Validation of a serum 4-microRNA signature for the detection of lung cancer
Introduction
Lung cancer (LC) is the leading cause of cancer death worldwide (1). Despite numerous advancements in LC therapies in recent years, the 5-year survival rate is only 18% (1). As LC symptoms occur late in the course of the disease, most patients present in advanced stages of disease and these patients are far less likely to respond to currently available therapies. Early diagnosis is key to the management of LC patients with curative intent treatment yielding 5-year survival of 75% (2). There are several LC risk prediction models based on patient characteristics and/or CT scan data with the area under the curve (AUC) of ranging from 0.57–0.86 (3-6). Low-dose screening CT (LDCT) scans detect more stage I LCs than chest radiography and can reduce LC relative risk for mortality of 20% (7). Although LDCT is more sensitive in detecting early-stage LCs, one of the major limitations is the occurrence of false-positive screen results (5). Several circulating molecular markers have been proposed, e.g., cell free DNA, gene methylation and multiple marker approaches (8-11). More recently, microRNAs (miRNAs) present in bodily fluids have been proposed as stable and reproducible biomarkers (12-16). We sought to develop novel noninvasive serum markers based upon the miRNA’s changes demonstrated in LC that could enhance the sensitivity and specificity of current diagnostic/predictive tools. Positive signals by such biomarkers could increase the pre-test probability of cancer.
MiRNAs are often highly dysregulated in human cancers, including LC, and may serve as oncogenes or tumor suppressor genes contributing to cancer initiation and progression (17). In addition to permitting sub-classification of LC (14,18), specific miRNA profiles also may predict prognosis and disease recurrence in early-stage LC (19-23). Several patterns of miRNAs were reported to be associated with lymphocytic leukemia, lung, breast, prostate and pancreas cancers by using microarray platforms or real-time quantitative reverse transcription polymerase chain reaction (qRT-PCR) (24).
Our previous studies have identified a serum 4-miRNA signature which could distinguish LC patients from non-cancer controls (NCs) with high accuracy (25). This study enrolled 154 LC patients and 45 NCs, of which 92 were used for discovery by miRNA array, and 107 for independent validation by RT-PCR. This 4-miRNA (miR-141, miR-193b, miR-200b, miR-301) signature exhibited an AUC of 0.985 in the discovery set and 0.993 in the validation set. Importantly, these 4-miRNAs were selected because they were highly expressed in LC tissue, increasing the likelihood that these were secreted from the tumor cells rather than blood cells (20). In order to verify this 4-miRNA signature, we expanded our evaluation of this 4-miRNA signature in a large independent cohort of 398 subjects including 213 LC patients and 185 NCs using qRT-PCR assay (Figure 1). The predictive signature for LC diagnosis was obtained using logistic regression models based on training-test analysis.
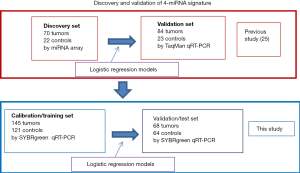
Methods
Patient and control sera collection
A total of 398 patient sera used in this study were collected from the University of Michigan Health System (UM) and the Veterans Affairs Ann Arbor Health System (VA) from 1991 to 2017. These samples included 213 LC patients and 185 NCs. Written consent was provided by all enrolled patients, and this study was approved by the University of Michigan Institutional and the VA Health System Review Board and Ethics Committee. The detailed clinical features are shown in Table S1 and Figure S1. Among 213 cancers, there were 113 adenocarcinomas (ACs), 56 squamous cell carcinomas (SCCs), 10 small cell LCs (SCLCs), 17 large cell cancers (LCCs) and 17 metastatic cancers (from other primary cancers) (Metas) (Figure S1A). Regarding tumor stage, there were 112 cases diagnosed as stage I, 39 stage II, 31 stage III, and 7 stage IV. In 185 NCs, the 99 sera from UM included patients with benign lung disease [5], lupus [48], reflux esophagitis [19] and normal healthy volunteers [27]; 86 sera from the VA included patients (all smokers) with benign lung nodules [39] and non-lung nodule controls [47] (Figure S1C,D). The subjects in controls have more young and male. Equally distributed with respect to smoking status (30–45 vs. >45) between LC and NC (Table S1).
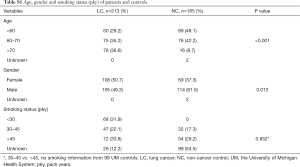
Full table
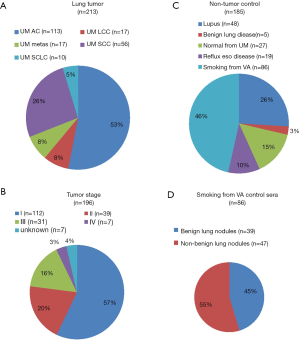
Peripheral blood from each subject was processed for serum extraction within 1 hour after blood-draw. After centrifuging at 3,000 rpm for 10 min at room temperature, serum was transferred into microfuge tubes (300 µL in each tube) and frozen instantly in liquid nitrogen, and then placed at –80 °C for long-term storage.
