A comparison of the microbiome composition in lower respiratory tract at different sites in early lung cancer patients
Highlight box
Key Findings
• The current study revealed that, compared to other lower respiratory tract tissues, lung tumors exhibited a higher abundance of specific bacterial species, while the alpha diversity of these tissues demonstrated an inverse correlation with body mass index (BMI).
What is known and what is new?
• Previous studies have suggested an association between lung microbiome dysbiosis and lung carcinogenesis, primarily through comparing microbiome differences between cancerous and non-cancerous individuals.
• In this study, we examined differences in microbiome composition in four different sites in lower respiratory tract of lung cancer patients. Although the difference in microbiome diversity among different tissues was insignificant, lung tumors were enriched with particular bacterial species. Additionally, we observed an inverse relationship between BMI and alpha diversity.
What is the implication, and what should change now?
• The enriched bacterial species in lung tumors may contribute to tumorigenesis. The inverse relationship between BMI and alpha diversity provides a novel clue for deciphering the mechanisms of lung cancer.
Introduction
Many parts of the human body, including the gastrointestinal tract, skin, mouth, lungs, and reproductive tract, are colonized by diverse microbial communities (microbiome) whose importance to human health is increasingly recognized. Many studies have revealed that specific patterns of human microbiome are correlated with various diseases, including autoimmune diseases, diabetes, obesity, and even mental illness (1,2). The association between cancer and microbes has also attracted broad interest in recent years (3,4). The International Association for Cancer Registries (IACR) has nominated 11 out of the approximately 1,012 types of globally known organisms as human carcinogens or “tumor microbes” (5). The epidemiology, molecular mechanisms, and clinical implications of these tumor microorganisms, estimated to cause 2.2 million tumor patients each year (approximately 13% of cancer cases worldwide), have been extensively studied.
Lung cancer is the most common cancer diagnosed worldwide and is the leading cause of mortality among all cancer types. Despite significant advances in targeted drugs and immunotherapy in recent years, the prognosis of lung cancer patients remains poor. Epidemiological studies have shown that repeated use of antibiotics increases the risk of lung cancer (6). However, the relationship between the microbiome in lower respiratory tract and lung cancer development remains to be elucidated.
Many studies have shown an association between specific bacteria to colorectal carcinogenesis. Fusobacterium nucleatum, a bacterium commonly present in the guts of patients with inflammatory bowel disease, has also been identified as a risk factor for colon cancer (7). Bacteroides fragilis has been reported to initiate colon cancer by secreting endotoxins that damage DNA (8). With the development of high-throughput sequencing of 16S rRNA and metagenomics, which enable us to inspect the microbiome at higher resolution and broader spectrum, the healthy lung, including the lower airways, has been recognized as a place for bacterial symbiosis (9). The microbiome characteristics of lung tissue are distinct from those of the lower gastrointestinal tract (10). The microbiome colonized in the host lung would be altered in different respiratory diseases, which have been described in both human and model systems (11,12).
The direct influence of lung microbiome dysbiosis on lung cancer occurrence has also been hotly investigated (13). A Study has documented the existence of Staphylococcus spp., Bacillus spp., Haemophilus influenza, and Candida albicans in lung cancer patients (14). The microbial composition of the lower respiratory tract of lung cancer patients is significantly different from that of the non-cancer control group, which has been shown to be related to the upregulation of the ERK and PI3K signaling pathways (15). According to Lee et al., two phyla (Firmictutes and TM7) and two genera (Veillonella and Megasphaera) are abundant in bronchoalveolar lavage fluid (BALF) in lung cancer patients (16). Two bacterial biomarkers, Capnocytophaga and Veillonella, have shown good efficacy in predicting squamous cell carcinoma (SCC) and adenocarcinoma (AC), which can facilitate lung cancer screening (17). In the KP (Kras-LSL-G12D; P53-flox/flox) lung cancer model, Veillonella parvula has been shown to mediate lower respiratory tract microbiome imbalance, reduce survival rate, increase tumor load, and cause activation of interleukin (IL)-17, PI3K, MAPK, and ERK signaling pathways and inhibitory checkpoint markers (18). By single-cell RNA sequencing, a recent study revealed that microbiome is highly concentrated in immune and epithelial cells that promote cancer progression, with transcriptional changes in pathways involved in inflammation, metastasis, and DNA repair (19). These signaling pathways are critical in the development and progression of cancer, thus indicating potential mechanisms of the microbiome cancer occurrence.
