Comparison of Veterans Affairs, Mayo, Brock classification models and radiologist diagnosis for classifying the malignancy of pulmonary nodules in Chinese clinical population
Introduction
Due to the widespread use of chest computed tomography (CT) and low-dose CT (LDCT), pulmonary nodules are encountered with increased frequency (1,2). This has created a great challenge to clinicians in discriminating malignant nodules from benign nodules. Several mathematical classification models based on clinical information and radiological characteristics have been developed to help clinicians to predict the malignancy probability of pulmonary nodules. The Mayo Clinic model was developed on the basis of a clinically relevant subset of patients in 1997 (3). The Veterans Affairs (VA) model that was designed in 2007 utilized data from the Department of Veterans Affairs administrative databases (4). The Brock model (PanCan model) was developed based on the Pan-Canadian Early Detection of Lung Cancer Study and validated using the British Columbia Cancer Agency (BCCA) cohort in 2013 (5). These models have demonstrated high predictive discrimination in independent cohorts (6-12).
Before choosing a classification model for pulmonary nodules, radiologists should think about the epidemiology of lung cancer in their region of the world. The above-mentioned classification models have been developed in Western populations. It is for example well known that sub-solid nodules are more frequent in non-smoking patients and women in Asian populations (13,14). These sub-solid nodules are often subsequently diagnosed as adenocarcinoma. In addition, the Brock model was derived from a large lung cancer screening trial, in which small and benign nodules are frequent findings. The high diagnostic performance of the classification models in screening cohorts may not be automatically extrapolated to a clinical population, in which larger nodules are more frequently found (15). Furthermore, the question whether the models are better compared to radiologist evaluation remains, since these models have not been compared to radiologist diagnosis. In contrast to mathematical classification models, in China, radiologists make a subjective judgment about the probability of malignancy of nodules in a CT report based on the qualitative evaluation of radiological characteristics and clinical information. Usually, the radiologist evaluation reflects the radiologist’s ability to summarize nodule information and judge the malignancy probability based on their work experience.
This study aimed to compare the diagnostic performance of radiologist diagnosis and three classification models in assessing the probability of malignancy of pulmonary nodules in a clinical setting population at a tertiary referral cancer center in China.
Methods
Study population
We conducted this retrospective study at Tianjin Medical University Cancer Institute and Hospital (TJMUCH). The institutional review board waived the need for informed consent because of its retrospective design and the use of anonymized data.
We systematically collected all newly detected lung nodules in Tianjin Medical University Cancer Institute and Hospital between January 2013 and December 2013. The inclusion criteria were: (I) newly detected pulmonary nodules on CT scans in 2013; (II) CT-derived nodule diameter between 4 and 25 mm; (III) nodules had confirmation of diagnosis (benign or malignant) based on histopathological result, or were considered benign when they either disappeared or reduced in size by at least 30% or remained stable for at least 2 years in CT follow-up; (IV) the patient’s clinical information needed to be complete. The exclusion criteria were: (I) patients with suspected or proven metastatic disease on chest CT, (II) nodules with unconfirmed diagnosis (for instance no, or too short follow-up period or no pathology), and (III) patients without clinical information.
Information on patients, nodules, and clinical characteristics was collected from the hospital information system. General patient characteristics included (I) gender; (II) age; (III) smoking history (smoking years, quit year); (IV) cancer history; and (V) family history of cancer. Nodule characteristics comprised (I) location (upper lobe, middle lobe, lower lobe); (II) size (average value of largest long diameter and short diameter measured in lung window); (III) margin (smooth, spiculated); (IV) nodule type (non-solid, part-solid, solid); and (V) density (attenuation value of the largest region of interest). Clinical characteristics included (I) clinical diagnostic examination (CT, PET-CT, and biopsy) and (II) histology results. All CT nodule characteristics were collected from the CT reports. The images were displayed using both mediastinal window setting (width, 350 HU; level, 40 HU) and lung window setting (width, 1,500 HU; level, −600 HU).