Preparation of serum total RNA and miRNA quantitative qRT-PCR
Total RNA from serum was purified using the miRNeasy Serum/Plasma Kit (Qiagen, Hilden, Germany) following the manufacturer’s protocol. The details of preparation of total RNA was described previously (25). For each sample, cel-miRNA-39 was used as a spike-in control, and added into the mixture with Qiazol and serum at a final concentration at 0.1 pM (volume ratio of Qiazol to serum was 5:1). After purification and assessment of concentration, all total RNA was kept at –80 °C until use.
Reverse transcription (RT) was conducted with 100 ng total RNA using the miScript II RT Kit (Qiagen, Hilden, Germany). qPCR reactions were performed by the 7900HT system (Applied Biosystems, Thermo Fisher Scientific, Waltham, MA, USA) using miScript SYBR® Green PCR Kit (Qiagen, Hilden, Germany). All protocols were followed according to the manufacturer’s instructions. The qRT-PCR conditions were 1 cycle at 95 °C for 15 min followed by 40 cycles at 94 °C for 15 sec, 55 °C for 30 sec and 70 °C for 30 sec. Primers for miRNAs of interest (miR-141, miR-193b, miR-200b and miR-301) were purchased from Invitrogen. We calculated the relative amounts of selected miRNAs using the equation 2-ΔΔCt. Cel-miRNA-39 detected by qRT-PCR was used as an internal loading control. We have tested the repeatability of the PCR assay and a significantly correlation was observed (Figure S2).
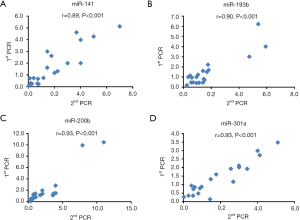
Statistical analysis
Data were analyzed using GraphPad Prism 6 (GraphPad Software Inc., CA, USA), Excel and R software. Receiver operating characteristic (ROC) curve and AUC analyses were used to show the tradeoff between sensitivity and specificity for the different possible cutoff-points for a diagnostic test. The different concentrations of miRNAs between subjects with cancer and controls were evaluated by unpaired Student’s t-test. A two-tailed P value <0.05 was considered significant. The gene ontology (GO) signaling analysis of miRNA target genes was performed using DAVID web at https://david.ncifcrf.gov.
Prediction model building with training set and test on test set
Log2 transformation of the 4-miRNA (miR-141, miR-193b, miR-200b and miR-301) qRT-PCR expression data was completed using the log(x+1, 2) formula which included 398 subjects (213 LCs and 185 NCs). We randomly sampled 2/3 of the entire data set as the training set with 266 subjects, the remaining 1/3 was treated as the test set with 132 subjects. The proposed prediction model is a logistic regression model defined as logit(πi) = β0 + β1X1i + β2X2i + β3X3i + β4X2i + εi where πi denotes the probability of having LC for the ith patient. X1i, X2i, X3i, X4i are the corresponding log2 transformed values of miRNA-141, miRNA-193b, miRNA-200b and miRNA-301 PCR batch for the ith patient. εi is the error term for this model.
We first fitted this logistic regression model on the training set only. With leave-one-out cross validation, we predicted the probability of having cancer for each subject based on the model fitted with all other subjects. Then the training set AUC was computed based on the training data only with ROC function in pROC R package. The corresponding training set ROC curve was also plotted based on the training set, and the cutoff probability for cancer prediction was selected with a training set specificity greater than 90%. Then we computed the predicted cancer indicator for each patient. If the predicted probability was greater than the cutoff value, the subject was predicted to have cancer; conversely if the predicted probability was lesser than the cutoff value, the subject was predicted to have a benign condition. Using the optimal cutoff value, we calculated the corresponding training set specificity and sensitivity.
Furthermore, the predicted probability of having cancer for each subject in the test set was predicted by the logistic regression model fitted on the training set. The test set AUC and ROC curves were then calculated. The same cutoff probability for cancer prediction was used to calculate the corresponding specificity, sensitivity, positive predictive value (PPV), negative predictive value (NPV) and accuracy of the test set.
Cell proliferation and colony formation
The cell proliferation was assessed using WST-1 (Roche, Basel, Switzerland) according to manufacturer instructions. Briefly, a total of approximately 1,000 cells were plated in 96-well plates, at 96–120 h after treated with miRNA mimics or inhibitors, added 10 µL/well of WST-1 solution and the cell proliferation curves were plotted using the 450 and 630 nm absorbance. All experiments were performed in triplicate. For colony formation, 200 miRNA mimics or inhibitors treated cells were plated into 6-well plates and incubated in RPMI-1640 or DMEM medium with 10% FBS at 37 °C. Seven to ten days later, the cells were fixed and stained with 0.1% crystal violet. The number of colonies was counted, with a colony being defined as greater than 50 cells.
