Age at first birth and lung cancer: a two-sample Mendelian randomization study
Introduction
Lung cancer (LC) is recognized as the major cause of cancer deaths worldwide, and the second-most commonly diagnosed cancer in women, with around 228,820 new cancer cases and 116,300 estimated deaths in the USA in 2020 (1). The 5-year relative survival rate for non-small-cell lung cancer (NSCLC) is 23% and for small-cell lung cancer (SCLC) is 6% (2). In view of the growing burden of LC, making progress in early detection of LC and better management of patients with LC contribute to lowering the morbidity and mortality. Consequently, it is crucial for investigators to identify potentially modifiable risk factors for better prevention. Taking cigarette smoking as an example, it is considered to be a leading high-risk factor for LC (3). Effective tobacco control policies carried out by governments greatly contributed to decreasing the morbidity of LC and further improving the long-term prognosis of the patients (4,5).
Notably, recent studies have revealed that a great amount of female cases of LC occur in non-smokers. Furthermore, adenocarcinoma makes up for around three quarters of all LC subtypes among non-smoking women and a higher frequency of mutations in the epidermal growth factor receptor (EGFR) was observed (6). Difference between men and women in the morbidity of LC suggests that the physiological characteristics, like hormonal factors, might potentially have an impact on the progression of LC (7). The effects of hormones contributing to the pathogenesis of LC was first described by laboratory evidence which identified progesterone receptors (PR) and estrogen receptors (ER) in human NSCLC tissue (8). Recent studies also revealed that steroid hormone-related receptors, including ER, PR and human EGFR-2, frequently express in tumour issue of LC (9). In addition, ER α nuclear expression associates significantly with lung adenocarcinoma (LUAD), non-smokers and female (10,11). Consequently, it has been hypothesized that factors of reproductive health, including age at first birth (AFB), age at menarche and number of pregnancies, could have an impact on women’s risk of developing LC through their effects on steroid hormones (12,13). However, findings pertaining to the contribution of AFB towards LC risk are rather controversial. Moreover, the causal effect between AFB and LC risk remains unclear.
In 2003, Kreuzer et al. reported a significantly decreased risk of LC with increased AFB through a case-control study including 811 female LC cases and 912 controls (14). Likewise, Kabat et al. published a prospective cohort study (89,835 women and 750 incident LC cases) which found that women’s AFB older than age 30 were at a 38% lower risk of LC compared with women’s AFB below age 23 (15). Nevertheless, subsequent studies concerning the relationship of AFB and LC risk have primarily been negative (16-19). In general, previous conventional observational studies provided evidence that late AFB can lower or have no significant effect on LC risk. However, in view of inherent shortcomings of previous observational studies, including confounders, inadequate attention to variation by histology or reverse causality, this correlation could be biased (20). Simultaneously, we cannot determine whether there is a cause-and-effect relationship between AFB and LC.
Considering the relative long incubation period between AFB and LC, the randomized controlled trials (RCTs), which recognized as the gold standard in investigating causality, may not be workable for this event. Consequently, we utilized a novel genetic epidemiological tool, the Mendelian randomization (MR) analysis. MR design is based on Mendel’s second law that genetic variants are randomly allocated before birth and fixed at conception, which are generally not affected by environmental risk factors and precede risk factors and the progression of diseases (21). Utilizing genetic variants as instrumental variables (IVs) for risk factors, mainly single-nucleotide polymorphisms (SNPs), MR can provide an analogy to RCTs in an observational setting (22). Besides, MR analysis can surmount the limitations of traditional approaches, such as reverse causality, confounding and measurement error, for the sake that the process between gene and disease is usually a one-way flow (23). In addition, we can employ a two-sample MR analysis which obtains IVs-exposure and IVs-outcome association from large-scale genome-wide association studies (GWASs), greatly improving the statistical power of MR (24,25).
Our study investigated the potential causality between AFB and LC risk by means of a two-sample MR approach for the first time.
Methods
MR procedures
Statistical analysis was applied using summary-level genetic data from the International Lung Cancer Consortium (ILCCO) and latest published GWAS meta-analysis studies, in a two-sample MR framework. MR is an approach utilizing genetic variants as instruments to obtain estimates for the causal effect of risk factors on disease outcomes. Our MR approach based on three basic assumptions as follows: (I) the genetic markers are associated with AFB robustly; (II) the IVs affect LC merely through their effect on AFB without any alternative causal pathways, that is, no pleiotropic effects do genetic markers have through pathways different from the exposure; and (III) the IVs are independent of confounders existing in the relation between AFB and LC (26) (Figure 1). All assumptions could not be violated, otherwise the causal link obtained by MR research would not have sufficient reliability.
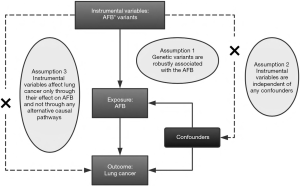
GWAS summary data
We utilized genetic summary data from large consortia for human reproductive behavior as well as ILCCO developed by the MRC Integrative Epidemiology Unit. Summary data of these two consortia were publicly available on the MR-Base platform (http://www.mrbase.org/), an analytical platform and database for MR (27).
Genetic variants associated with AFB
From 62 cohorts of European descent in total, Barban et al. reported the largest meta-analysis of GWASs, including 343,072 for number of children ever born (NEB) and 251,151 individuals for AFB. 12 independent loci were identified by the study that were significantly correlated with NEB and/or AFB (28). First, we identified 10 SNPs which robust association with AFB was confirmed in a threshold of statistical significance of P<5×10−8, including rs10056247, rs10908557, rs10953766, rs1160544, rs2347867, rs242997, rs2721195, rs2777888, rs293566 and rs6885307 (details of each of SNPs, including standard errors and effect sizes are available at Table S1). Across individuals, these 10 SNPs explained approximately 15% of the variation in AFB. The F-statistic of our study was 4,802.59 (>10) (Table 1), indicating the strong prediction of the AFB instruments we used. In addition, the number of LC cases demanded for 80% power with an odds ratio (OR) from previously estimated causal effect size of AFB of 0.68 (15) was at least 1530 subjects (Table 1). Consequently, based on these 10 SNPs, it was sufficient for the generation of a powerful genetic instrument. Second, using linkage disequilibrium (LD) analysis, all of the SNPs were not excluded (R2<0.001). Eventually,10 SNPs were applicable for the final IV set (Table S1). It is worth noting that none of the 10 SNPs robustly associated with AFB is correlated with men only while rs1160544, rs2777888, rs6885307, rs10953766, rs2347867 and rs2721195 are significantly associated with women. Consequently, mechanisms underlying AFB to LC are mainly decided by female reproductive factors (29). The study was conducted in accordance with the Declaration of Helsinki (as revised in 2013) (30).
