Clinical validity of oncogenic driver genes detected from circulating tumor DNA in the blood of lung cancer patients
Highlight box
Key findings
• Circulating tumor DNA (ctDNA) efficiently represents the tumor mutational profile and may provide additional information for lung cancer treatment.
What is known and what is new?
• ctDNA has been extensively studied in various types of cancers for clinical use.
• ctDNA can be a helpful alternative to tumor tissue and may be a reliable prognostic biomarker in treating lung cancer patients.
What is the implication, and what should change now?
• ctDNA is able to be widely applied in diagnosing and treating lung cancer by improving ctDNA analysis.
Introduction
Over the past few decades, the prevalence of lung cancer has dramatically increased, and lung cancer has become the leading cause of cancer-related mortality worldwide (1). Most lung cancer patients are diagnosed at an advanced stage, making lung cancer the most common cause of cancer-related deaths worldwide (2,3). The recent development of next-generation sequencing (NGS) technology has enabled the identification of the landscape of genomic mutations in tumors and has prolonged the survival of patients by facilitating molecular targeted therapy based on the tumor mutational profile (4). Various targeted agents have been developed based on understanding the genomic alterations in tumors, and targeted drugs have shown dramatic responses in many patients. However, acquiring tumor tissue via tumor biopsy from lung cancer is difficult, with high risk of complications and being unfeasible in several cases. Instead, liquid biopsy for lung cancer patients is considered an alternative method in clinical settings due to its accessibility (5). Liquid biopsy is a minimally invasive method for detecting and identifying the molecular properties of lung cancer with high sensitivity, facilitating integrative analyses of genomic, transcriptomic, or metabolomic markers. However, the nontrivial false-positive rates for tumor mutation detection, prohibitive costs, and reproducibility issues associated with the procedure are still needed to be resolved (6).
Because an increased amount of circulating cell-free DNA (cfDNA) is frequently observed in cancer patients compared with that in healthy individuals, cfDNA is one of the most investigated liquid biopsy-derived biomarkers (7). In addition, circulating tumor DNA (ctDNA) contained in cfDNA sufficiently represents the tumor genomic characteristics that ctDNA analysis has been widely applied in various clinical settings (8). The advantages of ctDNA analysis, such as the representation of tumor heterogeneity and dynamics, drug response monitoring for patients with progressive disease (PD), and the ability to detect genomic markers by minimally invasive procedure, have been reported in several studies (9,10). Although comprehensive ctDNA analysis has consistently demonstrated the viability of replacing tissue biopsy tests, several limitations or considerations exist. For example, ctDNA concentration is lower than genomic DNA concentration, which strongly correlates with tumor size and staging, and occasionally increases in patients with benign or premalignant tumors (11). Therefore, the clinical impact of ctDNA-based testing should be extensively investigated to avoid misleading treatment decisions for cancer patients. Here, we evaluated the clinical implementation of ctDNA in patients diagnosed with advanced non-small cell lung cancer (NSCLC) and investigated its potential clinical utility (prognosis prediction and molecular tumor diagnosis) using the NGS platform. We present this article in accordance with the REMARK reporting checklist (available at https://tlcr.amegroups.com/article/view/10.21037/tlcr-22-912/rc).
Methods
Patient enrollment
Patients over 20 years of age diagnosed with NSCLC at Seoul National University Bundang Hospital (SNUBH) were enrolled in a prospective study of ctDNA analysis during cancer treatment. The study enrolled patients in two separate cohorts (A and B). Cohort A included patients newly diagnosed with metastatic or recurrent NSCLC and scheduled to receive first-line systemic chemotherapy (to evaluate pre-treatment status at baseline). Patients in Cohort B had metastatic or recurrent NSCLC, had been receiving molecular targeted therapy or immune checkpoint inhibitors, and had once experienced tumor shrinkage and regrowth during treatment (to evaluate post-treatment status after disease progression). Patients with a history of cancer in other organs in the past three years or whose tumors had mixed small cell components were excluded from the study.
The study was conducted in accordance with the Declaration of Helsinki (as revised in 2013). The study was approved by the Institutional Review Board (IRB) of SNUBH (No. B-1703-387-301) and informed consent was obtained from all individual participants. The detailed study design and information are available from ClinicalTrials.gov (identifier: NCT03235765).
