Immune Phenotype Scores (IPS) of the patients. The results showed that patients in the low-risk group are more likely to benefit from immunotherapy (Figure 9D, P<0.001). The high- and low-risk groups were categorized based on the expression of OS genes and the assessment of risk scores following IHC analysis (Figure 10).
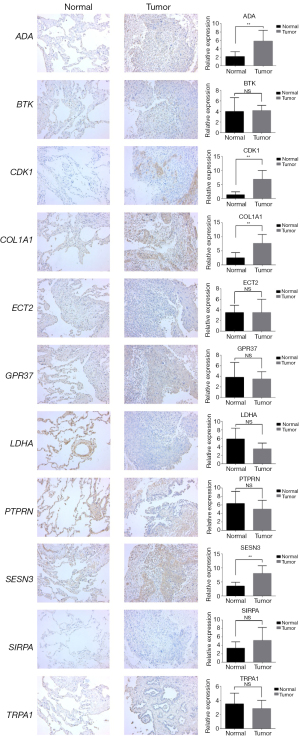
MIF staining was used to examine the immune microenvironment of NSCLC in the high- and low-risk groups. The results showed that the expression levels of the CD8 (P<0.05, Figure 11A-11C), and CD4 (P<0.05, Figure 11D-11F) differed significantly. However, the expression of CD11c cells did not show a significantly different between the high- and low-risk groups (P>0.05, Figure 11G-11I). Figure 11J-11L display the combined staining results.
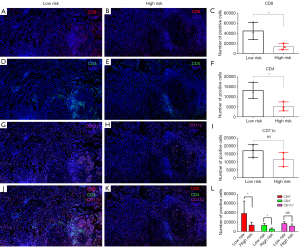
Interestingly, the expression of CD20 (P<0.05, Figure S6A-S6C) was significantly increased in the low-risk group, while the expression of cancer-associated fibroblasts (CAFs) was significantly higher in the high-risk group than in the low-risk group (FAP+, P<0.05, Figure S6D-S6F). Additionally, our MIF staining results indicated that PD-L1 expression was significantly higher in the low-risk group compared to the high-risk group (P<0.05, Figure S6G-S6I). The combined results are presented in Figure S6J-S6L.
The expression of macrophages (CD68, P<0.05, Figure S7A-S7C) and M2 macrophages (CD163, P<0.05, Figure S7D-S7F) was significantly increased in the high-risk group. The expression of CD86 cells was not significantly different between the high- and low-risk groups (P>0.05, Figure S7G-S7I). The combined results are presented in Figure S7J-S7L.
Relationship between the TMB and prognostic OS-genes
TMB was determined using somatic mutation data sourced from TCGA and then compared between the two groups. TMB was significantly higher in the high-risk group than in the low-risk group (Figure 12A). A visual representation in the form of a waterfall plot revealed that the most prevalent somatic mutations in the high-risk group involved the TP53, TTN, CSMD3, MUC16, and RYR2 genes (Figure 12B). Conversely, in the low-risk group, the most common mutations were observed in the TP53, TTN, MUC16, RYR2, and CSMD3 genes (Figure 12C). A univariate Cox analysis was conducted to compare the two groups, revealing that the mutation frequencies of KRAS and SETX were notably higher in the high-risk group than in the low-risk group (Figure 12D).
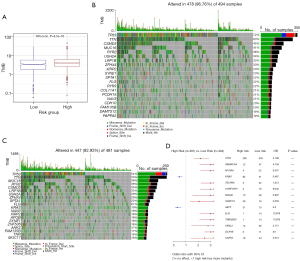
Discussion
As cancer treatment enters the era of precision therapy, a large number of clinical studies have shown that targeted drugs, such as tyrosine kinase inhibitors (TKIs) and anti-angiogenic drugs, significantly prolong progression-free survival and improve the quality of life of patients with NSCLC (29,30). However, research has shown that drug resistance is inevitable in targeted therapy (4). The advent of ICIs has led to promising treatment strategies. However, ICIs are associated with low response rates and irreversible fatal autoimmune toxicity in patients (31,32). The lack of reliable biomarkers, particularly effective predictive targets, contributes to the poor prognosis of NSCLC patients. Therefore, a comprehensive understanding of the NSCLC microenvironment is essential to enhance personalized treatment strategies. It is crucial to develop curative effect predictors validated by evidence-based medicine, and to further classify and refine treatment intervention decisions to ensure accurate and effective treatment (33,34).