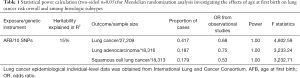
Full table
GWAS summary data on LC
Derived from the European ancestry, genetic data of 11,348 LC cases and 15,861 controls were used as epidemiological individual-level data from ILCCO (Table 2), a global group of LC researchers established in 2004 (31,32). For each of the 10 SNPs associated with AFB (rs10056247, rs10908557, rs10056247, rs10953766, rs2347867, rs293566, rs1160544, rs242997, rs2721195 and rs6885307), summary data for the same SNPs were retrieved through MR-Base platform.
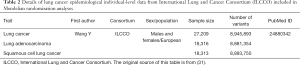
Full table
Statistical analysis
Several MR approaches were used to investigate MR estimates of AFB for LC. First, a random effects Wald-type estimator of inverse-variance weighted (IVW) was conducted to generate a MR estimate of multiple IVs. Given that the SNP has a cumulative effect on AFB, the IVW causal effect estimation can be combined with the ratio estimate and standard error of a single SNP using the method of Burgess et al. (33). All previous hypotheses are assumed to be consistent with the previously described genetic variant p (p = 1 ... P); which is link to the mean change in AFB (Xp) of the risk factor observed with each other variant allele with standard error (σXp) and observed logarithmic change (Yp) in the results of each allele with standard error (σYp). The calculation is as follows:
Using and , we presented the results as OR and 95% confidence intervals (CI). Second, the weighted median estimator was conducted which enabled us to find the weighted empirical distribution function out for all the selected SNPs ration estimates. Weighted median is able to provide a consistent estimate of causality, even if up to 50% of the information useful for the analysis comes from genetic variants that are invalid IVs, it can also ensure that the causal effect estimate is not biased (34). Third, a MR-Egger estimator was employed which assumes that the SNP-exposure effects are not involved with directional pleiotropy (35). Same analyses were additionally performed for two different histological subtypes of NSCLC, including the squamous cell lung cancer (LUSC) and LUAD. Presented as OR and 95% CI, the results provided an estimate of relative risk resulted from number unit (1 year) increase/decrease on AFB.
Since our chosen SNPs were selected at the genome-wide significance threshold of P<5×10–8 and the F-statistics was 4,802.59 (>10), the first assumption was met. We conducted MR-Egger and weighted median methods to test for the second assumption indirectly. For sensitivity analysis, we obtained potential pleiotropic effects from the MR-Egger analysis based on the intercept. The MR Pleiotropy Residual Sum and Outlier (MR-PRESSO) test was applied to identify the potential horizontal pleiotropic effects of the SNPs to detect and correct for possible outliers. Leave-one-out analysis was conducted to evaluate whether the estimation of MR was determined or biased by a SNP separately by successively omitting a single SNP. Aiming at verifying the third assumption, we employed additional MR analyses to investigate whether genetic susceptibility towards AFB could be related to the common risk factors of LC. For example, smoking is a leading LC risk factor, which is associated with about 80%-90% of LC around the world (36). We obtained genetic effects on cigarette smoking status (former vs. current smoker; ever vs. never smoked; age of smoking initiation; cigarettes smoked per day) from the Tobacco and Genetics consortium (TAG) (37). Sundermann et al. reported that during pregnancy, alcohol consumption is associated with an increased risk of dose-mediated miscarriage and might further lead to older age of pregnancy (38). Simultaneously, epidemiological evidence suggested that alcohol consumption is correlated with an increased LC risk (39). Therefore, alcohol consumption is a potential confounder of the AFB-LC relationship. Genetic summary data on alcohol consumption status (previous vs. never) were extracted from the Neale Lab. In addition, based on existing literature, we selected the potential mediators (apart from smoking and alcohol consumption), such as lipids and body mass index (BMI), the genetic instruments of which were obtained from the Global Lipids Genetics Consortium (GLGC) and the Genetic Investigation of ANthropometric Traits consortium (GIANT) (40-43) (Table 3). We also chose other traits that genetically overlap with AFB (years of schooling, type 2 diabetes and waist-hip ratio), which were also correlated with risk of LC, to perform additional MR analyses (28,44,45). The genetic data were obtained from Social Science Genetic Association Consortium (SSGAC) and GIANT, respectively. All of the MR analyses were performed in R (version 4.0.2) using the package TwoSampleMR (version 0.5.4) (27).
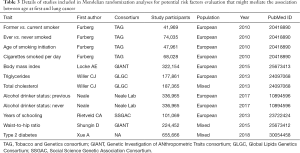
Full table
Power calculation
Assuming that the SNPs explain 15% of the total variation of AFB according to previous reports, our sample size of 113,48 LC cases and 15,861 controls had an estimated 100.0% power for detecting the causal effect size of AFB (OR =0.68) (15) at a level of significance (P=0.05) (46) (Table 1). Alternatively, given our sample size, we have 99.0% power for detecting a minimal odds ratio of 1.15 at a level of significance (P=0.05) (17).
