Does selected immunological panel possess the value of predicting the prognosis of early-stage resectable non-small cell lung cancer?
Introduction
Lung cancer is the most frequent cause of cancer death around the globe. Non-small cell lung cancer (NSCLC) is the predominant pathological type, and possesses distinct immunological status in tumor microenvironment (TME) (1,2). In NSCLC, program death-1/program death-ligand 1 (PD-1/PD-L1) blockade has become the standard for first-line therapy of metastatic tumors (3,4). Currently, the tissue PD-1/PD-L1 expression status and tumor mutation burden (TMB) are the accepted criteria indicating treatment with PD-1/PD-L1 blockade. Unfortunately, a substantial proportion (about 75%) of patients treated with PD-1/PD-L1 blockade therapy did not benefit (5), and some studies had also challenged the role of PD-1/PD-L1 in patient selection for immunological treatment and prognosis evaluation (6,7). The reason for the discrepancy could lie in the complexity of the immune environment, where the immune checkpoints expression status only represents part of the overall immune status in the TME. Further, the collective value of checkpoints and co-stimulatory receptor signals still remains vague for predicting prognosis. Therefore, a validated panel of biomarkers is essential, not only for screening patients prior to immunotherapy treatment but also for predicting the patients’ prognosis in early stage NSCLC.
The checkpoint and co-stimulatory receptor status were both valued as promising biomarkers in anti-cancer immunotherapy (8-15). Apart from PD-1/PD-L1, other immune checkpoints including Lymphocyte-activation gene-3 (LAG-3), T-cell immunoglobulin and mucin domains-containing protein-3 (TIM-3), T cell immunoglobulin and ITIM domains (TIGIT), Galectin-9 (GAL-9), V-domain immunoglobulin suppressor of T cell activation (VISTA), etc. were valued as promising biomarkers in determining the patients’ TME immune status (16,17). Studies revealed the role of LAG-3, TIM-3 and GAL-9 signaling in manipulating the T effector immune cells and predicting the survival of patients (18-20). Moreover, the co-stimulatory molecules, such as OX40 (CD134), were shown to be part of an emerging pathway to reinvigorate the attenuated immune system and fight malignant cells (21,22). In the TME, the status of some general immunological indicators, such as tumor infiltrate lymphocytes (TILs) and MHC class II expression, can also partly reflect the status of the immune cells combating the malignant cells (9,11). Taken together, these immune biomarkers lend some insights for predicting the patients’ survival and prognosis respectively, but evidence of their combinational value in predicting the patients’ prognosis is lacking. Biomarker status differs under various cancer mechanisms, therefore they only reflect a small part of the immunological status in the TME (17). In our view, by screening a panel of the appropriate biomarkers contributing to the survival and prognosis, and combining their instructive value, we may be able to draw a more extensive blueprint of the antitumoral TME. If proven, the combined biomarker results may illuminate clinical benefits to patients, including predicting prognosis, survival and identifying groups of patients who will benefit most from the immune blockade therapy.
In this study, we did a retrospective evaluation of early stage (IA, IB) NSCLC patients and conducted a panel of IHC staining on resected tumor tissue. We selected a panel of biomarkers including the immune checkpoints (PD-1, PD-L1, LAG-3, GAL-9, TIM3), co-stimulation molecules (OX40, OX40L), and TME general biomarkers (MHC Class II, TILs). The expression of OX40, OX40L, GAL-9, TIM3, and PD-L1 were validated on both TILs and tumor cells and renamed with a suffix (e.g., OX40TILs and OX40tumor). Based on the IHC data and the patients’ survival status, we were able to connect the immune status with survival and prognosis and predict the future progress of the disease. For patients in the cohort, immune status was calculated and designated a PCrank (Principal component ranking) score. The PCrank score is a reflection of the patients’ immune status as it was compounded from the 6 selected immune biomarkers’ expression conditions (continuous variables). The 6 selected immune biomarkers were each significant factor (P<0.05) that were determined by subgroup survival analysis and Cox regression analysis of overall survival (OS) and recurrent-free survival (RFS) to have the highest impact on the survival and prognosis. By using the dimensionality reduction and principal component extraction, the selected immune biomarkers can represent a wider range of the immune status in the TME and provide us with a numerical-list ranking of patients, with the higher score indicating better OS and PFS. In order to avoid bias in this study, a larger cohort of patients was also searched based on the expression status of AVCR2 (TIM3), TNFRSF4 (OX40), MHC2TA (MHCII), and LGALS9 (GAL-9) in order to validate the effect of stated biomarkers above, by using the KM plotter database. Finally, an Artificial Neuron Network (ANN) was also established to further verify the value of PCrank score from 6 selected biomarkers in predicting survival. Utilizing the analysis described above, we should be able to confirm the value of our concurrent immune biomarker panel in predicting survival and prognosis in early-stage NSCLC patients.
