Serum tumor markers for the prediction of concordance between genomic profiles from liquid and tissue biopsy in patients with advanced lung adenocarcinoma
Introduction
In the era of precision medicine, molecular testing is the cornerstone of precision therapies (1). Traditionally, tumor tissue-based pathological diagnosis and molecular profiling have been regarded as the gold standard for clinical diagnosis and treatment decisions. Plasma peripheral circulating free DNA (cfDNA) mainly derives from apoptotic or necrotic cells. In patients with cancer, cfDNA contains DNA from normal cells and that from tumor cells. The latter is known as cell-free circulating tumor DNA (ctDNA). With the advancements of sequencing technologies and liquid biopsy, the detection of ctDNA from peripheral blood has been widely used for treatment guidance and even for early detection in various solid tumors (2-11). Liquid biopsy is considered as a surrogate when tissue biopsy is difficult to obtain. Recently, the noninvasive nature of liquid biopsy and its ability to overcome tumor heterogeneity have attracted the attention of clinicians (2,12).
Although liquid biopsy has certain advantages in overcoming tumor heterogeneity, a major concern remains regarding whether the mutational profile derived from cfDNA can reliably capture the mutational profile of the tumor tissue, especially for treatment guidance in advanced lung adenocarcinoma patients in clinical practice. Several studies have compared the concordance of liquid biopsy and tissue biopsy in solid tumors. Kuderer et al. (13) reported a low concordance between liquid biopsy and tissue biopsy assayed using next generation sequencing (NGS)-based targeted panels from 2 companies in 9 patients with solid tumors including breast cancer, lung cancer, salivary gland cancer, thymic carcinoma, and pancreatic cancer. Other studies indicated higher concordance between liquid biopsy and tissue biopsy in breast cancer and solid tumor patients (14,15). Of note, in these studies, many plasma samples were collected during treatment when ctDNA levels were low (12). Furthermore, various factors, including spatial and temporal heterogeneity, interval treatment, the presence of subclonal mutations, and potential germline DNA contamination, can contribute to discordant tissue and plasma profiles (15). It is generally thought that tumor burden and ctDNA content are major factors influencing the concordance between liquid and tissue biopsy. When some of the above-mentioned external factors were minimized, the concordance rates significantly improved to 80–90% between plasma and tissue samples in various solid tumors (6,16). In advanced non-small cell lung cancer (NSCLC), most alterations detected by NGS-based methods from tissue biopsy can also be accurately detected from matched ctDNA (17).
In clinical practice, there is an urgent need to develop an easily detectable and reliable biomarker to predict the degree of concordance between liquid biopsy and tissue biopsy. Serum tumor markers have been widely used for early diagnosis (18) and prognosis (19) in lung cancer with the advantages of convenience, inexpensiveness and noninvasiveness. Examination of groups of serum tumor markers is more practical and has been more widely applied (20,21). More importantly, serum tumor marker testing is inexpensive and easy to do. Since a few studies have indicated that the proportion of ctDNA from the cfDNA reflected by the maximum allelic fraction is a reliable predictor of the concordance (6,16,17), we hypothesized that the concentration of serum tumor markers in the peripheral blood may also reflect the fraction of ctDNA and may serve as a biomarker to predict concordance between liquid biopsy and tissue biopsy. Here, we evaluated the feasibility of using serum tumor marker concentration for predicting the concordance of genomic profiles obtained from prospectively paired plasma and tissue samples of treatment-naïve patients diagnosed with lung adenocarcinoma. We present the following article in accordance with the REMARK reporting checklist (available at https://dx.doi.org/10.21037/tlcr-21-543).
Methods
Subjects
We prospectively and simultaneously collected genomic profiles of paired tissue and plasma samples from 185 patients newly diagnosed with stage IIIB or IV lung adenocarcinoma who submitted samples for NGS testing at initial diagnosis prior to any systemic treatment from 11 cancer centers in China between January 2017 to July 2020. Inclusion was limited to patients with paired tumor biopsy and blood samples taken within a span of 2 weeks, with the paired samples sequenced using the same gene panel. In all, 88 fresh tissues and 97 formalin-fixed paraffin-embedded (FFPE) tissue samples were collected. Serum tumor marker levels were also examined and recorded. This study was performed in accordance with the Declaration of Helsinki (as revised in 2013), and was approved by the Ethics Committee of the Changzheng Hospital (approval number: 2017SL016). Written informed consent was obtained from all patients included in the study.