The present study compared the microbiome in cancer tissues, matched para-cancerous tissues, matched normal tissues, and matched bronchial tissues (BT) from early lung cancer patients. We included the para-cancerous tissues because they may exhibit a transitional state between cancer and normal tissues (20), including in the microbial community (21), potentially reflecting the underlying processes of carcinogenesis. The results herein may provide novel insights for understanding the role of the microbiome in lung cancer development. We present this article in accordance with the MDAR reporting checklist (available at https://tlcr.amegroups.com/article/view/10.21037/tlcr-23-231/rc).
Methods
Patients and sample collection
In this study, 16 patients with non-small cell lung cancer (NSCLC) were recruited at the Cancer Hospital Chinese Academy of Medical Science between July 2021 and July 2022. Due to difficulties in clinical sampling, the final sample size of this study was restricted to 16 patients. The selection criteria were as follows: (I) patients should not have taken antibiotics for three months before the surgery; and (II) patients had no prior exposure to chemotherapy, radiation therapy, nor any other treatments for lung cancer prior to the surgery. Lung tissues were obtained from four sites, including lung tumor tissues (TT), matched para-tumor tissues (PT), matched distal normal lung tissue (DN), and matched BT, in accordance with aseptic procedures to minimize oral and environmental contamination. The fresh tissues at each sampling site were immediately flash-frozen in liquid nitrogen and subsequently stored at −80 ℃. The clinical data of all participants, including gender, age, body mass index (BMI), smoking history, pathology type, and tumor staging, were collated.
The study was conducted in accordance with the Declaration of Helsinki (as revised in 2013). The study was approved by the ethics committee of the National Cancer Center/Cancer Hospital, Chinese Academy of Medical Science and Peking Union Medical College, Beijing, China (approval No. 21/404-3075). All patients provided consent before participation.
Genome DNA extraction and 16S rRNA gene amplicon sequencing
Total genomic DNA was isolated using the TianGen kit following the instructions of the manufacturer. The concentration and purity of the DNA were evaluated using Qubit® dsDNA Assay Kit in Qubit® 3.0 Fluorometer (Life Technologies, CA, USA) and NanoPhotometer® spectrophotometer (IMPLEN, CA, USA) with 1.5% agarose gels. To amplify the V3–V4 regions of the 16S rRNA, the isolated DNA of tissues was diluted to a concentration of 1 ng/µL using sterile water. Specific primers containing the barcodes and Phusion® High-Fidelity PCR Master Mix (New England Biolabs) were used for amplification of the V3–V4 regions. PCR products were then subjected to electrophoresis on 2% agarose gels, and samples displaying distinct bands between 400–450 bp were selected. These selected samples were purified using the Qiagen Gel Extraction Kit (Qiagen, Germany). According to the manufacturer’s suggestions, sequencing libraries were constructed using the NEBNext® UltraTM II DNA Library Prep Kit (Illumina, USA), and an 8-bp index was incorporated. The quality of the library was evaluated on the Qubit® 3.0 Fluorometer and Agilent Bioanalyzer 2100 system. Finally, the libraries were sequenced on an Illumina NovaSeq6000 platform, generating 250 bp paired-end reads. To avoid any potential bias effects, the technicians who conducted these experiments were blinded to the sample information. Due to the limited accessibility of the samples, we performed amplicon sequencing on each specimen only once and did not perform technical nor biological replicates.
Data analysis
Sequence reads were demultiplexed, and then the paired-end sequences were merged using FLASH software. Joined sequence reads were aligned to the 16S rRNA sequence database [Silva database db128 (20)] and clustered into Operational Taxonomic Units (OTU) with a sequence similarity threshold of 97%. The taxonomic analysis was performed using the RDP Classifier algorithm using QIIME2 (21) pipeline software (2019.7). Alpha diversity metrics, including Chao1, Shannon, Simpson, and Richness index, were calculated. Beta diversity was measured by principal coordinate analysis (PCoA) and nonmetric multidimensional scaling (NMDS), based on Bray-Curtis, weighted, and unweighted UniFrac distance. Homogeneity of molecular variance (HOMOVA) and analysis of molecular variance (AMOVA) were utilized to access the diversity differences between groups. Significantly enriched microbiome was determined through linear discriminant analysis (LDA) using LEfSe software with an LDA score threshold of 2. Phylogenetic Investigation of Communities by Reconstruction of Unobserved States (PICRUSt2 Version 2.2.0-b) software (22) was applied to predict the potential functions of the microbial community. PICRUSt works as follows: (I) the 16S sequence in the OTU table is normalized by the 16S copy number of the corresponding species, (II) the normalized sequence abundance is multiplied by the sequence annotation number table of each metabolic pathway to obtain the predicted abundance of the metabolic pathway.