Western blot
Treated total cell lysates were prepared with sample buffer and boiled at 95 °C for 6 min. The samples were transferred to SDS-PAGE at 90 V for 2–3 h and then transferred to PVDF membranes for another 2–3 h. After incubation with specific primary antibodies at 4 °C overnight, the membranes were then washed by 1% TBST for three times, incubated with secondary antibodies for 1 h, and the membranes were developed using enhanced chemiluminescence (ECL) and exposed using Bio-Rad image system.
Results
Four miRNAs levels are higher in the serum of patients with LC as compared to controls
We previously used miRNA microarrays to analyze the expression profiles of 700 miRNAs in primary tumor tissue and sera from patients with LC (20,25). By analyzing both tissue and serum miRNAs expression, we discovered and verified that a 4-miRNA (miR-141, miR-193b, miR-200b, miR-301) signature could be used as candidate biomarkers for early detection of LC (25) (Figure 1). To further verify this 4-miRNA signature, we measured it by qRT-PCR in the serum of a large, independent cohort of subjects including 213 with LC and 185 NCs (Table S1 and Figure S1). We found that all four miRNAs showed a significantly higher expression in the sera of LC patients compared to NC controls (P<0.0001) (Figures 2,S3). These results were consistent with our previous findings (25), validating that these 4-miRNAs were increased in LC as compared to NCs.
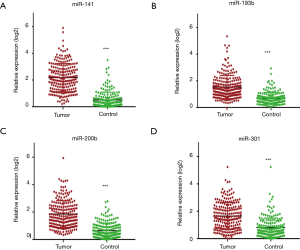
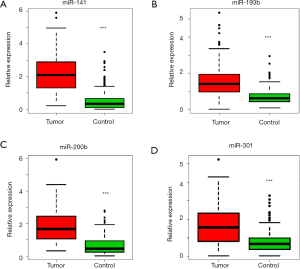
We next analyzed the variability of these 4-miRNAs using several basic clinical variables including patient age, gender, smoking status, stage and lymph nodal metastasis. We did not find significant differential expression of these 4-miRNAs with respect to age (Figure S4A,B), gender (Figure S4C,D) or smoking status [pack years (pky)] (Figure S4E,F). Additionally, the stage of cancer, presence of lymph node metastasis or tumor size did not have an impact on the expression levels of these 4-miRNAs. (Figure S4G,H,I).
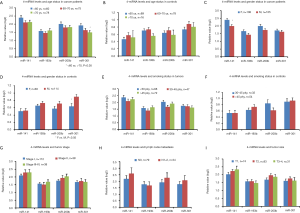
Change in levels of serum 4-miRNAs in subtypes of LC and controls
Among 213 cancers in this study, there were 113 ACs, 56 SCCs, 10 SCLCs, 17 LCCs and 17 pulmonary metastatic tumors (Figure S1A). Here we are asked whether there was a different abundance of these four miRNAs among these different histological subtypes of LC. We found that the serum 4-miRNAs were relatively lower in SCC than in AC (P<0.05) although these 4-miRNAs were still significantly higher in SCC as compared to NCs (Figures 3,S5A). In SCLC, miR-301 was relatively lower as compared to AC.
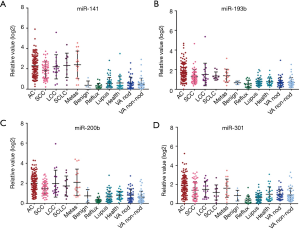
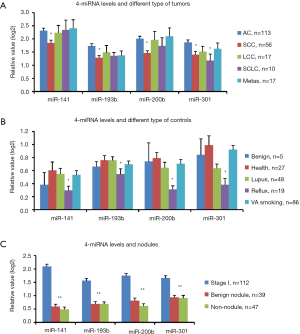
The 185 NCs originated from two cohorts. The UM cohort included 5 subjects with benign lung disease, 48 with lupus, 19 with reflux esophagitis and 27 normal healthy controls. The VA cohort included 86 subjects, with pky of smoking ranging from 30–210 (average 62 pky) (Figure S1C,D). We found that the serum 4-miRNAs were relatively lower in subjects with reflux esophagitis compared with other controls (P<0.05) (Figures 3,S5B). There was no significant variation in the concentration of these 4-miRNAs among VA smoker cohort or the UM cohort.