Preparation of tissue DNA and plasma cfDNA
DNA isolation and targeted sequencing were performed by Burning Rock Biotech, a College of American Pathologist (CAP)-accredited/Clinical Laboratory Improvement Amendments (CLIA)-certified clinical laboratory, according to a previously described method (22). Tissue DNA and plasma cfDNA were extracted using the QIAamp DNA formalin-fixed paraffin-embedded tissue kit (Qiagen, Hilden, Germany) and QIAamp Circulating Nucleic Acid kit (Qiagen, Hilden, Germany), respectively, following the manufacturer’s instructions. DNA shearing was performed on tissue DNA using a Covaris M220 ultrafocused sonicator. Fragments of 200–400 bp were purified (Agencourt AMPure XP Kit, Beckman Coulter, CA, USA), hybridized with capture probe baits, selected with magnetic beads, and amplified. Fragment quality and size were assessed using a Qubit 2.0 fluorimeter with the double-stranded DNA (dsDNA) high-sensitivity assay kit (Life Technologies, CA, USA). Target capture was performed using a panel consisting of 520 genes (OncoScreen Plus panel) or 168 genes (LungPlasma panel), interrogating 1.64 and 0.273 megabases (Mb) of the human genome, respectively. Indexed samples were sequenced on a Nextseq500 (Illumina, Inc., CA, USA) with paired-end reads and a target sequencing depth of 1,000× for tissue samples and 10,000× for liquid biopsy samples.
Sequence data analysis
All the reads were mapped to the reference human genome (hg19) with a Burrows-Wheeler Aligner (BWA) v.0.7.10 (23). Local alignment optimization, duplication marking, and variant calling were performed using the Genome Analysis Tool Kit v3.2 (24) and VarScan v.2.4.3 (25). Tissue samples were compared against their own white blood cell control to identify somatic variants. Variants were filtered using the VarScan fpfilter pipeline, and loci with a depth less than 100 were filtered out. In both tissue and plasma samples, at least 2 and 5 supporting reads were needed for insertion or deletions (INDELs), while 8 supporting reads were needed to call single-nucleotide variants (SNVs). According to the ExAC, 1000 Genomes, The Single Nucleotide Polymorphism Database (dbSNP), or ESP6500SI-V2 databases, variants with a population frequency over 0.1% were grouped as single nucleotide polymorphisms and excluded from further analysis. Remaining variants were annotated with ANNOVAR (26) and SnpEff v3.6 (27). DNA translocation analysis was performed using Factera version 1.4.3 (28). Copy number variations (CNVs) were analyzed based on the depth of coverage data of capture intervals. Coverage data were corrected against sequencing bias resulting from genetic concordance content and probe design. The limit of detection for CNVs was 1.5 for copy number deletions and 2.64 for CNVs.
Serum tumor biomarker assessment
Based on clinical application and literature reports (29-31), we focused on the following tumor markers: carcinoembryonic antigen (CEA, 0–5 µg/L), carbohydrate antigen 19-9 (CA19-9, 0–39 U/mL), carbohydrate antigen 125 (CA125, 0–35 U/mL), neuron-specific enolase (NSE, 0–16.3 µg/mL), squamous cell-associated antigen (SCC, 0–1.5 ng/mL), and cytokeratin 19 (CYFRA21-1, 0–3.3 µg/L).
Classification of concordance between genomic profiles obtained from tissue and plasma
The primary outcome measurement in the present study was the concordance of the testing results from paired tissue and blood samples. The main consideration for defining the concordance classification was clinical relevance to therapeutic decisions for patients with advanced lung adenocarcinoma. Given that targeted therapy and immunotherapy are the 2 major treatment options for patients with advanced lung adenocarcinoma, the patients were classified according to the number of clinically relevant genes that were found to be concordant between their paired tissue and blood samples into 3 classes based on different therapeutic and prognostic significance. If both the plasma and tissue samples from a given patient are detected with same alteration(s) or without any designated alteration, the patient was considered as to have concordant profiles.
Class 1 was defined as concordance of all targetable driver mutations in 8 genes, namely, EGFR, ALK, ROS1, RET, MET, NTRK, BRAF, and HER2, between the paired tissue and blood samples (Table S1 summarizes the list of targetable driver mutations). Class 2 was defined as concordance in 11 genes, including all targetable driver alterations in class 1 plus mutations in KRAS, STK11, and TP53, between the paired tissue and blood samples. It should be noted that only specific mutations in TP53 were taken into consideration for the concordance analysis. This criterion was based on studies demonstrating that the functional effect of TP53 mutations is dependent on the site of mutation (32). Class 3 was defined as the concordance in the same 11 genes as class 2 plus concordance of tumor mutation burden (TMB) high or low using 10 mutations/Mb as the cutoff. According to the KEYNOTE-158 study, a cutoff of TMB ≥10 mutations/Mb serves as an indicator for potential benefit from use of an immune checkpoint inhibitor (ICI) from monotherapy in previously treated solid tumors. We used the cutoff from the KEYNOTE-158 study to define TMB high and low for both tissue and liquid biopsies.