Given that ROS are implicated in the onset and progression of NSCLC, the heterogeneity of ROS in NSCLC is closely associated with drug response and prognosis (17,21). Previous studies have explored the differential classification of ROS and its prognostic implications in lung adenocarcinoma (LUAD) (35,36). Unfortunately, validations of the expression of relevant markers and their clinical utility remain inadequate. In this study, we analyzed the DEGs involved in redox homeostasis and successfully established a NSCLC risk-score model based on these DEGs. The prediction accuracy of the model was validated through Kaplan-Meier survival curve and ROC curve analyses using public datasets. The risk-score model we constructed was identified as an independent prognostic factor for NSCLC. GSVA immune cell infiltration, immune checkpoint, and TMB analyses were conducted to explore the underlying biological factors and drug sensitivity to ICIs. Finally, we assessed the expression of the risk genes in NSCLC and adjacent normal lung tissues, and the correlation between the risk score and drug sensitivity was confirmed by establishing organoids.
In this study, we used TCGA and GEO databases to obtain the NSCLC expression profile. We identified 74 ROS-related DEGs in NSCLC and control normal lung tissues, primarily involved in the biological processes and signaling pathway of ROS (37). Research indicates that ROS accumulation can induce DNA damage in lung cancer cells, while the removal of intracellular ROS can prevent cancer cell death (38,39). Further, the survival of lung cancer cells has been shown to depend on their ability to defend against ROS (40), and the excessive free radicals produced through oxidation are closely related to cancer development (41,42). ROS can directly damage hydrogen bonds in the DNA double helix, promote DNA double-strand breaks, and fully expose the internal base groups to ROS. This exposure promotes the oxidative modification of DNA bases and results in mismatches, deletions, insertions, and chromosomal translocation in the nucleus (43). Moreover, ROS may serve as a second messenger to regulate cell proliferation and its associated signaling pathways. For instance, PTP1B and PTPN2 phosphatases which contain cysteine active sites, can be oxidized and inactivated by ROS. This inactivation, in turn, can activate the mitogen-activated protein kinase (MAPK)/extracellular regulated kinase 1/2 (ERK1/2), PI3K/AKT, and nuclear factor κB (NF-κB) signaling pathways, thereby promoting tumor cell proliferation (44).
We further identified LDHA, PTPRN, TRPA1, GPR37, SIRPA, CDK1, ECT2, ADA, COL1A1, SESN3, and BTK as prognostic biomarkers of NSCLC through univariate Cox and LASSO regression analysis. Based on these biomarkers, we constructed a risk-score model. Both univariate and multivariate Cox regression analyses confirmed that the risk score is an independent prognostic factor for NSCLC, demonstrating stable predictive utility across independent datasets. Specifically, LDHA, a key enzyme in glycolysis, catalyzes the conversion of pyruvate to lactic acid. Research indicates that the accumulation of lactic acid promotes NSCLC tumorigenesis by activating the NF-κB signaling pathway (45). Additionally, lactic acid induces the production of hypoxia-inducible factor-α (HIF-α) and enhances the expression of SRY related HMGBOX-2 (Sox-2) and other tumor stem cell factors, ultimately leading to tumor stem cell formation (46). SIRPA, an inhibitory receptor containing multiple intracellular tyrosine-based immunosuppressive motifs (47,48), serves as the principal receptor of CD47. CD47 triggers SIRPA phosphorylation through Src family kinases, and phosphorylated SIRPA recruits the phosphatases SHP-1 and SHP-2. The CD47-SIRPA axis plays a crucial role in inhibiting phagocytosis, a key consideration in NSCLC immunotherapy (49). A study has shown that PTPRN is highly upregulated and associated with metastasis and poor OS in patients with LUAD (50), where its overexpression enhances metastatic capacity by modulating the MEK/ERK and PI3K/AKT pathways.
