Establishment and validation of a mathematical diagnosis model to distinguish benign pulmonary nodules from early non-small cell lung cancer in Chinese people
Introduction
Lung cancer has the highest death rate among all cancers and its incidence rate has increased worldwide. Lung cancer is mainly divided into small cell lung cancer (SCLC) and non-small cell lung cancer (NSCLC), of which NSCLC accounts for about 80% to 85% of all lung cancer cases (1). Solitary pulmonary nodules (SPNs) are a common incidental finding and are early manifestations of lung cancer. In addition, pulmonary nodules are increasingly detected, because of the increased implementation of screening lung cancer-screening programs using low-dose computed tomography (LDCT). Nevertheless, the sensitivity and specificity of LDCT for discriminating whether a nodule is a benign pulmonary nodule (BPN) or a malignant pulmonary nodule (MPN) are not optimal. The National Lung Screening Trial (NLST) reported that the rate of positive screening tests was 24.2% with LDCT with a false positive rate of 96.4% (2). Moreover, a retrospective study showed that only 5% of patients with pulmonary nodules identified with LDCT were diagnosed with lung cancer, indicating that the false positive rate was as high as 95% (3). The high prevalence of false positives using LDCT may lead to invasive and high-risk treatment, the induction of unwarranted anxiety and excessive cost. Therefore, it is clinically imperative to develop novel approaches with greater accuracy to aid in monitoring individuals with SPNs and allow for the safe and cost-effectively diagnosis of NSCLC while preventing benign growths from unnecessary procedures.
Serum tumour markers have been introduced into numerous clinical assessment tools for evaluating pulmonary nodules. In previous studies, it was shown that the combined detection of carcino-embryonic antigen (CEA), cytokeratin 19 fragment 21-1 (CYFRA21-1) and neuron-specific enolase (NSE) is an effective way to distinguish between benign and malignant SPNs. Furthermore, if the model added CYFRA 21-1, it could improve the prediction accuracy between the subgroups of benign and malignant SPNs (4,5).
In addition, inflammation has been confirmed to aid in the proliferation and survival of malignant cells, promotes angiogenesis and metastasis, subverts adaptive immune responses, and alters responses to hormones and chemotherapeutic agents (6). Several lung cancer patients exhibit elevated levels of distinct inflammatory markers in serum, including C-reactive protein (CRP) (7), interleukin (IL)-6, IL-8 (8), serum amyloid A (SAA) (9), and lactate dehydrogenase (LDH) (10) suggesting that the inflammatory markers could potentially be used as potential diagnostic markers for lung cancer. In recent studies, nodule radiographic and image characteristics coupled to clinical information such as age, smoking history, and hematological biomarkers have emerged as one of the main research directions to differentiate the BPNs from early-NSCLC (eNSCLC) (11,12).
Therefore, in our study, we aimed to establish a new tool for the differential diagnosis of eNSCLC based on candidate markers of clinical characteristics, radiographic image features, and hematological biomarkers, which may improve the accuracy of distinguishing benign from MPNs. In addition, we compared the diagnostic accuracy and the discriminative ability of our model with the Peking University People’s Hospital (PKUPH) model (13) and the Mayo Clinic model (14).
We present the following article in accordance with the TRIPOD reporting checklist (available at http://dx.doi.org/10.21037/tlcr-20-460).