We then evaluated differences in the concentration of these 4-miRNAs based on whether the 86 smoking subjects had benign nodules on CT (n=39) or non-nodule controls (n=47) (Figure S1D). There was a total of 112 stage I LC with nodule size less than 4 cm. By comparing these 3 groups of subjects, we found that the concentration of four miRNAs was significantly higher in early-stage LCs than in subjects with benign lung nodules or non-nodule controls (Figure S5C). There was no difference in serum 4-miRNA concentrations between subjects with benign nodules and non-nodules controls. These results further suggest that these 4-miRNAs could be considered to detect early LC.
Serum 4-miRNA signature can predict LC
We analyzed the performance of the 4 individual miRNAs by ROC curves to distinguish LC from NCs based in all 398 subjects. We obtained AUC values of 0.775–0.934 (Figure S6), indicating these 4 serum miRNAs concentrations are not only higher in cancers but also have excellent performance to distinguish cancer from NCs.
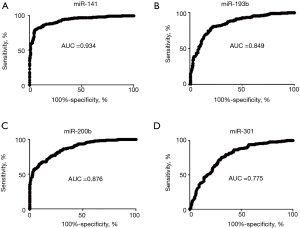
In our previously published study (25), we used a 4-miRNA signature by combining these 4-miRNAs using logistic regression analysis to ascertain their ability to diagnose LC. Consistent with our published study, in this study, we combined the 4-miRNAs as a 4-miRNA signature and used a logistic regression model to validate the performance of the signature as an accurate predictor of LC diagnosis. Since the methods used for miRNAs detection in this study were different from previous study (25) [In previous study, serum RNAs were isolated using miRVana PARIS kit (Ambion, Austin, TX, USA), cDNAs were pre-amplified before PCR and the assay for miRNA detection was TagMan technology], we have to calibrate the beta coefficients by a calibration (training) set. To do this, we first randomly sampled 2/3 of the subjects as the training (to calibrate the beta coefficients) set with 266 subjects (145 cancers and 121 controls), the remaining 1/3 was treated as the test (validation) set with 132 subjects (68 cancers and 64 controls) (Table 1, Figure 1). Next, in the training set, we fitted a logistic regression with these 4-miRNAs with leave-one-out cross validation to build a prediction model. The AUC in the training set was 0.946 [95% confidence interval (CI), 0.920–0.972] (Figure S7), and 0.921 (95% CI, 0.876–0.966) on the test set respectively with specificity of 90.6% and sensitivity 77.9%. The PPV was 89.8% and NPV 79.5% in the test set, with an accuracy of 84.1% (Figure 4). When we applied this prediction model to stage I and benign nodule control only in the test set, we obtained an AUC of 0.876 with specificity 78.6%, sensitivity 80.6%, PPV 89.3%, NPV 64.7% and accuracy 80.0% (Figure S8). Since AC was the most common type of LC (n=113) in this study, we also applied this prediction model to AC cases only. We obtained an AUC of 0.942 on the test set with specificity 90.6%, sensitivity 83.3%, PPV 83.3%, NPV 90.6% and accuracy 88.0% (Figure S9). These results suggest that the 4-miRNA serum-based signature could be used as predictor of LC and distinguish benign pulmonary nodule from early LC although this study may be still in a phase 2 or early phase 3 for biomarker development according to Pepe et al JNCI 2001 (26).
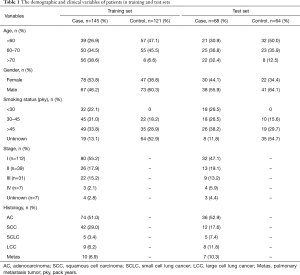
Full table
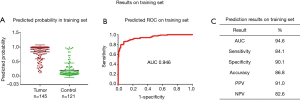
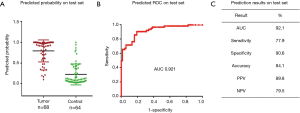
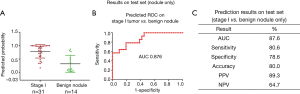
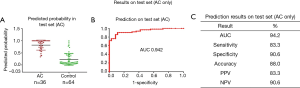
Cell proliferation and colony formation were affected by miR-141 and miR-193
In order to test if these 4-miRNAs are functional in LC, we performed cell proliferation and colony formation experiments with these miRNA mimic or inhibitors treatment. We found that miR-141-3p mimic could increase colony formation and cell proliferation in LC cell lines, while miR-193-3p mimic could inhibit colony formation and cell proliferation in LC cell lines (Figure 5). We also found that miR-200b-3p mimic could inhibit colony formation, while miR-300a-3p not significant (Figure S10). Western blot indicated that CCND1, p-STAT3 and c-Myc were decreased after miR-193b-3p treatment, while p27 and CCNE1 increased (Figure 6), indicated that these proteins were involved in miR-193b-3p signaling in LC.