Radiologist diagnosis
To reflect real clinical practice, we used the CT diagnostic report to represent the diagnostic performance of radiologist evaluation. Each CT diagnostic report was evaluated by two radiologists (one junior, one senior). The final results were determined by consensus of the two radiologists in case of disagreement, and assigned to one of five categories: (I) benign; (II) probably benign; (III) undetermined; (IV) probably malignant; (VI) high suspicion of malignancy. These categories represent the radiologist classification of malignancy probability of the evaluated nodule in real clinical setting. There were totally 15 junior and 12 senior radiologists in our department involved in the CT evaluations.
Three classification models
We used the clinical information and nodule characteristics to calculate the Mayo model, Brock model, and VA model outcomes, to assess malignancy probability. The detail calculation formulas of the three models are described in Appendix 1.
Decision analysis for the radiologist diagnosis and the three models
Decision curve analysis (DCA) (16,17) was used for the assessment of lung cancer diagnostic outcomes for radiologist diagnosis and all three models. For this analysis, we used R software version 3.0.3 (R Development Core Team, Vienna, Austria).
We also evaluated the malignancy risk thresholds of the Mayo model made by the American College of Chest Physicians (ACCP) (observe if <5%, indeterminate if 5–65%, surgery if >65%) (18), and the malignancy risk thresholds of the Brock model made by the British Thoracic Society (BTS) (observe if <10%, indeterminate if 10–70%, surgery if >70%) (19). The VA model also has recommended using malignancy risk thresholds (observe if <20%, indeterminate if 20–69%, surgery if >69%) (4). We defined the radiologist diagnosis risk thresholds based on the malignancy probability of the five categories (observe =1, indeterminate =2 or 3 or 4, surgery =5). The “Observe” group was recommended for 1-year CT follow-up, the “indeterminate” group was recommended for further examinations (PET-CT, contrast-enhanced CT, biopsy or short interval CT follow-up), and the “surgery” group was recommended for surgery.
Statistical analysis
Data were reported as numbers (%) for categorical variables. The Pearson’s Chi-square test was used to test for the difference between categorical variables. Normally distributed data were presented as mean [standard deviation (SD)] and were compared using Student’s t-test. Non-normally distributed continuous variables were presented as medians [inter-quartile range (IQR)] and were evaluated by Mann-Whitney U test. A receiver operating characteristic (ROC) curve was constructed to assess the diagnostic accuracy of each model by calculating the area under the ROC curve (AUC) and 95% CI. To compare the AUC values between each two models, the non-parametric approach of DeLong et al. (20) was used, and Bonferroni correction was applied to adjust for multiple comparisons. Decision curve analysis (DCA) (16,17) was also used for the assessment of diagnostic outcomes for all models. All statistical analyses were performed using SPSS software version 20.0 (IBM, New York, US), R software version 3.0.3 (R Development Core Team, Vienna, Austria), and MedCalc Statistical Software version 18.2.1 (Ostend, Belgium; http://www.medcalc.org; 2018). P values <0.05 were considered to indicate statistical significance.
Results
Clinical and nodule characteristics
In total, 277 eligible patients with 286 nodules remained in our study. For nine cases had multiple nodules (5 benign and 4 malignant). In four cases (non-solid nodules) the two nodules present were surgically removed, because they were in the same lobe. The other five cases received CT follow-up to confirm benign nature of the non-resected nodules. Mean patient age was 57.7 years (SD: 10.4). Patients with lung cancer were generally older than patients with benign nodules (59.4 vs. 52.9 years, P<0.001). Of the patients, 143 (51.6%) were male and 156 (56.3%) were non-smokers. Eleven (4.0%) patients had a history of extra-thoracic cancer diagnosed more than 5 years ago, and 52 (18.8%) patients had a family history of cancer. There were 32 cases of emphysema (11.6%), confirmed by CT diagnosis. The clinical characteristics of patients are summarized in Table 1.