Methods
Patients and specimens
This study included 139 NSCLC patients with resectable lung cancer from Department of Oncology and Radiotherapy in the Medical University of Gdansk (Gdansk, Poland). The recruitment of the patients started from April 2008 to August 2010. All the patients were newly diagnosed and treatment-free before surgery. All patients participated voluntarily and provided written consent. Sample size was estimated beforehand. Approval for this study was obtained from the Shanghai Pulmonary Hospital, Tongji University (ethical number 15-235), and was in accordance with the guidelines of the Helsinki Declaration of 1975, as revised in 1983. The patients’ TMN staging was determined by seventh edition of the IASLC staging system.
IHC of tissue immunological panel (PD-1, PD-L1, LAG-3, GAL-9, TIM3, OX40, OX40L and MHC class II)
All the tissue specimen IHC was conducted on the Ventana Benchmark XT platform (Ventana Medical Systems, Tucson, AZ), except PD-L1 IHC staining, which was performed on the Dako platform (Dako, Carpenteria, CA). All tissue samples were embedded in paraffin, and all the sections were pretreated by baking in drying oven at 60 °C for 1 hour. Primary antibodies [MHC Class II DP DQ DR, CR3/43, 1:100, Abcam; OX40 and OX40L, 1:1,000, EPR4392 (Abcam, Cambridge, MA); PD-1, predilute, NAT 105 (Cell Marque, Rocklin, CA); PD-L1, 22C3 (Dako); LAG-3, GAL-9, TIM3, 1:1000, EPR4392 (Abcam, Cambridge, MA); PD-1, predilute, NAT 105 (Cell Marque, Rocklin, CA)] were applied according to manufacturers’ specifications. Slides were visualized using an UltraView DAB detection and amplification kit (Ventana Medical Systems). The sample handling and staining procedures are in accordance with operating manuals and our previous published articles (9,23-25).
Validating the cutoff value for each marker within the immunological panel
All the pathology and IHC results were checked and reviewed independently by two qualified pathologists. The cutoff values chosen best predicted both RFS and OS. We validated this method in our previous studies (9,23-25). The cutoff value for MHCIItumor DP DQ DR was more than 30% staining, and MHCIITILs was more than 80% staining. The cutoff value for LAG-3, OX40tumor/TILs, OX40L tumor/TILs, GAL-9 tumor/TILs, TIM3 tumor/TILs were chosen as the value of more than 20% staining. The cutoff value for PD-1was in line with former studies (at least 8% staining). The cutoff value for PD-L1 on tumor cells was at least 50% staining, as approved by the U.S. Food and Drug Administration.
The evaluation of the abundance of TILs
The massive lymphocyte value in the TME was calculated and validated by two independent pathologists. Any discordance between two pathologists was jointly reviewed and a consensus value was established. The infiltration status of the lymphocytes was calculated by a semi-quantitative grade from 1+ (+) to 3+ (+++), which indicates low (<30%), moderate (30–60%) and high (>60%) percentages of TILs. The TILs were composed of several types of mononuclear lymphocytes, including the lymphocytes, macrophages and plasma cells. Intra-alveolar macrophages were not considered as part of the immune infiltration.
Principle components analysis (PCA) for the selected biomarker expression
The PCA was conducted to control for the selected biomarkers of continuous variables. The data standardization was performed before PCA. Kaiser-Meyer-Olkin (KMO) Bartlett’s Sphericity test was conducted to verify the feasibility of the data for use in the PCA, in which the value >0.5 and P<0.05 was considered suitable for the PCA. The initial whole factors were fully analyzed, and the extracted factors were selected concerning the percentage of variance and total feature generalization. The calculation of extracted principle (zn) components is according to the following formula.
(Xε: Variables for PCA; ε: The number of extracted variables; an= Extracted factors; λ: load coefficient)
After the principle components were calculated, the PCA regression was conducted in order to verify the correlation between extracted components and OS, RFS.
The establishment of immune score for the prediction of OS and RFS (PCrank)
The subgroup and COX regression analyses were conducted previously in order to find the biomarkers which might have significant effect in predicting the survival and prognosis. A P value <0.05 was considered significant. Factorial analysis was conducted to validate the independence of factors. Then, we performed dimensionality reduction and extracted the principal component of selected immune biomarkers, which was followed by PCA and Principal Component ranking (PCrank). The calculation of PCrank is based on the formula below.
The PCrank value was initially calculated as a normalized variable based on principle components. And they were transformed into a percentile based on the method of T grade score. The PCrank score is a visual variable that can directly reflect the immune status of each patient in numerical order, and is used as a survival predictor for each individual. After the determination of the cutoff values (0.2 and 0.25), the PCrank will be used to sort the patients into two groups, high PCrank group (subgrouphigh) and low PCrank group (subgrouplow), with different survival outcomes and prognosis.
The validation of the predictive value of selected biomarkers in the database
Based on the previous results, a larger cohort of patients was searched based on the expression status of selected biomarkers AVCR2 (TIM3), lymphocyte activating 3(LAG-3), TNFRSF4 (OX40), MHC2TA (MHCII), and LGALS9 (GAL-9) in order to validate the effect of predictive value, by using the KM plotter database (26). We used a larger sample size cohort to verify the results and avoid bias.