Methods
Patients and methods
We reviewed the medical records from 94 eNSCLC and 48 BPNs patients who first visited the Sun Yat-sen University Cancer Center (Guangzhou, China) between January 2018 and December 2018. Patients were divided into a training cohort (Group A) and a validation cohort (Group B). A total of 81 patients (27 BPNs, 54 eNSCLC) were collected as Group A to create a mathematical model for eNSCLC. Another 61 patients (21 BPNs, 40 eNSCLC) served as Group B to validate this new model. All procedures performed in this study were in accordance with the Declaration of Helsinki (as revised in 2013) and approved by the Ethics Committee of the Sun Yat-sen University Cancer Center (registration ID: GZR2018-051). Because of the retrospective nature of the research, the requirement for informed consent was waived. Inclusion criteria were as follows: (I) patients with a definitive benign diagnosis and confirmed pathological diagnosis of NSCLC, and TNM (tumor-node-metastasis) stage were at stage I or stage II; (II) patients with a complete set of clinical data; and (III) patients without secondary carcinomas as assessed by computed tomography (CT) and ultrasonographic examination. Clinical data collected include the age of the patient, gender, body mass index (BMI), smoking history, family history of cancer, and TNM stage. CT imaging included nodule position, diameter, clear border, spiculation, and calcification. Biomarkers of blood analysis included inflammatory-related factors: albumin (ALB), CRP, LDH, SAA, ALB/CRP ratio (ACR), SAA/CRP ratio (SCR), white blood cells (WBC), neutrophils (N), lymphocytes (L), platelet (PLT), neutrophil-to-lymphocyte ratio (NLR), platelet-to-lymphocyte ratio (PLR) and serum tumour markers: CEA, CYFRA21-1 and NSE.
Statistical analysis
All statistical analyses were performed using SPSS software, version 19.0 (SPSS Inc., Chicago, IL, USA) and R (version 3.4.4) for Windows. For all clinical characteristics in Group A, a least absolute shrinkage and selection operator (LASSO) regression was performed to select for the probability of eNSCLC. LASSO shrinks all regression coefficients towards zero and sets the coefficients of many irrelevant features exactly to zero (15). Next, multivariate logistic regression analysis was used to identify independent predictors of the probability of eNSCLC. A mathematical diagnostic model for distinguishing between BPNs and eNSCLC was established based on the results of the multivariate logistic regression analysis. The model calibration was assessed with the Hosmer-Lemeshow goodness of fit test (P≥0.10) (16). Receiver-operating characteristic (ROC) curve, a graphical technique for describing and comparing the accuracy of diagnostic tests, was used to evaluate the sensitivity and specificity of the mathematical model and to choose its best diagnosis cut-off points. Adopting the area under the ROC curve (AUC), net reclassification improvement index (NRI) (17), integrated discrimination improvement index (IDI) (17) and decision curve analysis (DCA) (18) were used to compare the diagnostic accuracy and discriminative ability of our model to the PKUPH and Mayo models. A larger AUC, NRI and IDI indicated a more accurate classification. Subsequently, data of the patients in Group B were used to verify the accuracy of the model. The difference was considered statistically significant when P<0.05.
Results
Patient characteristics
In the training cohort (81 cases), there were 54 cases (66.7%) diagnosed as eNSCLC. The other 27 cases (33.3%) were diagnosed as BPNs. The average age of patients with BPNs and eNSCLC was 56.63±8.46 years and 60.2±10.04 years, respectively. In the validation cohort, there were 40 cases (65.6%) of eNSCLC and 21 cases (34.4%) of BPNs. The average age of patients with BPNs and eNSCLC was 57.29±10.51 years and 60.33±10.02 years, respectively. The demographic and clinical characteristics of the study subjects are presented in Table 1.
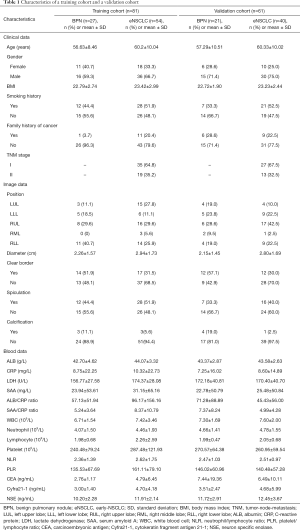
Full table
LASSO regression and multivariate logistic regression analysis
According to the LASSO regression, the potential predictors for predicting eNSCLC included age, BMI, family history of cancer, nodule diameter, nodule position, clear nodule border, calcification, ALB, LDH, ACR, SCR, PLR, CEA, CYFRA21-1, and NSE. Multivariate logistic regression analysis identified only age, clear nodule border, and ALB as independent diagnostic markers of eNSCLC (Table 2).
