Challenges in the target volume definition of lung cancer radiotherapy
Introduction
Radiotherapy with or without systemic treatment has an important role in the management of lung cancer. This treatment involves the precise delivery of ionising radiation to the tumour, with the aim to minimise the dose to normal tissue and hence reduce treatment side effects. Accurate definition of the treatment area is one of the most important steps in high-precision radiotherapy. This process involves defining the gross visible tumour volume (GTV) on computed tomography images. Margins are added around the GTV to account for microscopic disease, as well as random and systematic set-up errors to form the planning target volume (PTV) (1).
Failure to define the GTV accurately will, therefore, result in a systematic error and lower the precision of the overall radiotherapy workflow. Ironically, the definition of the GTV has also been described as the ‘weakest link’ in the radiotherapy treatment chain (1). Numerous studies have shown that this process is prone to interobserver variation and human errors, particularly for lung cancer (2-7). Lung tumours are often surrounded by interstitial lung tissue changes or atelectasis that look similar to the tumour making it difficult to distinguish tumour boundaries (Figure 1). Furthermore, the definition of the GTV requires the clinician to make complex judgements based on the patient’s clinical history, diagnostic images, and anatomical knowledge to identify the target and potential routes of spread.
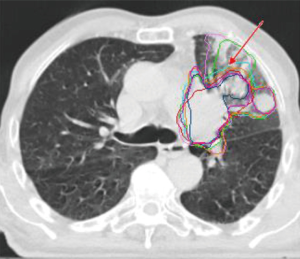
Another important limitation is that the final GTV delineation represents a snapshot of the tumour shape and position in time. The tumour can change during the course of treatment as a result of changes in respiratory motion, tumour baseline shifts, regression and progression and anatomical changes caused by pleural effusion and infiltrative changes (9). Large safety margins are required to account for this uncertainty, potentially limiting dose escalation. Image-guided radiotherapy has, therefore, a crucial role in identifying these changes during treatment and various techniques have been proposed to adapt the treatment accordingly.
In this article, the extent of this problem will be discussed together with techniques that could be used to reduce uncertainties in target volume delineation.
Quantifying interobserver variation
Interobserver variation in the definition of the GTV can be classified as minor or major (10). Minor interobserver variation includes small deviations caused by the difficulty to outline “fuzzy” tumour boundaries on the images using the contouring tools available. Major variations are clinically significant changes that may lead to a geographical tumour miss or unnecessary dose to healthy tissue. These are generally caused by differences in image interpretation and human errors, for example, by failing to contour involved lymph nodes or tumour extensions (4,10).
Various metrics have been proposed to quantify interobserver variation in relation to a gold standard including; simple volume and volume overlap measurements, the centre of mass, measures of surface shape variations and dosimetric analysis (11-13). A summary of these metrics, together with their advantages and limitations, is provided in Table S1. The accuracy of these metrics is case dependent and may not always reveal the impact of interobserver variation on the dose to the tumour, organs at risk (OARs) and ultimately, clinical outcomes (14). Furthermore, the lack of an absolute gold standard makes it difficult to accurately validate the accuracy of a delineated contour (10,15,16). It is, therefore recommended to use more than one metric to quantify interobserver variation (17). A qualitative assessment can also be performed whereby an expert or expert panel visually evaluates the contours and classify these as acceptable or unacceptable according to a consensus delineation protocol (9,16,18). The limitation of the latter approach is that it is subjective and time consuming (19). However, when used alongside other quantitative metrics, a qualitative assessment can provide a better understanding of the factors leading to interobserver variation.
Factors contributing to interobserver variation in lung cancer
Numerous studies have been conducted to assess the interobserver variation in lung cancer (3-7,20,21). These are summarised in Table 1 based on the number observer participating, case evaluated, methods used to analyse the data and factors contributing to interobserver variation. Comparison between studies is difficult as different metrics are used to analyse interobserver variation. The distance to a reference contour is one of the most commonly used metrics with studies reporting a distance ranging between 1.5 and 2.6 mm for early stage lung cancer treated with SBRT, up to 19mm for more advanced cases in particularly for tumours surrounded by atelectasis and for lymph nodes (3,5,7,20,27). Apart from case specific difficulties, other factors have been found to contribute to interobserver variation including; protocol violations, interpretational differences and human errors (5,6,19,21,28). These variations were found to have an impact on the dose to the PTV and normal tissue and ultimately on tumour control probability (TCP) and normal tissue complications probability (NTCP) (12,19,22). Protocol violations have been linked to worse survival in the CONVERT and PROCLAIM lung clinical trials (26,29) as well as other sites (30). Lack of experience, training and professional background has also been found to contribute to interobserver variation (20,22,23).
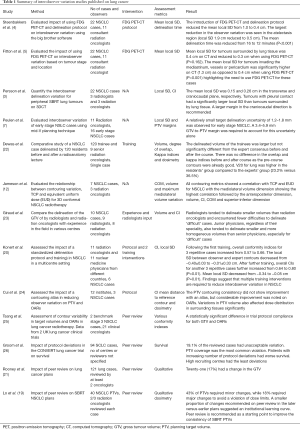
Full table
Optimising the definition of the GTV
Although interobserver variation in the definition of the GTV can be classified as a systematic error it is difficult to account for this variation through the use of margins since this variation is often not uniform, case depended and way too large in particularly for interpretational differences leading to an unacceptably large margin.
In view of this, various methods have been proposed in the literature to reduce the interobserver variation in target volume definition including; use of clearer protocols (4,20,31), inclusion of multimodality images (6,28), autosegmentation (32-34), respiratory motion management (35), training (20), and the introduction of peer review checks (18,19,21,25,36) (Table 1).
Multimodality images for target definition
CT is still considered to be the gold standard imaging modality in lung radiotherapy as it provides both 3D anatomical information and tissue densities, necessary for dose calculation. However, the contrast between tumour surrounding soft tissue and malignant changes is often limited.
When using 3DCT, a margin is added around the CTV to account for respiratory tumour motion to form the internal target volume. This margin is based on population respiratory motion data. It does not account for the patient’s individual respiratory motion, potentially leading to either an overestimation or an underestimation of the margin required to account for this uncertainty (37). These limitations can be overcome by improving the contrast and spatial resolution on CT. Additional imaging modalities including; positron emission computed tomography (PET-CT), magnetic resonance imaging (MRI), and/or respiratory correlated computed tomography (4DCT) also have an important role.