Blood sample collection and cfDNA extraction
All enrolled patients underwent routine blood draws before each clinical visit, and blood samples for the study were obtained along with blood tests for the clinical visit under informed consent. For Cohort A patients, three blood samples were obtained at starting treatment (baseline), before the second cycle of chemotherapy, and after confirming disease progression via imaging tumor evaluation. In Cohort B, blood samples were routinely collected after study enrollment following the treatment response evaluation schedule. And in the end, blood samples were collected when tumor progression was confirmed for Cohort B patients. Although this study was initially designed to analyze serial cfDNA samples from both Cohorts A and B, due to time and cost limitations, subsequent procedures were limited to analyzing only blood samples at baseline in Cohort A and those of PD in Cohort B.
Approximately 10 mL of whole blood was obtained from patients for the study. Each blood sample was collected in Roche Cell-Free DNA Collection Tubes® (Roche Diagnostics, Basel Switzerland) and centrifuged at 4 ℃ and 1,000 × g for 10 min within 2 h of blood collection. Next, 5 mL of plasma was separated from the buffy coat, and the isolated plasma was preserved at −70 to 80 ℃. cfDNA was extracted from plasma using a QIAamp Circulating Nucleic Acid Kit (Qiagen, Valencia, CA, USA), and the concentration and the total amount for eluted cfDNA were measured using a Qubit dsDNA HS Assay Kit, Qubit 2.0 fluorometer (Life Technologies, Carlsbad, CA, USA), and 2100 Bioanalyzer with High-Sensitivity DNA Chips (Agilent Technologies, Santa Clara, CA, USA), according to the manufacturer’s instructions.
Tumor tissue for genotyping and targeted gene sequencing
Driver mutations of lung cancer that could be eligible molecular targeted agents were tested from the tumor formalin-fixed paraffin-embedded (FFPE) tissue at diagnosis for clinical use. EGFR exon 19 deletion and exon 21 mutation (EGFR L858R) were confirmed by PANA Mutyper tests (PANAGENE, Daejeon, Republic of Korea), and ALK fusions were detected by applying VENTANA ALK (D5F3) CDx immunohistochemistry (IHC) and/or a break-apart fluorescence in situ hybridization assay. Expression of programmed death ligand-1 (PD-L1) was evaluated by IHC staining using one of the following antibodies and detection systems: mouse monoclonal primary anti-PD-L1 antibody, 22C3 pharmDx (prediluted, clone 22C3, Dako, Carpinteria, CA, USA) with an Autostainer Link 48 and an EnVision DAB Detection System (Agilent Technologies); rabbit monoclonal primary anti-PD-L1 antibody, Ventana SP263 (prediluted, Ventana Medical Systems, Tucson, AZ, USA) with a BenchMark XT staining system and BenchMark ULTRA with OptiView Universal DAB Detection Kit (Ventana Medical Systems).
Tumor tissues for target gene panel sequencing were prepared from the FFPE slides. Next, FFPE DNA was extracted using a QIAamp DNA FFPE Tissue Kit (Qiagen) according to the manufacturer’s instructions. Initial QC checks of FFPE DNA were performed using electrophoresis on 1% agarose gels and a Qubit dsDNA HS Assay Kit with a Qubit 2.0 fluorometer (Life Technologies), according to the manufacturer’s instructions.
Targeted gene panel sequencing and variant calling
Targeted sequencing on both ctDNA and tumor tissue DNA was performed using Axen Cancer Panel 2 (Macrogen Inc., Seoul, Korea), targeting approximately 1.1 Mb of the genomic region containing 171 genes and 25 fusion gene rearrangements (Table S1); the average sequencing depth for ctDNA was approximately 4,000×. Raw DNA sequencing reads were aligned to the human reference genome (hg19) using Burrow-Wheeler Aligner (12), and somatic variants were called from aligned reads using GATK v4.1.2 (13) and MuTect2 (14) based on genomic information from whole-genome sequencing resources of 1,950 Koreans (15).