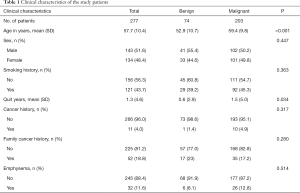
Full table
Among 286 nodules, 207 (72.4%) in 203 patients were malignant. The majority of nodules were solid, 238 (83.2%), of which 162 (68.4%) were malignant, while 34 (34/35, 97.1%) part-solid nodules were malignant. Median nodule diameter of the malignant nodules was 18.0 mm (IQR: 15.0–22.0 mm). This was significantly larger than the median diameter of 14.0 mm of benign nodules (IQR: 11.0–19.0 mm) (P=0.025). Malignant nodules were more likely to be spiculated than benign nodules (P<0.001). The CT characteristics of nodules are shown in Table 2.
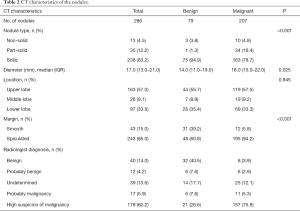
Full table
Histopathological results of nodules
Among 286 nodules, 26 nodules were confirmed by CT follow-up (11 malignant nodules and 15 benign nodules), and 260 nodules were confirmed by surgery (196 malignant nodules and 64 benign nodules). The most common histopathological diagnosis was adenocarcinoma [N=163 (83.2%)], followed by squamous cell carcinoma [N=18 (9.2%)]. There were 64 histologically proven benign nodules. The most common type was hamartoma [N=20 (31.3%)]; other common histopathological types were inflammatory pseudotumors [N=13 (20.3%)], and tuberculosis [N=13 (20.3%)]. The histopathological results are shown in Table 3.
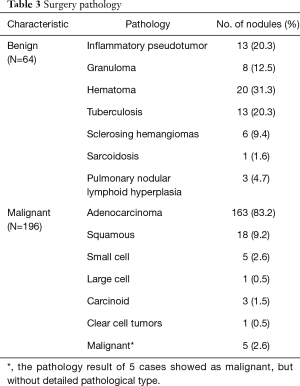
Full table
ROC curves for the radiologist diagnosis and three models
The area under the ROC curve (AUC, 95% CI) was used to compare the diagnostic performance between radiologist evaluation and the three models. For radiologist diagnosis, the AUC was 0.78 (0.73–0.83), for the Mayo model, 0.77 (0.72–0.82), for the Brock model, 0.77 (0.72–0.82), and for the VA model, 0.66 (0.60–0.71) (Figure 1). The radiologist diagnosis was slightly better in the estimation of the probability of cancer than the three models, although it was not statistically significant compared with the Brock model (P=0.712), or Mayo model (P=0.676). The diagnostic performance of the VA model was significantly worse than the radiologist diagnosis and the other models (vs. radiologist diagnosis, P=0.003; vs. Mayo model, P<0.001; vs. Brock model, P=0.004).
Decision analysis for the radiologist diagnosis and three models
Decision curve analysis was used to facilitate the comparison between different radiologist diagnosis and three classification models. As seen in Figure 2, the decision curve analysis graphically shows the clinical usefulness of each model based on a continuum of potential thresholds for lung cancer risk (x-axis) and the net benefit of using the model to risk stratify patients (y-axis) relative to assuming that no patient will have lung cancer. In this analysis, the radiologist diagnosis provided a larger net benefit across the range of lung cancer risk compared with the three models, at a threshold from about 0.22 to 0.88. Radiologist diagnosis had the highest discriminatory power for predicting malignant nodules, compared to the three risk classification models.
The three threshold decision analysis results are shown in Table 4. Radiologist diagnosis showed the highest negative predictive value [N=32 (80.0%)], while the three models showed higher positive predictive value (90.3–100%). The true positive rate of the radiologist’s diagnosis was much higher [157 (75.8%)] than the Mayo model [28 (13.5%)], the Brock model [2 (1.0%)] and the VA model [32 (15.5%)].