With the introduction of multimodality imaging, however, there is a need for improved protocols and collaboration between oncologists, radiologists, and nuclear medicine physicians (20,22). Most radiotherapy centres do not have “dedicated” PET-CT and MRI scanners that allow scanning of the patient in the treatment position for radiotherapy planning and therefore, a planning CT is required. When these images are not acquired with the patient in the treatment position mis-registration between the diagnostic images and planning CT is likely making it difficult to identify corresponding structures on the planning CT leading to misinterpretation. Hence, maintaining clear patient set-up and imaging protocols is essential to facilitate the use of multiple images. Furthermore, since the tumour can change over the course of treatment, image-guided radiotherapy can be used to identify the changes and adapt the treatment accordingly.
Improving the CT spatial and contrast resolution
Intravenous iodine contrast can be used to improve the contrast between the tumour tissue and blood vessels. However, due to underlying co-morbidities, not all patients can tolerate intravenous contrast (38). Diagnostic high-resolution CT scan can be used alongside treatment planning CT scans to improve the assessment of interstitial lung disease and lymph node involvement (39).
Role of FDG PET-CT
The tumour activity can be quantified by measuring the standard uptake value (SUV) of radioactive tracer on the PET-CT within a predefined region of interest (40). The PET image provides biological information but very limited anatomical detail. To overcome this problem, a CT is also acquired that is inherently registered (spatially aligned) with the PET to obtain anatomical information. The use of FDG PET-CT in radiotherapy has been shown to reduce interobserver variation, especially when defining tumours surrounded by atelectasis (4,5). Furthermore, it facilitates the detection of both metastatic lymph nodes and distant metastasis hence improve staging accuracy (Figure 2) (41,42). However, FDG PET-CT also has a number of limitations. PET has a low spatial resolution and can not detect very small nodules (<1 cm). False negatives and positives may occur in diabetic patients with high blood glucose levels at the time of scanning. Increased FDG uptake is observed in many non-neoplastic lesions, granulation tissue (e.g., wound healing), infections and other inflammatory processes, eventually resulting in false negatives and false positives (43).
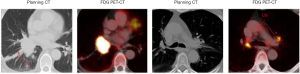
Role of MRI
MRI in lung cancer radiotherapy is mainly used to delineate Pancoast tumours a particular type of lung tumour located in the upper lobes of the lungs that tend to spread into the chest wall and nerves. The use of MRI in lung cancer radiotherapy is currently limited by the lack of tissue density information required for dose calculations, the low proton density of the lung tissue and motion artefacts introduced by the long duration of the scan. New imaging sequences are currently being developed to facilitate the introduction of MRI in lung cancer radiotherapy triggered by the development of the MRI guided adaptive radiotherapy (44,45).
Role of 4DCT
4DCT can be used to account for the patient’s individual tumour motion. With this technique, the respiratory cycle is measured using devices such as an abdominal belt or infrared marker. A large number of CT images are then acquired and correlated with the breathing cycle. These images are then sorted during reconstruction into 8 to 10 equal respiratory bins with each bin representing either a specific phase (from 0 to 100%) or amplitude position of the respiratory cycle (37).
While 4DCT can be used to account for the patient’s individual respiratory motion, it also introduces new challenges. The 4DCT is typically not used to calculate the dose distribution, and therefore a 3DCT is reconstructed from this data. Furthermore, 4DCT imaging is prone to motion artefacts, particularly in patients with irregular breathing patterns (46). This occurs due to a mismatch between the data acquisition and respiratory phases. Several methods have been proposed to reduce these artefacts, including improvements in signal acquisition, gating, sorting and post-processing techniques.
Visual and audio respiratory coaching can be used to regularise the breathing pattern and hence reduce these artefacts (47,48). However, the reported effectiveness of these techniques varies among patients. They are also time consuming and complex to implement clinically (47). Alternatively, the CT images can be acquired only at specific phases or amplitudes of the respiratory cycle (gating) and therefore, data from irregular breathing patterns is excluded. While gating reduces the number of artefacts, it comes at the cost of prolonging the scanning time.
Image sorting can be performed based on either the respiratory phase or amplitude. Amplitude sorting is less affected by outliers in the breathing cycle unless there are gaps in the respiratory signal (49). Furthermore sorting based on the movement of internal anatomy such as the diaphragm rather than external surrogates was found to reduce artefacts as it is more likely to represent the true internal anatomical movement (50). Alternatively, image post-processing techniques can be used to reduce artefacts (51).
Respiratory motion management
Several methods can be used to account for respiratory motion including; gating, tracking, internal target volume (ITV), mid-ventilation (Mid-V) and mid-position (Mid-P) (38).
Gating involves delivering the treatment only during specific amplitudes or phases within the respiratory cycle. Tracking involves continuously aligning and reshaping the radiation beam in real-time to account for variations in tumour position (37). However, while these techniques result in a very small PTV, they are complex and time consuming to implement clinically and therefore not widely used (37).
The ITV technique involves defining the CTV on either all or a selection of the 4DCT breathing phases. The ITV is then determined to be the envelope of motion of the CTV. When using this technique, the CTV has to be defined multiple times, making the delineation process time consuming (Figure 3). An alternative approach is to reconstruct the 4DCT image into a 3DCT that represents the full tumour motion (52). The GTV is delineated, and a margin is added to account for microscopic spread to form the ITV. Since the delineated GTV on the reconstructed 4DCT includes the tumour motion, it is referred to as the internal gross target volume (IGTV).
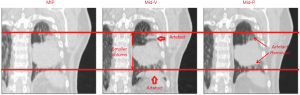
The maximum intensity projection (MIP) is one of the most commonly used reconstruction techniques (37). The MIP displays the highest density value encountered along the viewing ray for each pixel of volumetric data throughout the respiratory cycle (53,54). As such, these projections overlay all the CT phases and eventually represent the tumour position throughout the whole respiratory cycle. Delineations on the MIP generally show a good agreement with ITV generated from the 4DCT (54). However, the MIP reconstructed image is blurry making it difficult to distinguish the boundaries between tumour and tissue of equal tissue density such as blood vessels, diaphragm or mediastinum (53) potentially increasing the delineation uncertainty. Moreover, the ITV technique tends to overestimate the size of the PTV (35).
To overcome these issues, The Netherlands Cancer Institute (NKI), developed two new 4DCT image reconstruction techniques; Mid-Ventilation (Mid-V), and Mid-position (35,55). The Mid-V technique selects the frame of the 4D acquisition, where the tumour is closest to its mean time-weighted position. This frame can be selected visually or using rigid registration algorithms. The mid-position (Mid-P) technique uses deformable image registration to reconstruct every part of the anatomy in every frame to its average time-weighted mean position and then combines all frames.