Generation of scoring system for predicting concordance of mutation profiles
A combined sum score was developed by incorporating the relative fold changes of CEA, CA19-9, CYFRA21-1, and CA125 compared with their upper thresholds of clinical reference ranges according to the following formula:
Statistical analyses
Statistical analyses were performed using R version 3.3.3 software (R Foundation for Statistical Computing). Differences in the groups were calculated and presented using Fisher’s exact test and paired two-tailed Student’s t-test for proportions and continuous variables, respectively. Pearson correlation was performed to study the correlation between the plasma maximum allele fraction (maxAF) and the concentration of serum tumor makers. A logistic regression model was constructed to calculate a genetic concordance score (GCS). A receiver operating characteristic (ROC) curve was drawn based on the predictive likelihood, and an optimal cutoff value was identified by maximizing the Youden Index. P values <0.05 were considered statistically significant.
Results
Patients
A total of 185 treatment-naïve patients with advanced lung adenocarcinoma were enrolled in the study. The cohort included 59.5% (n=110) males and 40.5% (n=75) females with a median age of 64 years. Of the 185 patients, 87.6% were diagnosed with stage IV disease (Table 1), and the remaining had stage IIIB disease. All patients had their plasma samples sequenced in parallel with their paired tumor tissue samples. Pulmonary tissue samples were obtained via surgery from 8 patients, and through endoscopy and puncture biopsy from 19 and 157 patients, respectively. One patient provided pleural effusion sample for comparison.
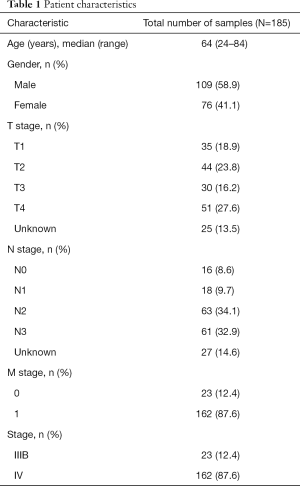
Full table
Genomic profile concordance between paired tissue and plasma samples
Overall, 98.9% of the tissue samples (183/185) had mutations identified from the panel, and only 2 samples had no mutation detected. EGFR was the most frequently mutated driver gene, with 49% of patients harboring mutations in this gene, followed by KRAS (16%), ALK (10%), BRAF (10%), ERBB2 (7%), and MET (6%). In most cases, mutations in driver genes were mutually exclusive. TP53 was the most commonly co-mutated gene with a 73% mutation rate. STK11, a negative prognostic predictor for the efficacy of ICI treatment, had a mutation rate of 8% in this cohort (Figure S1). Of the 185 plasma samples, 151 (81.6%) had at least 1 mutation. The mutation rates for driver genes were 37% for EGFR, 11% for KRAS, 6% for ALK, 5% for ERBB2, 3% for BRAF, and 5% for MET in plasma samples, which were slightly lower than those in tissues. Mutation rates were 54% for TP53 and 5% for STK11 (Figure S2).
Collectively, 150 out of 185 patients had mutations in both tissues and plasma samples, while 1 patient was mutation-negative for both tissue and plasma, resulting a concordance of 81.6%. Of the 34 patients with inconsistent results, 33 (17.8%) patients had mutations in the tissue and no mutation detected from the plasma sample. In contrast, the remaining one patient had mutations detected in the plasma but not the tissue sample. Figure 1A illustrates the genomic profile of the cohort, which covers the common 168 genes of the 2 gene panels used. Among the total of 2,444 mutation events detected from the panel, 1,096 (44.8%) were concordant between tissue and plasma samples (Figure 1B). In addition, 1,097 mutation events were only detected in tissue samples and 251 were only detected in plasma samples. For point mutations, a total of 981 mutation events (54.7%) were concordant between tissue and plasma samples. For CNVs, a total of 115 mutation events (17.6%) were concordant between tissue and plasma samples.
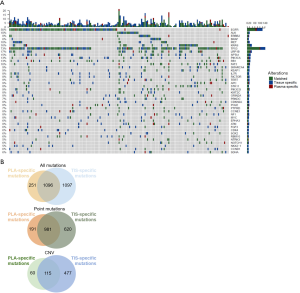
Distribution of the cohort according to concordance classification
We further stratified the cohort based on the concordance classification described in the Methods section. Among the 185 patients included in the cohort, 148 (80%) patients had concordant tissue and plasma results for the targetable mutations in 8 genes (class 1). A total of 120 (65%) patients had concordant tissue and plasma results for the targetable mutations in 8 genes plus any mutations in STK11 and selected mutations in KRAS and TP53 (class 2). Finally, 70 (69%) patients had concordant tissue and plasma results for 11 genes plus concordance of TMB high or low using 10 mutations/Mb as the cutoff (class 3).