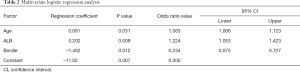
Full table
Model construction
The mathematical model established by logistic regression was as follows:
P=ex/(1+ ex), x=−11.02+(0.061× age) +(0.202× ALB) −(1.452× border), where e is the natural logarithm, age is recorded by year; ALB represents the serum ALB level (ng/mL) and the border is derived from the imaging report (1: yes, 0: no). Calibration by the Hosmer-Lemeshow method showed χ2=7.042, P=0.532 (Figure 1). A P value of 0.7072 was ultimately selected as a cut-off point, P values >0.7072 should be considered a malignant disease, and P<0.7072 should be considered benign. The sensitivity of this model for the training cohort was 66.7%, the specificity was 77.8%, the positive predict value (PPV) was 85.7%, and the negative predict value (NPV) was 53.8%.
Model validation
Data of patients in Group B were used to validate the accuracy of the model. The AUC of our model was 0.719 [95% confidence interval (95% CI): 0.582–0.857]. A P value of 0.7072 was used as a cut-off point as determined from the model construction from Group A. The sensitivity of this model for Group B was 63.4%, and the specificity was 70.0%, the PPV was 81.3%, and the NPV was 48.3%.
Comparison of the diagnostic performance of the prediction model with the PKUPH model and the Mayo model
Data for the training cohort and validation cohort were substituted into the proposed model, PKUPH model, and the Mayo model to compare the diagnostic accuracy and discriminative ability of determining whether pulmonary nodules were benign or malignant using AUC, NRI, IDI, and DCA.
In the PKUPH model, the independent factors were age, family history of cancer, spiculation, calcification, clear nodule border, and tumor diameter. The calculation was based on the formula: P=ex/(1+ ex), where x=−4.496+(0.007× age) +(0.676× diameter) +(0.736× spiculation) +(1.1267× family history of cancer) −(1.615× calcification) −(1.408× border). In the Mayo model, the independent factors were age, smoking history, cancer history, nodule diameter, spiculation, and site in left side. The calculation was based on the formula: P=ex/(1+ ex), where x=−6.8272+(0.0391× age) +(0.7917× smoking history) +(1.3388× cancer history) +(0.1274× diameter) +(1.0407× spiculation) +(0.7838× the upper lobe).
The results of our comparisons between the three models are presented in Table 3 and the respective ROC curves are shown in Figure 2. In the training cohort (Figure 2A), the AUC of our model was 0.740, which was larger than the AUCs for the PKUPH model (0.717, P=0.755) and the Mayo model (0.652, P=0.275). In the validation cohort (Figure 2B), the AUC of the three models was 0.719, 0.696, and 0.614, respectively. The AUC of our model was higher than the other two forecasting models.
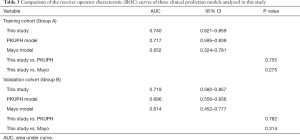
Full table
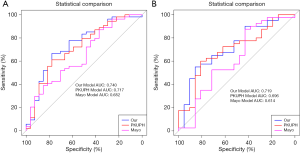
Table 4 shows that based on the NRI analysis, our model had improved the accuracy of the identification of benign and malignant nodules both in the training set (for the PKUPH model: NRI 3.7%; 95% CI: −17.44% to 24.84%; P=0.731; for the Mayo model: NRI 27.78%; 95% CI: 7.19% to 48.36%; P=0.008) and in the validation cohort (for the PKUPH model: NRI 12.26%; 95% CI: −12.11% to 36.64%; P=0.324; for the Mayo model: NRI 20.83%; 95% CI: −1.78% to 43.45%; P=0.071). Moreover, IDI analysis showed that the discrimination of our model was lower than that of the PKUPH model (for the training cohort: IDI −4.77%; 95% CI: −16.79% to 7.26%; P=0.437; for the validation cohort: IDI −4.55%; 95% CI: −16.76% to 7.67%; P=0.466), however, the difference was not significant. The discrimination of our model was higher than that of the Mayo model (for the training cohort: IDI 11.67%; 95% CI: 2.31% to 21.03%; P=0.015; for the validation cohort: IDI 11.13%; 95% CI: 0.87% to 21.39%; P=0.034). By DCA, our model had a higher overall net benefit compared to the previously published models both in the training cohort and validation cohort (Figure 3).