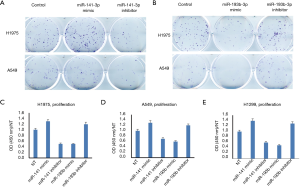
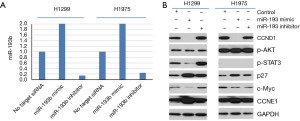
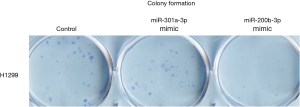
DAVID GO analysis of miRNA target genes
One miRNA could target many genes and one gene could be regulated by several miRNAs. In order to uncover potential molecular cellular biology process involved in these 4-miRNAs, we first selected the miRNA target genes using Targetscan website (http://www.targetscan.org), then analyzed the biology signaling of these genes using DAVID GO. We found that cellular metabolic, gene expression, cellular developmental and cell differentiation etc. were the most regulated biology processes by miR-141 (Figure 7), cell death regulation by miR193, gene expression and metabolic process regulation by miR200b, and metabolic process regulation by miR301 (Figures S11-S13).
Discussion
Detection of LC at an early-stage has the possibility of significantly reducing mortality with a greater chance of cure. MiRNAs are found in tissue, serum and plasma in a stable form protected from endogenous RNase activity and represent promising blood-based tumor markers (12,27-29). Two large-scale validation studies of serum/plasma miRNA signatures for LC detection were reported recently from Italy (15,16). Sozzi reported that the diagnostic performance of a plasma 24-miRNA signature classifier (MSC) for LC detection had an 87% sensitivity and 81% specificity in smokers within the randomized Multicenter Italian Lung Detection (MILD) trial (870 disease-free individuals and 69 LCs). Combination of both MSC and LDCT resulted in a five-fold reduction of LDCT false-positive rate to 3.7% (16). Another validation study of a 13-miR-Test was conducted in high-risk individuals (1,067 cancer-free individuals and 122 cancers) enrolled in the Continuous Observation of Smoking Subjects (COSMOS) LC screening program. The overall accuracy, sensitivity, and specificity of the miR-Test were 74.9%, 77.8%, and 74.8%, respectively, and the AUC is 0.85 (15). However, despite limitations addressed in the study, e.g., single randomized screening trial, these two studies included only a small number of LC subjects (69 and 122, respectively) potentially affecting the diagnostic accuracy as well as the greater number of miRNAs included in their panels (24 and 13 miRNAs, respectively) making it less cost-effective. A recent systematic review of 20 studies indicated that the AUCs of various miRNAs for LC detection ranges from 0.62 to 0.94 (14). These studies have the potential to enhance the utility of miRNAs as serum/plasma/sputum biomarkers for the diagnosis, prognosis or monitoring of LC, however, there was only a small overlap in the reported miRNAs. Possible reasons include: (I) small sample sizes, e.g., most of these studies were included around 100 subjects, (II) miRNAs in serum and plasma may be different, (III) the variations in sample preparation, assays for detection, and data normalization strategies; and (IV) most serum/plasma based miRNAs for LC diagnosis included miRNAs also overexpressed in blood cells, which might have recapitulated the tumor-host interaction, but probably were not derived from the tumor (14-16,28,30-35).
Our group discovered a novel 4-miRNA signature for LC diagnosis (25). To further verify this 4-miRNA signature for LC detection, we further examined the performance of this signature in a large independent cohort of sera from UM and VA institutes which included 213 LCs and 185 NCs. By means of qRT-PCR assay, we verified that these 4-miRNAs were significantly higher in sera of LC patients as compared to controls. This expression was not related to patient age, gender, and smoking status, as well as tumor stage, tumor size and lymph node metastasis. We also found the 4-miRNAs levels were not different among different non-cancerous benign conditions.
As described above, we used rigorous cut-off criteria for this 4-miRNA signature and random sampling to allocate training and test sets. A prediction model was built based on training set using logistic regression with leave-one-out cross validation. The predicted probability of having cancer for each subject in the test set was calculated by fitting logistic regression model. We found that the AUC was 0.921 on the test set with specificity 90.6%, sensitivity 77.9%, accuracy 84.1%, PPV 89.8% and NPV 79.5%. Using this prediction model, we also obtained an AUC of 0.876 with specificity 78.6%, sensitivity 80.6% for the distinguish of stage I tumor to benign lung nodules.