The establishment of the ANN model to utilize the prediction of survival
An ANN model was established to further verify the efficacy of select biomarkers and PCrank in predicting survival. The ANN model with Multilayer Perceptron consists of three parts, an input layer (with 5 dependent variables and 60 units included), one hidden layer and an output layer with 2 dimensions. All the samples were randomly divided into training and test groups with ratios of approximately 2:1 to 3:1, respectively. The activation function and error function of hidden layer and output layer were selected to avoid model overfitting. Two different results were presented by using the same model to test the efficiency of two PCranks with 2 different cutoff values, 0.2 and 0.25. The validation ROC curve of this model was also verified.
The data processing in this study was performed using SPSS 23.0 (SPSS, Inc., Chicago, IL). All statistics were two sided, and statistical significance was defined as P less than 0.05.
Results
Patients’ characteristics
A cohort of 139 patients were deemed eligible and recruited into the study. The median patient age was 64 years, and there were 105 patients (75.5%) younger than 70 years and 34 patients (24.5%) over 70 years old. Ninety-three patients were stage 1A (66.9%) and 46 patients were stage 1B (33.1%). See Table 1 for other patient characteristics.
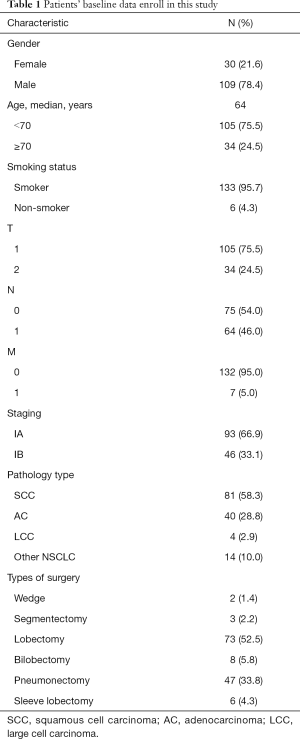
Full table
The expression status of the immune biomarker panel in the specimens
IHC and pathological results revealed the expression status of each biomarker. The expression status of OX40, OX40L, GAL-9, TIM3 and PD-L1 were identified on both TILs and tumor cells. The expression statuses of LAG-3, PD-1 and TILs were identified on TILs. The additional related expression statuses and ratios are presented in Table 2.
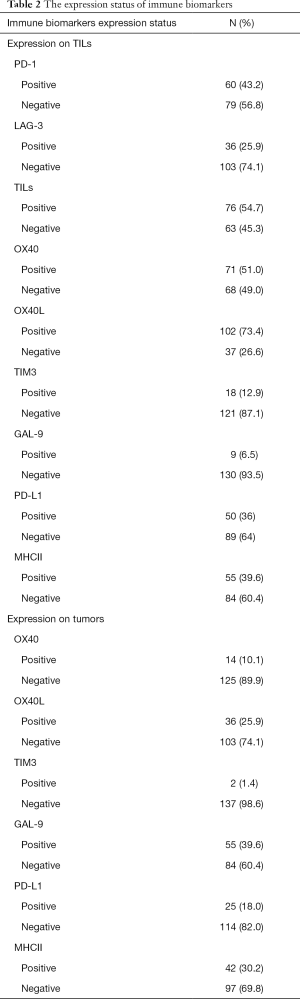
Full table
Subgroup analysis for the identification of significant biomarkers
The immune panel was evaluated for significant biomarkers which have a major impact on patient survival and prognosis. TIM3TILs expression and GAL-9LTILs expression were found to be factors that differentiate RFS, with a P value of 0.022 and 0.036 respectively. GAL-9tumor and OX40Ltumors expression were identified as factors that affect OS, with P values of 0.039 and 0.027 respectively. The LAG-3 and MHCIITILs expression statuses were validated as factors that both affect OS and RFS (the P values for OS and RFS were 0.039 and 0.025/0.028 and 0.014 respectively). The significant biomarkers were active immune factors that can direct the treatment of patients and predict their prognosis (Table 3).
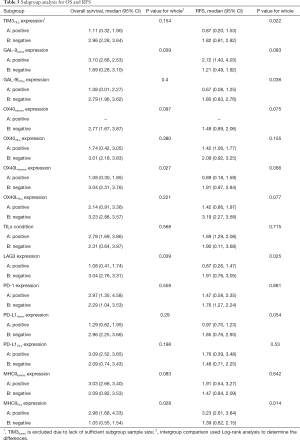
Full table
Cox regression analysis for OS and RFS
The Cox analysis results indicated that GAL-9tumor expression (RR 0.523, 95% CI: 0.336–0.814, P=0.004) and MHCIItumors expression (RR 0.474, 95% CI: 0.303–0.740, P=0.001) were protective factors for survival. The LAG3 expression (RR 1.956, 95% CI: 1.237–3.092, P=0.004) was a risk factor against survival. GAL-9tumor expression (RR 0.529, 95% CI: 0.338–0.829, P=0.005), MHCIItumors expression (RR 0.509, 95% CI: 0.327–0.791, P=0.003) were protective factors for RFS. GAL-9TILs expression (RR 2.551, 95% CI: 1.177–5.532, P=0.018) and LAG3 expression (RR 1.862, 95% CI: 1.172–2.959, P=0.008) were the risk factors against RFS (Table 4).