Results
Causal effect from AFB to LC
Genetically predisposed older AFB was correlated with significantly lower LC risk. Conventional IVW method demonstrated that number unit (1 year) increase of AFB was correlated with a 18% lower LC risk (OR =0.82, 95% CI: 0.69–0.97, P=0.029) (Figure 2). Using MR-Egger (OR =0.57, 95% CI: 0.04–8.00, P=0.700) and weighted median method (OR =0.84, 95% CI: 0.68–1.03, P=0.088), the causality estimation was similar in accordance with direction and magnitude. Similar causal trends were observed in LUAD subgroup (OR =0.75, 95% CI: 0.59–0.97, P=0.017) but not in LUSC subgroup (OR =0.77, 95% CI: 0.57–1.05, P=0.103) (Table 4). In regard to single SNP analysis, rs10056247 was observed to associate with a lower LUSC risk (Table S2). No association was observed between number unit (1 year) decrease of AFB and LC (OR 1.19, 95% CI: 0.96–1.48, P=0.113), neither in LUAD (OR =1.10, 95% CI: 0.78–1.55, P=0.575) nor LUSC subgroup (OR 1.32, 95% CI: 0.94–1.84, P=0.104) (Table 4). Through MR-Egger regression analysis, we found that there was no evidence for the presence of directional pleiotropy since the p-values for the intercept were large and the adjusted estimates of pleiotropy were invalid (Table 5, Table S3). In addition, using the MR-PRESSO global test, we did not detect any outlier SNPs or the horizontal pleiotropic effect of AFB on risk of any outcomes (P=0.412). Heterogeneity was not observed (Table 5, Table S4). No single SNP was found to strongly drive the overall effect of AFB on LC through leave-one-out sensitivity analysis (Figures S1-S6).
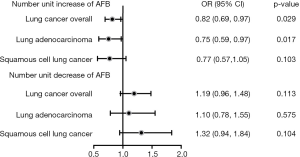
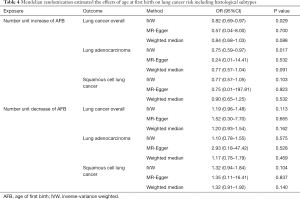
Full table
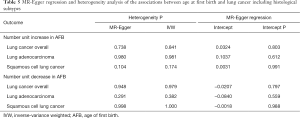
Full table
Causal effect from AFB on potential LC risk factors
Additional MR analyses in conventional IVW method were applied to identify whether the association between genetically predisposed AFB and LC was influenced by potential confounders and mediators. IVW method provided evidence that genetically predisposed number unit (1 year) increase in AFB was causally associated with longer years of schooling (OR =1.12, 95% CI: 1.08–1.16, P<0.001), lower BMI (OR =0.93, 95% CI: 0.88–0.98, P=0.004) and less alcohol consumption (OR =0.99, 95% CI: 0.99–1.00, P=0.004) while it provided no evidence for the relationship between AFB and smoking status, triglycerides, type 2 diabetes and total cholesterol (Table 6, Table S5).
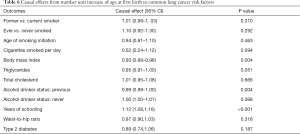
Full table
Discussion
This two-sample MR analysis gave evidence of causality between genetically predisposed number unit (1 year) increase of AFB and a reduced risk of LC. More specifically, 1 year older of AFB predicted a lower risk of LUAD by almost a quarter. In addition, to investigate the potential mechanisms mediating AFB to LC risk, we found that genetic inclination towards older AFB was correlated with long years of education, lower BMI and less alcohol consumption.
Actually, the genetic architecture of AFB is closely relevant to health, human development, psychiatric disorders and so on (47-49). Considered as a relatively precise means for measuring complex reproductive outcomes, AFB is frequently recorded as key parameter for forecasting population. Evidence suggests that heritability accounts for up to 50% for reproduction behaviors like AFB and NEB, implying that the genetic component plays an important role (50). Tropf FC’s findings put down 15% of the variance in AFB to common genetic variants (46). Moreover, they also found that AFB is positively correlated with age at menarche, voice breaking, education attainment and so on genetically. In contrast, more alleles correlated with increased AFB is relevant to a lower genetic risk of smoking, obesity and diabetes. Consequently, genetic component consists a relatively large part of AFB and the genetic effects are important in many aspects. Nevertheless, we should also notice that AFB is mainly determined by social factors and the genetic effects are unlikely to be independent of them.
In fact, reproductive factors involvement in LC incidence has long been a concern. Nevertheless, findings pertaining to the contribution of AFB towards LC risk have been rather controversial. In 2003, Kreuzer and her colleagues identified a significantly decreased risk of LC with increasing AFB from a case-control study including 811 female LC cases and 912 controls (14). Moreover, the results were presented for SCLC, LUAD and LUSC for smokers, but not for non-smokers. This inverse association was later supported by a prospective cohort study (89,835 Canadian women and 750 LC incident cases) which indicated that women’s AFB older than age 30 were at a 32% lower risk compared with women’s AFB below age 23 (15). In contrast, a prospective NIH-AARP cohort (American Association of Retired Persons) study involving 185,017 women and 3,512 LC cases by Brinton et al. did not report convincing evidence between late AFB and a decreased LC risk with adjustments for smoking status, education, BMI and so on (51). In 2015, with the Women’s Health Initiative clinical trials including 161,808 postmenopausal women, Schwartz’s findings supported a statistically significant relation between older AFB and a lower risk of LC overall, and NSCLC specifically. Nonetheless, the latest epidemiologic evidence from a pooled analysis comprised of eight studies using data from ILCCO (4,386 cases and 4,177 controls) demonstrated a lack of association between AFB and LC (52).
However, considering the characteristics of their observational design, several limitations existed. First, given that BMI is considered to be a potential risk factor of LC (45), none of studies have managed to control BMI. Consequently, a BMI-independent AFB-LC relation could not be assessed effectively. Likewise, the incomplete control for other confounding factors, including occupational exposures, alcohol consumption and smoking status, might contribute to the biased results. Second, most of the studies failed to evaluate variation in effects according to histologic subtypes and hence, it remains unclear which subtype of LC is indeed correlated with AFB. Third, previous studies mainly obtained information about reproductive history based on questionnaires. Therefore, they could not rule out the possibility of bias caused by inaccurate recall. More importantly, to date, no prospective large-scale longitudinal cohort studies have been conducted and thus, present studies could not provide adequate evidence on the causal relationship from AFB to LC.