Predictive value of serum tumor marker levels on the concordance rates of mutation profiles between paired tissue and plasma samples
To evaluate the feasibility of using serum tumor markers to predict the concordance of genomic profiles from paired tissue and plasma samples, we first identified which of the serum tumor markers were correlated with the concordance classification of our cohort. For the initial analysis, we designated the upper value of the clinical reference range as the cutoff to delineate the various serum tumor markers as high and low. We then analyzed the concordance distribution of the cohort according to this cutoff, which are listed in Table 2.
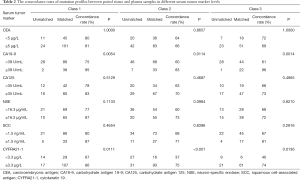
Full table
Among the tumor markers, higher serum levels of CA19-9 (≥39 U/mL) and CYFRA21-1 (≥3.3 µg/L) showed significant correlation with the higher concordance in class 1 (CA19-9, 95% vs. 75%, P=0.005; CYFRA21-1; 86% vs. 67%, P=0.011), class 2 (CA19-9, 83% vs. 60%, P=0.011; CYFRA21-1 75% vs. 37%, P<0.001), and class 3 (CA19-9, 96% vs. 61%; P=0.001; CYFRA21-1, 74% vs. 40%, P=0.014). Serum levels of CEA, CA125, NSE, and SCC were not correlated with any classification of concordance between tissue and blood mutation profiles (Table 2, Figure 2).
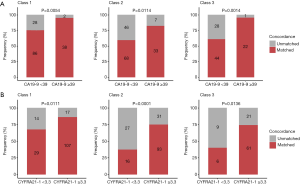
Next, we explored the optimal cutoffs for CA19-9 and CYFRA21-1 that predicted tissue and plasma mutation profile concordance. We explored the serum concentration of each tumor marker that achieved the designated predictive threshold of 90%, 95%, and 100% concordance in mutation profiles, as summarized in Table 3.
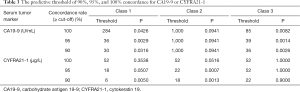
Full table
In class 1, the cutoff value for CA19-9 was 30 U/mL to reach a concordance rate of 90% (P=0.032), 36 U/mL to reach 95% (P=0.003), and 284 U/mL to reach 100% (P=0.043). Additionally, the cutoff value for CYFRA21-1 was 6 µg/L to reach a concordance rate of 90% (P=0.005), 18 µg/L to reach 95% (P=0.051), and 52 µg/L to reach 100% (P=0.354). In class 2, no suitable cutoff was predictive for CA19-9. Further, the cutoff value for CYFRA21-1 was 18 µg/L to reach a concordance rate of 90% (P=0.001), 22 µg/L to reach 95% (P=0.001), and 52 µg/L to reach 100% (P=0.052). In class 3, the cutoff value for CA19-9 was 36 U/mL to reach a concordance rate of 90% (P=0.003), 39 U/mL to reach 95% (P=0.001), and 85 U/mL to reach 100% (P=0.008). Additionally, the cutoff value for CYFRA21-1 was 22 µg/L to reach a concordance rate of 90% (P=0.900), 52 µg/L to reach 95% (P>0.99), and 52 µg/L to reach 100% (P>0.99; Figure 3, Table 3).
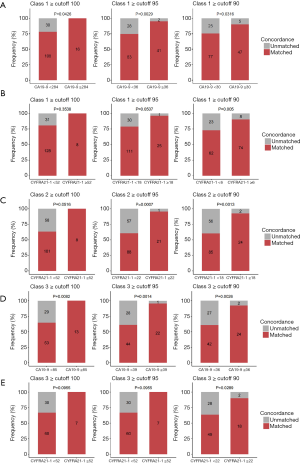
Taken together, these data indicate the positive correlation between serum levels of CA19-9 and CYFRA21-1 and the concordance of mutation profiles between tissue and plasma samples in genes included in class 1, class 2, and class 3. Furthermore, these data suggest the feasibility of using certain cutoff values for CA19-9 and CYFRA21-1 levels in predicting at least a 90% concordance rate in a certain subset of clinically relevant gene mutations between paired tissue and plasma samples.
