Correlation of peripheral blood biomarkers with clinical outcomes in NSCLC patients with high PD-L1 expression treated with pembrolizumab
Introduction
Lung cancer remains one of the cancers with the highest incidence and mortality rates worldwide (1,2). When considering the two sexes together, lung cancer is the most commonly diagnosed cancer (11.6% of the total cases) and the leading cause of cancer death (18.4% of the total cancer deaths) (2). Moreover, fewer than 19% of patients with lung cancer have a survival rate greater than 5 years from initial diagnosis (1). Despite advances in early diagnosis and therapeutic strategies, prognosis remains poor, primarily due to local recurrences and distant metastases (3). There are two main types of lung cancer, non-small cell lung cancer (NSCLC), which accounts for approximately 85% of all cases, and small cell lung cancer (SCLC), which accounts for 15%. In the clinical landscape of therapies in NSCLC, the use of immune checkpoint inhibitors (ICIs) that target programmed death 1 (PD-1) or its ligand (PD-L1) has improved survival and toxicity compared to standard chemotherapy treatments (4-7). In this regard, pembrolizumab, a highly selective anti-PD-1 humanized monoclonal antibody, is the current standard first-line therapeutic option in patients with advanced NSCLC with PD-L1 expression in greater than 50% of tumour cells (8,9) and in combination with traditional chemotherapy in patients with PD-L1 expression in fewer than 50% of tumour cells (10,11). The outcomes of these studies revealed that pembrolizumab monotherapy or in combination with chemotherapy significantly improved overall survival (OS) and progression-free survival (PFS) compared to chemotherapy alone. However, despite promising results obtained with immunotherapy in NSCLC, only a limited proportion of patients benefit from these treatments with respect to long term survival. Furthermore, many patients with high PD-L1 expression do not respond to ICI treatment, while other patients with low or negative PD-L1 expression do benefit from these treatments (12,13). Due to heterogeneity in the association between PD-L1 expression on tumour cells and the clinical response to ICIs, it is essential to establish biomarkers that help predict which patients will benefit from these therapies. With this aim, several biomarkers have been proposed or are being actively studied. In addition to the aforementioned PD-L1, tumour infiltrating lymphocytes (14), mutational burden (15) and immune gene signatures (16) have also been suggested as potential biomarkers for the response to immunotherapy in lung cancer. However, these biomarkers have several limitations, such as the difficulty obtaining tumour samples, high cost and technical approaches. Furthermore, a large number of studies have reported inconsistent results (17-19), and none of these biomarkers have been confirmed as predictors of response in these patients. Therefore, further studies in this area are necessary to establish the true validity of predictive biomarkers of response to ICIs.
Several studies have suggested that tumour-associated inflammation plays a decisive role in the immune response of patients with malignant neoplasms and could be applied to ICI therapies (20-22). In this context, different blood-derived parameters related to the systemic inflammatory response have been proposed, such as absolute neutrophil count (ANC) (23), neutrophil-to-lymphocyte ratio (NLR) (24), derived neutrophil-to-lymphocyte ratio (dNLR) (25), platelet-to-lymphocyte ratio (PLR) (26), monocyte-to-lymphocyte ratio (MLR) (27), systemic immune-inflammation index (SII) (28) or neutrophil-monocyte-to-lymphocyte ratio (NMLR) (29). In addition, scores involving various blood parameters, such as neutrophil-platelet score (NPS) (30) and inflammatory response biomarker (IRB) (31), have been developed. Of all these blood biomarkers, the most widely studied is certainly NLR.
Focusing on advanced NSCLC patients with PD-L1 expression ≥50% receiving pembrolizumab, Ksienski et al. (32) recently demonstrated that high baseline NLR and PLR levels are associated with reduced OS in patients. However, despite the growing number of studies reporting encouraging results from the use of several baseline biomarkers to predict clinical outcomes of NSCLC patients treated with ICIs, these have not yet been sufficiently conclusive, and other aspects should be taken into account.
Taking into account everything already exposed, the main objective of our study was to analyse the relationship between several baseline peripheral blood biomarkers, including NLR, PLR and MLR, and the effectiveness of the first-line pembrolizumab in NSCLC patients with PD-L1 expression greater than or equal to 50% of tumor cells. In addition, we determined a risk score termed risk blood biomarker (RBB) calculated from the combination of neutrophil-monocyte-to-lymphocyte ratio (NMLR) and white blood cell count (WBC) that enables the stratification of patients by clinical outcome (i.e., treatment response and survival). This score could be a very useful prognostic tool in daily clinical practice in advanced NSCLC patients who are suitable for first-line pembrolizumab. We present the following article in accordance with the REMARK reporting checklist (available at http://dx.doi.org/10.21037/tlcr-21-156).
Methods
Ethics statement
The study was conducted in accordance with the Declaration of Helsinki (as revised in 2013). The study was approved by institutional ethics committee of Hospital Virgen del Rocío and Hospital Virgen Macarena (0944-N-20) and individual consent for this retrospective analysis was waived.