Somatic variants were annotated using ANNOVAR (16) based on the RefSeq gene with gnomAD v3.1 (17), the Northeast Asian Reference Database (NARD) (15), the Single Nucleotide Polymorphism Database (NCBI dbSNP build 150), NCBI ClinVar and the Catalogue of Somatic Mutations in Cancer (COSMIC) (18). To perform the NGS analysis, variants were included based on the following criteria: exonic variants with an alternate allele frequency of less than 1% reported in gnomAD v3.1 and NARD, and damaging and deleterious variants according to Polymorphism Phenotyping (19) and the Sorting Intolerant From Tolerant database (20), respectively. Further filtration was performed for variants below the likelihood threshold and base quality using MuTect2 and benign variants from the ClinVar database. Variants with a sequencing depth <200 and variant allele frequency (VAF) <5% were further filtered for tissue samples, and the cut-off of depth and read number of the containing variant alleles were set at 50 and ten, respectively, for ctDNA samples because of the potential small fraction of variant alleles within ctDNA sequencing data from plasma. Then, the tumor mutation burden (TMB) was calculated as the total number of variants. Lung cancer-related variants were identified using the COSMIC database.
Statistical analyses
Statistical analyses were performed using R version 4.1 (R Foundation for Statistical Computing, Vienna, Austria). The Mann-Whitney U-test was used to compare cfDNA concentration and basic clinical characteristics, and the chi-squared test was used to investigate the association between TP53 mutations and smoking. Pearson’s correlation coefficients were calculated to examine the associations between the variables. Overall survival (OS) was defined as the time from study enrollment to death for Cohort A and from confirmed PD of studied treatment to death for Cohort B. Survival graphs were estimated using the Kaplan-Meier method, and log-rank tests were used for comparison. The sensitivity and precision for ctDNA analysis compared with those of tissue sequencing data were calculated using true positives as overlap variants between tissue and ctDNA, false negatives as tissue-specific variants, and false positives as ctDNA-specific variants.
Results
Patient characteristics
From March 2017 to January 2019, a total of 152 patients (Cohort A: 73 and Cohort B: 79) were enrolled in the study, and data from 129 patients (Cohort A: 65 and Cohort B: 64) with matched tumor samples and clinical data were analyzed. The median age of Cohort A patients was 62 years (range, 35–82 years) at diagnosis, and that of Cohort B was 66 years (range, 25–84 years) at study enrollment. Of the patients, 53.5% were male, and 49.6% were ex- or current smokers. In the pathological review, most patients (82.2%) were diagnosed with adenocarcinomas, and a few squamous carcinomas and sarcomatoid tumors were involved. In addition, 55 (42.6%) patients were confirmed to have EGFR variants by site-specific polymerase chain reaction (PCR)-based sequencing of tumor tissue, and eight patients had ALK fusion detected by IHC staining. Patients confirmed to have EGFR variants or ALK fusion underwent molecular targeted therapies. A total of 27.9% of patients had high PD-L1 expression (>50%) on the cell surface of tumor tissue cells and were candidates for treatment with immune checkpoint inhibitors, including nivolumab and pembrolizumab. The patient characteristics are summarized in Table 1. Meanwhile, univariate analysis using clinical variables showed that although a variety of patients were enrolled in Cohorts A and B, none of the patient characteristics significantly affected survival (Table S2).
Table 1
Categories | Cohort A (n=65) | Cohort B (n=64) | Total (n=129) |
---|---|---|---|
Age, median [range] | 62 [35–82] (at diagnosis) | 66 [25–84] (at enrollment) | |
Sex, n (%) | |||
Male | 43 (66.2) | 26 (40.6) | 69 (53.5) |
Female | 22 (33.8) | 38 (59.4) | 60 (46.5) |
Smoking, n (%) | |||
Non-smoker | 20 (30.8) | 40 (62.5) | 60 (46.5) |
Ex- or current smoker | 42 (64.6) | 22 (34.4) | 64 (49.6) |
Not available | 3 (4.6) | 2 (3.1) | 5 (3.9) |
Pathology, n (%) | |||
Adenocarcinoma | 49 (75.4) | 57 (89.1) | 106 (82.2) |
Squamous carcinoma | 6 (9.2) | 2 (3.1) | 8 (6.2) |