Exploring the serum tumor marker scoring system for predicting concordance of mutation profiles
To comprehensively analyze the predictive value of serum tumor markers, we developed a scoring system by combining the clinical values of 4 commonly assayed serum tumor biomarkers including CEA, CA19-9, CYFRA21-1, and CA125. These were first normalized with the upper values of the clinical reference range, the relative fold change was then calculated accordingly for each serum tumor marker, and finally all fold changes for the 4 tumor markers were summed to gain the sum score.
The sum score was significantly higher in patients with concordant tissue and plasma mutation profiles than in those with unmatched profiles for class 1 (P=0.014), class 2 (P=0.007), and class 3 (P=0.003; Figure 4A). As seen in Table 4, a sum score cutoff of >27 achieved a ≥95% mutation profile concordance in class 1 genes (P=0.002), while a sum score cutoff of >35 achieved a ≥90% concordance in class 2 genes (P=0.001). A sum score cutoff of >26 and 27 achieved a ≥90% and 95% concordance in class 3 genes (P=0.001, P<0.001), respectively. With a sum score cutoff of 35, the predictive threshold of 90% for mutation profile concordance was achieved for class 1, class 2, and class 3 genes (Figure 4B, Table 4).
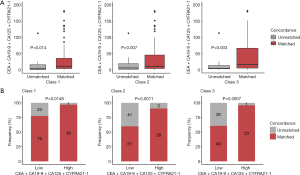
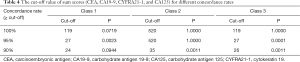
Full table
We further constructed a logistic regression model to calculate a GCS, which quantifies the relationship between the sum score for the combined serum tumor markers including, CA19-9, CA125, CYFRA21-1, and CEA and the concordance of mutation profiles between paired plasma and tissue biopsies. An ROC curve was constructed based on the predictive likelihood, and an optimal cutoff value was identified by maximizing the Youden Index. The optimal cutoff values were used to stratify the patients into 2 GCS groups. Consistent with the sum score, the use of a GCS cutoff of 0.811 achieved the predictive threshold of 90% mutation profile concordance for class 1 (P=0.005), class 2 (P<0.001), and class 3 (P<0.001; Figure 5).
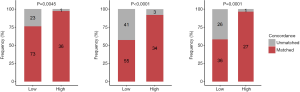
Taken together, these data indicate the feasibility of the combined analysis of 4 commonly used serum tumor markers as predictors of the concordance in mutation profiles for a subset of clinically relevant genes between paired plasma and tissue samples of patients with advanced lung adenocarcinoma.
Correlation between ctDNA content and serum tumor markers
The most common method for estimating the proportion of ctDNA is multiplication of the cfDNA yield of the plasma sample and the maxAF, which is defined as the highest allele fraction detected from the sample (22,33). In our cohort, the plasma maxAF was significantly higher among patients with concordant mutation profiles in class 1 (P<0.001), class 2 (P<0.001), and class 3 (P<0.001) genes between tissue and plasma samples than in those with unmatched mutation profiles (Figure 6). We further explored the correlation between plasma maxAF and the concentration of serum tumor markers. However, this relationship was nonlinear (R2 =0.031; P=0.043; Figure S3).
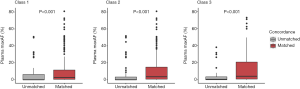
Discussion
Compared with tissue biopsy, liquid biopsy is minimally invasive or noninvasive. In addition, the capability of liquid biopsy, particularly plasma, to reflect the somatic mutations in real-time during the course of treatment can influence and raise its importance in the therapeutic management of advanced stage lung cancer patients (34). However, how to predict the concordance of liquid biopsy and tissue biopsy is still unknown. Hence, there is an urgent need to identify clinically relevant markers that enable prediction of the concordance between tissue biopsy and plasma samples.
Among the 185 advanced lung adenocarcinoma patients in our study, only 80% of patients had the same sequencing results in tissue and blood in 8 targetable genes. The consistency rate decreased to 65% when KRAS, Tp53, and STK11 were taken into consideration. When we took TMB into consideration together, the consistency rate was 69%.
In this study, we first analyzed the predictive value of serum tumor markers based on the clinically used cutoff, which identified the positive correlation between serum levels of CA19-9 and CYFRA21-1 and the concordance rate of mutation profiles between paired tissue and plasma samples. Since most cancer patients have elevated serum tumor markers that are much higher than the upper reference range, we further explored various cutoffs for CA19-9 and CYFRA21-1 could predict 90% to 100% concordance rates. Next, we explored the use of a scoring system that considers the values for 4 commonly used serum tumor markers in clinical practice. We found that a score above 35 enabled prediction of more than 90% concordance between paired tissue and plasma samples in our definition of class 1, 2, and 3. Finally, we used a logistic regression model to identify a reasonable value to predict concordance.