There are several advantages to the present study. (I) We have confirmed the findings of our prior report in a different cohort of LC subjects (25). In this validation study, we used 213 tumors and 185 NCs. Thus, a total of 367 LCs and 230 NCs was used for the discovery and validation of this 4-miRNA signature (Figure 1). (II) Multiple types of LC were examined. In our previous study and other published studies (14), only few types of LC were included, e.g., AC and SCC, but in this study, we included all types of LC and even LC metastasis from other organs. (III) Multiple types of NCs were included. Among the controls, we included subjects with normal healthy individuals, patients with benign lung disease, benign pulmonary nodules, individuals with heavy smoking history, and also subjects with non-pulmonary pathologies such as those with lupus and reflux esophagitis whose sera were not used in other studies (14). (IV) Most importantly, we used the preferred biomarker discovery/validation/prediction procedure (36-38), i.e., training-test model which was not used in most other studies (14). We also used other learning models e.g., support vector machine (SVM) and tree-based boosting, and obtained similar performance results (AUC, 0.91; data not shown). (V) This serum based 4-miRNA signature has better performance of AUC (0.921) than recently reported clinical variable and or CT-based 5 models (0.666–0.785) (6) regarding diagnosis/prediction of LC, as well as another two large-scale validation studies of serum/plasma miRNA signatures for LC detection from Italy (15,16).
The major limitations of this study include: (I) The subjects were come from only two institutes, UM and VA. We therefore plan to extend the study of this signature to other institutes, including China to compare the Caucasian and Asian populations. (II) This miRNA signature was not in combination with patient characteristics and/or image analysis on CT scans. The concurrent use of other methods such as tumor morphomics (TMP) may complement our blood-based signature biomarker. TMP analysis is a semi-automated approach that aims to increase the diagnostic accuracy of CTs by providing reproducible, quantitative methods to evaluate various aspects of pulmonary nodules including density, homogeneity, and eccentricity. In 2014, Aerts analyzed 440 TMPs on 1,019 patients with lung or head-and-neck cancer, and demonstrated the prognostic role of TMP features that became a hallmark in TMP studies (39). We plan to combine TMP and serum 4-miRNA signature in the future to develop a robust biomarker that will supplant the need for biopsies to diagnose LC, when faced with indeterminate lung nodules seen on screening CT scans of high-risk individuals.
In this study we have included 17 metastatic LCs with unknow original types of cancer. Since the average levels of these 4-miRNAs in the metastasis cancer were similar as other types of LC (P>0.05), we believe that these metastatic cancers could not affect our final prediction model. Regarding the 19 esophagitis controls, from Figure S5B, we can see that the average levels of these 4-miRNAs (0.30–0.54) in esophagitis controls were lower than other controls (e.g., 0.53–0.92 in smoking). Further analysis of the reason of the difference between esophagitis and other controls, as well as if esophagitis affects the prediction model are warranted.
The miR-141 plays dual roles in different cancers. It could suppress cell proliferation/tumor growth in papillary thyroid, prostatic, liver, ovarian, brain, colorectal, pancreatic and renal cancers (40,41), but promote cell proliferation in LC (42). We found that miR-141-3p could increase colony formation and cell proliferation in LC cell lines. We found that miR-193-3p mimic could inhibit colony formation and cell proliferation via targeting CCND1 which was consistent with others (43,44). In most reports, miR-200b plays a tumor suppression role in cancer, we found that miR-200b was also inhibit colony formation in LC. We didn’t find miR-301b had roles on colony formation in LC. In DAVID GO of biology process analysis of target genes by these 4-miRNAs, we found that cellular metabolic, gene expression, and cell death regulation were the most regulated signaling. Further functional and mechanistic studies were warranted in the future in order to understand the role of these miRNAs in LC.
We have successfully validated our serum 4-miRNA signature in a large cohort of subjects by RT-PCR. This serum 4-miRNA signature may be used for detection of early LC in a heavy smoking population, but also may provide a complementary noninvasive biomarker for the diagnosis of LC in patients with lung nodules on screening CT scans. Further study of phase 4 prospective screening, phase 5 cancer control (26,37), as well as the function/mechanism of these 4-miRNAs is warranted.
Acknowledgments
The authors thank doctors and nurses from University of Michigan Health System and the Veterans Affairs Ann Arbor Health System for the collection of blood samples used in this study.
Funding: This work was supported by the National Institutes of Health (R21CA205414 to Guoan Chen; R01CA154365 to David G. Beer and Andrew C. Chang; U01CA157715 to David G. Beer); the University of Michigan Rogel Cancer Center Support Grant (P30 CA46592), the University of Michigan Rogel Cancer Center (Guoan Chen), University of Michigan Department of Surgery Research Advisory Committee (Guoan Chen), generous patient and family donations to the Section of Thoracic Surgery, University of Michigan (Guoan Chen) and Southern University of Science and Technology support found (Guoan Chen).
Footnote
Conflicts of Interest: The authors have no conflicts of interest to declare.
Ethical Statement: The authors are accountable for all aspects of the work in ensuring that questions related to the accuracy or integrity of any part of the work are appropriately investigated and resolved. This study was approved by the University of Michigan Institutional and the VA Health System Review Board and Ethics Committee (No.00004969 and No. 2016-030233).