The advantage of using the Mid-V and Mid-P over the MIP technique is that respiratory motion is decoupled from the GTV definition and is taken into account as a random error to be combined quadratically with other error sources and not linearly (55). These methods result in generally a smaller PTV (about 33% smaller), eventually sparing normal tissue (35,56). Peulen et al. (56), reported that the Mid-V technique was safely and easily implemented clinically at NKI with a 2-year local control rate of 98% for patients treated with SBRT (n=297). However, more clinical trials are required to assess the impact of using different motion management techniques on clinical outcomes.
Moreover, since the Mid-P reconstruction does not depend on a single frame, the motion artefacts are reduced, potentially facilitating the delineation process (51,55). However, this improvement comes at the cost of a somewhat reduced spatial resolution (Figure 3).
Mercieca et al. (2), compared the impact of using these three image reconstructions on interobserver variation in lung cancer. The overall difference in interobserver variation between the MIP, Mid-V and Mid-P was small. The benefit of using the Mid-V and Mid-P was more prominent in some specific tumour interfaces including the lung, chest wall and regions with a large tumour motion. An advantage of using the Mid-V and Mid-P technique is that it does not require the observer to review the delineations on the 4DCT making it easier to define tumour boundaries resulting in reduced interobserver variation in regions with large tumour motion. There was no benefit in using the Mid-P for lymph node delineation due to interpretational differences when incorporating diagnostic data in the delineation.
Role of 4D FDG PET-CT
A limitation of 3D FDG PET-CT is that respiratory motion can degrade the quality of the images in particular for small tumours located close to the diaphragm that tends to be more mobile. This can eventually result in mis-registration between the PET and the CT leading to interpretational difference when defining the GTV and inaccurate attenuation correction. Furthermore, the SUV measurements are blurred, eventually leading to an inaccurate segmentation of the GTV. An alternative approach is to acquire the images using deep inspiration breath-hold. However, a study by Nygård et al. (57) found that the deep inspiration breath-hold scans did not have a clinically relevant impact on the uptake metrics and did not improve the test-retest repeatability of FDG uptake metrics in lung cancer patients when compared with free-breathing scans.
To overcome these issues, the use of 4D FDG PET-CT has been proposed. This technique improves the diagnostic accuracy in particularly for the detection of lymph nodes and small lung tumours (58), eventually reducing the interobserver variation in the definition of central lung target tumours (59). The benefit of using 4D FDG PET-CT for radiotherapy planning is also hampered by the long acquisition time eventually increase the chances of patient movement during the scan while also lowering the machine throughput. As a result, 4D FDG PET-CT is not commonly used in clinical practice. An alternative approach to 4D FDG PET-CT is the use of a motion-compensated Mid-P PET-CT scan as proposed by Kruis et al. (60). This technique could be used to reduce the blurring of the SUV signal improving the appearance of both tumour and boost volumes. However, this improvement was mainly noted for tumours with respiratory motion amplitude larger than 10 mm. Compared to a 3D PET scan, the lesions in the motion-compensated scans had higher SUV values and a smaller 50% SUVmax volumes, eventually altering the volume used in PET boost studies. Kruis et al. (60), also noted that an irregular breathing cycle could increase the number of artefacts.
Image-guided adaptive radiotherapy (ART)
With the integration of cone-beam computed tomography (CBCT) and MRI on the linear accelerator, it is now possible to identify intrathoracic anatomical changes prior to treatment and adapt the treatment accordingly if necessary. During adaptive radiotherapy, the planning CT is first registered with the localisation image, and any variations in the tumour and OAR shape and position are assessed. This is then followed by the application of an adaptive strategy. These strategies can be divided into two categories, ‘adapt-to-position’ (ATP) and ‘adapt-to-shape’ (ATS) (61). For ATP, rigid image registration is used to assess and account for variations in the isocentre position only (for e.g., by adjusting the couch position). On the other hand, for ATS strategies, deformable image registration is used to transfer anatomic contours and dose between the CBCT and planning CT images. This is used to assess dose deviations caused by the intrathoracic tumour and anatomical changes, providing guidance to when the dose distribution must be reoptimised. In general, contour propagation is followed by contour editing, creating a new source of inter- and intra-observer variation that has not received much attention yet.
Intrathoracic tumour and anatomical changes have been reported in 72% of NSCLC (9) with about a third requiring adaptive therapy to ensure tumour coverage and reduce lung dose (62). Replanning to account for tumour shrinkage may reduce the dose to normal tissue and hence reducing toxicity. However, replanning needs to be balanced against the risk of missing microscopic disease. The LARTIA trial investigated the failure pattern in locally advanced-NSCLC patients with an adaptive approach (63). A re-planning was performed based on tumour regression seen on weekly CBCT scans performed during treatment in 50 out of 217 patients. A 6% marginal relapse and low incidence of acute pulmonary and oesophagal toxicity (2% and 4% respectively) were reported in this study. Several studies indicated that tumour volume change during treatment might be predictive for treatment outcome (64,65) and hence might improve current baseline prediction models for treatment outcome. However, these findings were not confirmed in the large study by Kwint et al. (66) that found no correlation between tumour volume changes and overall survival. Their findings indicate that ART after primary tumour regression might be safe, but this approach needs further validation in prospective trials. Functional tumour information from MRI and PET-CT may also have an important role in developing prediction outcome models. Furthermore, the implementation of ART techniques in routine clinical practice still remains challenging. Adaptive treatment changes can be performed offline between treatments, online immediately prior to treatment delivery, or in real-time during treatment. Online and real-time adaptations improve treatment delivery accuracy, potentially allowing for margin reduction (62). However, these come at the cost of increasing the treatment time and may not be feasible for all tumours. On the other hand, the optimal time point and cutoff points for offline replanning are still not known and could be different for individual patients. Replanning is time consuming, and the accuracy of the dose evaluation depends on the accuracy of the deformable image registration and the accuracy of autosegmentation tools on CT, CBCT and MRI. The latter is currently limited for the definition of lung tumours (62).
The introduction of onboard MRI on the linac is opening new doors for adaptive radiotherapy in lung cancer. The MR linac allows for the acquisition of high-quality soft-tissue contrast images with functional information without using ionising radiation, allowing the oncologist to make daily treatment adaptation. Furthermore, MR-linacs now allow for cross-sectional beam-on imaging, making it possible to monitor tumour and organ at risk motion during treatment delivery without the need to use external surrogates or statistical respiratory models. This, together with the ability to acquire images in the sagittal and coronal plane results in higher image quality with less binning artefact, and more realistic motion estimation as the uncertainty from an imperfect external-internal surrogate is eliminated. Moreover, it also facilitates the use of gating and tumour tracking techniques.