The ion-channel protein TRPA1 is associated with LUAD, where its anchor protein plays an essential role in regulating fibroblast growth factor receptor 2 (FGFR2)-driven carcinogenesis (51). GPR37 has been implicated in promoting the malignant progression of LUAD through the TGF-β/Smad pathway (52). Depletion of GPR37 inhibits the expression of TGF-β1 and the phosphorylation of Smad2 and Smad3. Another study reported that GPR37 can promote LUAD by binding to CDK6 and thus presenting a potential theranostic target (53). CDK1, when phosphorylated, becomes inactivated, leading to inhibition of cell proliferation, colony formation, cell-cycle entry, and resistance to apoptosis, ultimately suppressing tumor growth in vivo (54). The expression of ECT2 is upregulated in LUAD, and targeting by miR-30a-5p results in the downregulation of ECT2, which inhibits the viability, migration, and invasion of LUAD cells (55). ADA expression in serum or malignant pleural effusion serves as a diagnostic marker for lung cancer (56). A study indicates that LINC00511 enhances COL1A1-mediated cell proliferation and the cell motility of LUAD by sponging miR-126-5p and miR-218-5p, and activates the PI3K/Akt signaling pathway via COL1A1 (57). SESN3, induced by ROS and activated through forkhead box O (FoxO), has an undefined mechanism in NSCLC that warrants further investigation (58). BTK mediates the stemness and epithelial mesenchymal transition characteristics of NSCLC, and its inhibition augments the efficacy of gefitinib and osimertinib in TKI-resistant NSCLC cells (59).
In the present study, we explored the potential mechanism through which 11 prognostic-related ROS markers influence the development of NSCLC. We discovered that risk scores based on these genes exhibited significant differences according to T stage, N stage, and sex, suggesting an association with higher clinical stages and increased mortality. Subsequent GSVA revealed significant enrichment of BPs and signaling pathways, such as the p53 signaling pathway, which are involved in the cell cycle, in the high-risk group. The p53 gene, a critical tumor suppressor, has its transcriptional activity influenced by ROS (60). ROS and p53 are linked through a mutation in the 8-oxoG gene, typically a response to ROS. The presence of 8-oxoG recruits the p53 protein and engages two base excision repair pathways (i.e., hOGG1 and APE). The interaction with p53 markedly enhances the combined activity of APE and hOGG1, enabling effective excision of the 8-oxoG residue (61). In addition, the interplay between p53 and ROS extends to p53-regulated metabolism. A balance between optimal p53 levels and elevated ROS can be maintained through p53-regulated antioxidant products. P53mitigates ROS by promoting antioxidant activity through the regulation of antioxidant and metabolic genes, rather than genes associated with cell-cycle and apoptosis, potentially leading to a more favorable tumor status (62,63). These observations suggest that prognosis ROS genes may regulate the onset and progression of NSCLC through various pathways. ROS has also been shown to inactivate PI3K/Akt phosphatase, thus facilitating the transduction of the PI3K/Akt signal. Beyond inducing proliferation and tumor cell survival, the PI3K/Akt signaling pathway is also implicated in chemotherapy resistance and the inhibition of cell death (64).
Numerous predictive models exist for tumor drug sensitivity for tumor drug sensitivity, yet the absence of efficacy tests curtails their clinical application. Given the high genetic congruence between organoids and their source tumor tissues, the drug sensitivity results from organoids closely align with those derived from tumor tissues (65). Therefore, it is feasible to use organoid to validate the therapeutic effect of chemotherapeutic drugs. In this study, organoid-based drug sensitivity tests confirmed that patients with low-risk scores experienced more favorable outcomes from chemotherapy, thereby validating the effectiveness of our model.