Sarcomatoid carcinoma | 4 (6.2) | 0 (0.0) | 4 (3.1) |
Others† | 6 (9.2) | 5 (7.8) | 11 (8.5) |
PD-L1, n (%) | |||
<1% | 20 (30.8) | 20 (31.3) | 40 (31.0) |
1–50% | 22 (33.8) | 17 (26.6) | 39 (30.2) |
>50% | 22 (33.8) | 14 (21.9) | 36 (27.9) |
Not available | 1 (1.5) | 13 (20.3) | 14 (10.9) |
EGFR, n (%) | |||
Wild type | 48 (73.8) | 16 (25.0) | 64 (49.6) |
Exon 19 deletion | 5 (7.7) | 24 (37.5) | 29 (22.5) |
L858R | 1 (1.5) | 20 (31.3) | 21 (16.3) |
Other variants | 3 (4.6) | 2 (3.1) | 5 (3.9) |
Not available | 8 (12.3) | 2 (3.1) | 10 (7.8) |
ALK, n (%) | |||
Wild type | 55 (84.6) | 50 (78.1) | 105 (81.4) |
Fusion | 1 (1.5) | 7 (10.9) | 8 (6.2) |
Not available | 9 (13.8) | 7 (10.9) | 16 (12.4) |
MTT, n (%) | |||
Gefitinib | 2 (3.1) | 15 (23.4) | 17 (13.2) |
Erlotinib | 4 (6.2) | 21 (32.8) | 25 (19.4) |
Afatinib | 1 (1.5) | 2 (3.1) | 3 (2.3) |
Osimertinib | 0 (0.0) | 2 (3.1) | 2 (1.6) |
Crizotinib/ceritinib | 1 (1.5) | 6 (9.4) | 7 (5.4) |
Treatment without MTT‡ | 57 (87.7) | 18 (28.1) | 75 (58.1) |
Others†, NSCLC with neuroendocrine differentiation, pleomorphic carcinoma, or NSCLC-not otherwise specified. Treatment without MTT‡, cytotoxic chemotherapies (gemcitabine, pemetrexed, or paclitaxel + cisplatin or carboplatin) or immunotherapies (pembrolizumab, atezolizumab, or nivolumab, etc.). Cohort A, newly diagnosed patients; Cohort B, patients treated with targeted therapy. PD-L1, programmed death ligand-1; EGFR, epidermal growth factor receptor; ALK, anaplastic lymphoma kinase; MTT, molecular targeted therapy; NSCLC, non-small cell lung cancer.
Poor survival rates and improved sensitivity rates to mutations detected from tissue in patients with high cfDNA concentration at diagnosis
Many studies have shed light on the strong associations between the amount of cfDNA and the characteristics of cancer patients, highlighting its potential as a robust prognostic factor (21,22). In this study, the amount of cfDNA was evaluated as a biomarker for the survival rates of cancer patients. Most patients had cfDNA concentration below 1 ng/µL (0.226, 0.370 and 0.603 ng/µL; Cohort A and 0.330, 0.455 and 0.714; Cohort B for 25%, 50% and 75% of samples, respectively). And the minimum and maximum cfDNA concentrations were 0.010 and 4.680 ng/µL for Cohort A and 0.188 and 2.580 ng/µL for Cohort B, respectively (Figure S1). First, patients were stratified into cfDNA-high and -low groups based on the median cfDNA concentration value (Cohort A: 0.370 ng/µL, Cohort B: 0.455 ng/µL) and conducted a survival analysis between these two groups. In Cohort A, patients with higher cfDNA concentrations had significantly poorer OS than those with low cfDNA levels (median 22.6 months vs. not reached, P=0.04; Figure 1A), while no significant difference between the cfDNA-high and -low groups was observed in Cohort B (median not reached vs. 33.1 months, P=0.3; Figure 1A). The cfDNA concentrations between groups of sex, smoking status, and expression of PD-L1, EGFR, and ALK genotyping results were compared; however, none of the differences were significant (Figure S2).
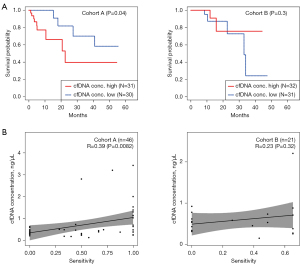
Among the recruited patients, ctDNA sequencing data were available for 78 patients (50 in Cohort A and 28 in Cohort B), and 70 patients (46 in Cohort A and 24 in Cohort B) had matched tumor tissue NGS data. Of the 78 ctDNA-sequenced patients, 60 (76.9%) had lung cancer-associated variants based on the COSMIC database, which is the largest catalog of somatic mutations across most cancer types (18). The ctDNA and tumor tissue NGS data were extensively compared by calculating the average sensitivity and precision of ctDNA compared with the results derived from tumor tissue samples. The average sensitivity rate and precision of ctDNA sequencing data from patients in Cohort A were 58.4% and 61.5%, respectively (Table 2); the rates for Cohort B were relatively low (48.4% and 43.7%, respectively; Table 2). To test whether higher amounts of cfDNA increase concordance with tissue sequencing, the correlation between cfDNA concentration and sensitivity rates was investigated. Within patients of both Cohorts A and B, the cfDNA concentration positively correlated with the sensitivity rates of ctDNA compared with those of tumor tissue sequencing data (R=0.39, P=0.0082; R=0.23, P=0.32, respectively; Figure 1B).