Statistical analysis
All statistical analyses were performed using R software (Version 4.2.2). Differential analysis among multiple groups was compared using the Kruskal-Wallis rank sum test, and the P value was adjusted using the Benjamini-Hochberg method. The relationships between microbiome and clinical characteristics were evaluated using the Spearman correlation test or Chi-squared test. Statistical significance was determined by a two-sided P value of less than 0.05.
Results
Characteristics of the study participants
A total of 63 samples, including the lung TT, matched PT, matched distal normal lung tissues (DN), and matched BT, were collected from 16 patients. The BT from one patient was unavailable due to the surgical procedure. Table 1 describes the characteristics of the study population. The median age of the participants was 57.5 years, with females (87.5%) making up the majority. Most of the participants were non-smokers (93.8%). Four patients (25%) were diagnosed with minimally invasive adenocarcinoma (MIA); 1 patient (6.3%) was diagnosed with lung metastasis of colorectal cancer; and all other patients (68.8%) were diagnosed with lung adenocarcinoma in situ (AIS). A total of 14 patients (87.5%) were diagnosed with stages IA and IB, and the other 2 (12.5%) patients had more advanced cancer stages (IIB, IIIA).
Table 1
Variables | Patients with lung cancer (N=16) |
---|---|
Age (years, median) | 57.5 [32–65]a |
Gender | |
Male | 2 (12.5)b |
Female | 14 (87.5) |
BMI (kg/m2, median) | 23.57 [18.31–27.34] |
Smoking status | |
Non-smoker | 15 (93.8) |
Smoker | 1 (6.2) |
Histology | |
LUAD (AIS) | 11 (68.8) |
MIA | 4 (25.0) |
mCRC_LU | 1 (6.3) |
Tumor stage | |
I | 14 (87.5) |
II | 1 (6.3) |
III | 1 (6.3) |
a, for continuous variables, the numbers in parentheses are the minimum and maximum values; b, for counting variables, the number in each parenthesis presents the percentage of the corresponding category. BMI, body mass index; LUAD, lung adenocarcinoma; AIS, adenocarcinoma in situ; MIA, minimally invasive adenocarcinoma; mCRC_LU, lung metastasis of colorectal cancer.
The correlation between clinical characteristics and alpha diversity in different lung tissues of lung cancer patients
The correlation between the clinical characteristics and the alpha diversity of the samples at each site was examined. The Wilcox rank-rum test was performed to compare categorical variables, and the Spearman correlation test was used for continuous variables. The results showed an inverse correlation between BMI and alpha diversity (as indicated by Chao1, ACE, Shannon, and Simpson) in TT (Figure 1A), PT (Figure 1B), DN (Figure 1C), and BT (Figure 1D). This relationship was significant in TT tissues, as indicated by the Shannon index (R2=0.24, P=0.030), and in BT tissues, as shown by the Simpson index (R2=0.38, P=0.009) and the Shannon index (R2=0.39, P=0.008). No significant correlation was found between age and alpha diversity, and no significant difference in alpha diversity was observed between LUAD (AIS) and MIA. However, due to the small sample sizes in certain categories (such as male, smoker, mCRC_LU, tumor stage II, and tumor stage III), comparing the alpha diversity of these groups is not statistically meaningful.