We also investigated the relationship between the prognostic ROS genes and tumor microenvironment, findings significant differences in immune infiltration cells between high- and low-risk groups, with the risk score strongly correlating with the immune score. Notably, the low-risk group appeared more responsive to immunotherapy. Given the critical role of the tumor immune microenvironment in the response to ICIs and the prognosis of NSCLC patients, we speculate that the expression of these genes may affect the tumor microenvironment, ultimately determining the immune specificity of tumors. Tumor infiltrating lymphocytes (TILs), including DCs, macrophages, natural killer cells, and B cells, are known to affect tumor progression and responses to immunotherapy (66). CD8+ T cells, recognized as primary anti-tumor immune effectors, can specifically recognize tumor-associated antigens presented by major histocompatibility complex (MHC) I and bind to tumor cells, releasing perforin and other cytotoxins that destroy cancer cells. However, CD8+ T cells that recognize antigen epitopes within tumors or the peripheral blood of the tumor microenvironment are often exhausted (67). CD4+ T cells contribute to anti-tumor immunity by assisting CD8+ T cells or by directly eliminating tumor cells as cytotoxic T cells (68). B cells are humoral immune cells that play a role in the adaptive immune system. A previous study revealed that the formation of tertiary lymphoid structures and the coexistence of CD20+ B cells and CD8+ T cells in tumors are associated with improved survival in patients with metastatic melanoma and can predict clinical outcomes in treatments involving ICIs (69). TAMs in the tumor microenvironment typically exhibit an M2 phenotype, characterized by elevated expression of anti-inflammatory cytokines, clearance receptors, angiogenic factors, and proteases, with M2-type macrophages predominating over M1-type. These anti-inflammatory cytokines foster an immunosuppressive tumor microenvironment that promotes tumor progression (70). Additionally, CAFs secrete numerous cytokines through paracrine pathways to activate tumor cells. CAFs also facilitate tumor cell invasion and metastasis by promoting extracellular matrix remodeling, angiogenesis, and immunosuppression (71).
This study acknowledges its limitations, including the inability to establish an organoid system for assessing the efficacy of immunotherapeutic drugs and the need for further investigation into the molecular mechanisms underlying our findings. Additionally, the study’s limitations are evident in the lack of validation for the proposed signature within a larger clinical NSCLC sample cohort, a shortcoming that will be addressed in future, more comprehensive research.
Conclusions
This study identified 11 ROS-related genes that are significantly associated with prognosis in NSCLC, and established a model that accurately predict the therapeutic responses to chemotherapeutic drugs. Our findings underscore the pivotal role of ROS-related genes in modulating the efficacy of drug therapy in NSCLC, suggesting potential pathways for the development of precise and personalized treatment strategies for patients with this malignant disease.
Acknowledgments
Funding: This work was supported by
Footnote
Reporting Checklist: The authors have completed the TRIPOD reporting checklist. Available at https://tlcr.amegroups.com/article/view/10.21037/tlcr-24-888/rc
Data Sharing Statement: Available at https://tlcr.amegroups.com/article/view/10.21037/tlcr-24-888/dss
Peer Review File: Available at https://tlcr.amegroups.com/article/view/10.21037/tlcr-24-888/prf
Conflicts of Interest: All authors have completed the ICMJE uniform disclosure form (available at https://tlcr.amegroups.com/article/view/10.21037/tlcr-24-888/coif). The authors have no conflicts of interest to declare.
Ethical Statement: The authors are accountable for all aspects of the work in ensuring that questions related to the accuracy or integrity of any part of the work are appropriately investigated and resolved. The study was conducted in accordance with the Declaration of Helsinki (as revised in 2013). This study was approved by the Ethics Committee of The First Affiliated Hospital of Shandong First Medical University (No. YXLL-KY-081). Informed consent was obtained from all the patients.
Open Access Statement: This is an Open Access article distributed in accordance with the Creative Commons Attribution-NonCommercial-NoDerivs 4.0 International License (CC BY-NC-ND 4.0), which permits the non-commercial replication and distribution of the article with the strict proviso that no changes or edits are made and the original work is properly cited (including links to both the formal publication through the relevant DOI and the license). See: https://creativecommons.org/licenses/by-nc-nd/4.0/.