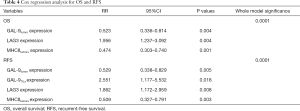
Full table
Principle components extraction and factorial analysis
Factors with continuous variables were selected for factorial analysis. The selected factors were TIM3TILs, GAL-9tumor, GAL-9LTILs, OX40Ltumors, LAG3 and MHCIITILs based on the results above. 6 factors were initially analyzed, and 6 independent initial factors were created. By utilizing the Variance maximization rotation method, 6 significant initial factors were generated in different dimensions, indicating the independence among the factors and the necessity of debasing the dimension of data (Table 5). The results of factorial analysis indicated the necessity of principle extraction. Before the principle components extraction, the Kaiser-Meyer-Olkin Bartlett’s Sphericity test (test value =0.658>0.5, P=0.0001) verified the feasibility of principle extraction. Among the 6 initial factors, 4 factors features were extracted, which can explain the 78.795% of the total feature of the original factors, indicating the final principle components were a 4-dimensional data set (Table 5). The principle component regression results indicate the OS is correlated with the principle component Z4(Y=0.316×Z4+2.298, R=0.189, R2=0.036, P=0.026, Durbin-Watson=1.913). Also, PFS is correlated with the principle component Z4 and Z2 (Y=0.314×Z4+0.255×Z2+2.061, R=0.249, R2=0.062, P=0.013, Durbin-Watson =2.057).
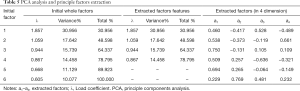
Full table
PCrank and related subgroup analysis
The PCrank was calculated and transformed into a percentile in order to create a sequential list of patients with different immune conditions. Cut-off values of 0.2 and 0.25 were selected in order to best differentiate the subgroups of patients. When the cutoff value of 0.2 was chosen, the intergroup comparison of subgrouphigh (median, 3.01 years) and subgrouplow (median, 0.89 years) was significant for OS (P=0.025). However, the RFS was not significant. When the cutoff value of 0.25 was chosen, the intergroup comparison was not statistically significant for OS and RFS. However, a clear intergroup differential tendency was shown as subgrouphigh had longer OS (median, 3.01 years) than the subgrouplow (median, 1.39 years). The PCrank results revealed that it is a potential prognostic tool for survival in early-stage resectable NSCLC patients (Table 6, Figure 1).
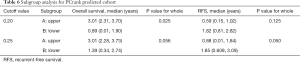
Full table
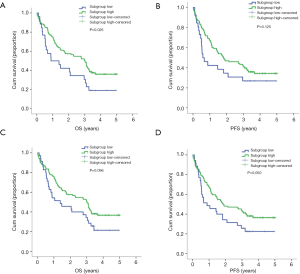
Validation of the predictive value of selected biomarkers
mRNA expression levels of 5 selected genes [AVCR2 (TIM3), lymphocyte activating 3 (LAG-3), TNFRSF4 (OX40), MHC2TA (MHCII), and LGALS9 (GAL-9)], based on previous NSCLC cohorts, were searched in the KM Plotter database. A high- and low- expression inter-cohort comparison was conducted, and all the comparisons were statistically significant (P<0.05). Higher expression for TIM3, LAG-3, OX40, and GAL-9 suggested poor survival. However, higher MHCII expression was correlated with better survival (Figure 2). The results from the database have verified our selection of these biomarkers to predict survival and prognosis.
The establishment of ANN model and its prediction of survival
The dependent variable is the survival status (survival or death), which is in accordance with the PCrank and related subgroup analysis. GAL-9 tumor expression, GAL-9L TILs expression, OX40Ltumors expression, LAG3 expression and MHCII TILs expression were the input variables. Two models’ results were demonstrated where the cutoff values were selected as 0.2 or 0.25. In model 1, the training dataset comprised 100 case (74.6%) and test dataset included 34 (25.4%). 5 invalid cases were excluded due to inadequate biomarker expression status. The total prediction accuracy for training was 94% and 94.1% for testing. The areas under the ROC curve is 0.978 for subgrouplow (death) and subgrouphigh (survival). In model 2, the training dataset included 83 patients (61.5%) and test dataset comprised 52(38.5%). The total prediction accuracy for training was 100% and 96.2% for testing. The areas under the ROC curve are 0.998 for subgrouplow (death) and subgrouphigh (survive). The results indicated that the immune biomarker panel and its related PCrank are valid and efficient predictors of patients’ outcome (Table 7).
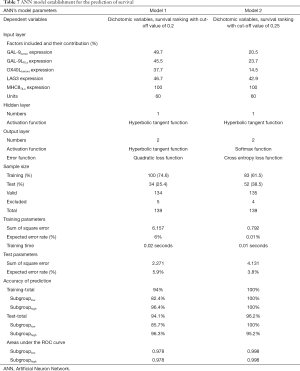
Full table
Discussion
Lung cancer is one of the most deadly cancer types around the world. The early detection of relevant clinicopathological features of a tumor is closely correlated with clinical outcome. Therefore, as we enter the age of immunotherapy, the systematic detection of immune status and related immune conditions in the TME are critical (27).