Nevertheless, there are a number of challenges that need to be addressed for the clinical implementation of the MR-linac (67). Patient movement can increase as a result of the prolonged treatment time and the claustrophobic environment of the MRI. Workflows and imaging sequences need to be developed for radiotherapy purposes. Software also needs to be developed to account for the lack of tissue density information required for dose calculations and the time consuming step of contour propagation, editing and QA should be optimised, for instance by introducing simultaneous remote review. Ultimately, the clinical and cost-effectiveness of this technique must be proven with well designed clinical trials.
Auto-segmentation
Auto-segmentation involves converting an image into a collection of pixels that share the same characteristics such as intensity, shape or texture, thus facilitating the distinction between tumour and normal tissue. The advantage of incorporating FDG PET-CT into radiotherapy planning is that FDG tends to accumulate in cancer cells, thus facilitating tumour localisation and the development of auto-segmentation tools. On the other hand, CT based auto-segmentation tools are more complex to develop due to the poor contrast between tumour and adjacent soft tissue. Numerous semi-automatic and fully automated segmentation algorithms have been developed to facilitate this process, including algorithms based on thresholding, region growing, edge detection, statistical and machine learning algorithms (33,68). These algorithms tend to vary significantly in complexity, accuracy, degree of user intervention and availability.
Threshold algorithms are the simplest and most widely used (68). This technique defines the tumour by selecting all the image voxels above a certain SUV intensity threshold, usually the SUVmax (hottest pixel) within a pre-defined region of interest.
The development of auto-segmentation algorithms for FDG PET-CT remains challenging as the SUV measurements are affected by physiological factors such as body mass and plasma glucose, biological characteristics of the tumour, the low resolution of the PET images and variations in scan parameters (69). Shepherd et al. (33), reviewed 30 different segmentation algorithms used in 13 different institutions. The findings of this study indicate that manual contouring is still the most accurate. However, simple threshold segmentation algorithms performed well compared to more complex algorithms. Mercieca et al. (70) compared such segmentations with pathology data and concluded that the threshold algorithm on the maximum SUV or SUVpeak performed equally well. The provision of auto-segmentation tools followed by manual editing has been found to reduce contouring time and, interobserver variation, and correlated well with pathology data (32,33,71).
Machine and deep learning methods are also showing promising results for OARs delineations as the shape of these organs are similar for most patients (68). However, these algorithms are highly dependent on the accuracy of predefined contours. Since the shape and texture of lung tumours can vary significantly between patients, it is more difficult to develop these algorithms to delineate lung tumours. Moreover, the lack of reliable gold standards makes it difficult to validate the accuracy of these algorithms.
The main barrier for clinical implementation of machine and deep learning algorithms is the availability of high-quality clinical contouring data for training. This data is often stored in secure servers across a number of hospitals that are not linked. Improvements in workflows and logistics would be required in order to securely link all the patient data required to develop contouring databases (72). An important question is whether to include all the oncologists’ contours in the training database. Training of algorithms can be supervised whereby the algorithm learns from labelled datasets (i.e., good contours) or unsupervised whereby the algorithms tries to make sense of unlabelled data (i.e., not providing contours that have been peer-reviewed) by independently extracting features and patterns from the images.
Training of algorithms using non-reviewed physician contours can introduce a bias by the particular physician’s medical training, experience, goals, or misconceptions, eventually leading to an inaccurate segmentation (23,73). An extensive database is required to reduce the effects of major outliers, but this will also increase the computation time. This problem can be resolved through the use of supervised training data whereby only the contours that have been delineated using a specific protocol and peer-reviewed by experts are included in the database (72). Alternatively, only the contours from patients that had acceptable local control rates and toxicity could be used to develop the training database. The latter would automatically exclude cases whereby the tumour recurred as a result of a geographical miss or cases that had unacceptable toxicities due to an excessive inclusion of normal tissue. The limitation of this approach is that it still requires a manual intervention to label the data making it time consuming to develop the algorithm. Also, cases where the PTV coverage is compromised due to proximity or OARs may need to be excluded.
Delineation protocols
Numerous consensus delineation guidelines (38,74,75) have been published by professional bodies providing detailed information to facilitate the interpretation of clinical information, diagnostic images and biopsies necessary to define the GTVp and GTVln. These protocols also provide information on the process that should be followed to define the GTV such as setting the optimal window/level on CT based on the tumour location and provide guidelines on how to incorporate diagnostic images to facilitate the definition of the GTV.
For the definition of the lymph node GTV, the European Society of Radiotherapy and Oncology together with the Advisory Committee in Radiation Oncology Practice (ESTRO-ACROP) (38,75) proposed an algorithm that could be used to identify the lymph nodes that should be included in the GTV based on the diagnostic CT, FDG PET-CT and biopsy information.
Elective lymph node irradiation is no longer recommended as this procedure leads to increased toxicity while it also limits dose escalation (38,76,77). The ESTRO-ACROP guidelines identified two acceptable methods that can be used to determine the boundary for the GTVln (38). The GTVln can be defined by either defining the positive lymph node with an 8mm expansion to account for microscopic spread or by defining the entire lymph node station.
Both lymph node delineation methods have been used in large multicentre clinical trials without unacceptable out-field mediastinal recurrence rates (38). However, the definition of the lymph node station results in a larger GTV when compared with defining only the involved node, potentially increasing the toxicity for the patient. Anatomical atlases illustrating the location of specific lymph node stations and how to define the GTV for specific cases have also been developed (74,78,79).
Studies have shown that guidelines can reduce the interobserver variability in the definition of the GTV and OARs in lung cancer (53,61). However, significant interobserver variation remains even amongst experts (24), and therefore training is essential to ensure the correct interpretation and application of these guidelines in routine clinical practice. It is essential to acknowledge that the use of different protocols between different centres may also result in variations when defining the GTV, thus highlighting the need to harmonise protocols. Moreover, protocols may not always provide guidance for all clinical scenarios, and hence discussion of difficult cases in a multidisciplinary team is recommended.
Training
Vinod et al. (80) evaluated the impact of several training programmes on reducing interobserver variation. The impact of training varied across studies as the delivery method as well as the target audience varied. Larger group didactic lectures did not have a significant impact on interobserver variation while courses that had a practical component and provided individual feedback were reported to be more effective in reducing interobserver variation (80). An international delineation study conducted by Konert et al. (20), showed that more than one training intervention might be required in order to have a significant impact in reducing interobserver variation when delineating the GTV in lung cancer and eventually lead to a change to clinical practice. Mercieca et al. (81) compared individually made delineations to delineation made by group consensus, and showed that the latter had more improvement than training, illustrating the need for collaboration and peer review.