As far as we know, our study described the association between AFB and risk of LC by means of MR for the first time. Interestingly, the correlation between AFB and LC risk might be mediated by many intermediate phenotypes. Previous researches suggested that early AFB is related to lower social class and less education (53). People with older AFB tend to complete longer years of education and usually lead a healthier lifestyle than those with lower social class and less education, i.e., less smoking, less alcohol consumption and a healthy BMI (18.5–24.9 kg/m2) (54-56). Our further detailed analysis provided evidence that older age of giving first birth led to a reduced risk of alcohol consumption. Given that alcohol consumption is identified as a high-risk factor of LC and is correlated with AFB, it might be a potential mediator on the AFB-LC pathway (38,39). However, based on the characteristics of the data we utilized, stratified analysis concerning alcohol consumption was infeasible. Moreover, our study confirmed that older age of giving first birth was associated with a lower BMI. Further studies elucidating the exact degree of the mediating effect are warranted. Late AFB was also causally associated with longer years of education. Given Zhou et al. reported high education attainment, mainly considered as a social factor, is a causal protective factor in the development of LC, it might be a key mediator on the AFB-LC pathway (57).
According to the data established by International Agency for Research on Cancer (WHO) in 2018, the global averaged incidence age-standardized incidence rate (ASIR) was 14.6 (per 100,000) for LC in females and the estimated deaths were 600 thousand (58). During the past few decades, the incidence rates for new LC diagnoses in males declined gradually whereas a tendency to increase in females was observed globally (59,60). Female LC is more prevalent in the developed regions like in Western and Northern Europe, followed by Northern America and Australia. For instance, in Europe, the female LC incidence has been climbing since 21st century and now is the second most common cancer after breast cancer (61). Developing countries like China also faces heavy disease burden of LC since the ASIR was 23.5 (per 100,000) and age-standardized mortality rate (ASMR) was 16.5 (per 100,000) in female population (62).
The etiology of female LC has been the research focus in recent years. Specifically, evidence concerning the susceptibility to developing LC in women was incompletely defined. Studies from Europe showed that only 47% of female LC cases were a consequence of cigarette smoking and the proportion in male were 85% (63). Subsequent studies also demonstrated a higher incidence rate of LC in non-smoking women compared with non-smoking men (64). Among never-smoking women, exposure to biomass fuel and cooking were common and possibly responsible for an increased risk of LC (65). Other air pollutants like particulate matter 2.5 (PM2.5) were associated with approximately 18% of LC deaths in women in China (66). The role of second-hand smoke (SHS) exposure is also being highlighted with the emerging evidence (67,68). Nevertheless, since counting the intensity of SHS is challenging, more studies still need to be done to confirm the positive association.
Possible mechanisms for intrinsic predisposition of LC in women are as follow. A higher frequency of P53 gene mutation, considered as a crucial factor in the incubation of LC, was examined in female LC patients than male (69). Later studies further confirm that the frequency of P53 gene mutation was higher in smokers than non-smokers among women (70). In addition, a large-scale and prospective study found that LUAD in women were more likely to harbor K-ras mutation after controlling for asbestos exposure and smoking. The results also implied a potential role of estrogen exposure in the initiation of K-ras mutant clones (71). In 2012, a large-scale GWAS containing 5,510 never-smoking female LC cases and 4,544 controls identified three new susceptibility loci for LC at 6q22.2, 6p21.32 and 10q25.2 (72). Moreover, significantly reduced DNA repair capacity in women compared with men was reported, possibly increasing the risk of LC (73,74). Gastrin-releasing peptide (GRP) has been shown to play a crucial role in carcinogenesis by promoting cell proliferation and epithelial differentiation. Meanwhile, GRP receptor gene is observed to be activated earlier and express more frequently in women than men in response to tobacco exposure, suggesting it may be an agent in increasing the susceptibility in women of LC (75).
With respect to estrogen, it is postulated as a vital factor in the formation of female LC. 17-β-estradiol, the most potent form of estrogen, can promote adenocarcinoma cells in vitro (76). Scientists found that elevated expression of ERβ was associated with a higher frequency of EGFR mutations (77). Higher levels of estrogen were also found to be connected with worse survival in premenopausal women (78). Studies showed that aromatase can also have an influence in carcinogenesis of LC by means of the conversion of androgen to estrogen locally (79). Lim et al. reported the COMT rs4680 A allele, considered as the estrogen pathway gene polymorphism, was positively correlated with LC in non-smokers (80). The above-mentioned findings regarding the role of estrogen may raise the question that whether hormone replacement therapy can contribute to LC development but the answers are still conflicting (81-83).
Notably, our MR study showed that the causal effect in the LUAD group were more significant than the effect in LUSC group. This phenomenon might be associated with the involvement of gender-dependent factors, such as hormonal factors and reproductive factors (84). Steroid hormone-related receptors, including ER, PR and human EGFR-2, have been shown to frequently express in tumour tissue of lung (9). Among sex steroids, progesterone is the second-most common female steroid hormone, which is involved in various of metabolic and physiological changes throughout life, such as pregnancy, puberty and menstrual cycle (85). It is well known that progesterone is capable to facilitate differentiation and inhibit cellular proliferation through the PR (86). Recent advances in progesterone biology further demonstrated that the growth inhibition mediated by progesterone was mainly preceded by downregulating the expression of cyclins A, and E and/or upregulating the expression of cyclin-dependent kinase inhibitors, like p21 and p27 (87). Additionally, several investigators revealed that PR was likely to be an effective prognostic factor in NSCLC and a possible target for progesterone therapy among NSCLC patients (88). Consequently, the progesterone might inhibit the cellular proliferation of tumor cells through combination with the PR in lung tissue and contribute to suppressing the progression of LC (89). However, in vitro, in vivo and clinical data concerning the role of progesterone on the progression of LC are relatively rare and further studies are warranted.