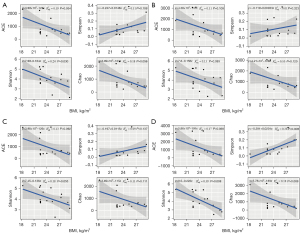
Alpha and beta diversity among different lung tissues of lung cancer patients
The richness and evenness of the microbiome were generally consistent among the TT, PT, DN, and BT groups in the lung cancer patients as estimated by Chao1 (K-W, P=0.415), richness (K-W, P=0.445), Shannon (K-W, P=0.981), and Simpson (K-W, P=0.887). The differences among TT, PT, DN, and BT were not statistically significant, as shown by the HOMOVA (P=0.778) and AMOVA algorithms (P=0.991) (Figure 2A). In addition, PCoA and NMDS based on Bray-Curtis, weighted and unweighted UniFrac distance, showed no distinct separation trend among the four groups (Figure 2B, Figure S1).
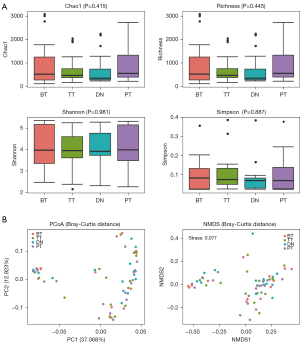
Taxonomic profiles of the microbiome composition
Classification and analyses were performed on the phylum and genus levels of the most abundant microbiome in the four lung tissue sites. The phylum taxonomic profile showed that Proteobacteria, Firmicutes, Bacteroidota, and Desulfobacterota were the most common among the four tissue groups. BT tissues showed the highest abundance of Firmicutes and the lowest abundance of Proteobacteria, while TT tissues showed the highest abundance of Proteobacteria and lowest abundance of Firmicutes (Figure 3A). At the genus level, Pseudomonas, Desulfovibrio, Limosilactobacillus, and Lactobacillus were the core genera in the study samples (Figure 3B). LDA indicated that Qipengyuania, Barnesiellaceae, and Bdellovibrio were enriched in the BT tissues; Rubellimicrobium and Fictibacillus were higher in the TT tissues; Lysobacter and Acidobacteriae were increased in the DN tissues; and Delftia, Aureimonas, and Peptoniphilus were abundant in the PT tissues (Figure 3C).
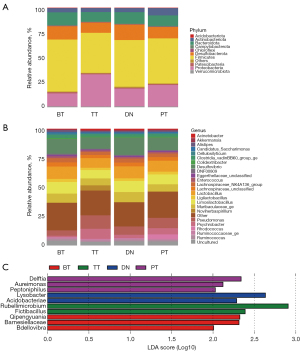
Predicted functional profiles of the microbiome
The functional analysis evaluated by PICRUSt indicated that biosynthesis of ansamycins and synthesis and degradation of ketone bodies were abundant among samples. The Kruskal-Wallis rank sum test was applied to identify the significantly imbalanced Kyoto Encyclopedia of Genes and Genomes (KEGG) metabolic pathways among TT, PT, DN, and BT tissues in lung cancer patients. There were no specifically discrepant pathways among the four tissue groups. No imbalanced microbiome was found in the three groups compared to TT tissues (Figure 4).
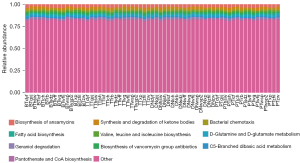
Discussion
This study investigated the microbiome composition of lung tissues from different sites of lung cancer patients and analyzed their associations with clinical characteristics. From the taxonomic profiles, we demonstrated that specific species at both the phylum and genus levels were enriched at particular lung sites of lung cancer patients. There was an inverse relationship between BMI and alpha diversity. However, there were no differences in the alpha diversity among different tissues, and no distinct biological pathways were found.
Our findings were consistent with previous studies documenting Firmicutes, Proteobacteria, and Bacteroidetes as the most common microbiotic phyla in the lungs (23). The results herein revealed that cancer tissues had a higher abundance of Proteobacteria and a lower abundance of Firmicutes, which is consistent with previous studies (24,25). A large study that collected lung tissues from 143 lung cancer patients and 33 controls showed that the abundance of Proteobacteria is higher and that of Firmicutes is lower in cancer tissues compared with non-cancer control lung tissues (24). When compared to the lung tissues of lung emphysema patients, lung tumors showed enrichment of Proteobacteria and a decreased richness of Firmicutes (25). Moreover, the literature has also reported a link between Proteobacteria and a poorer response to immunotherapy and a lower programmed death-1 (PD-1) expression in lung cancer patients (26).