Peer review
Training and clear protocols are important to improve consistency in contouring. However, these may not necessarily lead to a change in routine clinical practice or eliminate human errors (20).
Furthermore, the task of defining the GTV requires a range of expertise from radiologists, physicist, radiographers and oncologists. Numerous studies have shown that peer review by a second oncologist or within a multidisciplinary team can reduce the interobserver variation in target volume definition and facilitate the identification of unacceptable gross errors (19,82,83). Studies have shown that when peer review is introduced, unacceptable errors are identified in about 17% of target volumes (19,21,84). These errors have been linked with worse survival in clinical trials (18,26).
As a result, several professional bodies have now issued guidelines to establish minimum standards for peer review as part of the Radiotherapy department’s quality assurance processes (10,38,85,86). Although these guidelines indicate that peer review is essential, it is not a common practice in many radiotherapy centres (10,38). Various barriers exist for the routine implementation of peer review in clinical practice including allocated time to review contours, shortage of staff, availability of radiology services, delays to start treatment, availability of workstations and appropriate software (10,87,88). Workflows and cases reviewed also varied widely across centres (87). Outcomes from peer-review should be clearly documented, and the data generated used to improve delineations protocols, training and the accuracy of autosegmentation tools.
Artificial intelligence could also be used to develop computer-assisted peer review software. Hui et al. (89) developed an algorithm that could be used to evaluate OARs in the thoracic region. In this study, the researchers simulated common delineation errors, including boundary deviations, missing slices, incorrect labelling, and craniocaudal over-extension for OARs in the thoracic region. The algorithm was able to detect 37% of the minor and 85% of the major errors. The reason for lack of precision in detecting minor errors was attributed to the fact that these errors were inconsistently judged by the reviewers. The use of this tool also improved the reviewers’ error detection sensitivity from 61% to 68% for minor errors and from 78% to 87% for major error. The findings of these studies suggest that such tools could be used to assist the oncologists in reviewing contours, but they should not be used to replace human judgement. Over-reliance on the system might end up becoming counterproductive and actually reduce the ability of the reviewer to identify errors. Further research is required to develop similar algorithms for lung tumours.
Conclusions
The findings of this review indicate that to date, it is still not possible to eliminate interobserver variation in the definition of GTV. Positron Emission Tomography (PET-CT) has an important role in improving the staging accuracy and the definition of the tumour. Various autosegmentation tools have also been proposed to fully or partially automate the delineation process. However, their development is currently hindered by the unavailability of absolute gold standards that can be used to validate these algorithms as well as the wide morphological and shape variations of lung tumours. Hence, manual delineation is still considered to be the gold standard. Nevertheless, auto-segmented contours can provide a good starting point, eventually reducing the delineation time and interobserver variation. Improvements in image quality can also reduce the delineation uncertainty in some cases. However, the main factor leading to interobserver variation is image interpretation differences between clinicians. Therefore, protocols, training and peer review of contours are essential to address this challenge.
Acknowledgments
Professor Marcel van Herk is supported by NIHR Manchester Biomedical Research Centre.
Funding: None.
Footnote
Provenance and Peer Review: This article was commissioned by the Guest Editors (Jacek Jassem and Rafal Dziadziuszko) for the series “Radiotherapy in thoracic malignancies” published in Translational Lung Cancer Research. The article was sent for external peer review organized by the Guest Editors and the editorial office.
Peer Review File: Available at http://dx.doi.org/10.21037/tlcr-20-627
Conflicts of Interest: All authors have completed the ICMJE uniform disclosure form (available at http://dx.doi.org/10.21037/tlcr-20-627). The series “Radiotherapy in thoracic malignancies” was commissioned by the editorial office without any funding or sponsorship. MvH reports being supported by the NIHR Manchester Biomedical Research Centre, outside the submitted work. The authors have no other conflicts of interest to declare.
Ethical Statement: The authors are accountable for all aspects of the work in ensuring that questions related to the accuracy or integrity of any part of the work are appropriately investigated and resolved.
Open Access Statement: This is an Open Access article distributed in accordance with the Creative Commons Attribution-NonCommercial-NoDerivs 4.0 International License (CC BY-NC-ND 4.0), which permits the non-commercial replication and distribution of the article with the strict proviso that no changes or edits are made and the original work is properly cited (including links to both the formal publication through the relevant DOI and the license). See: https://creativecommons.org/licenses/by-nc-nd/4.0/.
References
- International Commission of Radiation Units and Measurements (ICRU). ICRU Report 62: Prescribing, recording and reporting photon beam therapy (supplement to ICRU report 50). I. Bethesda, MD: 1999.
- Mercieca S, Belderbos JSA, De Jaeger K, et al. Interobserver variability in the delineation of the primary lung cancer and lymph nodes on different four-dimensional computed tomography reconstructions. Radiother Oncol 2018;126:325-32. [Crossref] [PubMed]
- Persson GF, Nygaard DE, Hollensen C, et al. Interobserver delineation variation in lung tumour stereotactic body radiotherapy. BJR 2012;85:e654-60. [Crossref] [PubMed]
- Steenbakkers RJHM, Duppen JC, Fitton I, et al. Reduction of observer variation using matched CT-PET for lung cancer delineation: A three-dimensional analysis. Int J Radiat Oncol Biol Phys 2006;64:435-48. [Crossref] [PubMed]
- Fitton I, Steenbakkers RJHM, Gilhuijs K, et al. Impact of Anatomical Location on Value of CT-PET Co-Registration for Delineation of Lung Tumors. Int J Radiat Oncol Biol Phys 2008;70:1403-7. [Crossref] [PubMed]
- Steenbakkers RJHM, Duppen JC, Fitton I, et al. Observer variation in target volume delineation of lung cancer related to radiation oncologist-computer interaction: A ‘Big Brother’ evaluation. Radiother Oncol 2005;77:182-90. [Crossref] [PubMed]
- Peulen H, Belderbos J, Guckenberger M, et al. Target delineation variability and corresponding margins of peripheral early stage NSCLC treated with stereotactic body radiotherapy. Radiother Oncol 2015;114:361-6. [Crossref] [PubMed]
- Mercieca S, Belderbos J, van Herk MB. Optimising the definition of the target volume in lung cancer radiotherapy. University of Amsterdam; 2020.
- Kwint M, Conijn S, Schaake E, et al. Intra thoracic anatomical changes in lung cancer patients during the course of radiotherapy. Radiother Oncol 2014;113:392-7. [Crossref] [PubMed]
- The Royal College of Radiologists. Radiotherapy target volume definition and peer review RCR guidance 2017: 1-35. Available online: https: //www.rcr.ac.uk/publication/radiotherapy-target-volume-definition-andpeer-review (accessed 15 May 2018).