In this study, we assembled an IHC-based immune biomarker panel as a new method to detect the immune status of the TME and provide new insights for treatment-naïve early-stage NSCLC patients’ prognosis. This immune biomarker panel consists of immune checkpoints, co-stimulatory molecules and general TME biomarkers. We selected such a wide range of biomarkers with different mechanisms in recognition of the fact that immune status is a network-like complex that needs to be analyzed in multiple dimensions. By using principle component analysis, the data characteristics of biomarker expression status can be easily analyzed, and the concurrent use of immune biomarkers can inform us more fully about the patients’ active immune status. The PCrank indicator is a reflection of patients’ immune status and predicts survival and prognosis. In order to eliminate bias as much as possible, we conducted a database search to verify biomarker-related survival in larger sample cohorts. Finally, the ANN model uses the PCrank indicator to predict the patients’ clinical outcome, which reinforces the validity of the indicator.
In this research, the selected factors were TIM3TILs, GAL-9tumor, GAL-9LTILs, OX40Ltumors, LAG3 and MHCIITILs, which can represent the immune wholeness of the TME. In general, the TIM-3, GAL-9/GAL-9L and LAG-3 are the immune inhibitory pathway molecules which may restrain the effectiveness of the anti-tumoral effect of the T cell. On the contrary, the OX40 and MHCII represent the vitality of the whole environment. To combine the effect of biomarkers from both pathways, the contribution of variance by PCA can represent the actual TME immune status from different biomarkers with different mechanisms. Therefore, the PCrank indicators calculated for early-staged NSCLC patients can lend us with more perspectives about the immune status of patients and may guide healthcare providers in evaluating patients for precision treatment. It is possible that when the disease is in advanced stage, certain antibodies that targeted the biomarkers stated above could be implemented in future treatment scenario.
To our knowledge, based on an extensive literature search, this is the first study to use a concurrent immune biomarker panel and biomarker expression datamining in IHC-based clinical samples to obtain a PCrank indicator ranking that reflects the active immune status of early-stage NSCLC patients. Some researchers have made progress in mining immune biomarker profiling data for the purpose of estimating the prognosis or screening suitable patients for checkpoint blockade therapy. Mezquita et al. suggested that an immune prognostic index could be effective in predicting the prognosis of NSCLC patient with checkpoint blockade treatment (28). The index was designed based on the neutrophils/(leukocytes-neutrophils) ratio and lactate dehydrogenase level, which was previously demonstrated effective in predicting clinical outcome. In another study, the authors assessed the metastatic immune landscape in colorectal cancer patients and its impact on the treatment responses (29). The immune densities (cells/mm2) were recorded and used to calculate the CD3/CD8, CD8/CD20 immunoscore and T cell & B cell immunoscores (TBscore). The authors found that patients with high TBscore and immunoscore had a longer median survival than the low-score group. Meanwhile, the treatment non-responding patients displayed higher levels of immune infiltrates and prolonged disease-free survival. These studies indicated the importance of combined immune biomarkers in predicting prognosis and survival. Nonetheless, some bias and weaknesses still existed in these studies, and they need to be conducted in large sample size cohorts with various types of cancer. Further, some investigators have proposed using immune-related genes to estimate prognosis for early-stage NSCLC patients (30). By combing multiple gene expression data sets, they developed an individualized prognostic signature based on the immune-related gene pairs, which significantly stratifies the patients into low- and high-risk groups with different survival conditions. With the development of algorithm and computing science, machine learning has gradually become a promising method for predicting clinical outcome based on acquired immune gene expression. A study was performed to capture the cancer-immune set point based on nCounter RNA expression data from the archival biopsy samples of stage IV NSCLC (31). Using the established machine learning model, they identified a subset of approximately 20 (out of 770) genes that were associated with clinical outcomes in the training group (n=55). Surprisingly, this method successfully identified the top responders and identified the non-responding patients. As in our study, the application of a machine learning model potentiated the usefulness of acquired data and enabled the possibility of clinical application. In short, studies related to the profiling of immune biomarkers or immune-related genes for predicting patient prognosis are progressing. However, large scale prospective studies are still needed to find the best-match immune biomarkers for NSCLC or other cancer types, resulting in a more comprehensive biomarker panel capable of yielding conclusive guidance for patient treatment.