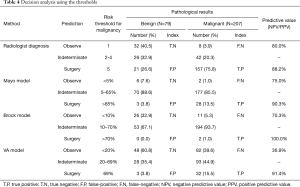
Full table
Discussion
Before choosing a classification model for the assessment of malignancy risk in pulmonary nodules, models should be executed with care and validated in local populations. In our retrospective study in a Chinese population, the AUC of the Mayo model and Brock model were similar to radiologist diagnosis. But, the three-class classifying threshold analysis and DCA showed that the radiologist evaluation had higher discriminatory power for malignancy than the three classification models. Therefore, for the three common classification models used in our study, which were developed within Western populations, applicability to Asian populations should be optimized.
Our AUC results showed that discriminatory ability of the Mayo model and the Brock model did not differ significantly from the radiologist diagnosis (Figure 2) but the diagnostic accuracy was lower than the results reported by Swensen et al. (Mayo, AUC 0.833±0.022 SE) (3), and McWilliams et al. (Brock, AUC: 0.96; 95% CI: 0.93–0.98) (5). The AUC of the VA model (0.658, 95% CI: 0.600–0.713) was significantly lower than radiologist diagnosis (P=0.003) and also lower than the results reported by Michael et al. (VA, AUC: 0.78; 95% CI: 0.73–0.83) (3). The difference in accuracy between prior studies and ours can be explained by several factors. First, the Brock model (5) was trained on data from a lung cancer screening trial, and most of the nodules were smaller (median: 3.5 mm, 25th–75th percentile: 2.8–5.0 mm) and were more often benign (98.5%) than our study (median: 17.0 mm, 25th–75th percentile: 13.0–21.0 mm; 27.6% benign). The previous study also showed that the Brock classification model has limited use in predicting the malignancy of larger pulmonary nodules (15). Second, our inclusion criteria differed from the Mayo study. The Mayo study identified patients based on pulmonary nodules visible on chest X-rays, while our cohort was based on CT scan findings, and may have included more nodules. The malignancy proportion in our cohort (72.4%) was much higher than that in the Mayo study (23.2%), which inevitably led to an inaccuracy. Third, the VA model calculation formula included only four predictors: smoking history, years after quitting smoking, age, and diameter of the nodule. Contrary to the other models, the VA model did not include radiological features, this may explain its lower classification accuracy. Fourth, there are well-known differences in characteristics and in tumor types of lung cancer patients from Asian and Western populations. For example, the incidence of lung cancer is higher in middle-aged, non-smoking Asian women, and sub-solid nodules are more often detected and diagnosed as adenocarcinoma (2,13,14,21-24). The lower discriminatory power of the three classification models was also shown in some previous studies in Asian populations (7,8,12).
A ROC curve is designed as a discriminator, to illustrate the diagnostic ability of a binary classifier system and return an optimal threshold value (25). However, pulmonary nodule risk assessment is not a simple binary classification; often a three-class classifying system, based on malignancy risk thresholds, is recommended (26). Based on the result of using thresholds, only 28 (13.5%), 2 (1.0%), and 32 (15.5%) out of 207 malignancies were predicted above the surgical threshold using the Mayo, Brock and VA models, respectively. The majority of the remaining nodules were indeterminate (Mayo model 85.5%; Brock model 93.7%; VA model 44.9%). In contrast to the three models, the radiologist diagnosis predicted 157 (75.8%) above the surgical threshold, 42 (20.3%) indeterminate, and 8 (3.9%) under the observe threshold out of 207 malignancies, thus showing a higher discriminatory power. The large proportion of patients classified as indeterminate in the three models could inevitably lead to several drawbacks, such as an increase in costs due to more PET-CT examinations, biopsies, and short interval CT follow-up examinations. Additionally, it also may result in delayed diagnosis or misdiagnosis. Some previous studies showed similar findings (27,28).
Previous studies have also reported that radiologist’s diagnosis as a means for predicting malignancy in pulmonary nodules is more accurate than nodule classification models (29,30). However, this does not mean that the models are limited use. As an accessorial classification method, malignancy classification models can effectively avoid the subjective and unreliable for radiologist diagnosis, especially when evaluated by radiologists with little experience. But many parameters and characteristics need to be optimized before using in clinical, especially the best cut-off value for three-class classifying threshold.