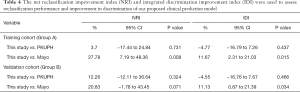
Full table
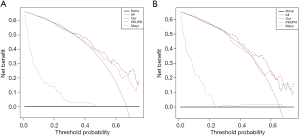
Discussion
In this study, multivariate logistic regression analysis identified only age, ALB levels, and nodule border as identified as independent predictors for estimating eNSCLC in SPNs. Based on these results, a clinical prediction model for SPNs was established, which showed our clinical prediction model had better diagnostic accuracy and discriminatory ability compared to the older models in discriminating SPNs.
With increasing patient age, the carcinogenic factors give more stimulation to the body and the capability of self-renewal, and repairing somatic cells was gradually decreased in humans. Swensen et al. reported that age, cigarette-smoking status, history of cancer, nodule diameter, spiculation, and upper lobe location of the SPNs were independent factors of malignancy and with advanced age, the possibility for malignancy of SPNs increased significantly (14,19). MPNs tend to form unclear, matte border features. An intensely clear border intensely predicted the presence of a benign nodules (20). In previous studies, it was shown that in 20–30% of malignant tumors, the borders were smooth, especially in metastatic tumors. Zheng et al. recently reported that ALB levels were new independent predictors of malignancy in SPNs (21). In the current study, multivariate logistic regression analysis of our research showed that age, border and ALB levels were independent factors for malignancy of SPNs.
The Mayo model was the most widely used to predict malignant SPNs, and the PKUPH model had been recently published with claims of superiority over traditional models (22,23). Compared to the previous studies, our model has the following advantages: first, in this study, data on 6 clinical features, 5 CT imaging indices, and 15 serum biomarkers were collected, which resulted in higher specificity and sensitivity compared to using current imaging models or biomarkers. Second, it is well known that it is a challenge to distinguish BPNs from NSCLC (especially early lung cancer). Therefore, we only chose to include eNSCLC (stage I or stage II) patients in our study, which may allow our results to improve the rate of early diagnosis of lung cancer. Third, predictor selection was one of the most important steps in downscaling procedures. We utilized a LASSO method to select the probability of eNSCLC for SPNs. The merits of LASSO include: a. a smaller mean squared error (MSE) than conventional methods; b. it handled the multi-collinearity problem; and c. overall variable selection (24-26). Finally, the diagnostic accuracy and discriminative ability of our model were compared with the PKUPH model and the Mayo model using multiple methods including, AUC, NRI, IDI, and DCA, making it the most comprehensive study reported to date. The multiple methods used to compare our model to the PKUPH model and the Mayo model demonstrated that our model outperformed competing models in distinguishing eNSCLC from SPNs.
Our study does have some limitations. First, we cannot avoid potential selection bias due to the retrospective nature of the study. Furthermore, our data were obtained from a single center and the sample size was relatively small. Therefore, this model needs further studies involving multiple center and ample samples to verify our results. In addition, circulating miRNAs (c-miRNAs) might be an ideal class of biomarkers for blood-based cancer detection. In a number of previous studies, it was reported that c-miRNAs were able to distinguish with remarkable accuracy lung cancer patients from non-cancer subjects with remarkable accuracy (27-29). However, the high cost of the detection of c-miRNAs prevents this method from being a common practice in our cancer center.
Conclusions
In conclusion, the newly proposed model only uses one general clinical index (age), one imaging index (border of nodule), and a serum marker (ALB level). To our knowledge, our model has better diagnostic accuracy and discriminatory ability than the older models in discriminating SPNs. Therefore, this model will help clinicians to accurately discriminate between benign and malignant nodules, and improve the rate of early diagnosis of lung cancer. Furthermore, a large-scale clinical study will be required to verify the importance and utility of our model.