Table 2
Type | Total | |
---|---|---|
Sensitivity (recall) | Precision | |
Cohort A | 58.4% | 61.5% |
Cohort B | 48.4% | 43.7% |
Total | 55.1% | 55.5% |
Sensitivity (recall) = TP/(TP + FN); Precision = TP/(TP + FP). TP: overlap variants between tissue and ctDNA; FP: ctDNA-specific variants; FN: tissue-specific variants. ctDNA, circulating tumor DNA; TP, true positive; FP, false positive; FN, false negative.
ctDNA mutations of cancer-related genes and correlation between ctDNA TP53 mutation and smoking status
TP53, KRAS, STK11, EGFR, NF1, ATM, APC, EPHA3, CDKN2A, and ERBB4 are frequently mutated in lung adenocarcinoma. From tissue sequencing data, TP53 was also the most frequently mutated gene (37%), and EGFR (25%), KRAS (15%), APC (8%), and CDKN2A (7%) were included in the top ten most frequently mutated genes across Cohorts A and B (Figure S3). The mutation profiles of the 78 patients were also investigated using ctDNA sequencing. The most frequently mutated gene was TP53 (37%), followed by EGFR (25%), DNMT3A (15%), KRAS (8%), and APC (7%), indicating that renowned genes related to lung cancer were also present in the top list based on tumor tissue data (Figure 2A). In Cohort A, patients were divided into TP53 positive and negative groups (Figure 2A). The strong correlation between TP53 mutations and smoking status has been well investigated (23). Consistently, in Cohort A, a significantly higher abundance of smokers among patients with TP53 mutations at diagnosis was observed in both the tumor tissue and ctDNA sequencing data (P=0.005 and P=0.037, respectively; Figure 2A and Figure S3).
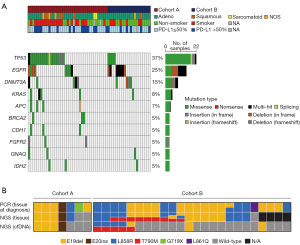
Comparison between PD-L1 status and TMB from tumor sequencing
Immunotherapy targeting PD-1 or PD-L1 has emerged as a highly efficient treatment strategy for lung cancer. In clinics, the IHC of PD-L1 protein is used for decision-making, and patients with ≥50% stained tumor cells are highly sensitive to PD-1/PD-L1 inhibitors. In addition, several studies have shown that TP53, KRAS, and EGFR mutation statuses can be used as predictive factors for anti-PD-1/PD-L1 (24-26). However, although the associations between PD-L1 IHC results and TP53, KRAS, and EGFR mutation status in tumor tissue and ctDNA sequencing for Cohorts A and B were tested, none were significant (Cohort A, tissue: P=0.77, 0.73 and 1.0; Cohort A, cfDNA: P=0.89, 0.82 and 1.0; Cohort B, tissue: P=1.0, 0.12, and 0.53; Cohort B, cfDNA: P=1.0, 1.0 and 1.0 for TP53, EGFR and KRAS, respectively).
The TMB of each patient was also calculated in both cohorts. Positive correlations between calculated TMB derived from tissue and ctDNA sequencing data in Cohorts A and B were observed (R=0.38 and P=0.02, and R=0.40 and P=0.11 for Cohorts A and B, respectively; Figure S4). TMB is well characterized for guiding PD-L1/PD-1 targeted therapy responses; therefore, the TMB in PD-L1-high and -low groups were compared using IHC results. At diagnosis, there was no significant difference in TMB between PD-L1-high and -low expression in Cohort A for both tumor tissue and ctDNA (P=0.99 and P=0.53, respectively; Figure S5). In addition, patients with PD in Cohort B showed similar TMB between PD-L1-high and -low expression for tumor tissue and ctDNA (P=0.78 and P=0.50, respectively; Figure S5). The two cohorts were stratified into TMB-low and -high groups using the median TMB value (2.0 mutations/Mb for ctDNA and tissue of Cohort B and 2.5 mutations/Mb for the tissue of Cohort A), and none showed significant differences in OS (Cohort A: P=0.50 and P=0.40, and Cohort B: P=0.20 and P=0.80 for tissue and ctDNA, respectively; Figure S6).