Patients and study design
This retrospective study performed from June 2017 to December 2019 aimed to analyse the potential role of several peripheral blood parameters and the efficacy of first-line pembrolizumab in advanced NSCLC patients. The inclusion criteria were: confirmed stage IV or recurrent NSCLC, PD-L1 ≥50% and use of pembrolizumab monotherapy as first-line treatment. Exclusion criteria were: previous ICI therapy and any driver mutations (EGFR, ALK, ROS1) (Figure S1). Thus, this study included a cohort of 51 subjects treated with 200 mg of pembrolizumab every 3 weeks (21 days) at the Virgen del Rocío University Hospital in Seville, Spain. A complete blood count at baseline was obtained before starting pembrolizumab treatment. The last follow-up period was December 31, 2019.
Statistical analysis
Baseline clinicopathological and demographic characteristics of the patients were collected through December 31, 2019. Descriptive analyses were used to characterize the most relevant clinical variables. Categorical parameters were explored using the Chi-squared test or Fisher’s exact test. The corresponding parametric (t-test) or nonparametric (Wilcoxon test) tests were employed to assess the distribution of continuous variables among study groups. Statistical analyses were performed using Statistical Package for the Social Sciences software (SPSS version 20, Chicago, Illinois, USA).
Several baseline peripheral blood biomarkers, such as white blood cell (WBC) count, absolute neutrophil count (ANC), absolute lymphocyte count (ALC), absolute monocyte count (AMC) and platelet levels at baseline, were analysed. WBC counts were categorized according to the upper limit of normality (ULN). NLR, MLR, PLR and RBB scores (NMLR+WBC) were calculated as reported in Table 1. We determined the optimum cut-off values for NLR, PLR, MLR and NMLR to predict the efficacy and prognosis of patients with NSCLC who received pembrolizumab monotherapy from receiver operating characteristic (ROC) curves. Figure 1 shows ROC curves for the obtained NLR, MLR, PLR and NMLR. The area under the curve (AUC) represents the discriminative power of the test, and the highest AUC value was used to determine the cut-off value. In all cases, both specificity and sensitivity were greater than 62%, and the P value of the ROC curves was <0.05. Based on these analyses, we classified the patients into two groups (low expression and high expression groups).
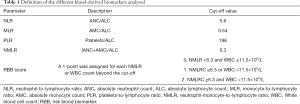
Full table
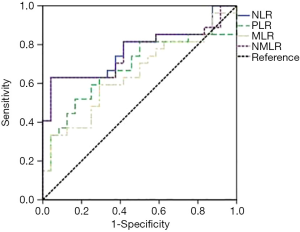
Tumour response was evaluated by computed tomography and assessed with Response Evaluation Criteria in Solid Tumours version 1.1 (33). The objective tumour response was considered the best clinical response during the course of treatment, with the disease control rate (DCR) group defined by patients displaying stable disease (SD), partial response (PR) or complete response (CR) and progressive disease (PD) group. PFS was measured from the time of initiating pembrolizumab treatment to clinical or radiographic progression, death from any cause or was censored on the day of cut-off. OS was measured from the time of initiating pembrolizumab treatment to death from any cause or was censored on the day of cut-off. OS and PFS were calculated using the Kaplan-Meier method and log-rank test. Hazard ratios (HRs) and 95% confidence intervals (CIs) were calculated using the univariate Cox proportional hazard model. Parameters with a value of P<0.05 (considered statistically significant) in the univariate analysis were selected for inclusion in the multivariate analysis.
Results
Patient characteristics
A total of 51 subjects with advanced NSCLC treated with pembrolizumab as the first-line treatment were included in this study. PD-L1 expression levels in tumour cells of all patients were greater than or equal to 50%. Baseline clinicopathological characteristics of the patients are summarized in Table 2. The median age at the start of treatment was 66 years old (range, 46–85), and the majority of patients were male (72.55%). Only 23.53% had an Eastern Cooperative Oncology Group (ECOG) performance status of 2 or more. The most common histological subtype was adenocarcinoma (62.74%), and most patients were current smokers or had quit smoking fewer than 10 years ago (70.59%). Median number of cycles of pembrolizumab administered was 7 (range, 1–28). Median follow-up for the cohort was 6.93 months (range, 0.20–26.19) and only one patient was lost to follow-up.
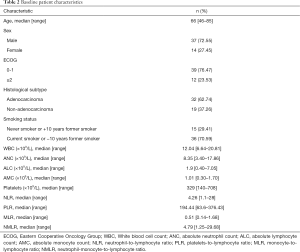
Full table
The relation of blood biomarkers and baseline clinicopathologic characteristics are shown in Table 3. Low baseline NLR was significantly associated with low baseline MLR (P<0.001) and PLR (P<0.001), likewise there was an association between low baseline MLR and PLR (P<0.001). Moreover, using continuous variables, a strong correlation between NLR and PLR (Spearman’s r=0.75, P<0.001), NLR and MLR (Spearman’s r=0.83, P<0.001) and PLR and MLR (Spearman’s r=0.76, P<0.001) was found.