Our study has a number of limitations. First, our study population may reflect a degree of selection bias since our hospital is a tertiary referral cancer center. Most patients were referred with suspected malignant nodules. For patients with very small nodules, we did not perform imaging follow-up at our center. Therefore, they were excluded in case they were not resected. Second, our radiologist diagnosis was based on the CT reports, signed by two radiologists (one junior, one senior), so the impact of radiologists’ experience could not be considered. In addition, radiologist diagnosis utilized CT characteristics including contrast enhancement and more image features (pleural indentation, vacuole, vascular invasion) of the nodule, which the three models did not take into consideration for their risk classification. Finally, due to the lack of PET information and quantitative nodule features, we did not evaluate nodule classification models that incorporated PET information (31,32) and radiomics features (33-35), which might be more accurate than the classification models evaluated in our study.
Conclusions
In conclusion, the AUC of the Mayo model and Brock model were similar to radiologist diagnosis, and the radiologist’s evaluation demonstrated better diagnostic performance in a Chinese clinical population. This suggests for the radiologists, it might of value to work on the development of a classification model based on more radiological features and the characteristics of population.
Supplementary
Appendix 1 The calculation formulas of the three models
The Mayo model calculation formula was Logodds = (0.0391 * Age) + (0.7917 * Smoking) + (1.3388 * Cancer) + (0.12 74 * Diameter) + (1.0407 * Spiculation) + (0.7838 * upper) − 6.8272; Cancer probability = 100 * [e(Logodds) / (1 + e(Logodds))], where age is the patient’s age in years; Cigarettes =1 if the patient is a current or former cigarette smoker (otherwise =0), Cancer =1 if the patient has a history of an extrathoracic cancer that was diagnosed more than 5 years ago (otherwise =0), Diameter is the largest diameter of the SPN in millimeters; spiculation =l if the edge of the SPN has spicules (otherwise =0), and upper =l if the SPN is located in an upper lobe (otherwise =0).
The VA model calculation formula was Logodds = −8.404 +2.061 * Smoke + 0.779 * Age / 10 + 0.112 * Diameter + 0.567 * Yearsquit/10; Cancer probability =100 * [e(Logodds) / (1 + e(Logodds))], where smoke is 1 if a current or former smoker (otherwise 0), Age/10 is age in years divided by 10, Diameter is the largest diameter of the nodule in millimeters, and yearsquit/10 is the number of years since quitting smoking divided by 10.
The Brock model calculation formula was Logodds = [0.0287 * (Age − 62)] + Sex + FamilyHistoryLungCa + Emphysema – {5.3854 * [(Nodulesize/10)−0.5 − 1.58113883)] + Noduletype + (0.6581 * NoduleUpperLung) – [0.0824 * (Nodulecount − 4)] + Spiculation − 6.7892}; Cancer probability = 100 * {e(Logodds) / [1 + e(Logodds)]}; Female =0.6011 and male =0, FamilyHistoryLungCa =0.2961 if the patient has a family cancer history (otherwise =0); Emphysema =0.2953 if the patient CT image showed emphysema (otherwise =0); Nonsolid =-0.1276, sub-solid =0.377, solid =0; NoduleUpperLung =l if the SPN is located in an upper lobe (otherwise =0), and spiculation =0.7729 if the edge of the nodule has spicules (otherwise =0).
Acknowledgments
I wish to thank Diane Black for her linguistic assistance during the preparation of this manuscript, and thank Yisong Wang for her full support for my work.
Funding: This work was supported by a grant from the Royal Netherlands Academy of Arts and Sciences (grant number. PSA_SA_BD_01) and Ministry of Science and Technology of the People’s Republic of China, National Key R&D Program of China (grant number. 2016YFE0103000).
Footnote
Conflict of Interest: The authors have no conflicts of interest to declare.
Ethical Statement: The authors are accountable for all aspects of the work in ensuring that questions related to the accuracy or integrity of any part of the work are appropriately investigated and resolved. The study was approved by institutional ethics committee board of Tianjin Medical University Cancer Institute and Hospital (No. bc2018039).