References
- Siegel RL, Miller KD, Jemal A. Cancer statistics, 2018. CA Cancer J Clin 2018;68:7-30. [Crossref] [PubMed]
- Hassanein M, Callison JC, Callaway-Lane C, et al. The state of molecular biomarkers for the early detection of lung cancer. Cancer Prev Res (Phila) 2012;5:992-1006. [Crossref] [PubMed]
- Gray EP, Teare MD, Stevens J, et al. Risk prediction models for lung cancer: a systematic review. Clin Lung Cancer 2016;17:95-106. [Crossref] [PubMed]
- Schultz EM, Sanders GD, Trotter PR, et al. Validation of two models to estimate the probability of malignancy in patients with solitary pulmonary nodules. Thorax 2008;63:335-41. [Crossref] [PubMed]
- Patz EF Jr, Greco E, Gatsonis C, et al. Lung cancer incidence and mortality in National Lung Screening Trial participants who underwent low-dose CT prevalence screening: a retrospective cohort analysis of a randomised, multicentre, diagnostic screening trial. Lancet Oncol 2016;17:590-9. [Crossref] [PubMed]
- Schreuder A, Schaefer-Prokop CM, Scholten ET, et al. Lung cancer risk to personalise annual and biennial follow-up computed tomography screening. Thorax 2018. [Epub ahead of print]. [Crossref] [PubMed]
- National Lung Screening Trial Research Team., Aberle DR, Adams AM, et al. Reduced lung-cancer mortality with low-dose computed tomographic screening. N Engl J Med 2011;365:395-409. [Crossref] [PubMed]
- Fleischhacker M, Dietrich D, Liebenberg V, et al. The role of DNA methylation as biomarkers in the clinical management of lung cancer. Expert Rev Respir Med 2013;7:363-83. [Crossref] [PubMed]
- Mamdani H, Ahmed S, Armstrong S, et al. Blood-based tumor biomarkers in lung cancer for detection and treatment. Transl Lung Cancer Res 2017;6:648-60. [Crossref] [PubMed]
- Patz EF Jr, Campa MJ, Gottlin EB, et al. Panel of serum biomarkers for the diagnosis of lung cancer. J Clin Oncol 2007;25:5578-83. [Crossref] [PubMed]
- Sozzi G, Conte D, Leon M, et al. Quantification of free circulating DNA as a diagnostic marker in lung cancer. J Clin Oncol 2003;21:3902-8. [Crossref] [PubMed]
- Ulivi P, Zoli W. miRNAs as non-invasive biomarkers for lung cancer diagnosis. Molecules 2014;19:8220-37. [Crossref] [PubMed]
- Hou J, Meng F, Chan LW, et al. Circulating plasma microRNAs as diagnostic markers for NSCLC. Front Genet 2016;7:193. [Crossref] [PubMed]
- Moretti F, D'Antona P, Finardi E, et al. Systematic review and critique of circulating miRNAs as biomarkers of stage I-II non-small cell lung cancer. Oncotarget 2017;8:94980-96. [Crossref] [PubMed]
- Montani F, Marzi MJ, Dezi F, et al. miR-Test: a blood test for lung cancer early detection. J Natl Cancer Inst 2015;107:djv063. [Crossref] [PubMed]
- Sozzi G, Boeri M, Rossi M, et al. Clinical utility of a plasma-based miRNA signature classifier within computed tomography lung cancer screening: a correlative MILD trial study. J Clin Oncol 2014;32:768-73. [Crossref] [PubMed]
- Croce CM. Causes and consequences of microRNA dysregulation in cancer. Nat Rev Genet 2009;10:704-14. [Crossref] [PubMed]
- Bishop JA, Benjamin H, Cholakh H, et al. Accurate classification of non-small cell lung carcinoma using a novel microRNA-based approach. Clin Cancer Res 2010;16:610-9. [Crossref] [PubMed]
- Raponi M, Dossey L, Jatkoe T, et al. MicroRNA classifiers for predicting prognosis of squamous cell lung cancer. Cancer Res 2009;69:5776-83. [Crossref] [PubMed]
- Nadal E, Zhong J, Lin J, et al. A MicroRNA cluster at 14q32 drives aggressive lung adenocarcinoma. Clin Cancer Res 2014;20:3107-17. [Crossref] [PubMed]
- Yanaihara N, Caplen N, Bowman E, et al. Unique microRNA molecular profiles in lung cancer diagnosis and prognosis. Cancer Cell 2006;9:189-98. [Crossref] [PubMed]
- Yu SL, Chen HY, Chang GC, et al. MicroRNA signature predicts survival and relapse in lung cancer. Cancer Cell 2008;13:48-57. [Crossref] [PubMed]