Ongoing study of checkpoint molecules has revealed some with promising status for anti-tumoral immunity, such as LAG-3, GAL-9, TIM-3, etc. Previous studies have revealed the role of LAG-3 in downregulating anticancer effects by re-modulating the cells in the TME (32-34). The upregulation of LAG-3 on the surface of CD4+T cells indicates chronic cell exhaustion and leads to downstream signaling, inhibiting the T cell from entering the proliferation phase of the cell cycle (35). The blockade of LAG-3 on the CD4+ T cell can reinvigorate the exhausted immune cells, contributing to the potentiation of anti-tumoral immunity (36). Studies reveal that LAG-3 is overexpressed on the tumor infiltrating CD8+T cells, which may contribute to the exhaustion and inactivation of T effector cells (37,38). The interaction between LAG-3 and PD-1 and the independence of LAG-3 inhibition also aroused interest recently. Wang et al. reported the discovery of the Fibrinogen-like Protein 1 (FGL1), which was the major and independent ligand of LAG-3 (39). This finding opens up the potential for futureanti-LAG-3 immunotherapy and creates a more concise strategy for the LAG blockade. Tim-3 is also a rising immune checkpoint expressed on a larger variety of immune cells, including the T effectors, Treg and innate immune cells (40,41). GAL-9 is one of its four ligands, and the binding of TIM-3 and GAL-9 inhibits the Th1 immune response (42). TIM-3 can inhibit T cell function and cause cell exhaustion. Its expression can cause down-regulation of some cytokines including IFN-γ and IL-2 (43-45). The expression of TIM-3 on innate cells can also downregulate T cells and suppress their proliferation. Preclinical studies targeting TIM-3 have shown anti-TIM-3 and PD-1 blockade produce similar anti-tumoral effects (46). Given that anti-PD-1 antibodies can induce incremental expression of TIM-3, it is possible that the mechanisms of PD-1 and TIM-3 may be correlated (47). The investigation of LAG-3, GAL-9, and TIM-3 as potential biomarkers will hopefully give us more insight in diagnosis and treatment evaluation. Clinical studies are ongoing: LAG-3 and TIM-3 blockades as new targets of immune checkpoint inhibitors may demonstrate promising results in the era of immunotherapy.
There are limitations to our study. Although we tried to avoid bias as much as possible in the design of this study, selection and survival bias could have occurred. The retrospective nature of the study which neglected some clinical traits and phenomenon may have missed some latent patterns. Also, the limited sample size of 139 patients did not demonstrate statistically significant (P<0.05) intergroup comparison, but a clear tendency was observed. A consistently controlled study with larger cohorts would remedy this weakness. Secondly, this study lacked data on certain clinicopathological features. We did not have the driver gene mutation status for every patient, which could have provided important insights. Meanwhile, it is essential to follow-up with study of any subsequent immune system alteration that may occur. Finally, we lacked related cell and molecular experiments. We did not have the resources to establish cell lines from resected samples. Doing so would provide verification and give us more insights into the underlying signaling mechanisms.
Conclusions
In conclusion, this study has introduced a new method utilizing multiple IHC-based biomarkers for evaluating patients’ immune status. Six biomarkers (TIM3TILs, GAL-9tumor, GAL-9LTILs, OX40Ltumors, LAG3 and MHCIITILs) were selected and determined to be biomarkers that have significant influence in the TME. Future studies should investigate the underlying mechanisms of immune biomarker panels’ use in predicting clinical outcome and focus on the selection of a suitable biomarker panel verified in multi-cancer type and large sample size clinical settings. In short, immune biomarker panels as a means of predicting clinical outcome may be a powerful tool, and further study in multiple cancer types with large sample size in a clinical setting is warranted.
Acknowledgments
Funding: This study was supported in part by a grant from National Natural Science Foundation of China (81802255), Shanghai Pujiang Program (17PJD036) and a grant from Shanghai Municipal Commission of Health and Family Planning Program (20174Y0131), National key research & development project (2016YFC0902300), Major disease clinical skills enhancement program of three year action plan for promoting clinical skills and clinical innovation in municipal hospitals, Shanghai Shen Kang Hospital Development Center Clinical Research Plan of SHDC (16CR1001A), “Dream Tutor” Outstanding Young Talents Program (fkyq1901), key disciplines of Shanghai Pulmonary Hospital (2017ZZ02012), grant of Shanghai Science and Technology Commission (16JC1405900). The fundamental research funds for the central universities.
Footnote
Conflicts of Interest: The authors have no conflicts of interest to declare.
Ethical Statement: Approval for this study was obtained from the Shanghai Pulmonary Hospital, Tongji University (ethical number 15-235), and was in accordance with the guidelines of the Helsinki Declaration of 1975, as revised in 1983. The authors are accountable for all aspects of the work in ensuring that questions related to the accuracy or integrity of any part of the work are appropriately investigated and resolved.