Previous studies have documented an association between lung cancer and several bacterial genera, including Granulicatella, Abiotrophia, Streptococcus, Veillonella, Megasphaera, and Selenomonas (16,27-29). Differences in microbiome diversity between lung tumors and matched non-malignant specimens have also been reported; however, these results were inconsistent between different studies (24,30,31). Specific microbiome compositions have also been related to cancer recurrence and patient survival (30,32). The present study found an enrichment of Rubellimicrobium and Fictibacillus in cancerous lung tissues. While Rubellimicrobium has been previously documented to increase in lung cancer tissue (33), a lower level of Fictibacillus in esophageal TT as compared to nontumor tissues has been reported (34). Thus, the link between these two bacterial species and cancer development requires further investigation.
Many studies have demonstrated an association between low alpha diversity of the gut microbiome and increased BMI (35-37), although inconsistent results have also been reported (38). To our knowledge, the present study is the first to report a relationship between BMI and the alpha diversity of the lung microbiome. The underlying mechanism for the negative correlation between BMI and microbiome diversity remains unknown, while high BMI and low microbiome diversity are both risk factors for lung cancer (39,40). Besides BMI, microbiome diversity has also been associated with cancer stages, tobacco smoking, and other air pollutants, all of which are risk factors for lung cancer (14).
Lower microbiome diversity has been identified as a risk factor for respiratory disease (41), including lung cancer (29). Another study compared the difference in alpha diversity in lung tumor and non-malignant lung tissue samples and found a lower alpha diversity in tumor samples (14). In contrast, our study did not observe any difference in diversity among different sites of lung cancer patients. This non-significant result might be due to the limited number of samples recruited in this study.
Our analysis using PICURSt has identified several functional pathways that the bacterial population may be involved in. While the top 10 most enriched pathways have been identified, the proportion of these pathways was found to be very small. The top pathway was related to the biosynthesis of ansamycin, which belongs to the higher pathway class of “Metabolism of terpenoids and polyketides”. Previous studies have shown that patients with NSCLC have a lower level of biosynthesis of ansamycin (42), and tumor microbiomes have reduced activity in the metabolism of terpenoids and polyketides compared to normal tissues (43). However, in the current study, the differences between groups were not found to be significant. Therefore, further validation of the role of biosynthesis of ansamycin in larger sample sets is warranted to gain a better understanding of its potential role in lung cancer.
Previous studies have shown that microbiomes play specific roles under different conditions (44-46). In the case of the healthy lung, it has been confirmed that diverse communities of bacteria play an important role in maintaining an immune-tolerant environment and preserving lung homeostasis (44). However, exposure to certain taxa such as Streptococcus and Veillonella has been shown to initiate lung cancer development by inducing the ERK/PI3K pathway in airway epithelial cells, which is recognized as an early event in lung carcinogenesis (45). Additionally, an animal study suggested that the microbiome isolated from advanced mouse lung tumors could promote tumorigenesis (46). Certain microbial characteristics have been associated with poor prognosis in lung cancer patients. For example, a higher diversity, lower abundance of Koribacteraceae, and greater abundance of Bacteroidaceae, Lachnospiraceae, and Ruminococcaceae were associated with reduced RFS and DFS (30). In late-stage lung cancer patients (IIIB, IV), the genus Thermus was found to be higher, while Legionella was more abundant in metastatic patients (14). In the current study, we only recruited early-stage lung cancer patients because surgery is the primary treatment for these patients, making the tissues of different sites more accessible for investigation. The results of this study could facilitate early detection and provide new clues in deciphering the mechanism of carcinogenesis.
The present study has strengths and limitations. We collected matched lung tissues from four different sites of lung cancer patients, which facilitated the direct examination of the tumor microbial environment and compared them between malignant and non-malignant tissues. However, the number of patients recruited in this study was limited, which might be the reason for the insignificant difference in microbiome diversity and function between different tissue sites.
Conclusions
Although the microbiome diversity among different tissues showed no significant difference, we found that specific lung sites in lung cancer patients were enriched with particular species, which might contribute to tumorigenesis. Moreover, we detected an inverse relationship between BMI and alpha diversity among these tissues, providing a novel clue for deciphering the mechanisms of lung carcinogenesis.