References
- Siegel RL, Miller KD, Fuchs HE, et al. Cancer Statistics, 2021. CA Cancer J Clin 2021;71:7-33. [Crossref] [PubMed]
- Gridelli C, Rossi A, Carbone DP, et al. Non-small-cell lung cancer. Nat Rev Dis Primers 2015;1:15009. [Crossref] [PubMed]
- Kong Y, Hong L, Xu X, et al. Maintenance treatment of combination with bevacizumab vs single agent for advanced non-squamous non-small cell lung cancer: A systematic review and meta-analysis. Medicine (Baltimore) 2021;100:e26862. [Crossref] [PubMed]
- Wang S, Xie K, Liu T. Cancer Immunotherapies: From Efficacy to Resistance Mechanisms - Not Only Checkpoint Matters. Front Immunol 2021;12:690112. [Crossref] [PubMed]
- Krishna C, Tervi A, Saffern M, et al. An immunogenetic basis for lung cancer risk. Science 2024;383:eadi3808. [Crossref] [PubMed]
- Pelosi G, Pasini F. Over-Time Risk of Lung Cancer Is Largely Owing to Continuing Smoking Exposition: A Good Reason to Quit. J Thorac Oncol 2021;16:e57-9. [Crossref] [PubMed]
- Raaschou-Nielsen O, Beelen R, Wang M, et al. Particulate matter air pollution components and risk for lung cancer. Environ Int 2016;87:66-73. [Crossref] [PubMed]
- Chen KC, Tsai SW, Shie RH, et al. Indoor Air Pollution Increases the Risk of Lung Cancer. Int J Environ Res Public Health 2022;19:1164. [Crossref] [PubMed]
- Mallah MA, Changxing L, Mallah MA, et al. Polycyclic aromatic hydrocarbon and its effects on human health: An overeview. Chemosphere 2022;296:133948. [Crossref] [PubMed]
- Nigro E, Perrotta F, Monaco ML, et al. Implications of the Adiponectin System in Non-Small Cell Lung Cancer Patients: A Case-Control Study. Biomolecules 2020;10:926. [Crossref] [PubMed]
- Turner MC, Andersen ZJ, Baccarelli A, et al. Outdoor air pollution and cancer: An overview of the current evidence and public health recommendations. CA Cancer J Clin 2020;70:460-79. [Crossref] [PubMed]
- Nigro E, Stiuso P, Matera MG, et al. The anti-proliferative effects of adiponectin on human lung adenocarcinoma A549 cells and oxidative stress involvement. Pulm Pharmacol Ther 2019;55:25-30. [Crossref] [PubMed]
- Jin J, Zhang L, Li X, et al. Oxidative stress-CBP axis modulates MOB1 acetylation and activates the Hippo signaling pathway. Nucleic Acids Res 2022;50:3817-34. [Crossref] [PubMed]
- Song YS, Annalora AJ, Marcus CB, et al. Cytochrome P450 1B1: A Key Regulator of Ocular Iron Homeostasis and Oxidative Stress. Cells 2022;11:2930. [Crossref] [PubMed]
- Palackal NT, Lee SH, Harvey RG, et al. Activation of polycyclic aromatic hydrocarbon trans-dihydrodiol proximate carcinogens by human aldo-keto reductase (AKR1C) enzymes and their functional overexpression in human lung carcinoma (A549) cells. J Biol Chem 2002;277:24799-808. [Crossref] [PubMed]
- Vattanasit U, Navasumrit P, Khadka MB, et al. Oxidative DNA damage and inflammatory responses in cultured human cells and in humans exposed to traffic-related particles. Int J Hyg Environ Health 2014;217:23-33. [Crossref] [PubMed]
- Yu J, Zhong B, Zhao L, et al. Fighting drug-resistant lung cancer by induction of NAD(P)H:quinone oxidoreductase 1 (NQO1)-mediated ferroptosis. Drug Resist Updat 2023;70:100977. [Crossref] [PubMed]
- Iqbal MJ, Kabeer A, Abbas Z, et al. Interplay of oxidative stress, cellular communication and signaling pathways in cancer. Cell Commun Signal 2024;22:7. [Crossref] [PubMed]
- Jiang S, Xiao M, Shi Y, et al. Identification of m7G-Related miRNA Signatures Associated with Prognosis, Oxidative Stress, and Immune Landscape in Lung Adenocarcinoma. Biomedicines 2023;11:1569. [Crossref] [PubMed]