Based on our results, higher serum levels of CA19-9 and CYFRA21-1 were predictive of the higher concordance in treatment-related genes between paired tissue and plasma samples. CYFRA21-1 or cytokeratin 19 fragment is a tumor marker for NSCLC, especially for squamous-cell carcinoma (SCC) (29,30). A previous study observed that higher serum CYFRA21-1 levels were predictive of shorter progression-free survival for EGFR tyrosine kinase inhibitor treatment in advanced NSCLC (31). Our study further expanded the clinical application of this tumor marker.
Previous studies indicated that the proportion of ctDNA present in a plasma sample is related to the concordance of mutation profiles between tissue and blood (16) A study in prostate cancer demonstrated the detection of all somatic mutations from matched metastatic tissue biopsy and ctDNA samples when the ctDNA proportion was greater than 2% of total cfDNA (35). In our study, plasma maxAF was significantly higher among patients with concordant mutation profiles. Our research also hypothesized that serum tumor markers partly reflected the condition of ctDNA. However, the correlation between plasma maxAF and the concentration of serum tumor markers was nonlinear. Furthermore, based on the economic and time costs of NGS, ctDNA is not a good clinical judgment index for the concordance of liquid and tissue biopsy. We could conclude that serum tumor markers are a better predictor than the proportion of ctDNA in total cfDNA due to its universal application, lower cost, shorter testing time, and better accuracy. In clinical practice, these markers can serve as screening markers for prioritizing patients who may benefit from liquid biopsy. It is recommended that patients with serum tumor marker levels greater than the threshold can have plasma samples sequenced first, which have a high likelihood of comprehensively reflecting the mutation profile of tumor samples. In the reverse scenario, tissue biopsy should be preferred. However, further investigations are warranted to validate and optimize the predictive performance of these markers.
Our study has several limitations. As this study was conducted in various cancer centers, the number of tested serum tumor markers was not uniform. Hence, values of some tumor markers were unavailable for some patients. In clinical practice, the levels of serum tumor markers are not always elevated in all of the patients with advanced lung cancer. Therefore, the conclusions from this study may not be applicable to all patients with advanced stage lung cancer. In addition, all of the samples analyzed in this study, including serum for the tumor biomarker assays and paired plasma and tissue samples for sequencing, were collected at initial diagnosis before any systemic treatment. The predictive value of these tumor markers for the concordance rates during the treatment course or at progression were not investigated and should be explored. Using these serum markers to screen patients who are suitable for liquid biopsy may still miss some patients with concordant profiles who may have avoided the invasive tissue biopsy. Moreover, this study only focused on the concordance between plasma and tissue samples from patients with lung adenocarcinoma. In the future, we can further expand the sample size and stratify the patients according to different pathological types to confirm and expand our findings.
In conclusion, our study provides evidence that high serum levels of tumor markers, particularly of CA19-9 and CYFRA21-1, can be used as easy and practical clinical predictors of concordance in mutation profiles between blood and tissue samples from patients with advanced lung adenocarcinoma.
Acknowledgments
We would like to thank LetPub (
Funding: This work was funded by Chinese the National Natural Science Program (81702249, 2017), the Project of Ministry of Science and Technology of China (2017YFC1309202), Shanghai Distinguished Young Medical Talents Training Program (QNYXRC01), the Medical Innovation Research Project of Shanghai Science and Technology Commission (20Y11914400), the Military Program of Logistics support department of PLA (19BJZ03), and the Military Program of Changzheng Hospital (2019CZJS208-1).
Footnote
Reporting Checklist: The authors have completed the REMARK reporting checklist. Available at https://dx.doi.org/10.21037/tlcr-21-543
Data Sharing Statement: Available at https://dx.doi.org/10.21037/tlcr-21-543
Conflicts of Interest: All authors have completed the ICMJE uniform disclosure form (available at https://dx.doi.org/10.21037/tlcr-21-543). Dr. XDJ reported funding support from the Logistics Support Department of PLA (19BJZ03) and Changzheng Hospital (2019CZJS208-1). Dr. YSZ reported funding support from the Project of Ministry of Science and Technology of China (2017YFC1309202), the Shanghai Distinguished Young Medical Talents Training Program (QNYXRC01) and the Medical Innovation Research Project of Shanghai Science and Technology Commission (20Y11914400). Dr. NI reported that he had got grants from Chugai Pharmaceutical Co., Ltd., Boehringer Ingelheim, TAIHO Pharmaceutical Co., Ltd.; and received fee from Eli Lilly Japan K.K, MSD K.K. Dr. BDQ reported funding support from Chinese National Natural Science Funding (grant number 81702249, 2017). The funder has no role in the study design, data collection and analysis, decision to publish, or preparation of the manuscript. LS, HZW, YC, and JJZ reported that they are employees of Burning Rock Biotech. The other authors have no conflicts of interest to declare.