Acknowledgments
Funding: This work was supported by the National Natural Science Foundation of China (Grant No. 81803110).
Footnote
Reporting Checklist: The authors have completed the TRIPOD reporting checklist. Available at http://dx.doi.org/10.21037/tlcr-20-460
Data Sharing Statement: Available at http://dx.doi.org/10.21037/tlcr-20-460
Conflicts of Interest: All authors have completed the ICMJE uniform disclosure form (available at http://dx.doi.org/10.21037/tlcr-20-460). The authors have no conflicts of interest to declare.
Ethical Statement: The authors are accountable for all aspects of the work in ensuring that questions related to the accuracy or integrity of any part of the work are appropriately investigated and resolved. All procedures performed in this study were in accordance with the Declaration of Helsinki (as revised in 2013) and approved by the Ethics Committee of the Sun Yat-sen University Cancer Center (registration ID: GZR2018-051). Because of the retrospective nature of the research, the requirement for informed consent was waived.
Open Access Statement: This is an Open Access article distributed in accordance with the Creative Commons Attribution-NonCommercial-NoDerivs 4.0 International License (CC BY-NC-ND 4.0), which permits the non-commercial replication and distribution of the article with the strict proviso that no changes or edits are made and the original work is properly cited (including links to both the formal publication through the relevant DOI and the license). See: https://creativecommons.org/licenses/by-nc-nd/4.0/.
References
- Chen W, Zheng R, Baade PD, et al. Cancer statistics in China, 2015. CA Cancer J Clin 2016;66:115-32. [Crossref] [PubMed]
- Aberle DR, Adams AM, Berg CD, et al. Reduced lung-cancer mortality with low-dose computed tomographic screening. N Engl J Med 2011;365:395-409. [Crossref] [PubMed]
- Gould MK, Tang T, Liu IL, et al. Recent Trends in the Identification of Incidental Pulmonary Nodules. Am J Respir Crit Care Med 2015;192:1208-14. [Crossref] [PubMed]
- Seemann MD, Beinert T, Furst H, et al. An evaluation of the tumour markers, carcinoembryonic antigen (CEA), cytokeratin marker (CYFRA 21-1) and neuron-specific enolase (NSE) in the differentiation of malignant from benign solitary pulmonary lesions. Lung Cancer 1999;26:149-55. [Crossref] [PubMed]
- Zhang M, Zhuo N, Guo Z, et al. Establishment of a mathematic model for predicting malignancy in solitary pulmonary nodules. J Thorac Dis 2015;7:1833-41. [PubMed]
- Mantovani A, Allavena P, Sica A, et al. Cancer-related inflammation. Nature 2008;454:436-44. [Crossref] [PubMed]
- McKeown DJ, Brown DJ, Kelly A, et al. The relationship between circulating concentrations of C-reactive protein, inflammatory cytokines and cytokine receptors in patients with non-small-cell lung cancer. Br J Cancer 2004;91:1993-5. [Crossref] [PubMed]
- Brenner DR, Fanidi A, Grankvist K, et al. Inflammatory Cytokines and Lung Cancer Risk in 3 Prospective Studies. Am J Epidemiol 2017;185:86-95. [Crossref] [PubMed]
- Biaoxue R, Hua L, Wenlong G, et al. Increased serum amyloid A as potential diagnostic marker for lung cancer: a meta-analysis based on nine studies. BMC Cancer 2016;16:836. [Crossref] [PubMed]
- Yuh YJ, Kim SR. actate Dehydrogenase (LDH) as a Tumor Marker for Non-small Cell Lung Cancer. Cancer Res Treat 2002;34:339-44. [Crossref] [PubMed]
- Li X, Zhang Q, Jin X, et al. Combining serum miRNAs, CEA, and CYFRA21-1 with imaging and clinical features to distinguish benign and malignant pulmonary nodules: a pilot study: Xianfeng Li et al.: Combining biomarker, imaging, and clinical features to distinguish pulmonary nodules. World J Surg Oncol 2017;15:107. [Crossref] [PubMed]