- Vinod SK, Jameson MG, Min M, et al. Uncertainties in volume delineation in radiation oncology: A systematic review and recommendations for future studies. Radiother Oncol 2016;121:169-79. [Crossref] [PubMed]
- Jameson MG, Kumar S, Vinod SK, et al. Correlation of contouring variation with modeled outcome for conformal non-small cell lung cancer radiotherapy. Radiother Oncol 2014;112:332-6. [Crossref] [PubMed]
- Taha AA, Hanbury A. Metrics for evaluating 3D medical image segmentation: analysis, selection, and tool. BMC Med Imaging 2015;15:29. [Crossref] [PubMed]
- Kristensen I, Nilsson K, Agrup M, et al. A dose based approach for evaluation of inter-observer variations in target delineation. Tech Innov Patient Support Radiat Oncol 2017;3-4:41-7. [Crossref] [PubMed]
- Kirov AS, Fanchon LM. Pathology-validated PET image data sets and their role in PET segmentation. Clin Transl Imaging 2014;2:253-67. [Crossref]
- Gwynne S, Gilson D, Dickson J, et al. Evaluating Target Volume Delineation in the Era of Precision Radiotherapy: FRCR, Revalidation and Beyond. Clin Oncol (R Coll Radiol) 2017;29:436-8. [Crossref] [PubMed]
- Hanna GG, Hounsell AR, O’Sullivan JM. Geometrical analysis of radiotherapy target volume delineation: a systematic review of reported comparison methods. Clin Oncol (R Coll Radiol) 2010;22:515-25. [Crossref] [PubMed]
- Cox S, Cleves A, Clementel E, et al. Impact of deviations in target volume delineation - Time for a new RTQA approach? Radiother Oncol 2019;137:1-8. [Crossref] [PubMed]
- Lo AC, Liu M, Chan E, et al. The Impact of Peer Review of Volume Delineation in Stereotactic Body Radiation Therapy Planning for Primary Lung Cancer: A Multicenter Quality Assurance Study. J Thorac Oncol 2014;9:527-33. [Crossref] [PubMed]
- Konert T, Vogel WV, Everitt S, et al. Multiple training interventions significantly improve reproducibility of PET/CT-based lung cancer radiotherapy target volume delineation using an IAEA study protocol. Radiother Oncol 2016;121:39-45. [Crossref] [PubMed]
- Rooney KP, McAleese J, Crockett C, et al. The Impact of Colleague Peer Review on the Radiotherapy Treatment Planning Process in the Radical Treatment of Lung Cancer. Clin Oncol (R Coll Radiol) 2015;27:514-8. [Crossref] [PubMed]
- Dewas S, Bibault JE, Blanchard P, et al. Delineation in thoracic oncology: A prospective study of the effect of training on contour variability and dosimetric consequences. Radiat Oncol 2011;6:118. [Crossref] [PubMed]
- Giraud P, Elles S, Helfre S, et al. Conformal radiotherapy for lung cancer: Different delineation of the gross tumor volume (GTV) by radiologists and radiation oncologists. Radiother Oncol 2002;62:27-36. [Crossref] [PubMed]
- Cui Y, Chen W, Kong FM, et al. Contouring variations and the role of atlas in non-small cell lung cancer radiation therapy: Analysis of a multi-institutional preclinical trial planning study. Pract Radiat Oncol 2015;5:e67-75. [Crossref] [PubMed]
- Tsang Y, Hoskin P, Spezi E, et al. Assessment of contour variability in target volumes and organs at risk in lung cancer radiotherapy. Tech Innov Patient Support Radiat Oncol 2019;10:8-12. [Crossref] [PubMed]
- Groom N, Wilson E, Faivre-Finn C. OA 01.05 Analysis of Radiotherapy Quality Assurance Data for the Convert Trial - Does Non-Compliance to Protocol Affect Survival? J Thorac Oncol 2017;12:S1745. [Crossref]
- Rasch CR, Steenbakkers RJ, Fitton I, et al. Decreased 3D observer variation with matched CT-MRI, for target delineation in Nasopharynx cancer. Radiat Oncol 2010;5:21. [Crossref] [PubMed]
- Fitton I, Duppen JC, Steenbakkers RJHM, et al. Impact of coronal and sagittal views on lung gross tumor volume delineation. Phys Med 2016;32:1082-7. [Crossref] [PubMed]
- Brade AM, Wenz F, Koppe F, et al. Radiation Therapy Quality Assurance (RTQA) of Concurrent Chemoradiation Therapy for Locally Advanced Non-Small Cell Lung Cancer in the PROCLAIM Phase 3 Trial. Int J Radiat Oncol Biol Phys 2018;101:927-34. [Crossref] [PubMed]
- Ohri N, Shen X, Dicker AP, et al. Radiotherapy Protocol Deviations and Clinical Outcomes: A Meta-analysis of Cooperative Group Clinical Trials. J Natl Cancer Inst 2013;105:387-93. [Crossref] [PubMed]
- Spoelstra FOB, Senan S, Péchoux C, Le , et al. Variations in Target Volume Definition for Postoperative Radiotherapy in Stage III Non-Small-Cell Lung Cancer: Analysis of an International Contouring Study. Int J Radiat Oncol Biol Phys 2010;76:1106-13. [Crossref] [PubMed]
- van Baardwijk A, Bosmans G, Boersma L, et al. PET-CT-Based Auto-Contouring in Non-Small-Cell Lung Cancer Correlates With Pathology and Reduces Interobserver Variability in the Delineation of the Primary Tumor and Involved Nodal Volumes. Int J Radiat Oncol Biol Phys 2007;68:771-8. [Crossref] [PubMed]
- Shepherd T, Teras M, Beichel RR, et al. Comparative Study With New Accuracy Metrics for Target Volume Contouring in PET Image Guided Radiation Therapy. IEEE Trans Med Imaging 2012;31:2006-24. [Crossref] [PubMed]
- Hatt M, Laurent B, Ouahabi A, et al. The first MICCAI challenge on PET tumor segmentation. Med Image Anal 2018;44:177-95. [Crossref] [PubMed]
- Wolthaus JWHH, Sonke JJ, van Herk M, et al. Comparison of Different Strategies to Use Four-Dimensional Computed Tomography in Treatment Planning for Lung Cancer Patients. Int J Radiat Oncol Biol Phys 2008;70:1229-38. [Crossref] [PubMed]
- Rasch C, Belderbos J, van Giersbergen A, et al. The Influence of a Multi-disciplinary Meeting for Quality Assurance on Target Delineation in Radiotherapy Treatment Preparation. Int J Radiat Oncol 2009;75:S452-3. [Crossref]