References
- Hirsch FR, Scagliotti GV, Mulshine JL, et al. Lung cancer: current therapies and new targeted treatments. Lancet 2017;389:299-311. [Crossref] [PubMed]
- Siegel RL, Miller KD, Jemal A. Cancer statistics, 2018. CA Cancer J Clin 2018;68:7-30. [Crossref] [PubMed]
- Champiat S, Ferrara R, Massard C, et al. Hyperprogressive disease: recognizing a novel pattern to improve patient management. Nat Rev Clin Oncol 2018;15:748-62. [Crossref] [PubMed]
- Horn L, Mansfield AS, Szczesna A, et al. First-Line Atezolizumab plus Chemotherapy in Extensive-Stage Small-Cell Lung Cancer. N Engl J Med 2018;379:2220-9. [Crossref] [PubMed]
- Dempke WCM, Fenchel K, Uciechowski P, et al. Second- and third-generation drugs for immuno-oncology treatment-The more the better? Eur J Cancer 2017;74:55-72. [Crossref] [PubMed]
- Kelly PN. The Cancer Immunotherapy Revolution. Science 2018;359:1344-5. [Crossref] [PubMed]
- Soria JC, Marabelle A, Brahmer JR, et al. Immune checkpoint modulation for non-small cell lung cancer. Clin Cancer Res 2015;21:2256-62. [Crossref] [PubMed]
- Patil NS, Righi L, Koeppen H, et al. Molecular and Histopathological Characterization of the Tumor Immune Microenvironment in Advanced Stage of Malignant Pleural Mesothelioma. J Thorac Oncol 2018;13:124-33. [Crossref] [PubMed]
- He Y, Rozeboom L, Rivard CJ, et al. MHC class II expression in lung cancer. Lung Cancer 2017;112:75-80. [Crossref] [PubMed]
- Ma QY, Huang DY, Zhang HJ, et al. Function and regulation of LAG3 on CD4(+)CD25(-) T cells in non-small cell lung cancer. Exp Cell Res 2017;360:358-64. [Crossref] [PubMed]
- Cai W, Zhou D, Wu W, et al. MHC class II restricted neoantigen peptides predicted by clonal mutation analysis in lung adenocarcinoma patients: implications on prognostic immunological biomarker and vaccine design. BMC Genomics 2018;19:582. [Crossref] [PubMed]
- Charrier M, Mezquita L, Lueza B, et al. Circulating innate immune markers and outcomes in treatment-naive advanced non-small cell lung cancer patients. Eur J Cancer 2019;108:88-96. [Crossref] [PubMed]
- Jiang T, Qiao M, Zhao C, et al. Pretreatment neutrophil-to-lymphocyte ratio is associated with outcome of advanced-stage cancer patients treated with immunotherapy: a meta-analysis. Cancer Immunol Immunother 2018;67:713-27. [Crossref] [PubMed]
- Nakaya A, Kurata T, Yoshioka H, et al. Neutrophil-to-lymphocyte ratio as an early marker of outcomes in patients with advanced non-small-cell lung cancer treated with nivolumab. Int J Clin Oncol 2018;23:634-40. [Crossref] [PubMed]
- Morrison BJ, Steel JC, Morris JC. Reduction of MHC-I expression limits T-lymphocyte-mediated killing of Cancer-initiating cells. BMC Cancer 2018;18:469. [Crossref] [PubMed]
- Granier C, De Guillebon E, Blanc C, et al. Mechanisms of action and rationale for the use of checkpoint inhibitors in cancer. ESMO Open 2017;2:e000213. [Crossref] [PubMed]
- Gorris MAJ, Halilovic A, Rabold K, et al. Eight-Color Multiplex Immunohistochemistry for Simultaneous Detection of Multiple Immune Checkpoint Molecules within the Tumor Microenvironment. J Immunol 2018;200:347-54. [Crossref] [PubMed]
- Burugu S, Gao D, Leung S, et al. LAG-3+ tumor infiltrating lymphocytes in breast cancer: clinical correlates and association with PD-1/PD-L1+ tumors. Ann Oncol 2017;28:2977-84. [Crossref] [PubMed]
- Franchini DM, Lanvin O, Tosolini M, et al. Microtubule-Driven Stress Granule Dynamics Regulate Inhibitory Immune Checkpoint Expression in T Cells. Cell Rep 2019;26:94-107.e7. [Crossref] [PubMed]
- Mochizuki K, Kawana S, Yamada S, et al. Various checkpoint molecules, and tumor-infiltrating lymphocytes in common pediatric solid tumors: Possibilities for novel immunotherapy. Pediatr Hematol Oncol 2019;36:17-27. [Crossref] [PubMed]
- Ruby CE, Redmond WL, Haley D, et al. Anti-OX40 stimulation in vivo enhances CD8+ memory T cell survival and significantly increases recall responses. Eur J Immunol 2007;37:157-66. [Crossref] [PubMed]
- Babic M, Romagnani C. Boosting Type 2 Immunity: When OX40L Comes from ILC2s. Immunity 2018;48:1067-9. [Crossref] [PubMed]
- He Y, Bunn PA, Zhou C, et al. KIR 2D (L1, L3, L4, S4) and KIR 3DL1 protein expression in non-small cell lung cancer. Oncotarget 2016;7:82104-11. [Crossref] [PubMed]
- He Y, Rozeboom L, Rivard CJ, et al. PD-1, PD-L1 Protein Expression in Non-Small Cell Lung Cancer and Their Relationship with Tumor-Infiltrating Lymphocytes. Med Sci Monit 2017;23:1208-16. [Crossref] [PubMed]