Given the relatively long latency between AFB and LC, it might be infeasible to investigate the causality by means of RCTs. In this regard, our study is capable to give evidence from a novel type of study design, the MR approach, which also supports the causality between AFB and LC. Our analysis presents several important strengths. First, as far as we know, it is the largest study to investigate the causal relationship between AFB and risk of LC using genetic variants. With large sample sizes (n=27,209) and robustly associated IVs (F statistics =4,802.59), our MR study with adequate statistical power (100%) could offer a relatively precise estimation of causal effect. It is also the first to elucidate whether effects differed between subgroups stratified by histology subtypes. Second, we performed additional MR analyses to identify potential risk factors that could mediate the correlation between AFB and LC. The results indicated that genetically predisposed number unit (1 year) increase in AFB was associated with longer years of schooling, less alcohol consumption and lower BMI, which deserved our further investigation.
Several limitations in our study could not be ignored. First, all the participants included in our study were of European origin. Consequently, whether our findings can extend to other regions and populations remains uncertain. Second, though we’ve used the most comprehensive set of genetic variants so far, it merely explained a part of variance of AFB across individuals. It is possible that some unknown AFB-related SNPs could also play an important role in the progression of LC. Second, all three MR assumptions could not be fully verified in our study and potential violations against the assumptions may occur. Due to the fact that the second assumption cannot be tested directly in our study, additional sensitivity analyses were carried out. No horizontal pleiotropic effects were presented in our study, suggesting the second MR assumption was not violated. Moreover, due to the characteristics of data from consortia, to fully assess the third assumption is impossible. Moreover, despite that we have made endeavor in eliminating the bias by adjustments for confounders, including LC risk factors and factors genetically overlap with AFB, these confounders cannot be directly compared and unobserved confounders may still have an effect on AFB-LC relationship given the differences in the methodological approaches and interpretation of estimates. Further work using individual-level data which have not yet been discovered may provide more robust evidence for understanding the mechanisms underlying AFB to LC. Notably, despite that we found late AFB is associated with a reduced LC risk, we could not conclude the optimum AFB since it is also concerned with various risk factors like cardiovascular disease, stillbirth and so on, which are important for women’s subsequent health and survival (90-92). Hence, researchers need to be cautious in interpreting our findings. Lastly, considering the summary data utilizing for our MR approach, stratified analyses by covariates of interest, including smoking, alcohol consumption, age and so on are infeasible.
In brief, our MR study provided relatively strong evidence to suggest that older AFB plays a causal role in decreasing the risk of LC, LUAD specifically. There is no doubt that cancer prevention is the key to reducing the morbidity and mortality of cancers. Consequently, we ought to attach great importance to identifying more modifiable risk factors correlated with cancers. Afterwards, we are able to conduct effective interventions to lower the disease burden worldwide, especially in developing countries. In present, both epidemiologic and basic studies concerning the effects of reproductive factors on LC are relatively insufficient. More studies investigating the potential mechanisms that mediate the association between AFB and LC are warranted.
Acknowledgments
The authors thank Ms. Lindsey Hamblin for helping to edit the manuscript. The authors acknowledge the efforts of the International Lung Cancer Consortium (ILCCO) in providing high quality GWAS data for researchers. The authors acknowledge the efforts of the genome-wide association study consortia in providing high-quality resources in the MR-Base platform (
Funding: This work was supported by China National Science Foundation (Grant number 81871893); Key Project of Guangzhou Scientific Research Project (Grant number 201804020030); Cultivation of Guangdong College Students’ Scientific and Technological Innovation (“Climbing Program” Special Funds) (Grant number pdjh2020a0480).
Footnote
Data Sharing Statement: Available at http://dx.doi.org/10.21037/tlcr-20-1216
Conflicts of Interest: All authors have completed the ICMJE uniform disclosure form (available at http://dx.doi.org/10.21037/tlcr-20-1216). The authors have no conflicts of interest to declare.
Ethical Statement: The authors are accountable for all aspects of the work in ensuring that questions related to the accuracy or integrity of any part of the work are appropriately investigated and resolved. The study was conducted in accordance with the Declaration of Helsinki (as revised in 2013).
Open Access Statement: This is an Open Access article distributed in accordance with the Creative Commons Attribution-NonCommercial-NoDerivs 4.0 International License (CC BY-NC-ND 4.0), which permits the non-commercial replication and distribution of the article with the strict proviso that no changes or edits are made and the original work is properly cited (including links to both the formal publication through the relevant DOI and the license). See: https://creativecommons.org/licenses/by-nc-nd/4.0/.