- Cole AJ, Hanna GG, Jain S, et al. Motion Management for Radical Radiotherapy in Non-small Cell Lung Cancer. Clin Oncol (R Coll Radiol) 2014;26:67-80. [Crossref] [PubMed]
- Nestle U, De Ruysscher D, Ricardi U, et al. ESTRO ACROP guidelines for target volume definition in the treatment of locally advanced non-small cell lung cancer. Radiother Oncol 2018;127:1-5. [Crossref] [PubMed]
- Elicker BM, Kallianos KG, Henry TS. The role of high-resolution computed tomography in the follow-up of diffuse lung disease. Eur Respir Rev 2017;26:170008 [Crossref] [PubMed]
- Lasnon C, Desmonts C, Quak E, et al. Harmonizing SUVs in multicentre trials when using different generation PET systems: prospective validation in non-small cell lung cancer patients. Eur J Nucl Med Mol Imaging 2013;40:985-96. [Crossref] [PubMed]
- Ambrosini V, Fanti S, Chengazi VU, et al. Diagnostic accuracy of FDG PET/CT in mediastinal lymph nodes from lung cancer. Eur J Radiol 2014;83:1301-2. [Crossref] [PubMed]
- Li J, Xu W, Kong F, et al. Meta-analysis: Accuracy of 18FDG PET-CT for distant metastasis staging in lung cancer patients. Surg Oncol 2013;22:151-5. [Crossref] [PubMed]
- Boellaard R, Delgado-Bolton R, Oyen WJG, et al. FDG PET/CT: EANM procedure guidelines for tumour imaging: version 2.0. Eur J Nucl Med Mol Imaging 2015;42:328-54. [Crossref] [PubMed]
- Kumar S, Liney G, Rai R, et al. Magnetic resonance imaging in lung: a review of its potential for radiotherapy. Br J Radiol 2016;89:20150431 [Crossref] [PubMed]
- Bainbridge H, Salem A, Tijssen RHN, et al. Magnetic resonance imaging in precision radiation therapy for lung cancer. Transl Lung Cancer Res 2017;6:689. [Crossref] [PubMed]
- Persson GF, Nygaard DE, Brink C, et al. Deviations in delineated GTV caused by artefacts in 4DCT. Radiother Oncol 2010;96:61-6. [Crossref] [PubMed]
- Persson GF, Nygaard DE, Olsen M, et al. Can audio coached 4D CT emulate free breathing during the treatment course? Acta Oncol 2008;47:1397-405. [Crossref] [PubMed]
- Sano N, Saito M, Onishi H, et al. Audio-Visual Biofeedback for Respiratory Motion Management: Comparison of the Reproducibility of Breath-Holding between Visual and Audio Guidance. J Mod Phys 2018;09:2286-94. [Crossref]
- Abdelnour AF, Nehmeh SA, Pan T, et al. Phase and amplitude binning for 4D-CT imaging. Phys Med Biol 2007;52:3515-29. [Crossref] [PubMed]
- Kruis MF, Van De Kamer JB, Belderbos JSA, et al. 4D CT amplitude binning for the generation of a time-averaged 3D mid-position CT scan. Phys Med Biol 2014;59:5517-29. [Crossref] [PubMed]
- Wolthaus JW, Sonke JJ, van Herk M, et al. Reconstruction of a time-averaged midposition CT scan for radiotherapy planning of lung cancer patients using deformable registration. Med Phys 2008;35:3998-4011. [Crossref] [PubMed]
- Underberg RW, Lagerwaard FJ, Slotman BJ, et al. Use of maximum intensity projections (MIP) for target volume generation in 4DCT scans for lung cancer. Int J Radiat Oncol Biol Phys 2005;63:253-60. [Crossref] [PubMed]
- Muirhead R, McNee SG, Featherstone C, et al. Use of Maximum Intensity Projections (MIPs) for Target Outlining in 4DCT Radiotherapy Planning. J Thorac Oncol 2008;3:1433-8. [Crossref] [PubMed]
- Slotman BJ, Lagerwaard FJ, Senan S. Acta Oncologica 4D imaging for target definition in stereotactic radiotherapy for lung cancer. Acta Oncol (Madr) 2009;45:966-72. [Crossref]
- Wolthaus JWH, Schneider C, Sonke JJ, et al. Mid-ventilation CT scan construction from four-dimensional respiration-correlated CT scans for radiotherapy planning of lung cancer patients. Int J Radiat Oncol Biol Phys 2006;65:1560-71. [Crossref] [PubMed]
- Peulen H, Belderbos J, Rossi M, et al. Mid-ventilation based PTV margins in Stereotactic Body Radiotherapy (SBRT): A clinical evaluation. Radiother Oncol 2014;110:511-6. [Crossref] [PubMed]
- Nygård L, Aznar MC, Fischer BM, et al. Repeatability of FDG PET/CT metrics assessed in free breathing and deep inspiration breath hold in lung cancer patients. Am J Nucl Med Mol Imaging 2018;8:127-36. [PubMed]
- Frood R, McDermott G, Scarsbrook A. Respiratory-gated PET/CT for pulmonary lesion characterisation-promises and problems. Br J Radiol 2018;91:20170640 [Crossref] [PubMed]
- Chirindel A, Adebahr S, Schuster D, et al. Impact of 4D-18FDG-PET/CT imaging on target volume delineation in SBRT patients with central versus peripheral lung tumors. Multi-reader comparative study. Radiother Oncol 2015;115:335-41. [Crossref] [PubMed]
- Kruis MF, van de Kamer JB, Houweling AC, et al. PET Motion Compensation for Radiation Therapy Using a CT-Based Mid-Position Motion Model: Methodology and Clinical Evaluation. Int J Radiat Oncol Biol Phys 2013;87:394-400. [Crossref] [PubMed]
- Winkel D, Bol GH, Kroon PS, et al. Adaptive radiotherapy: The Elekta Unity MR-linac concept. Clin Transl Radiat Oncol 2019;18:54-9. [Crossref] [PubMed]
- Zhong H, Jy J. Recent Advances and Challenges in Adaptive Radiotherapy for Patients with Locally Advanced NSCLC. Ann Radiat Ther Oncol 2017;1:1-5. [Crossref]
- Ramella S, Fiore M, Silipigni S, et al. Local Control and Toxicity of Adaptive Radiotherapy Using Weekly CT Imaging: Results from the LARTIA Trial in Stage III NSCLC. J Thorac Oncol 2017;12:1122-30. [Crossref] [PubMed]
- Jabbour SK, Kim S, Haider SA, et al. Reduction in tumor volume by cone beam computed tomography predicts overall survival in non-small cell lung cancer treated with chemoradiation therapy. Int J Radiat Oncol Biol Phys 2015;92:627-33. [Crossref] [PubMed]