References
- Oudkerk M, Devaraj A, Vliegenthart R, et al. European position statement on lung cancer screening. Lancet Oncol 2017;18:e754-66. [Crossref] [PubMed]
- Liang W, Shao W, Jiang G, et al. Chinese multi-institutional registry (CMIR) for resected non-small cell lung cancer: survival analysis of 5,853 cases. J Thorac Dis 2013;5:726-9. [PubMed]
- Swensen SJ, Silverstein MD, Ilstrup DM, et al. The probability of malignancy in solitary pulmonary nodules. Application to small radiologically indeterminate nodules. Arch Intern Med 1997;157:849-55. [Crossref] [PubMed]
- Gould MK, Ananth L, Barnett PG. A clinical model to estimate the pretest probability of lung cancer in patients with solitary pulmonary nodules. Chest 2007;131:383-8. [Crossref] [PubMed]
- McWilliams A, Tammemagi MC, Mayo JR, et al. Probability of cancer in pulmonary nodules detected on first screening CT. N Engl J Med 2013;369:910-9. [Crossref] [PubMed]
- Winkler Wille MM, van Riel SJ, Saghir Z, et al. Predictive Accuracy of the PanCan Lung Cancer Risk Prediction Model -External Validation based on CT from the Danish Lung Cancer Screening Trial. Eur Radiol 2015;25:3093-9. [Crossref] [PubMed]
- Zhang X, Yan HH, Lin JT, et al. Comparison of three mathematical prediction models in patients with a solitary pulmonary nodule. Chin J Cancer Res 2014;26:647-52. [PubMed]
- Xiao F, Liu D, Guo Y, et al. Novel and convenient method to evaluate the character of solitary pulmonary nodule-comparison of three mathematical prediction models and further stratification of risk factors. PLoS One 2013;8:e78271. [Crossref] [PubMed]
- Soardi GA, Perandini S, Larici AR, et al. Multicentre external validation of the BIMC model for solid solitary pulmonary nodule malignancy prediction. Eur Radiol 2017;27:1929-33. [Crossref] [PubMed]
- Al-Ameri A, Malhotra P, Thygesen H, et al. Risk of malignancy in pulmonary nodules: A validation study of four prediction models. Lung Cancer 2015;89:27-30. [Crossref] [PubMed]
- Perandini S, Soardi GA, Motton M, et al. Solid pulmonary nodule risk assessment and decision analysis: comparison of four prediction models in 285 cases. Eur Radiol 2016;26:3071-6. [Crossref] [PubMed]
- Yang B, Jhun BW, Shin SH, et al. Comparison of four models predicting the malignancy of pulmonary nodules: A single-center study of Korean adults. 2018;13:e0201242.
- Kobayashi Y, Ambrogio C, Mitsudomi T. Ground-glass nodules of the lung in never-smokers and smokers: clinical and genetic insights. Transl Lung Cancer Res 2018;7:487-97. [Crossref] [PubMed]
- Zhou W, Christiani DC. East meets West: ethnic differences in epidemiology and clinical behaviors of lung cancer between East Asians and Caucasians. Chin J Cancer 2011;30:287-92. [Crossref] [PubMed]
- Hammer MM, Nachiappan AC, Barbosa EJM Jr. Limited Utility of Pulmonary Nodule Risk Calculators for Managing Large Nodules. Curr Probl Diagn Radiol 2018;47:23-7. [Crossref] [PubMed]
- Vickers AJ. Decision analysis for the evaluation of diagnostic tests, prediction models and molecular markers. Am Stat 2008;62:314-20. [Crossref] [PubMed]
- Vickers AJ, Elkin EB. Decision curve analysis: a novel method for evaluating prediction models. Med Decis Making 2006;26:565-74. [Crossref] [PubMed]
- Gould MK, Donington J, Lynch WR, et al. Evaluation of individuals with pulmonary nodules: when is it lung cancer? Diagnosis and management of lung cancer, 3rd ed: American College of Chest Physicians evidence-based clinical practice guidelines. Chest 2013;143:e93S-e120S.