References
- Siegel RL, Miller KD, Jemal A. Cancer statistics, 2020. CA Cancer J Clin 2020;70:7-30. [Crossref] [PubMed]
- Miller KD, Nogueira L, Mariotto AB, et al. Cancer treatment and survivorship statistics, 2019. CA Cancer J Clin 2019;69:363-85. [Crossref] [PubMed]
- Nabi H, Estaquio C, Auleley GR. Smoking and mortality--beyond established causes. N Engl J Med 2015;372:2169. Erratum in: N Engl J Med 2016;375:2410. [PubMed]
- Dobson Amato KA, Hyland A, Reed R, et al. Tobacco Cessation May Improve Lung Cancer Patient Survival. J Thorac Oncol 2015;10:1014-9. [Crossref] [PubMed]
- Wong KY, Seow A, Koh WP, et al. Smoking cessation and lung cancer risk in an Asian population: findings from the Singapore Chinese Health Study. Br J Cancer 2010;103:1093-6. [Crossref] [PubMed]
- Cheng TD, Cramb SM, Baade PD, et al. The International Epidemiology of Lung Cancer: Latest Trends, Disparities, and Tumor Characteristics. J Thorac Oncol 2016;11:1653-71. [Crossref] [PubMed]
- Sathish V, Martin YN, Prakash YS. Sex steroid signaling: implications for lung diseases. Pharmacol Ther 2015;150:94. [Crossref] [PubMed]
- Siegfried JM, Hershberger PA, Stabile LP. Estrogen receptor signaling in lung cancer. Semin Oncol 2009;36:524-31. [Crossref] [PubMed]
- Cheng TD, Darke AK, Redman MW, et al. Smoking, Sex, and Non-Small Cell Lung Cancer: Steroid Hormone Receptors in Tumor Tissue (S0424). J Natl Cancer Inst 2018;110:734-42. [Crossref] [PubMed]
- Raso MG, Behrens C, Herynk MH, et al. Immunohistochemical expression of estrogen and progesterone receptors identifies a subset of NSCLCs and correlates with EGFR mutation. Clin Cancer Res 2009;15:5359-68. [Crossref] [PubMed]
- Stabile LP, Davis ALG, Gubish CT, et al. Human non-small cell lung tumors and cells derived from normal lung express both estrogen receptor alpha and beta and show biological responses to estrogen. Cancer Res 2002;62:2141-50. [PubMed]
- Wu AH, Yu MC, Thomas DC, et al. Personal and family history of lung disease as risk factors for adenocarcinoma of the lung. Cancer Res 1988;48:7279-84. [PubMed]
- Baik CS, Strauss GM, Speizer FE, et al. Reproductive factors, hormone use, and risk for lung cancer in postmenopausal women, the Nurses' Health Study. Cancer Epidemiol Biomarkers Prev 2010;19:2525-33. [Crossref] [PubMed]
- Kreuzer M, Gerken M, Heinrich J, et al. Hormonal factors and risk of lung cancer among women? Int J Epidemiol 2003;32:263-71. [Crossref] [PubMed]
- Kabat GC, Miller AB, Rohan TE. Reproductive and hormonal factors and risk of lung cancer in women: a prospective cohort study. Int J Cancer 2007;120:2214-20. [Crossref] [PubMed]
- Gallagher LG, Rosenblatt KA, Ray RM, et al. Reproductive factors and risk of lung cancer in female textile workers in Shanghai, China. Cancer Causes Control 2013;24:1305-14. [Crossref] [PubMed]
- Tan HS, Tan M-H, Chow KY, et al. Reproductive factors and lung cancer risk among women in the Singapore Breast Cancer Screening Project. Lung Cancer 2015;90:499-508. [Crossref] [PubMed]
- Vohra SN, Sapkota A, Lee M-LT, et al. Reproductive and Hormonal Factors in Relation to Lung Cancer Among Nepali Women. Front Oncol 2019;9:311. [Crossref] [PubMed]
- Liu Y, Inoue M, Sobue T, et al. Reproductive factors, hormone use and the risk of lung cancer among middle-aged never-smoking Japanese women: a large-scale population-based cohort study. Int J Cancer 2005;117:662-6. [Crossref] [PubMed]
- Boyko EJ. Observational research--opportunities and limitations. J Diabetes Complications 2013;27:642-8. [Crossref] [PubMed]
- Smith GD, Ebrahim S. Mendelian randomization: prospects, potentials, and limitations. Int J Epidemiol 2004;33:30-42. [Crossref] [PubMed]
- Sekula P, Del Greco M F, Pattaro C, et al. Mendelian Randomization as an Approach to Assess Causality Using Observational Data. J Am Soc Nephrol 2016;27:3253-65. [Crossref] [PubMed]
- Bochud M, Rousson V. Usefulness of Mendelian randomization in observational epidemiology. Int J Environ Res Public Health 2010;7:711-28. [Crossref] [PubMed]
- Pierce BL, Burgess S. Efficient design for Mendelian randomization studies: subsample and 2-sample instrumental variable estimators. Am J Epidemiol 2013;178:1177-84. [Crossref] [PubMed]
- Burgess S, Scott RA, Timpson NJ, et al. Using published data in Mendelian randomization: a blueprint for efficient identification of causal risk factors. Eur J Epidemiol 2015;30:543-52. [Crossref] [PubMed]
- VanderWeele TJ, Tchetgen Tchetgen EJ, Cornelis M, et al. Methodological challenges in mendelian randomization. Epidemiology 2014;25:427-35. [Crossref] [PubMed]
- Hemani G, Zheng J, Elsworth B, et al. The MR-Base platform supports systematic causal inference across the human phenome. Elife 2018;7:e34408 [Crossref] [PubMed]
- Barban N, Jansen R, de Vlaming R, et al. Genome-wide analysis identifies 12 loci influencing human reproductive behavior. Nat Genet 2016;48:1462-72. [Crossref] [PubMed]
- Bulik-Sullivan BK, Loh P-R, Finucane HK, et al. LD Score regression distinguishes confounding from polygenicity in genome-wide association studies. Nat Genet 2015;47:291-5. [Crossref] [PubMed]
- World Medical Association Declaration of Helsinki. ethical principles for medical research involving human subjects. JAMA 2013;310:2191-4. [Crossref] [PubMed]
- Peng H, Li C, Wu X, et al. Association between systemic lupus erythematosus and lung cancer: results from a pool of cohort studies and Mendelian randomization analysis. J Thorac Dis 2020;12:5299-302. [Crossref] [PubMed]