- Liu L, Hou Q, Chen B, et al. Identification of molecular subgroups and establishment of risk model based on the response to oxidative stress to predict overall survival of patients with lung adenocarcinoma. Eur J Med Res 2023;28:333. [Crossref] [PubMed]
- Arcangeli A. Ion channels and transporters in cancer. 3. Ion channels in the tumor cell-microenvironment cross talk. Am J Physiol Cell Physiol 2011;301:C762-71. [Crossref] [PubMed]
- Remigante A, Spinelli S, Marino A, et al. Oxidative Stress and Immune Response in Melanoma: Ion Channels as Targets of Therapy. Int J Mol Sci 2023;24:887. [Crossref] [PubMed]
- Zhao H, Huang Y, Tong G, et al. Identification of a Novel Oxidative Stress- and Anoikis-Related Prognostic Signature and Its Immune Landscape Analysis in Non-Small Cell Lung Cancer. Int J Mol Sci 2023;24:16188. [Crossref] [PubMed]
- Xu T, Liu X, Liu C, et al. Development and validation of a nomogram for predicting the overall survival in non-small cell lung cancer patients with liver metastasis. Transl Cancer Res 2023;12:3061-73. [Crossref] [PubMed]
- Zhang Z, Xie S, Cai W, et al. A nomogram to predict the recurrence-free survival and analyze the utility of chemotherapy in stage IB non-small cell lung cancer. Transl Lung Cancer Res 2022;11:75-86. [Crossref] [PubMed]
- Wang Q, Wang Y, Wang X, et al. Development and validation of a dynamic survival nomogram for metastatic non-small cell lung cancer based on the SEER database and an external validation cohort. Transl Lung Cancer Res 2022;11:1678-91. [Crossref] [PubMed]
- Sun D, Chen C, Hu W, et al. Low expression level of ASK1-interacting protein-1 correlated with tumor angiogenesis and poor survival in patients with esophageal squamous cell cancer. Onco Targets Ther 2018;11:7699-707. [Crossref] [PubMed]
- Öjlert ÅK, Halvorsen AR, Nebdal D, et al. The immune microenvironment in non-small cell lung cancer is predictive of prognosis after surgery. Mol Oncol 2019;13:1166-79. [Crossref] [PubMed]
- Akamatsu H, Toi Y, Hayashi H, et al. Efficacy of osimertinib plus bevacizumab vs osimertinib in patients with EGFR T790M-mutated non-small cell lung cancer previously treated with epidermal growth factor receptor-tyrosine kinase inhibitor: West Japan Oncology Group 8715L Phase 2 Randomized Clinical Trial. JAMA Oncol 2021;7:386-94. [Crossref] [PubMed]
- de Marinis F, Bria E, Ciardiello F, et al. International Experts Panel Meeting of the Italian Association of Thoracic Oncology on Antiangiogenetic Drugs for Non-Small Cell Lung Cancer: Realities and Hopes. J Thorac Oncol 2016;11:1153-69. [Crossref] [PubMed]
- Huang MY, Jiang XM, Wang BL, et al. Combination therapy with PD-1/PD-L1 blockade in non-small cell lung cancer: strategies and mechanisms. Pharmacol Ther 2021;219:107694. [Crossref] [PubMed]
- Zhou F, Qiao M, Zhou C. The cutting-edge progress of immune-checkpoint blockade in lung cancer. Cell Mol Immunol 2021;18:279-93. [Crossref] [PubMed]
- Yu P, Tong L, Song Y, et al. Systematic profiling of invasion-related gene signature predicts prognostic features of lung adenocarcinoma. J Cell Mol Med 2021;25:6388-402. [Crossref] [PubMed]
- Zhou T, Yang P, Tang S, et al. Classification of Lung Adenocarcinoma Based on Immune Checkpoint and Screening of Related Genes. J Oncol 2021;2021:5512325. [Crossref] [PubMed]