Ethical Statement: The authors are accountable for all aspects of the work in ensuring that questions related to the accuracy or integrity of any part of the work are appropriately investigated and resolved. This study was performed in accordance with the Declaration of Helsinki (as revised in 2013). This study was approved by the Ethics Committee of the Changzheng Hospital (approval number: 2017SL016). Written informed consent was obtained from all patients included in the study.
Open Access Statement: This is an Open Access article distributed in accordance with the Creative Commons Attribution-NonCommercial-NoDerivs 4.0 International License (CC BY-NC-ND 4.0), which permits the non-commercial replication and distribution of the article with the strict proviso that no changes or edits are made and the original work is properly cited (including links to both the formal publication through the relevant DOI and the license). See: https://creativecommons.org/licenses/by-nc-nd/4.0/.
References
- Garraway LA, Jänne PA. Circumventing cancer drug resistance in the era of personalized medicine. Cancer Discov 2012;2:214-26. [Crossref] [PubMed]
- Chalfin HJ, Glavaris SA, Gorin MA, et al. Circulating Tumor Cell and Circulating Tumor DNA Assays Reveal Complementary Information for Patients with Metastatic Urothelial Cancer. Eur Urol Oncol 2021;4:310-4. [Crossref] [PubMed]
- Strickler JH, Loree JM, Ahronian LG, et al. Genomic Landscape of Cell-Free DNA in Patients with Colorectal Cancer. Cancer Discov 2018;8:164-73. [Crossref] [PubMed]
- Oxnard GR, Thress KS, Alden RS, et al. Association Between Plasma Genotyping and Outcomes of Treatment With Osimertinib (AZD9291) in Advanced Non-Small-Cell Lung Cancer. J Clin Oncol 2016;34:3375-82. [Crossref] [PubMed]
- Schrock AB, Pavlick D, Klempner SJ, et al. Hybrid Capture-Based Genomic Profiling of Circulating Tumor DNA from Patients with Advanced Cancers of the Gastrointestinal Tract or Anus. Clin Cancer Res 2018;24:1881-90. [Crossref] [PubMed]
- Zill OA, Greene C, Sebisanovic D, et al. Cell-Free DNA Next-Generation Sequencing in Pancreatobiliary Carcinomas. Cancer Discov 2015;5:1040-8. [Crossref] [PubMed]
- Chan KCA, Woo JKS, King A, et al. Analysis of Plasma Epstein-Barr Virus DNA to Screen for Nasopharyngeal Cancer. N Engl J Med 2017;377:513-22. [Crossref] [PubMed]
- Wen L, Li J, Guo H, et al. Genome-scale detection of hypermethylated CpG islands in circulating cell-free DNA of hepatocellular carcinoma patients. Cell Res 2015;25:1376. [Crossref] [PubMed]
- Fernandez-Cuesta L, Perdomo S, Avogbe PH, et al. Identification of Circulating Tumor DNA for the Early Detection of Small-cell Lung Cancer. EBioMedicine 2016;10:117-23. [Crossref] [PubMed]
- Genovese G, Kähler AK, Handsaker RE, et al. Clonal hematopoiesis and blood-cancer risk inferred from blood DNA sequence. N Engl J Med 2014;371:2477-87. [Crossref] [PubMed]
- Müller JN, Falk M, Talwar J, et al. Concordance between Comprehensive Cancer Genome Profiling in Plasma and Tumor Specimens. J Thorac Oncol 2017;12:1503-11. [Crossref] [PubMed]
- Corcoran RB, Chabner BA. Application of Cell-free DNA Analysis to Cancer Treatment. N Engl J Med 2018;379:1754-65. [Crossref] [PubMed]
- Kuderer NM, Burton KA, Blau S, et al. Comparison of 2 Commercially Available Next-Generation Sequencing Platforms in Oncology. JAMA Oncol 2017;3:996-8. [Crossref] [PubMed]
- Chae YK, Davis AA, Jain S, et al. Concordance of Genomic Alterations by Next-Generation Sequencing in Tumor Tissue versus Circulating Tumor DNA in Breast Cancer. Mol Cancer Ther 2017;16:1412-20. [Crossref] [PubMed]
- Chae YK, Davis AA, Carneiro BA, et al. Concordance between genomic alterations assessed by next-generation sequencing in tumor tissue or circulating cell-free DNA. Oncotarget 2016;7:65364-73. [Crossref] [PubMed]