- He Y, Yu H, Rozeboom L, et al. LAG-3 Protein Expression in Non-Small Cell Lung Cancer and Its Relationship with PD-1/PD-L1 and Tumor-Infiltrating Lymphocytes. J Thorac Oncol 2017;12:814-23. [Crossref] [PubMed]
- Nagy Á, Lanczky A, Menyhart O, et al. Validation of miRNA prognostic power in hepatocellular carcinoma using expression data of independent datasets. Sci Rep 2018;8:9227. [Crossref] [PubMed]
- Postow MA, Callahan MK, Wolchok JD. Immune Checkpoint Blockade in Cancer Therapy. J Clin Oncol 2015;33:1974-82. [Crossref] [PubMed]
- Mezquita L, Auclin E, Ferrara R, et al. Association of the Lung Immune Prognostic Index With Immune Checkpoint Inhibitor Outcomes in Patients With Advanced Non-Small Cell Lung Cancer. JAMA Oncol 2018;4:351-7. [Crossref] [PubMed]
- Mlecnik B, Van den Eynde M, Bindea G, et al. Comprehensive Intrametastatic Immune Quantification and Major Impact of Immunoscore on Survival. J Natl Cancer Inst 2018. [Crossref] [PubMed]
- Li B, Cui Y, Diehn M, et al. Development and Validation of an Individualized Immune Prognostic Signature in Early-Stage Nonsquamous Non-Small Cell Lung Cancer. JAMA Oncol 2017;3:1529-37. [Crossref] [PubMed]
- Wiesweg M, Mairinger F, Reis H, et al. Machine learning-based predictors for immune checkpoint inhibitor therapy of non-small cell lung cancer. Ann Oncol 2019;30:655-7. [Crossref] [PubMed]
- Wei T, Zhang J, Qin Y, et al. Increased expression of immunosuppressive molecules on intratumoral and circulating regulatory T cells in non-small-cell lung cancer patients. Am J Cancer Res 2015;5:2190-201. [PubMed]
- Joyce JA, Fearon DT. T cell exclusion, immune privilege, and the tumor microenvironment. Science 2015;348:74-80. [Crossref] [PubMed]
- Pedersen M, Westergaard MCW, Milne K, et al. Adoptive cell therapy with tumor-infiltrating lymphocytes in patients with metastatic ovarian cancer: a pilot study. Oncoimmunology 2018;7:e1502905. [Crossref] [PubMed]
- Workman CJ, Dugger KJ, Vignali DA. Cutting edge: molecular analysis of the negative regulatory function of lymphocyte activation gene-3. J Immunol 2002;169:5392-5. [Crossref] [PubMed]
- Goding SR, Wilson KA, Xie Y, et al. Restoring immune function of tumor-specific CD4+ T cells during recurrence of melanoma. J Immunol 2013;190:4899-909. [Crossref] [PubMed]
- Hemon P, Jean-Louis F, Ramgolam K, et al. MHC class II engagement by its ligand LAG-3 (CD223) contributes to melanoma resistance to apoptosis. J Immunol 2011;186:5173-83. [Crossref] [PubMed]
- Matsuzaki J, Gnjatic S, Mhawech-Fauceglia P, et al. Tumor-infiltrating NY-ESO-1-specific CD8+ T cells are negatively regulated by LAG-3 and PD-1 in human ovarian cancer. Proc Natl Acad Sci U S A 2010;107:7875-80. [Crossref] [PubMed]
- Wang J, Sanmamed MF, Datar I, et al. Fibrinogen-like Protein 1 Is a Major Immune Inhibitory Ligand of LAG-3. Cell 2019;176:334-347.e12. [Crossref] [PubMed]
- Monney L, Sabatos CA, Gaglia JL, et al. Th1-specific cell surface protein Tim-3 regulates macrophage activation and severity of an autoimmune disease. Nature 2002;415:536-41. [Crossref] [PubMed]
- Phong BL, Avery L, Sumpter TL, et al. Tim-3 enhances FcepsilonRI-proximal signaling to modulate mast cell activation. J Exp Med 2015;212:2289-304. [Crossref] [PubMed]
- Cao E, Zang X, Ramagopal UA, et al. T cell immunoglobulin mucin-3 crystal structure reveals a galectin-9-independent ligand-binding surface. Immunity 2007;26:311-21. [Crossref] [PubMed]
- Gonçalves Silva I, Yasinska IM, Sakhnevych SS, et al. The Tim-3-galectin-9 Secretory Pathway is Involved in the Immune Escape of Human Acute Myeloid Leukemia Cells. EBioMedicine 2017;22:44-57. [Crossref] [PubMed]
- Sakuishi K, Apetoh L, Sullivan JM, et al. Targeting Tim-3 and PD-1 pathways to reverse T cell exhaustion and restore anti-tumor immunity. J Exp Med 2010;207:2187-94. [Crossref] [PubMed]
- Cai C, Xu YF, Wu ZJ, et al. Tim-3 expression represents dysfunctional tumor infiltrating T cells in renal cell carcinoma. World J Urol 2016;34:561-7. [Crossref] [PubMed]
- Ngiow SF, von Scheidt B, Akiba H, et al. Anti-TIM3 antibody promotes T cell IFN-gamma-mediated antitumor immunity and suppresses established tumors. Cancer Res 2011;71:3540-51. [Crossref] [PubMed]
- Koyama S, Akbay EA, Li YY, et al. Adaptive resistance to therapeutic PD-1 blockade is associated with upregulation of alternative immune checkpoints. Nat Commun 2016;7:10501. [Crossref] [PubMed]