- Tu J, Tang M, Li G, et al. Molecular Typing Based on Oxidative Stress Genes and Establishment of Prognostic Characteristics of 7 Genes in Lung Adenocarcinoma. Oxid Med Cell Longev 2022;2022:9683819. [Crossref] [PubMed]
- Miao TW, Yang DQ, Gao LJ, et al. Construction of a redox-related gene signature for overall survival prediction and immune infiltration in non-small-cell lung cancer. Front Mol Biosci 2022;9:942402. [Crossref] [PubMed]
- Ebrahimi SO, Reiisi S, Shareef S. miRNAs, oxidative stress, and cancer: A comprehensive and updated review. J Cell Physiol 2020;235:8812-25. [Crossref] [PubMed]
- Yang JX, Chuang YC, Tseng JC, et al. Tumor promoting effect of PDLIM2 downregulation involves mitochondrial ROS, oncometabolite accumulations and HIF-1α activation. J Exp Clin Cancer Res 2024;43:169. [Crossref] [PubMed]
- Yin Y, Chen F. Targeting human MutT homolog 1 (MTH1) for cancer eradication: current progress and perspectives. Acta Pharm Sin B 2020;10:2259-71. [Crossref] [PubMed]
- Takahashi N, Chen HY, Harris IS, et al. Cancer Cells Co-opt the Neuronal Redox-Sensing Channel TRPA1 to Promote Oxidative-Stress Tolerance. Cancer Cell 2018;33:985-1003.e7. [Crossref] [PubMed]
- Zabłocka-Słowińska K, Płaczkowska S, Skórska K, et al. Oxidative stress in lung cancer patients is associated with altered serum markers of lipid metabolism. PLoS One 2019;14:e0215246. [Crossref] [PubMed]
- Kgatle MM, Spearman CW, Kalla AA, et al. DNA Oncogenic Virus-Induced Oxidative Stress, Genomic Damage, and Aberrant Epigenetic Alterations. Oxid Med Cell Longev 2017;2017:3179421. [Crossref] [PubMed]
- Srinivas US, Tan BWQ, Vellayappan BA, et al. ROS and the DNA damage response in cancer. Redox Biol 2019;25:101084. [Crossref] [PubMed]
- Wang Y, Qi H, Liu Y, et al. The double-edged roles of ROS in cancer prevention and therapy. Theranostics 2021;11:4839-57. [Crossref] [PubMed]
- Arora S, Singh P, Tabassum G, et al. miR-16-5p regulates aerobic glycolysis and tumorigenesis of NSCLC cells via LDH-A/lactate/NF-κB signaling. Life Sci 2022;304:120722. [Crossref] [PubMed]
- Tirino V, Camerlingo R, Bifulco K, et al. TGF-β1 exposure induces epithelial to mesenchymal transition both in CSCs and non-CSCs of the A549 cell line, leading to an increase of migration ability in the CD133+ A549 cell fraction. Cell Death Dis 2013;4:e620. [Crossref] [PubMed]
- Morrissey MA, Kern N, Vale RD. CD47 Ligation Repositions the Inhibitory Receptor SIRPA to Suppress Integrin Activation and Phagocytosis. Immunity 2020;53:290-302.e6. Erratum in: Immunity 2023;56:2172. [Crossref] [PubMed]
- Zhang W, Huang Q, Xiao W, et al. Advances in Anti-Tumor Treatments Targeting the CD47/SIRPα Axis. Front Immunol 2020;11:18. [Crossref] [PubMed]
- Catalán R, Orozco-Morales M, Hernández-Pedro NY, et al. CD47-SIRPα Axis as a Biomarker and Therapeutic Target in Cancer: Current Perspectives and Future Challenges in Nonsmall Cell Lung Cancer. J Immunol Res 2020;2020:9435030. [Crossref] [PubMed]
- Song X, Jiao X, Yan H, et al. Overexpression of PTPRN Promotes Metastasis of Lung Adenocarcinoma and Suppresses NK Cell Cytotoxicity. Front Cell Dev Biol 2021;9:622018. [Crossref] [PubMed]
- Berrout J, Kyriakopoulou E, Moparthi L, et al. TRPA1-FGFR2 binding event is a regulatory oncogenic driver modulated by miRNA-142-3p. Nat Commun 2017;8:947. [Crossref] [PubMed]
- Wang J, Xu M, Li DD, et al. GPR37 promotes the malignancy of lung adenocarcinoma via TGF-β/Smad pathway. Open Med (Wars) 2021;16:24-32. [Crossref] [PubMed]