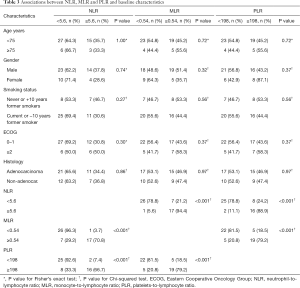
Full table
Baseline immune blood biomarkers and survival outcomes
Pre-treatment baseline results revealed that the median total WBC count was 12.04×109/L (range, 6.64×109/L–20.81×109/L), ANC was 8.35×109/L (range, 3.40×109/L–17.86×109/L) and AMC was 1.01×109/L (range, 0.30×109/L–1.70×109/L). These median values were higher than the ULN, 11.5×109/L, 7.5×109/L and 0.8×109/L, respectively. The median values of NLR, PLR, MLR and NMLR were 4.26 (range, 1.10–28.00), 194.44 (range, 63.60–376.43), 0.51 (range, 0.14–1.68) and 4.79 (range, 1.25–29.68), respectively (Table 2).
For the whole cohort included in the analysis median OS and PFS were 10.64 months (95% CI: 4.5–17.05) and 5.52 months (95% CI: 2.96–8.08) respectively. To classify patients into two groups according to NLR, PLR, MLR and NMLR, we calculated their optimal cut-off values to predict the efficacy of pembrolizumab monotherapy in NSCLC patients and their associated prognosis. The results were a cut-off value (Table 1) of 5.6 (AUC: 0.77, 95% CI: 0.63–0.90) for NLR, 198 (AUC: 0.68, 95% CI: 0.52–0.83) for PLR, 0.54 (AUC: 0.64, 95% CI: 0.49–0.80) for MLR and 6.3 (AUC: 0.76, 95% CI: 0.63–0.90) for NMLR.
For NLR, 33 patients were classified into the low expression group (NLR <5.6), and 18 were classified into the high expression group (NLR ≥5.6). Patients with a lower NLR rate were significantly associated with improved PFS and OS compared to subjects with a higher NLR rate (Figure 2A,B and Table 4). Median PFS was 8.51 months (95% CI: 4.95–12.06) versus 0.70 months (95% CI: 0.37–1.01), HR =0.24, 95% CI: 0.12–0.49, P<0,001. PFS at 12 months was 38.2% in the low NLR group. Median OS was 20.30 months (95% CI: 11.27–29.34) versus 1.21 months (95% CI: 0.12–2.31), HR =0.11, 95% CI: 0.04–0.26, P<0.001. The 12- and 24-month OS in patients in the low NLR group was 73.9% and 30.8%, respectively, while in the high NLR group, none of the patients survived 12 months.
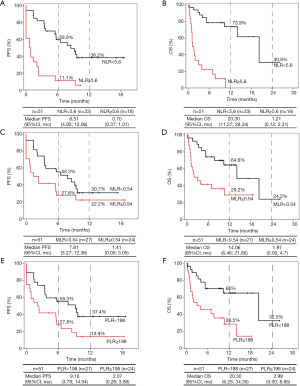
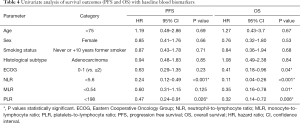
Full table
With respect to MLR, 27 patients were in the low expression group (MLR <0.54), and 24 were in the high expression group (MLR ≥0.54) at baseline. Once again, the low MLR group was significantly associated with improved OS compared to the high MLR group (HR =0.35, 95% CI: 0.16–0.78, P=0.01) but not with improved PFS (HR =0.60, 95% CI: 0.31–1.15, P=0.125) (Figure 2C,D and Table 4). Median PFS was 7.81 months (95% CI, 3.27–12.36) in the group with low expression of MLR versus 1.41 months (95% CI, 0.00–3.05) in the group with high expression of MLR. Median OS was 14.06 months (95% CI, 6.46–21.66) in the group with low expression of MLR versus 1.97 months (95% CI, 0.00–4.70) in subjects with higher MLR. At 12 and 24 months, the OS rates were 64.6% and 24.2%, respectively, in the low MLR group and 29.20% at 12 months in the high MLR group; however, there were no survivors after 24 months in this group.
In the case of PLR, 24 patients had a PLR value greater than or equal to 198 at baseline, and 27 patients had values below 198. As in the case with NLR patients, the low expression group was significantly associated with better PFS (HR =0.47, 95% CI: 0.24–0.91, P=0.026) and OS (HR =0.32, 95% CI: 0.14–0.72, P=0.006) (Figure 2E,F and Table 4). Median PFS and OS were again superior in low PLR patients (PFS: 9.16 months; 95% CI: 3.78–14.54 and OS: 20.30 months; 95% CI: 6.25–34.35) versus high PLR patients (PFS: 2.07 months; 95% CI: 0.26–3.88 and OS: 2.99 months; 95% CI: 0.00–8.85). PFS at 12 months was 37.4% in the low PLR group and 13.9% in the high PLR group. The 12- and 24-month OS rates of patients in the low PLR group were 65% and 32.5%, respectively, while in the high PLR group, they were 28.5% at 12 months, and there were no survivors at 24 months.