- Adalsteinsson VA, Ha G, Freeman SS, et al. Scalable whole-exome sequencing of cell-free DNA reveals high concordance with metastatic tumors. Nat Commun 2017;8:1324. [Crossref] [PubMed]
- Schrock AB, Welsh A, Chung JH, et al. Hybrid Capture-Based Genomic Profiling of Circulating Tumor DNA from Patients with Advanced Non-Small Cell Lung Cancer. J Thorac Oncol 2019;14:255-64. [Crossref] [PubMed]
- Seijo LM, Peled N, Ajona D, et al. Biomarkers in Lung Cancer Screening: Achievements, Promises, and Challenges. J Thorac Oncol 2019;14:343-57. [Crossref] [PubMed]
- Ludwig JA, Weinstein JN. Biomarkers in cancer staging, prognosis and treatment selection. Nat Rev Cancer 2005;5:845-56. [Crossref] [PubMed]
- Molina R, Marrades RM, Augé JM, et al. Assessment of a Combined Panel of Six Serum Tumor Markers for Lung Cancer. Am J Respir Crit Care Med 2016;193:427-37. [Crossref] [PubMed]
- Abbas M, Kassim SA, Habib M, et al. Clinical Evaluation of Serum Tumor Markers in Patients With Advanced-Stage Non-Small Cell Lung Cancer Treated With Palliative Chemotherapy in China. Front Oncol 2020;10:800. [Crossref] [PubMed]
- Mao X, Zhang Z, Zheng X, et al. Capture-Based Targeted Ultradeep Sequencing in Paired Tissue and Plasma Samples Demonstrates Differential Subclonal ctDNA-Releasing Capability in Advanced Lung Cancer. J Thorac Oncol 2017;12:663-72. [Crossref] [PubMed]
- Li H, Durbin R. Fast and accurate short read alignment with Burrows-Wheeler transform. Bioinformatics 2009;25:1754-60. [Crossref] [PubMed]
- McKenna A, Hanna M, Banks E, et al. The Genome Analysis Toolkit: a MapReduce framework for analyzing next-generation DNA sequencing data. Genome Res 2010;20:1297-303. [Crossref] [PubMed]
- Koboldt DC, Zhang Q, Larson DE, et al. VarScan 2: somatic mutation and copy number alteration discovery in cancer by exome sequencing. Genome Res 2012;22:568-76. [Crossref] [PubMed]
- Wang K, Li M, Hakonarson H. ANNOVAR: functional annotation of genetic variants from high-throughput sequencing data. Nucleic Acids Res 2010;38:e164 [Crossref] [PubMed]
- Cingolani P, Platts A. A program for annotating and predicting the effects of single nucleotide polymorphisms, SnpEff: SNPs in the genome of Drosophila melanogaster strain w1118; iso-2; iso-3. Fly (Austin) 2012;6:80-92. [Crossref] [PubMed]
- Newman AM, Bratman SV, Stehr H, et al. FACTERA: a practical method for the discovery of genomic rearrangements at breakpoint resolution. Bioinformatics 2014;30:3390-3. [Crossref] [PubMed]
- Stieber P, Hasholzner U, Bodenmüller H, et al. CYFRA 21-1. A new marker in lung cancer. Cancer 1993;72:707-13. [Crossref] [PubMed]
- Lequaglie C, Marino P, Maioli C, et al. Cyfra 21-1 - a new tumor-marker for lung-cancer. Oncol Rep 1995;2:119-22. [Crossref] [PubMed]
- Tanaka K, Hata A, Kaji R, et al. Cytokeratin 19 fragment predicts the efficacy of epidermal growth factor receptor-tyrosine kinase inhibitor in non-small-cell lung cancer harboring EGFR mutation. J Thorac Oncol 2013;8:892-8. [Crossref] [PubMed]
- Jiao XD, Qin BD, You P, et al. The prognostic value of TP53 and its correlation with EGFR mutation in advanced non-small cell lung cancer, an analysis based on cBioPortal data base. Lung Cancer 2018;123:70-5. [Crossref] [PubMed]
- Zhou C, Yuan Z, Ma W, et al. Clinical utility of tumor genomic profiling in patients with high plasma circulating tumor DNA burden or metabolically active tumors. J Hematol Oncol 2018;11:129. [Crossref] [PubMed]
- Rijavec E, Coco S, Genova C, et al. Liquid Biopsy in Non-Small Cell Lung Cancer: Highlights and Challenges. Cancers (Basel) 2019;12:17. [Crossref] [PubMed]
- Wyatt AW, Annala M, Aggarwal R, et al. Concordance of Circulating Tumor DNA and Matched Metastatic Tissue Biopsy in Prostate Cancer. J Natl Cancer Inst 2017; [Crossref] [PubMed]