Acknowledgments
Funding: This work was supported by the National Key R&D Program of China (No. 2020AAA0109500), National Natural Science Foundation of China (No. 82122053), the Beijing Municipal Science & Technology Commission (No. Z191100006619118), R&D Program of Beijing Municipal Education Commission (No. KJZD20191002302), CAMS Initiative for Innovative Medicine (Nos. 2021-1-I2M-012, and 2021-1-I2M-015), Key-Area Research and Development Program of Guangdong Province (No. 2021B0101420005), and Shenzhen Science and Technology Program (Nos. RCJC20221008092811025, and ZDSYS20220606101604009).
Footnote
Reporting Checklist: The authors have completed the MDAR reporting checklist. Available at https://tlcr.amegroups.com/article/view/10.21037/tlcr-23-231/rc
Data Sharing Statement: Available at https://tlcr.amegroups.com/article/view/10.21037/tlcr-23-231/dss
Peer Review File: Available at https://tlcr.amegroups.com/article/view/10.21037/tlcr-23-231/prf
Conflicts of Interest: All authors have completed the ICMJE uniform disclosure form (available at https://tlcr.amegroups.com/article/view/10.21037/tlcr-23-231/coif). All authors report that this work was supported by the National Key R&D Program of China (No. 2020AAA0109500), National Natural Science Foundation of China (No. 82122053), the Beijing Municipal Science & Technology Commission (No. Z191100006619118), R&D Program of Beijing Municipal Education Commission (No. KJZD20191002302), CAMS Initiative for Innovative Medicine (Nos. 2021-1-I2M-012, and 2021-1-I2M-015), Key-Area Research and Development Program of Guangdong Province (No. 2021B0101420005), and Shenzhen Science and Technology Program (Nos. RCJC20221008092811025, and ZDSYS20220606101604009). The authors have no other conflicts of interest to declare.
Ethical Statement: The authors are accountable for all aspects of the work in ensuring that questions related to the accuracy or integrity of any part of the work are appropriately investigated and resolved. The study was conducted in accordance with the Declaration of Helsinki (as revised in 2013). This study was approved by the ethics committee of National Cancer Center/Cancer Hospital, Chinese Academy of Medical Science and Peking Union Medical College, Beijing, China (approval No. 21/404-3075). All patients provided consent before participation.
Open Access Statement: This is an Open Access article distributed in accordance with the Creative Commons Attribution-NonCommercial-NoDerivs 4.0 International License (CC BY-NC-ND 4.0), which permits the non-commercial replication and distribution of the article with the strict proviso that no changes or edits are made and the original work is properly cited (including links to both the formal publication through the relevant DOI and the license). See: https://creativecommons.org/licenses/by-nc-nd/4.0/.
References
- Qin J, Li Y, Cai Z, et al. A metagenome-wide association study of gut microbiota in type 2 diabetes. Nature 2012;490:55-60. [Crossref] [PubMed]
- Foster JA, McVey Neufeld KA. Gut-brain axis: how the microbiome influences anxiety and depression. Trends Neurosci 2013;36:305-12. [Crossref] [PubMed]
- Chen C, Cai Y, Liu Y, et al. Pan-Cancer Analysis of Microbiome Quantitative Trait Loci. Cancer Res 2022;82:3449-56. [Crossref] [PubMed]
- Georgiou K, Marinov B, Farooqi AA, et al. Gut Microbiota in Lung Cancer: Where Do We Stand? Int J Mol Sci 2021;22:10429. [Crossref] [PubMed]
- Biological agents. IARC Monogr Eval Carcinog Risks Hum 2012;100:1-441. [PubMed]
- Boursi B, Mamtani R, Haynes K, et al. Recurrent antibiotic exposure may promote cancer formation--Another step in understanding the role of the human microbiota? Eur J Cancer 2015;51:2655-64. [Crossref] [PubMed]