- Peng XX, Yan LX, Liu C, et al. Benign disease prone to be misdiagnosed as malignant pulmonary nodules: Minute meningothelioid nodules. Thorac Cancer 2019;10:1182-7. [Crossref] [PubMed]
- Li Y, Chen KZ, Wang J. Development and validation of a clinical prediction model to estimate the probability of malignancy in solitary pulmonary nodules in Chinese people. Clin Lung Cancer 2011;12:313-9. [Crossref] [PubMed]
- Swensen SJ, Silverstein MD, Ilstrup DM, et al. The probability of malignancy in solitary pulmonary nodules. Application to small radiologically indeterminate nodules. Arch Intern Med 1997;157:849-55. [Crossref] [PubMed]
- Usai MG, Goddard ME, Hayes BJ. LASSO with cross-validation for genomic selection. Genet Res (Camb) 2009;91:427-36. [Crossref] [PubMed]
- Lemeshow S, Hosmer DW Jr. A review of goodness of fit statistics for use in the development of logistic regression models. Am J Epidemiol 1982;115:92-106. [Crossref] [PubMed]
- Burch PM, Glaab WE, Holder DJ, et al. Net Reclassification Index and Integrated Discrimination Index Are Not Appropriate for Testing Whether a Biomarker Improves Predictive Performance. Toxicol Sci 2017;156:11-3. [PubMed]
- Zhang Z, Rousson V, Lee WC, et al. Decision curve analysis: a technical note. Ann Transl Med 2018;6:308. [Crossref] [PubMed]
- Swensen SJ, Silverstein MD, Edell ES, et al. Solitary pulmonary nodules: clinical prediction model versus physicians. Mayo Clin Proc 1999;74:319-29. [Crossref] [PubMed]
- Khan A, Herman PG, Vorwerk P, et al. Solitary pulmonary nodules: comparison of classification with standard, thin-section, and reference phantom CT. Radiology 1991;179:477-81. [Crossref] [PubMed]
- Zheng B, Zhou X, Chen J, et al. A Modified Model for Preoperatively Predicting Malignancy of Solitary Pulmonary Nodules: An Asia Cohort Study. Ann Thorac Surg 2015;100:288-94. [Crossref] [PubMed]
- Xiao F, Liu D, Guo Y, et al. Novel and convenient method to evaluate the character of solitary pulmonary nodule-comparison of three mathematical prediction models and further stratification of risk factors. PLoS One 2013;8:e78271. [Crossref] [PubMed]
- Li Y, Wang J. A mathematical model for predicting malignancy of solitary pulmonary nodules. World J Surg 2012;36:830-5. [Crossref] [PubMed]
- Xu CJ, van der Schaaf A, Schilstra C, et al. Impact of statistical learning methods on the predictive power of multivariate normal tissue complication probability models. Int J Radiat Oncol Biol Phys 2012;82:e677-84. [Crossref] [PubMed]
- Xu CJ, van der Schaaf A, Van't Veld AA, et al. Statistical validation of normal tissue complication probability models. Int J Radiat Oncol Biol Phys 2012;84:e123-9. [Crossref] [PubMed]
- Xu J, Yin J. Kernel least absolute shrinkage and selection operator regression classifier for pattern classification. Iet Computer Vision 2013;7:48-55. [Crossref]
- Boeri M, Verri C, Conte D, et al. MicroRNA signatures in tissues and plasma predict development and prognosis of computed tomography detected lung cancer. Proc Natl Acad Sci U S A 2011;108:3713-8. [Crossref] [PubMed]
- Sozzi G, Boeri M, Rossi M, et al. Clinical utility of a plasma-based miRNA signature classifier within computed tomography lung cancer screening: a correlative MILD trial study. J Clin Oncol 2014;32:768-73. [Crossref] [PubMed]
- Hennessey PT, Sanford T, Choudhary A, et al. Serum microRNA biomarkers for detection of non-small cell lung cancer. PLoS One 2012;7:e32307. [Crossref] [PubMed]