Multivariate Cox proportional regression analysis was used to identify independent factors related to the efficacy of pembrolizumab with respect to PFS and OS. Age, ECOG, the two main histological subtypes of NSCLC and smoking status were included as covariates. Blood immune cell biomarkers with a P value <0.05 in the univariate analysis were used in the multivariate analysis. To avoid multicollinearity in the regression analysis, we established independent Cox models for NLR, PLR and MLR. Thus, only one of the three immune indicators was included in each test along with the rest of the covariates. The results, shown in Table 5, revealed that the low NLR (HR: 0.19, 95% CI: 0.09–0.44, P<0.001) and PLR (HR: 0.46, 95% CI: 0.23–0.92, P=0.03) groups were independently associated with improved PFS, and low ECOG (HR: 0.39, 95% CI: 0.16–0.94, P<0.036), NLR (HR: 0.07, 95% CI: 0.02–0.19, P<0.001), MLR (HR: 0.34, 95% CI: 0.15–0.76, P=0.01) and PLR (HR: 0.29, 95% CI: 0.13–0.67, P=0.004) were independently associated with better OS.

Full table
RBB as a prognostic factor for response to pembrolizumab in advanced NSCLC patients
Finally, we hypothesized that the combination of two markers, NMLR and WBC, which provided good results separately (29,34), could be correlated with survival outcomes. Therefore, NMLR and WBC were used to create a new risk score, RBB. We stratified patients into three groups to determine the effect of these combined biomarkers on survival and response in patients with advanced NSCLC. Figure 3 shows Kaplan-Meier curves and univariate analysis for PFS (Figure 3A) and OS (Figure 3B). Univariate analysis for PFS and OS revealed a significant association with better survival when the score groups were decreased (PFS: group 0: HR: 0.18, 95% CI: 0.08–0.42, P<0.001; group 1: HR: 0.34, 95% CI: 0.15–0.77, P=0.01 and OS: group 0: HR: 0.05, 95% CI: 0.01–0.17, P<0.001, group 1: HR: 0.19, 95% CI: 0.07–0.48, P=0.001). We identified OS rates of 83.1% and 33.2% at 12 and 24 months, respectively, for group 0. In group 1, the OS rate was 43% at 12 months. There were no survivors at 24 months in this group. PFS rates at 12 months were 41.5% in group 0 and 26.2% in group 1. On multivariate analysis (Table 6), adjusting for age, ECOG, histologic subtype and smoking status, we corroborated that the RBB score resulting in the combination of baseline NMLR and WBC was independently associated with poor PFS (group 0: HR: 0.16, 95% CI: 0.06–0.41, P<0.001 and group 1: HR: 0.29, 95% CI: 0.12–0.73, P=0.01) and OS (group 0: HR: 0.04, 95% CI: 0.01–0.17, P<0.001 and group 1: HR: 0.15, 95% CI: 0.05–0.42, P<0.001), i.e., the group with lower scores survived longer. Next, we evaluated the relationship between group of RBB score and response to treatment (Figure 4). We observed that 80% of patients in group 0, 56.2% in group 1, and 13.3% in group 2 experienced DCR (SD, PR or CR).

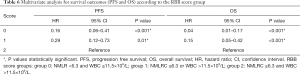
Full table
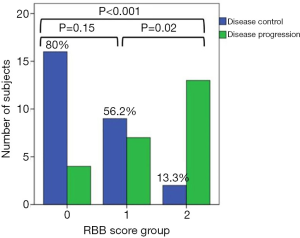
Discussion
In the present study, we retrospectively investigated the usefulness of baseline NLR, MLR and PLR to determine their use as prognostic biomarkers. We correlated the association among NLR, MLR, and PLR with PFS and OS in NSCLC patients with high PD-L1 expression treated with pembrolizumab. In addition, we developed the RBB score, which involves several inflammatory parameters. This risk score demonstrated that high levels are a prognostic and predictive marker of inferior survival and poor response to therapy.
One of the main limitations in the use of ICIs is the limited proportion of patients who respond to treatment when stratified by PD-L1 (12,13). Thus, several blood cell biomarkers have been studied to help stratify these patients. It is well known that inflammation plays a key role in the growth and spread of many types of tumours. High levels of tumour-associated neutrophils (TANs) and tumour-associated macrophages (TAMs) derived from peripheral neutrophil and monocyte precursors, respectively, have been described as mediators in tumour progression because they promote the growth of malignant cells by inhibiting apoptosis and assisting angiogenesis, which promotes the formation of metastases (35,36). Likewise, high platelet counts have been proposed as a key factor in tumour angiogenesis (37), which can protect circulating tumour cells from immune attack and help these cells metastasize through blood transmission (38). In contrast, low lymphocyte values are associated with an impaired cellular immune response (39) since lymphocytes are involved in the regulation of host cell-mediated immunity, which is important for destroying residual malignant cells and related micrometastases (31). Therefore, lymphocytes play a key role in the antitumour immunity, and tumour-infiltrating lymphocytes (TILs) have been demonstrated to be correlated with improved clinical outcomes in patients with solid tumours (39).