- Kostic AD, Chun E, Robertson L, et al. Fusobacterium nucleatum potentiates intestinal tumorigenesis and modulates the tumor-immune microenvironment. Cell Host Microbe 2013;14:207-15. [Crossref] [PubMed]
- Sears CL, Geis AL, Housseau F. Bacteroides fragilis subverts mucosal biology: from symbiont to colon carcinogenesis. J Clin Invest 2014;124:4166-72. [Crossref] [PubMed]
- Goto T. Microbiota and lung cancer. Semin Cancer Biol 2022;86:1-10. [Crossref] [PubMed]
- Dickson RP, Huffnagle GB. The Lung Microbiome: New Principles for Respiratory Bacteriology in Health and Disease. PLoS Pathog 2015;11:e1004923. [Crossref] [PubMed]
- Erb-Downward JR, Thompson DL, Han MK, et al. Analysis of the lung microbiome in the "healthy" smoker and in COPD. PLoS One 2011;6:e16384. [Crossref] [PubMed]
- Twomey KB, Alston M, An SQ, et al. Microbiota and metabolite profiling reveal specific alterations in bacterial community structure and environment in the cystic fibrosis airway during exacerbation. PLoS One 2013;8:e82432. [Crossref] [PubMed]
- Xu N, Wang L, Li C, et al. Microbiota dysbiosis in lung cancer: evidence of association and potential mechanisms. Transl Lung Cancer Res 2020;9:1554-68. [Crossref] [PubMed]
- Yu G, Gail MH, Consonni D, et al. Characterizing human lung tissue microbiota and its relationship to epidemiological and clinical features. Genome Biol 2016;17:163. [Crossref] [PubMed]
- Yan X, Yang M, Liu J, et al. Discovery and validation of potential bacterial biomarkers for lung cancer. Am J Cancer Res 2015;5:3111-22. [PubMed]
- Lee SH, Sung JY, Yong D, et al. Characterization of microbiome in bronchoalveolar lavage fluid of patients with lung cancer comparing with benign mass like lesions. Lung Cancer 2016;102:89-95. [Crossref] [PubMed]
- Maddi A, Sabharwal A, Violante T, et al. The microbiome and lung cancer. J Thorac Dis 2019;11:280-91. [Crossref] [PubMed]
- Tsay JJ, Wu BG, Sulaiman I, et al. Lower Airway Dysbiosis Affects Lung Cancer Progression. Cancer Discov 2021;11:293-307. [Crossref] [PubMed]
- Galeano Niño JL, Wu H, LaCourse KD, et al. Effect of the intratumoral microbiota on spatial and cellular heterogeneity in cancer. Nature 2022;611:810-7. [Crossref] [PubMed]
- Quast C, Pruesse E, Yilmaz P, et al. The SILVA ribosomal RNA gene database project: improved data processing and web-based tools. Nucleic Acids Res 2013;41:D590-6. [Crossref] [PubMed]
- Bolyen E, Rideout JR, Dillon MR, et al. Reproducible, interactive, scalable and extensible microbiome data science using QIIME 2. Nat Biotechnol 2019;37:852-7. [Crossref] [PubMed]
- Douglas GM, Maffei VJ, Zaneveld JR, et al. PICRUSt2 for prediction of metagenome functions. Nat Biotechnol 2020;38:685-8. [Crossref] [PubMed]
- Lemon KP, Klepac-Ceraj V, Schiffer HK, et al. Comparative analyses of the bacterial microbiota of the human nostril and oropharynx. mBio 2010;1:e00129-10. [Crossref] [PubMed]
- Greathouse KL, White JR, Vargas AJ, et al. Interaction between the microbiome and TP53 in human lung cancer. Genome Biol 2018;19:123. [Crossref] [PubMed]
- Liu Y, O'Brien JL, Ajami NJ, et al. Lung tissue microbial profile in lung cancer is distinct from emphysema. Am J Cancer Res 2018;8:1775-87. [PubMed]
- Jang HJ, Choi JY, Kim K, et al. Relationship of the lung microbiome with PD-L1 expression and immunotherapy response in lung cancer. Respir Res 2021;22:322. [Crossref] [PubMed]
- Cameron SJS, Lewis KE, Huws SA, et al. A pilot study using metagenomic sequencing of the sputum microbiome suggests potential bacterial biomarkers for lung cancer. PLoS One 2017;12:e0177062. [Crossref] [PubMed]