- Callister ME, Baldwin DR, Akram AR, et al. British Thoracic Society guidelines for the investigation and management of pulmonary nodules. Thorax 2015;70 Suppl 2:ii1-54. [Crossref] [PubMed]
- DeLong ER, DeLong DM, Clarke-Pearson DL. Comparing the areas under two or more correlated receiver operating characteristic curves: a nonparametric approach. Biometrics 1988;44:837-45. [Crossref] [PubMed]
- Jemal A, Bray F, Center MM, et al. Global cancer statistics. CA Cancer J Clin 2011;61:69-90. [Crossref] [PubMed]
- Kawaguchi T, Matsumura A, Fukai S, et al. Japanese ethnicity compared with Caucasian ethnicity and never-smoking status are independent favorable prognostic factors for overall survival in non-small cell lung cancer: a collaborative epidemiologic study of the National Hospital Organization Study Group for Lung Cancer (NHSGLC) in Japan and a Southern California Regional Cancer Registry databases. J Thorac Oncol 2010;5:1001-10. [Crossref] [PubMed]
- Zhang J, Xu Y, Gao P, et al. Assessment of Lung Cancer Risk-Regional Respiratory Disease Screening Report in Jilin, China. J Thorac Oncol 2017;12:S578. [Crossref]
- She J, Yang P, Hong Q, et al. Lung cancer in China: challenges and interventions. Chest 2013;143:1117-26. [Crossref] [PubMed]
- Linden A. Measuring diagnostic and predictive accuracy in disease management: an introduction to receiver operating characteristic (ROC) analysis. J Eval Clin Pract 2006;12:132-9. [Crossref] [PubMed]
- Perandini S, Soardi GA, Motton M, et al. - Risk of malignancy in pulmonary nodules: A validation study of four prediction models. Lung Cancer 2015;90:118-9. [Crossref] [PubMed]
- Isbell JM, Deppen S, Putnam JB Jr, et al. Existing general population models inaccurately predict lung cancer risk in patients referred for surgical evaluation. Ann Thorac Surg 2011;91:227-33; discussion 33. [Crossref] [PubMed]
- Perandini S, Soardi GA, Motton M, et al. Limited value of logistic regression analysis in solid solitary pulmonary nodules characterization: a single-center experience on 288 consecutive cases. J Surg Oncol 2014;110:883-7. [Crossref] [PubMed]
- Iaccarino JM, Simmons J, Gould MK, et al. Clinical Equipoise and Shared Decision-making in Pulmonary Nodule Management. A Survey of American Thoracic Society Clinicians. Ann Am Thorac Soc 2017;14:968-75. [Crossref] [PubMed]
- Tanner NT, Porter A, Gould MK, et al. Physician Assessment of Pretest Probability of Malignancy and Adherence With Guidelines for Pulmonary Nodule Evaluation. Chest 2017;152:263-70. [Crossref] [PubMed]
- Herder GJ, van Tinteren H, Golding RP, et al. Clinical prediction model to characterize pulmonary nodules: validation and added value of 18F-fluorodeoxyglucose positron emission tomography. Chest 2005;128:2490-6. [Crossref] [PubMed]
- Flechsig P, Frank P, Kratochwil C, et al. Radiomic Analysis using Density Threshold for FDG-PET/CT-Based N-Staging in Lung Cancer Patients. Mol Imaging Biol 2017;19:315-22. [Crossref] [PubMed]
- She Y, Zhang L, Zhu H, et al. The predictive value of CT-based radiomics in differentiating indolent from invasive lung adenocarcinoma in patients with pulmonary nodules. Eur Radiol 2018;28:5121-8. [Crossref] [PubMed]
- Zhou Y, He L, Huang Y, et al. CT-based radiomics signature: a potential biomarker for preoperative prediction of early recurrence in hepatocellular carcinoma. Abdom Radiol (NY) 2017;42:1695-704. [Crossref] [PubMed]
- Liao Z, Mohan R, Court L, et al. Radiomics of pulmonary nodules and lung cancer. Transl Lung Cancer Res 2017;6:86-91. [PubMed]