The results of our analysis for baseline NLR, PLR and MLR revealed the relationship between low values and better survival outcomes, confirming the aforementioned exposure. The current findings are in agreement with those reported in several previous studies (24,26,27,32,40). Zhang et al. (40) performed a meta-analysis of 1,845 NSCLC patients treated with three ICIs (nivolumab, atezolizumab and pembrolizumab), evaluating the influence of NLR (20 studies and 1,817 patients) and PLR (8 studies and 686 patients) on survival outcomes. They reported that elevated NLR values were associated with unfavourable survival outcomes (OS: HR =2.50, 95% CI: 1.79–3.51, P<0.001 and PFS: HR =1.77, 95% CI: 1.51–2.01, P<0.001). Similarly, the results for PLR showed that elevated values were related to poor survival (OS: HR =1.93, 95% CI: 1.51–2.01, P<0.001 and PFS: HR =1.57, 95% CI: 1.30–1.90, P<0.001). Furthermore, in a study of a large cohort (1,466 cases) of patients who underwent curative resection with stage I-IIIA NSCLC, Yuan et al. (27) concluded that elevated MLR was an independent predictor for poor OS (HR =1.25, 95% CI: 1.01–1.54, P=0.035). Likewise, our results indicated that MLR is an independent factor for OS but not for PFS. More recently, Ksienski et al. (32) investigated the association of survival outcomes with baseline NLR and PLR in advanced NSCLC patients with PD-L1 ≥50% treated with pembrolizumab, and the cut-off values for NLR and PLR were 6.4 and 441.8, respectively. The authors concluded that high baseline NLR and PLR were associated with worse OS (NLR HR =2.31, 95% CI: 1.46–3.64, P<0.001, PLR HR =2.03, 95% CI: 1.22–3.37, P=0.006).
One of the most controversial and limiting issues in the application of blood biomarkers as prognostic factors for use in clinical practice is identifying the optimal cut-off value. Different reference cut-off points have been used in the literature (40). Discrepancies in these values could be attributed to various factors, such as baseline differences in the patient population, the timing of sample collection in relation to the treatment and statistical methods (41). Our optimal cut-off values of 5.6, 198 and 0.54 for NLR, PLR and MLR, respectively, were determined using ROC curves. These values are similar to the cut-off values tested in other studies (41-44). Nevertheless, discrepancies in the cut-off values make the use of these markers in clinical practice difficult, and studies with larger populations are needed to determine optimal cut-off values. Despite these discrepancies, the conclusion was similar in all studies: high baseline ratios in patients with NSCLC treated with ICIs are correlated with poor survival outcomes.
In the last part of this study, we developed the RBB score based on NMLR and WBC. The NSCLC population was stratified into 3 groups according to both parameters. We hypothesized that the combination of baseline NMLR and WBC would be correlated with survival outcomes in patients with advanced NSCLC treated with pembrolizumab. For this hypothesis, we based on that WBC, composed primarily of neutrophils, monocytes and lymphocytes, are key markers of immune function and inflammatory response, and several studies have shown that high levels of baseline WBC are correlated with poor prognosis in NSCLC patients (34,45-47). Furthermore, NMLR, which groups ANC, AMC and ALC, has been described as a powerful marker for survival outcomes in patients with different solid tumours (29,48). Our results for WBC and NMLR are in good agreement with those described in the bibliography for both PFS and OS (Figure S2 and Table S1). However, a problem we observed in NMLR, as well as in NLR and MLR, is that there are patients with low values of these ratios, even though they exhibit high individual count values (ANC, AMC and ALC), and patients with these characteristics are included in the low group ratios. To solve this problem and considering the aforementioned exposure, we study NMLR with a cut-off value of 6.3 (obtained from the ROC curve) and WBC level greater than the upper limit of normal, creating a new group (group 1) that takes into account not only the ratio but also the fact that the values of the studied parameters are altered. Thus, we stratified patients into three groups based on these two parameters. In our study, group 2 (high NMLR and WBC levels) was significantly associated with the worst response to ICIs since 13.3% had DCR as their best response compared to group 1 (DCR: 56.2%) and group 0 (DCR: 80%). In addition, group 2 patients exhibited both shorter PFS (median 0.69 months) and OS (median 1.21 months) compared to groups 1 (PFS median 3.48 months; OS median 10.64 months) and 0 (PFS median 9.39 months; OS median 20.30 months).
There are other systemic inflammation-based scores that have been evaluated in patients with advanced NSCLC, such as the Glasgow Prognostic Score (GPS) (49), based on albumin and C-reactive protein, the prognostic index (50) based on CRP and WBC or the Aarhus composite biomarker score (ACBS) (44) based on albumin, CRP, neutrophils, lymphocytes and haemoglobin. However, these scores require measurement of two proteins, which makes their implementation difficult since the mentioned proteins are not routinely evaluated at many centres.