- Xie X, Cai X, Zhou F, et al. GPR37 promotes cancer growth by binding to CDK6 and represents a new theranostic target in lung adenocarcinoma. Pharmacol Res 2022;183:106389. [Crossref] [PubMed]
- Li WH, Huang K, Wen FB, et al. PLOD3 regulates the expression of YAP1 to affect the progression of non-small cell lung cancer via the PKCδ/CDK1/LIMD1 signaling pathway. Lab Invest 2022;102:440-51. [Crossref] [PubMed]
- Chen S, Zhu X, Zheng J, et al. miR-30a-5p Regulates Viability, Migration, and Invasion of Lung Adenocarcinoma Cells via Targeting ECT2. Comput Math Methods Med 2021;2021:6241469. [Crossref] [PubMed]
- Saraya T, Ohkuma K, Koide T, et al. A novel diagnostic method for distinguishing parapneumonic effusion and empyema from other diseases by using the pleural lactate dehydrogenase to adenosine deaminase ratio and carcinoembryonic antigen levels. Medicine (Baltimore) 2019;98:e15003. [Crossref] [PubMed]
- Wang Y, Mei X, Song W, et al. LncRNA LINC00511 promotes COL1A1-mediated proliferation and metastasis by sponging miR-126-5p/miR-218-5p in lung adenocarcinoma. BMC Pulm Med 2022;22:272. [Crossref] [PubMed]
- Rupp M, Hagenbuchner J, Rass B, et al. FOXO3-mediated chemo-protection in high-stage neuroblastoma depends on wild-type TP53 and SESN3. Oncogene 2017;36:6190-203. [Crossref] [PubMed]
- Yeh CT, Chen TT, Satriyo PB, et al. Bruton's tyrosine kinase (BTK) mediates resistance to EGFR inhibition in non-small-cell lung carcinoma. Oncogenesis 2021;10:56. [Crossref] [PubMed]
- Bykov VJN, Eriksson SE, Bianchi J, et al. Targeting mutant p53 for efficient cancer therapy. Nat Rev Cancer 2018;18:89-102. [Crossref] [PubMed]
- Achanta G, Huang P. Role of p53 in sensing oxidative DNA damage in response to reactive oxygen species-generating agents. Cancer Res 2004;64:6233-9. [Crossref] [PubMed]
- Schieber M, Chandel NS. ROS function in redox signaling and oxidative stress. Curr Biol 2014;24:R453-62. [Crossref] [PubMed]
- Dharshini LCP, Rasmi RR, Kathirvelan C, et al. Regulatory Components of Oxidative Stress and Inflammation and Their Complex Interplay in Carcinogenesis. Appl Biochem Biotechnol 2023;195:2893-916. [Crossref] [PubMed]
- Zhang Y, Xu Y, Lu W, et al. Upregulation of Antioxidant Capacity and Nucleotide Precursor Availability Suffices for Oncogenic Transformation. Cell Metab 2021;33:94-109.e8. [Crossref] [PubMed]
- Vlachogiannis G, Hedayat S, Vatsiou A, et al. Patient-derived organoids model treatment response of metastatic gastrointestinal cancers. Science 2018;359:920-6. [Crossref] [PubMed]
- Kumar AR, Devan AR, Nair B, et al. Harnessing the immune system against cancer: current immunotherapy approaches and therapeutic targets. Mol Biol Rep 2021;48:8075-95. [Crossref] [PubMed]
- Dolina JS, Van Braeckel-Budimir N, Thomas GD, et al. CD8(+) T Cell Exhaustion in Cancer. Front Immunol 2021;12:715234. [Crossref] [PubMed]
- Borst J, Ahrends T, Bąbała N, et al. CD4(+) T cell help in cancer immunology and immunotherapy. Nat Rev Immunol 2018;18:635-47. [Crossref] [PubMed]
- Cabrita R, Lauss M, Sanna A, et al. Tertiary lymphoid structures improve immunotherapy and survival in melanoma. Nature 2020;577:561-5. Erratum in: Nature 2020;580:E1. [Crossref] [PubMed]
- Boutilier AJ, Elsawa SF. Macrophage Polarization States in the Tumor Microenvironment. Int J Mol Sci 2021;22:6995. [Crossref] [PubMed]
- Mao X, Xu J, Wang W, et al. Crosstalk between cancer-associated fibroblasts and immune cells in the tumor microenvironment: new findings and future perspectives. Mol Cancer 2021;20:131. [Crossref] [PubMed]