- Hosgood HD 3rd, Sapkota AR, Rothman N, et al. The potential role of lung microbiota in lung cancer attributed to household coal burning exposures. Environ Mol Mutagen 2014;55:643-51. [Crossref] [PubMed]
- Vogtmann E, Hua X, Yu G, et al. The Oral Microbiome and Lung Cancer Risk: An Analysis of 3 Prospective Cohort Studies. J Natl Cancer Inst 2022;114:1501-10. [Crossref] [PubMed]
- Peters BA, Hayes RB, Goparaju C, et al. The Microbiome in Lung Cancer Tissue and Recurrence-Free Survival. Cancer Epidemiol Biomarkers Prev 2019;28:731-40. [Crossref] [PubMed]
- Liu HX, Tao LL, Zhang J, et al. Difference of lower airway microbiome in bilateral protected specimen brush between lung cancer patients with unilateral lobar masses and control subjects. Int J Cancer 2018;142:769-78. [Crossref] [PubMed]
- Patnaik SK, Cortes EG, Kannisto ED, et al. Lower airway bacterial microbiome may influence recurrence after resection of early-stage non-small cell lung cancer. J Thorac Cardiovasc Surg 2021;161:419-429.e16. [Crossref] [PubMed]
- Chen Y, Huang Y, Ding X, et al. A Multi-Omics Study of Familial Lung Cancer: Microbiome and Host Gene Expression Patterns. Front Immunol 2022;13:827953. [Crossref] [PubMed]
- Zhang B, Xiao Q, Chen H, et al. Comparison of tumor-associated and nontumor-associated esophageal mucosa microbiota in patients with esophageal squamous cell carcinoma. Medicine (Baltimore) 2022;101:e30483. [Crossref] [PubMed]
- Turnbaugh PJ, Hamady M, Yatsunenko T, et al. A core gut microbiome in obese and lean twins. Nature 2009;457:480-4. [Crossref] [PubMed]
- Osborne G, Wu F, Yang L, et al. The association between gut microbiome and anthropometric measurements in Bangladesh. Gut Microbes 2020;11:63-76. [Crossref] [PubMed]
- Flores R, Shi J, Gail MH, et al. Assessment of the human faecal microbiota: II. Reproducibility and associations of 16S rRNA pyrosequences. Eur J Clin Invest 2012;42:855-63. [Crossref] [PubMed]
- Sze MA, Schloss PD. Erratum for Sze and Schloss, "Looking for a Signal in the Noise: Revisiting Obesity and the Microbiome". mBio 2017;8:e01995-17. [Crossref] [PubMed]
- Hosgood HD, Cai Q, Hua X, et al. Variation in oral microbiome is associated with future risk of lung cancer among never-smokers. Thorax 2021;76:256-63. [Crossref] [PubMed]
- Vithayathil M, Carter P, Kar S, et al. Body size and composition and risk of site-specific cancers in the UK Biobank and large international consortia: A mendelian randomisation study. PLoS Med 2021;18:e1003706. [Crossref] [PubMed]
- Einarsson GG, Comer DM, McIlreavey L, et al. Community dynamics and the lower airway microbiota in stable chronic obstructive pulmonary disease, smokers and healthy non-smokers. Thorax 2016;71:795-803. [Crossref] [PubMed]
- Wei YF, Huang MS, Huang CH, et al. Impact of Gut Dysbiosis on the Risk of Non-Small-Cell Lung Cancer. Int J Environ Res Public Health 2022;19:15991. [Crossref] [PubMed]
- Liu B, Zhou Z, Jin Y, et al. Hepatic stellate cell activation and senescence induced by intrahepatic microbiota disturbances drive progression of liver cirrhosis toward hepatocellular carcinoma. J Immunother Cancer 2022;10:e003069. [Crossref] [PubMed]
- Sommariva M, Le Noci V, Bianchi F, et al. The lung microbiota: role in maintaining pulmonary immune homeostasis and its implications in cancer development and therapy. Cell Mol Life Sci 2020;77:2739-49. [Crossref] [PubMed]
- Tsay JJ, Wu BG, Badri MH, et al. Airway Microbiota Is Associated with Upregulation of the PI3K Pathway in Lung Cancer. Am J Respir Crit Care Med 2018;198:1188-98. [Crossref] [PubMed]
- Jin C, Lagoudas GK, Zhao C, et al. Commensal Microbiota Promote Lung Cancer Development via gammadelta T Cells. Cell 2019;176:998-1013.e16. [Crossref] [PubMed]
(English Language Editor: J. Teoh)