- Wald P, Mo X, Barney C, et al. Prognostic Value of Primary Tumor Volume Changes on kV-CBCT during Definitive Chemoradiotherapy for Stage III Non-Small Cell Lung Cancer. J Thorac Oncol 2017;12:1779-87. [Crossref] [PubMed]
- Kwint M, Stam B, Proust-Lima C, et al. The prognostic value of volumetric changes of the primary tumor measured on Cone Beam-CT during radiotherapy for concurrent chemoradiation in NSCLC patients. Radiother Oncol 2020;146:44-51. [Crossref] [PubMed]
- van Herk M, Mcwilliam A, Dubec M, et al. Magnetic Resonance Imaging-Guided Radiation Therapy: A Short Strengths, Weaknesses, Opportunities, and Threats Analysis. Int J Radiat Oncol Biol Phys 2018;101:1057-60. [Crossref] [PubMed]
- Hatt M, Lee JA, Schmidtlein CR, et al. Classification and evaluation strategies of auto-segmentation approaches for PET: Report of AAPM task group No 211. Med Phys 2017;44:e1-42. [Crossref] [PubMed]
- Foster B, Bagci U, Mansoor A, et al. A review on segmentation of positron emission tomography images. Comput Biol Med 2014;50:76-96. [Crossref] [PubMed]
- Mercieca S, Belderbos J, van Loon J, et al. Comparison of SUVmax and SUVpeak based segmentation to determine primary lung tumour volume on FDG PET-CT correlated with pathology data. Radiother Oncol 2018;129:227-33. [Crossref] [PubMed]
- Obara P, Liu H, Wroblewski K, et al. Quantification of metabolic tumor activity and burden in patients with non-small-cell lung cancer: Is manual adjustment of semiautomatic gradient-based measurements necessary? Nucl Med Commun 2015;36:782-9. [Crossref] [PubMed]
- Nikolov S, Blackwell S, Mendes R, et al. Deep learning to achieve clinically applicable segmentation of head and neck anatomy for radiotherapy. Arxiv 2018;abs/1809.0.
- Boon IS, Au Yong TPT, Boon CS. Assessing the Role of Artificial Intelligence (AI) in Clinical Oncology: Utility of Machine Learning in Radiotherapy Target Volume Delineation. Medicines (Basel) 2018;5:131. [Crossref] [PubMed]
- Radiotherapy Oncology Group. Lung Atlas: RTOG 1106 Target Atlas 2019. Available online: https: //www.rtog.org/CoreLab/ContouringAtlases/LungAtlas.aspx (accessed 22 March 2018).
- Peeters ST, Dooms C, Van Baardwijk A, et al. Selective mediastinal node irradiation in non-small cell lung cancer in the IMRT/VMAT era: How to use E(B)US-NA information in addition to PET-CT for delineation? Radiother Oncol 2016;120:273-8. [Crossref] [PubMed]
- Li R, Yu L, Lin S, et al. Involved field radiotherapy (IFRT) versus elective nodal irradiation (ENI) for locally advanced non-small cell lung cancer: a meta-analysis of incidence of elective nodal failure (ENF). Radiat Oncol 2016;11:124. [Crossref] [PubMed]
- Yuan S, Sun X, Li M, et al. A Randomized Study of Involved-Field Irradiation Versus Elective Nodal Irradiation in Combination With Concurrent Chemotherapy for Inoperable Stage III Non small Cell Lung Cancer. Am J Clin Oncol 2007;30:239-44. [Crossref] [PubMed]
- Cohen JG, Reymond E, Jankowski A, et al. Lung adenocarcinomas: correlation of computed tomography and pathology findings. Diagn Interv Imaging 2016;97:955-63. [Crossref] [PubMed]
- Smithius R, International Association for the Study of Lung Cancer (IASLC). The Radiology Assistant: Mediastinum lymph node map 2009. Available online: http://www.radiologyassistant.nl/en/p4646f1278c26f/mediastinum-lymph-node-map.html (accessed 29 September 2018).
- Vinod SK, Min M, Jameson M, et al. A review of interventions to reduce inter-observer variability in volume delineation in radiation oncology. J Med Imaging Radiat Oncol 2016;60:393-406. [Crossref] [PubMed]
- Mercieca S, Belderbos J, van Baardwijk A, et al. The impact of training and professional collaboration on the interobserver variation of lung cancer delineations: a multi-institutional study. Acta Oncol 2019;58:200-8. [Crossref] [PubMed]
- Hanna GG, McAleese J, Carson KJ, et al. 18F-FDG PET-CT Simulation for Non-Small-Cell Lung Cancer: Effect in Patients Already Staged by PET-CT. Int J Radiat Oncol Biol Phys 2010;77:24-30. [Crossref] [PubMed]
- Huo M, Gorayski P, Poulsen M, et al. Evidence-based Peer Review for Radiation Therapy - Updated Review of the Literature with a Focus on Tumour Subsite and Treatment Modality. Clin Oncol (R Coll Radiol) 2017;29:680-8. [Crossref] [PubMed]
- Chang ATY, Tan LT, Duke S, et al. Challenges for Quality Assurance of Target Volume Delineation in Clinical Trials. Front Oncol 2017;7:221. [Crossref] [PubMed]
- Marks LB, Adams RD, Pawlicki T, et al. Enhancing the role of case-oriented peer review to improve quality and safety in radiation oncology: Executive summary. Pract Radiat Oncol 2013;3:149-56. [Crossref] [PubMed]
- The Royal Australian and New Zealand College of Radiologists. Quality Guidelines for Volume Delineation in Radiation Oncology | RANZCR. Sydney; 2015.
- Mercieca S, Belderbos J, Gilson D, et al. Implementing the Royal College of Radiologists’ Radiotherapy Target Volume Definition and Peer Review Guidelines: More Still To Do? Clin Oncol (R Coll Radiol) 2019;31:706-10. [Crossref] [PubMed]
- Caissie A, Rouette J, Jugpal P, et al. A pan-Canadian survey of peer review practices in radiation oncology. Pract Radiat Oncol 2016;6:342-51. [Crossref] [PubMed]
- Hui CB, Nourzadeh H, Watkins WT, et al. Quality assurance tool for organ at risk delineation in radiation therapy using a parametric statistical approach. Med Phys 2018;45:2089-96. [Crossref] [PubMed]