- Wang Y, McKay JD, Rafnar T, et al. Rare variants of large effect in BRCA2 and CHEK2 affect risk of lung cancer. Nat Genet 2014;46:736-41. [Crossref] [PubMed]
- Burgess S, Butterworth A, Thompson SG. Mendelian randomization analysis with multiple genetic variants using summarized data. Genet Epidemiol 2013;37:658-65. [Crossref] [PubMed]
- Bowden J, Davey Smith G, Haycock PC, et al. Consistent Estimation in Mendelian Randomization with Some Invalid Instruments Using a Weighted Median Estimator. Genet Epidemiol 2016;40:304-14. [Crossref] [PubMed]
- Bowden J, Davey Smith G, Burgess S. Mendelian randomization with invalid instruments: effect estimation and bias detection through Egger regression. Int J Epidemiol 2015;44:512-25. [Crossref] [PubMed]
- Heath CW. Environmental tobacco smoke and lung cancer. Lancet 1993;341:526. [Crossref] [PubMed]
- Tobacco and Genetics Consortium. Genome-wide meta-analyses identify multiple loci associated with smoking behavior. Nat Genet 2010;42:441-7. [Crossref] [PubMed]
- Sundermann AC, Zhao S, Young CL, et al. Alcohol Use in Pregnancy and Miscarriage: A Systematic Review and Meta-Analysis. Alcohol Clin Exp Res 2019;43:1606-16. [Crossref] [PubMed]
- Larsson SC, Carter P, Kar S, et al. Smoking, alcohol consumption, and cancer: A mendelian randomisation study in UK Biobank and international genetic consortia participants. PLoS Med 2020;17:e1003178 [Crossref] [PubMed]
- Locke AE, Kahali B, Berndt SI, et al. Genetic studies of body mass index yield new insights for obesity biology. Nature 2015;518:197-206. [Crossref] [PubMed]
- Catalano PM, Shankar K. Obesity and pregnancy: mechanisms of short term and long term adverse consequences for mother and child. BMJ 2017;356:j1. [Crossref] [PubMed]
- Wynder EL, Hebert JR, Kabat GC. Association of dietary fat and lung cancer. J Natl Cancer Inst 1987;79:631-7. [PubMed]
- Willer CJ, Schmidt EM, Sengupta S, et al. Discovery and refinement of loci associated with lipid levels. Nat Genet 2013;45:1274-83. [Crossref] [PubMed]
- Goto A, Yamaji T, Sawada N, et al. Diabetes and cancer risk: A Mendelian randomization study. Int J Cancer 2020;146:712-9. [Crossref] [PubMed]
- Carreras-Torres R, Johansson M, Haycock PC, et al. Obesity, metabolic factors and risk of different histological types of lung cancer: A Mendelian randomization study. PLoS One 2017;12:e0177875 [Crossref] [PubMed]
- Tropf FC, Stulp G, Barban N, et al. Human fertility, molecular genetics, and natural selection in modern societies. PLoS One 2015;10:e0126821 [Crossref] [PubMed]
- Elks CE, Perry JRB, Sulem P, et al. Thirty new loci for age at menarche identified by a meta-analysis of genome-wide association studies. Nat Genet 2010;42:1077-85. [Crossref] [PubMed]
- Rahmioglu N, Nyholt DR, Morris AP, et al. Genetic variants underlying risk of endometriosis: insights from meta-analysis of eight genome-wide association and replication datasets. Hum Reprod Update 2014;20:702-16. [Crossref] [PubMed]
- Mehta D, Tropf FC, Gratten J, et al. Evidence for Genetic Overlap Between Schizophrenia and Age at First Birth in Women. JAMA Psychiatry 2016;73:497-505. [Crossref] [PubMed]
- Mills MC, Tropf FC. The Biodemography of Fertility: A Review and Future Research Frontiers. Kolner Z Soz Sozpsychol 2015;67:397-424. [Crossref] [PubMed]
- Brinton LA, Gierach GL, Andaya A, et al. Reproductive and hormonal factors and lung cancer risk in the NIH-AARP Diet and Health Study cohort. Cancer Epidemiol Biomarkers Prev 2011;20:900-11. [Crossref] [PubMed]
- Ben Khedher S, Neri M, Papadopoulos A, et al. Menstrual and reproductive factors and lung cancer risk: A pooled analysis from the international lung cancer consortium. Int J Cancer 2017;141:309-23. [Crossref] [PubMed]
- Hobcraft J, Kiernan K. Childhood poverty, early motherhood and adult social exclusion. Br J Sociol 2001;52:495-517. [Crossref] [PubMed]
- Jacobs JL. Gender, race, class, and the trend toward early motherhood. A feminist analysis of teen mothers in contemporary society. J Contemp Ethnogr 1994;22:442-62. [Crossref] [PubMed]
- Lawrence EM. Why Do College Graduates Behave More Healthfully than Those Who Are Less Educated? J Health Soc Behav 2017;58:291-306. [Crossref] [PubMed]
- Brown WJ, Kabir E, Clark BK, et al. Maintaining a Healthy BMI: Data From a 16-Year Study of Young Australian Women. Am J Prev Med 2016;51:e165-78. [Crossref] [PubMed]
- Zhou H, Zhang Y, Liu J, et al. Education and lung cancer: a Mendelian randomization study. Int J Epidemiol 2019;48:743-50. [Crossref] [PubMed]
- Bray F, Ferlay J, Soerjomataram I, et al. Global cancer statistics 2018: GLOBOCAN estimates of incidence and mortality worldwide for 36 cancers in 185 countries. CA Cancer J Clin 2018;68:394-424. [Crossref] [PubMed]
- Mao Y, Yang D, He J, et al. Epidemiology of Lung Cancer. Surg Oncol Clin N Am 2016;25:439-45. [Crossref] [PubMed]
- de Groot PM, Wu CC, Carter BW, et al. The epidemiology of lung cancer. Transl Lung Cancer Res 2018;7:220-33. [Crossref] [PubMed]
- Malvezzi M, Carioli G, Bertuccio P, et al. European cancer mortality predictions for the year 2017, with focus on lung cancer. Ann Oncol 2017;28:1117-23. [Crossref] [PubMed]
- Gao S, Li N, Wang S, et al. Lung Cancer in People's Republic of China. J Thorac Oncol 2020;15:1567-76. [Crossref] [PubMed]