The important clinical significance of the current research is that the baseline blood biomarkers and their combination in the form of ratios and scores not only predicted the prognostic survival outcomes of NSCLC patients but may also help in developing treatment strategies. However, this is a retrospective study, and it has several limitations that must be taken into account when interpreting the conclusions. First, the small number of patients in our cohort might not be sufficient to support the stability of the results. Second, comorbidities and metastasis have not been taken into account in survival studies. Third, we utilized an ROC curve-derived optimum cut-off of variables as the grouping criterion since a standard cut-off value of NLR, MLR, PLR and NMLR has not been defined clearly in NSCLC; therefore, a unified cut-off value is needed. The statistical significance of the prognostic effect of our biomarkers was preceded by a determination of the cut-off point of the same sample, therefore the results may be magnified. This cut-off point should be validated by an independent cohort. Finally, this was a single-arm study, and all patients received pembrolizumab treatment. Thus, to study the relationship between the predictive effect of blood biomarkers and survival outcomes, a multicentre, randomized, double-arm study is needed.
In conclusion, due to the limited number of patients who benefit from immunotherapy, there is a need for identification of biomarkers that enable better stratification. In this study, we demonstrated prognostic value for survival outcomes of baseline blood biomarkers. Finally, we developed an easy-to-apply, novel prognostic scoring system, the RBB score, using NMLR and WBC. This novel score predicts survival (PFS and OS) and response to therapy in NSCLC patients treated with pembrolizumab. Therefore, if this score is validated in a prospective study, it could be useful to help stratify NSCLC patients in future trials.
Acknowledgments
The authors thank American Journal Experts (AJE) team for her professional editing and technical assistance with the manuscript.
Funding: SMP was funded by the Fundación Mutua Madrileña (2014), Ministry of Health and Social Welfare of Junta de Andalucía (PI-0046-2012, Nicolas Monardes Program RC-0004-2020 and OH-0022-2018), ISCIII (PI17/00033 and PI20/01109) and co-funded by FEDER from Regional Development European Funds (European Union). LB was funded by the Ministry of Health and Social Welfare of Junta de Andalucía (RH-0051-2020).
Footnote
Reporting Checklist: The authors have completed the REMARK reporting checklist. Available at http://dx.doi.org/10.21037/tlcr-21-156
Data Sharing Statement: Available at http://dx.doi.org/10.21037/tlcr-21-156
Peer Review File: Available at http://dx.doi.org/10.21037/tlcr-21-156
Conflicts of Interest: All authors have completed the ICMJE uniform disclosure form (available at http://dx.doi.org/10.21037/tlcr-21-156). The authors have no conflicts of interest to declare.
Ethical Statement: The authors are accountable for all aspects of the work in ensuring that questions related to the accuracy or integrity of any part of the work are appropriately investigated and resolved. The study was conducted in accordance with the Declaration of Helsinki (as revised in 2013). The study was approved by institutional ethics committee of Hospital Virgen del Rocío and Hospital Virgen Macarena (0944-N-20) and individual consent for this retrospective analysis was waived.
Open Access Statement: This is an Open Access article distributed in accordance with the Creative Commons Attribution-NonCommercial-NoDerivs 4.0 International License (CC BY-NC-ND 4.0), which permits the non-commercial replication and distribution of the article with the strict proviso that no changes or edits are made and the original work is properly cited (including links to both the formal publication through the relevant DOI and the license). See: https://creativecommons.org/licenses/by-nc-nd/4.0/.
References
- Siegel RL, Miller KD, Jemal A. Cancer statistics, 2019. CA Cancer J Clin 2019;69:7-34. [Crossref] [PubMed]
- Bray F, Ferlay J, Soerjomataram I, et al. Global cancer statistics 2018: GLOBOCAN estimates of incidence and mortality worldwide for 36 cancers in 185 countries. CA Cancer J Clin 2018;68:394-424. [Crossref] [PubMed]
- Wang Y, Li Y, Chen P, et al. Prognostic value of the pretreatment systemic immune-inflammation index (SII) in patients with non-small cell lung cancer: a meta-analysis. Ann Transl Med 2019;7:433. [Crossref] [PubMed]
- Brahmer J, Reckamp KL, Baas P, et al. Nivolumab versus docetaxel in advanced squamous-cell non-small-cell lung cancer. N Engl J Med 2015;373:123-35. [Crossref] [PubMed]