- Patnaik SK, Kannisto E, Knudsen S, et al. Evaluation of microRNA expression profiles that may predict recurrence of localized stage I non-small cell lung cancer after surgical resection. Cancer Res 2010;70:36-45. [Crossref] [PubMed]
- Schwarzenbach H, Nishida N, Calin GA, et al. Clinical relevance of circulating cell-free microRNAs in cancer. Nat Rev Clin Oncol 2014;11:145-56. [Crossref] [PubMed]
- Nadal E, Truini A, Nakata A, et al. A novel serum 4-microRNA signature for lung cancer detection. Sci Rep 2015;5:12464. [Crossref] [PubMed]
- Pepe MS, Etzioni R, Feng Z, et al. Phases of biomarker development for early detection of cancer. J Natl Cancer Inst 2001;93:1054-61. [Crossref] [PubMed]
- Mitchell PS, Parkin RK, Kroh EM, et al. Circulating microRNAs as stable blood-based markers for cancer detection. Proc Natl Acad Sci U S A 2008;105:10513-8. [Crossref] [PubMed]
- Hu Z, Chen X, Zhao Y, et al. Serum microRNA signatures identified in a genome-wide serum microRNA expression profiling predict survival of non-small-cell lung cancer. J Clin Oncol 2010;28:1721-6. [Crossref] [PubMed]
- Bianchi F, Nicassio F, Marzi M, et al. A serum circulating miRNA diagnostic test to identify asymptomatic high-risk individuals with early stage lung cancer. EMBO Mol Med 2011;3:495-503. [Crossref] [PubMed]
- Boeri M, Verri C, Conte D, et al. MicroRNA signatures in tissues and plasma predict development and prognosis of computed tomography detected lung cancer. Proc Natl Acad Sci U S A 2011;108:3713-8. [Crossref] [PubMed]
- Shen J, Liao J, Guarnera MA, et al. Analysis of microRNAs in sputum to improve computed tomography for lung cancer diagnosis. J Thorac Oncol 2014;9:33-40. [Crossref] [PubMed]
- Tang D, Shen Y, Wang M, et al. Identification of plasma microRNAs as novel noninvasive biomarkers for early detection of lung cancer. Eur J Cancer Prev 2013;22:540-8. [Crossref] [PubMed]
- Heegaard NH, Schetter AJ, Welsh JA, et al. Circulating micro-RNA expression profiles in early stage nonsmall cell lung cancer. Int J Cancer 2012;130:1378-86. [Crossref] [PubMed]
- Hennessey PT, Sanford T, Choudhary A, et al. Serum microRNA biomarkers for detection of non-small cell lung cancer. PLoS One 2012;7:e32307. [Crossref] [PubMed]
- Leng Q, Lin Y, Jiang F, et al. A plasma miRNA signature for lung cancer early detection. Oncotarget 2017;8:111902-11. [Crossref] [PubMed]
- McShane LM, Altman DG, Sauerbrei W, et al. Reporting recommendations for tumor marker prognostic studies. J Clin Oncol 2005;23:9067-72. [Crossref] [PubMed]
- Pepe MS, Feng Z, Janes H, et al. Pivotal evaluation of the accuracy of a biomarker used for classification or prediction: standards for study design. J Natl Cancer Inst 2008;100:1432-8. [Crossref] [PubMed]
- Cohen J, Bossuyt P, Levy C, et al. How to use the STARD statement and the QUADAS-2 tool? Arch Pediatr 2015;22:190-1. [Crossref] [PubMed]
- Aerts HJ, Velazquez ER, Leijenaar RT, et al. Decoding tumour phenotype by noninvasive imaging using a quantitative radiomics approach. Nat Commun 2014;5:4006. [Crossref] [PubMed]
- Fang M, Huang W, Wu X, et al. MiR-141-3p suppresses tumor growth and metastasis in papillary thyroid cancer via targeting yin yang 1. Anat Rec (Hoboken) 2019;302:258-68. [Crossref] [PubMed]
- Xu S, Ge J, Zhang Z, et al. miR-141 inhibits prostatic cancer cell proliferation and migration, and induces cell apoptosis via targeting of RUNX1. Oncol Rep 2018;39:1454-60. [PubMed]
- Mei Z, He Y, Feng J, et al. MicroRNA-141 promotes the proliferation of non-small cell lung cancer cells by regulating expression of PHLPP1 and PHLPP2. FEBS Lett 2014;588:3055-61. [Crossref] [PubMed]
- Hu H, Li S, Liu J, et al. MicroRNA-193b modulates proliferation, migration, and invasion of non-small cell lung cancer cells. Acta Biochim Biophys Sin (Shanghai) 2012;44:424-30. [Crossref] [PubMed]
- Sun L, He M, Xu N, et al. Regulation of RAB22A by mir-193b inhibits breast cancer growth and metastasis mediated by exosomes. Int J Oncol 2018;53:2705-14. [PubMed]