- Bray F, Tyczynski JE, Parkin DM. Going up or coming down? The changing phases of the lung cancer epidemic from 1967 to 1999 in the 15 European Union countries. Eur J Cancer 2004;40:96-125. [Crossref] [PubMed]
- Patel JD. Lung cancer in women. J Clin Oncol 2005;23:3212-8. [Crossref] [PubMed]
- Kc R, Shukla SD, Gautam SS, et al. The role of environmental exposure to non-cigarette smoke in lung disease. Clin Transl Med 2018;7:39. [Crossref] [PubMed]
- Parascandola M, Xiao L. Tobacco and the lung cancer epidemic in China. Transl Lung Cancer Res 2019;8:S21-S30. [Crossref] [PubMed]
- Carreras G, Lachi A, Cortini B, et al. Burden of disease from second-hand tobacco smoke exposure at home among adults from European Union countries in 2017: an analysis using a review of recent meta-analyses. Prev Med 2021;145:106412 [Crossref] [PubMed]
- Ni X, Xu N, Wang Q. Meta-Analysis and Systematic Review in Environmental Tobacco Smoke Risk of Female Lung Cancer by Research Type. Int J Environ Res Public Health 2018;15:1348. [Crossref] [PubMed]
- Kure EH, Ryberg D, Hewer A, et al. p53 mutations in lung tumours: relationship to gender and lung DNA adduct levels. Carcinogenesis 1996;17:2201-5. [Crossref] [PubMed]
- Toyooka S, Tsuda T, Gazdar AF. The TP53 gene, tobacco exposure, and lung cancer. Hum Mutat 2003;21:229-39. [Crossref] [PubMed]
- Nelson HH, Christiani DC, Mark EJ, et al. Implications and prognostic value of K-ras mutation for early-stage lung cancer in women. J Natl Cancer Inst 1999;91:2032-8. [Crossref] [PubMed]
- Lan Q, Hsiung CA, Matsuo K, et al. Genome-wide association analysis identifies new lung cancer susceptibility loci in never-smoking women in Asia. Nat Genet 2012;44:1330-5. [Crossref] [PubMed]
- Vodicka P, Andera L, Opattova A, et al. The Interactions of DNA Repair, Telomere Homeostasis, and p53 Mutational Status in Solid Cancers: Risk, Prognosis, and Prediction. Cancers (Basel) 2021;13:479. [Crossref] [PubMed]
- Spitz MR, Wei Q, Dong Q, et al. Genetic susceptibility to lung cancer: the role of DNA damage and repair. Cancer Epidemiol Biomarkers Prev 2003;12:689-98. [PubMed]
- Shriver SP, Bourdeau HA, Gubish CT, et al. Sex-specific expression of gastrin-releasing peptide receptor: relationship to smoking history and risk of lung cancer. J Natl Cancer Inst 2000;92:24-33. [Crossref] [PubMed]
- Kligerman S, White C. Epidemiology of lung cancer in women: risk factors, survival, and screening. AJR Am J Roentgenol 2011;196:287-95. [Crossref] [PubMed]
- Deng F, Li M, Shan W-L, et al. Correlation between epidermal growth factor receptor mutations and the expression of estrogen receptor-β in advanced non-small cell lung cancer. Oncol Lett 2017;13:2359-65. [Crossref] [PubMed]
- Musial C, Zaucha R, Kuban-Jankowska A, et al. Plausible Role of Estrogens in Pathogenesis, Progression and Therapy of Lung Cancer. Int J Environ Res Public Health 2021;18:648. [Crossref] [PubMed]
- Niikawa H, Suzuki T, Miki Y, et al. Intratumoral estrogens and estrogen receptors in human non-small cell lung carcinoma. Clin Cancer Res 2008;14:4417-26. [Crossref] [PubMed]
- Lim WY, Chen Y, Chuah KL, et al. Female reproductive factors, gene polymorphisms in the estrogen metabolism pathway, and risk of lung cancer in Chinese women. Am J Epidemiol 2012;175:492-503. [Crossref] [PubMed]
- Slatore CG, Chien JW, Au DH, et al. Lung cancer and hormone replacement therapy: association in the vitamins and lifestyle study. J Clin Oncol 2010;28:1540-6. [Crossref] [PubMed]
- Chlebowski RT, Anderson GL, Manson JE, et al. Lung cancer among postmenopausal women treated with estrogen alone in the women's health initiative randomized trial. J Natl Cancer Inst 2010;102:1413-21. [Crossref] [PubMed]
- Brinton LA, Schwartz L, Spitz MR, et al. Unopposed estrogen and estrogen plus progestin menopausal hormone therapy and lung cancer risk in the NIH-AARP Diet and Health Study Cohort. Cancer Causes Control 2012;23:487-96. [Crossref] [PubMed]
- Ivanova MM, Mazhawidza W, Dougherty SM, et al. Sex differences in estrogen receptor subcellular location and activity in lung adenocarcinoma cells. Am J Respir Cell Mol Biol 2010;42:320-30. [Crossref] [PubMed]
- Mulac-Jericevic B, Conneely OM. Reproductive tissue selective actions of progesterone receptors. Reproduction 2004;128:139-46. [Crossref] [PubMed]
- Clarke CL, Sutherland RL. Progestin regulation of cellular proliferation. Endocr Rev 1990;11:266-301. [Crossref] [PubMed]
- Musgrove EA, Hunter LJ, Lee CS, et al. Cyclin D1 overexpression induces progestin resistance in T-47D breast cancer cells despite p27(Kip1) association with cyclin E-Cdk2. J Biol Chem 2001;276:47675-83. [Crossref] [PubMed]
- Ishibashi H, Suzuki T, Suzuki S, et al. Progesterone receptor in non-small cell lung cancer--a potent prognostic factor and possible target for endocrine therapy. Cancer Res 2005;65:6450-8. [Crossref] [PubMed]
- Luu-The V, Labrie F. The intracrine sex steroid biosynthesis pathways. Prog Brain Res 2010;181:177-92. [Crossref] [PubMed]
- Mirowsky J. Age at first birth, health, and mortality. J Health Soc Behav 2005;46:32-50. [Crossref] [PubMed]
- Lacey RE, Kumari M, Sacker A, et al. Age at first birth and cardiovascular risk factors in the 1958 British birth cohort. J Epidemiol Community Health 2017;71:691-8. [Crossref] [PubMed]
- Sakai T, Sugawara Y, Watanabe I, et al. Age at first birth and long-term mortality for mothers: the Ohsaki cohort study. Environ Health Prev Med 2017;22:24. [Crossref] [PubMed]