- Borghaei H, Paz-Ares L, Horn L, et al. Nivolumab versus docetaxel in advanced nonsquamous non-small-cell lung cancer. N Engl J Med 2015;373:1627-39. [Crossref] [PubMed]
- Rittmeyer A, Barlesi F, Waterkamp D, et al. Atezolizumab versus docetaxel in patients with previously treated non-small-cell lung cancer (OAK): a phase 3, open-label, multicentre randomised controlled trial. Lancet 2017;389:255-65. [Crossref] [PubMed]
- Herbst RS, Baas P, Kim DW, et al. Pembrolizumab versus docetaxel for previously treated, PD-L1-positive, advanced non-small-cell lung cancer (KEYNOTE-010): A randomised controlled trial. Lancet 2016;387:1540-50. [Crossref] [PubMed]
- Reck M, Rodríguez-Abreu D, Robison AG, et al. Pembrolizumab versus Chemotherapy for PD-L1–Positive Non–Small-Cell Lung Cancer. N Engl J Med 2016;375:1823-33. [Crossref] [PubMed]
- Mok TSK, Wu Y, Kudaba I, et al. Pembrolizumab versus chemotherapy for previously untreated, PD-L1-expressing, locally advanced or metastatic non-small-cell lung cancer (KEYNOTE-042): a randomised, open-label, controlled, phase 3 trial. Lancet 2019;393:1819-30. [Crossref] [PubMed]
- Gandhi L, Rodríguez-Abreu D, Gadgeel S, et al. Pembrolizumab plus chemotherapy in metastatic non-small-cell lung cancer. N Engl J Med 2018;378:2078-92. [Crossref] [PubMed]
- Paz-Ares L, Luft A, Vicente D, et al. Pembrolizumab plus chemotherapy for squamous non-small-cell lung cancer. N Engl J Med 2018;379:2040-51. [Crossref] [PubMed]
- Zhang M, Yang J, Hua W, et al. Monitoring checkpoint inhibitors: predictive biomarkers in immunotherapy. Front Med 2019;13:32-44. [Crossref] [PubMed]
- Havel JJ, Chowell D, Chan TA, et al. The evolving landscape of biomarkers for checkpoint inhibitor immunotherapy. Nat Rev Cancer 2019;19:133-50. [Crossref] [PubMed]
- Zeng DQ, Yu YF, Ou QY, et al. Prognostic and predictive value of tumor-infiltrating lymphocytes for clinical therapeutic research in patients with non-small cell lung cancer. Oncotarget 2016;7:13765-81. [Crossref] [PubMed]
- Rizvi NA, Hellmann MD, Snyder A, et al. Mutational landscape determines sensitivity to PD-1 blockade in non–small cell lung cancer. Science 2015;348:124-8. [Crossref] [PubMed]
- Hwang S, Kwon AY, Jeong JY, et al. Immune gene signatures for predicting durable clinical benefit of anti-PD-1 immunotherapy in patients with non-small cell lung cancer. Sci Rep 2020;10:643. [Crossref] [PubMed]
- Gibney GT, Weiner LM, Atkins MB. Predictive biomarkers for checkpoint inhibitor-based immunotherapy. Lancet Oncol 2016;17:e542-51. [Crossref] [PubMed]
- Taube JM, Klein A, Brahmer JR, et al. Association of PD-1, PD-1 ligands, and other features of the tumor immune microenvironment with response to anti-PD-1 therapy. Clin Cancer Res 2014;20:5064-74. [Crossref] [PubMed]
- Topalian SL, Taube JM, Anders RA, et al. Mechanism-driven biomarkers to guide immune checkpoint blockade in cancer therapy. Nat Rev Cancer 2016;16:275-87. [Crossref] [PubMed]
- Dougan M, Dranoff G. Immune Therapy for Cancer. Annu Rev Immunol 2009;27:83-117. [Crossref] [PubMed]
- Elinav E, Nowarski R, Thaiss CA, et al. Inflammation-induced cancer: Crosstalk between tumours, immune cells and microorganisms. Nat Rev Cancer 2013;13:759-71. [Crossref] [PubMed]
- Crusz SM, Balkwill FR. Inflammation and cancer: Advances and new agents. Nat Rev Clin Oncol 2015;12:584-96. [Crossref] [PubMed]
- Tanizaki J, Haratani K, Hayashi H, et al. Peripheral Blood Biomarkers Associated with Clinical Outcome in Non–Small Cell Lung Cancer Patients Treated with Nivolumab. J Thorac Oncol 2018;13:97-105. [Crossref] [PubMed]
- Jiang T, Bai Y, Zhou F, et al. Clinical value of neutrophil-to-lymphocyte ratio in patients with non-small-cell lung cancer treated with PD-1/PD-L1 inhibitors. Lung Cancer 2019;130:76-83. [Crossref] [PubMed]
- Russo A, Franchina T, Ricciardi GRR, et al. Baseline neutrophilia, derived neutrophil-to-lymphocyte ratio (dNLR), platelet-to-lymphocyte ratio (PLR), and outcome in non small cell lung cancer (NSCLC) treated with Nivolumab or Docetaxel. J Cell Physiol 2018;233:6337-43. [Crossref] [PubMed]
- Diem S, Schmid S, Krapf M, et al. Neutrophil-to-Lymphocyte ratio (NLR) and Platelet-to-Lymphocyte ratio (PLR) as prognostic markers in patients with non-small cell lung cancer (NSCLC) treated with nivolumab. Lung Cancer 2017;111:176-81. [Crossref] [PubMed]
- Yuan C, Li N, Mao X, et al. Elevated pretreatment neutrophil/white blood cell ratio and monocyte/lymphocyte ratio predict poor survival in patients with curatively resected non-small cell lung cancer: Results from a large cohort. Thorac Cancer 2017;8:350-8. [Crossref] [PubMed]
- Guo W, Cai S, Zhang F, et al. Systemic immune-inflammation index (SII) is useful to predict survival outcomes in patients with surgically resected non-small cell lung cancer. Thorac Cancer 2019;10:761-8. [Crossref] [PubMed]
- Xue W, Xu X, Tan Y, et al. Evaluating and validating the predictive ability of preoperative systemic inflammatory/immune cells in gastric cancer following R0 resection. Oncol Lett 2019;18:5205-14. [Crossref] [PubMed]
- Watt DG, Proctor MJ, Park JH, et al. The neutrophil-platelet score (NPS) predicts survival in primary operable colorectal cancer and a variety of common cancers. PLoS One 2015;10:e0142159 [Crossref] [PubMed]
- Wang Y, Hu X, Xu W, et al. Prognostic value of a novel scoring system using inflammatory response biomarkers in non-small cell lung cancer: A retrospective study. Thorac Cancer 2019;10:1402-11. [Crossref] [PubMed]
- Ksienski D, Wai ES, Alex D, et al. Prognostic significance of the neutrophil-to-lymphocyte ratio and platelet-to-lymphocyte ratio for advanced non-small cell lung cancer patients with high PD-L1 tumor expression receiving pembrolizumab. Transl Lung Cancer Res 2021;10:355-67. [Crossref] [PubMed]
- Eisenhauer EA, Therasse P, Bogaerts J, et al. New response evaluation criteria in solid tumours: Revised RECIST guideline (version 1.1). Eur J Cancer 2009;45:228-47. [Crossref] [PubMed]
- Tibaldi C, Vasile E, Bernardini I, et al. Baseline elevated leukocyte count in peripheral blood is associated with poor survival in patients with advanced non-small cell lung cancer: A prognostic model. J Cancer Res Clin Oncol 2008;134:1143-9. [Crossref] [PubMed]
- Gu XB, Tian T, Tian X, et al. Prognostic significance of neutrophil-to-lymphocyte ratio in non-small cell lung cancer : a meta- analysis. Sci Rep 2015;5:12493. [Crossref] [PubMed]
- Galdiero MR, Garlanda C, Jaillon S, et al. Tumor associated macrophages and neutrophils in tumor progression. J Cell Physiol 2013;228:1404-12. [Crossref] [PubMed]
- Pinedo HM, Verheul HMW, D’Amato RJ, et al. Involvement of platelets in tumour angiogenesis? Lancet 1998;352:1775-7. [Crossref] [PubMed]
- Li N. Platelets in cancer metastasis: To help the “villain” to do evil. Int J Cancer 2016;138:2078-87. [Crossref] [PubMed]
- Gooden MJM, De Bock GH, Leffers N, et al. The prognostic influence of tumour-infiltrating lymphocytes in cancer: A systematic review with meta-analysis. Br J Cancer 2011;105:93-103. [Crossref] [PubMed]
- Zhang N, Jiang J, Tang S, et al. Predictive value of neutrophil-lymphocyte ratio and platelet-lymphocyte ratio in non-small cell lung cancer patients treated with immune checkpoint inhibitors: A meta-analysis. Int Immunopharmacol 2020;85:106677 [Crossref] [PubMed]
- Soyano AE, Dholaria B, Marin-Acevedo JA, et al. Peripheral blood biomarkers correlate with outcomes in advanced non-small cell lung Cancer patients treated with anti-PD-1 antibodies. J Immunother Cancer 2018;6:129-37. [Crossref] [PubMed]
- Wang L, Liang D, Xu X, et al. The prognostic value of neutrophil to lymphocyte and platelet to lymphocyte ratios for patients with lung cancer. Oncol Lett 2017;14:6449-56. [Crossref] [PubMed]
- Bagley SJ, Kothari S, Aggarwal C, et al. Pretreatment neutrophil-to-lymphocyte ratio as a marker of outcomes in nivolumab-treated patients with advanced non-small-cell lung cancer. Lung Cancer 2017;106:1-7. [Crossref] [PubMed]
- Sandfeld-Paulsen B, Meldgaard P, Sorensen BS, et al. The prognostic role of inflammation-scores on overall survival in lung cancer patients. Acta Oncol 2019;58:371-6. [Crossref] [PubMed]
- Hao L, Zhang J, Di Y, et al. Prognostic value of white blood cells detected for the first time after adjuvant chemotherapy in primary operable non-small cell lung cancer. Technol Cancer Res Treat 2018;17:1533033818802813 [Crossref] [PubMed]
- Tomita M, Shimizu T, Hara M, et al. Preoperative leukocytosis, anemia and thrombocytosis are associated with poor survival in non-small cell lung cancer. Anticancer Res 2009;29:2687-90. [PubMed]
- Mandrekar SJ, Schild SE, Hillman SL, et al. A prognostic model for advanced stage nonsmall cell lung cancer: Pooled analysis of north central cancer treatment group trials. Cancer 2006;107:781-92. [Crossref] [PubMed]
- Liao R, Peng C, Li M, et al. Comparison and validation of the prognostic value of preoperative systemic immune cells in hepatocellular carcinoma after curative hepatectomy. Cancer Med 2018;7:1170-82. [Crossref] [PubMed]
- Zhu L, Chen S, Ma S, et al. Glasgow prognostic score predicts prognosis of non-small cell lung cancer: A Meta-Analysis. Springerplus 2016;5:439. [Crossref] [PubMed]
- Proctor MJ, Morrison DS, Talwar D, et al. A comparison of inflammation-based prognostic scores in patients with cancer. A Glasgow Inflammation Outcome Study. Eur J Cancer 2011;47:2633-41. [Crossref] [PubMed]