Potential of ctDNA-based NGS as a disease monitoring approach at disease progression
To further evaluate ctDNA-based panel sequencing, the results of the EGFR genotyping test using PCR-based Sanger sequencing were compared. In Cohort A, seven patients were EGFR positive, accounting for 14% of Cohort A. Mutations in EGFR were consistently found in five and four out of seven patients in tumor tissue and ctDNA NGS panel sequencing, respectively (Figure 2B). In Cohort B, most patients had EGFR mutations because patients receiving EGFR-targeted therapy were enrolled. Patients in Cohort B showed PD after targeted treatment; therefore, EGFR resistance mutations were prevalent in both tumor tissue and ctDNA sequencing results. At diagnosis, 24 patients in Cohort B tested positive in the EGFR routine PCR-based genotyping test. Among the EGFR-positive patients, a total of 20 patients had available tissue samples for PD, and EGFR driver mutations were found in 95.0% (nineteen out of 20 samples) of them, while 47.4% (nine out of nineteen samples) had the EGFR T790M mutation, which is known to be associated with tyrosine kinase inhibitor relapse. Among the patients with EGFR mutations in PD from tissue sequencing data, 31.6% (six out of nineteen samples) had consistent EGFR mutations in ctDNA sequencing data, and interestingly, EGFR T790M mutations found in ctDNA in two patients were not detected in tumor tissue (Figure 2B). These two patients were clinically reported to have PD, and the identified EGFR T790M variants had high confidence, with VAFs of 1.79% and 5.55%, respectively (Table 3). Based on the EGFR T790M resistance mutation in ctDNA, these two patients could change their treatment regimen to osimertinib, for which tumors with the EGFR T790M mutation are sensitive. And one of the two patients actually treated with osimertinib and had a response with decreasing tumor size. In addition, a ctDNA-specific variant of the KRAS G12C mutation was observed in one patient, which might overcome treatment resistance by using targeted therapies such as sotorasib. Also, additional ctDNA-specific variants were detected in TP53 that are related to lung cancer according to the COSMIC database (Table 3).
Table 3
Gene | Chr | Position (hg19) | Ref | Alt | Depth | VAF (%) | Function | Pathogenicity (ClinVar) | Amino acid change | rsID (dbSNP) | Sample |
---|---|---|---|---|---|---|---|---|---|---|---|
EGFR | 7 | 55249071 | C | T | 1007 | 1.79 | Missense | Pathogenic | T790M | rs121434569 | B-001 |
EGFR | 7 | 55249071 | C | T | 937 | 5.55 | Missense | Pathogenic | T790M | rs121434569 | B-075 |
KRAS | 12 | 25398285 | C | A | 225 | 32.0 | Missense | Pathogenic | G12C | rs121913530 | A-051 |
TP53 | 17 | 7574026 | C | A | 636 | 3.77 | Missense | N/A | G202V | N/A | A-067 |
TP53 | 17 | 7577114 | C | A | 860 | 3.60 | Missense | Likely pathogenic | C143F | rs863224451 | A-027 |
TP53 | 17 | 7577575 | A | G | 2241 | 9.95 | Missense | Conflicting interpretations of pathogenicity | Y104H | rs587782289 | A-013 |
TP53 | 17 | 7577580 | T | C | 937 | 5.55 | Missense | Pathogenic | Y102C | rs587780073 | B-008 |
TP53 | 17 | 7578403 | C | T | 1246 | 15.3 | Missense | Pathogenic | C44Y | rs786202962 | A-072 |
Chr, chromosome; Ref, reference allele; Alt, alternative allele; VAF, variant allele frequency; dbSNP, Single Nucleotide Polymorphism Database; N/A, not available.
Discussion
In this study, we prospectively recruited a group of patients with advanced NSCLC at diagnosis (Cohort A) and during targeted treatment (Cohort B) to evaluate the clinical utility of ctDNA in metastatic or recurrent lung cancer. The cfDNA concentration of pre-treatment blood samples could be used as an effective biomarker to predict patient prognosis; a higher cfDNA concentration showed better sensitivity for detecting ctDNA variants. Baseline ctDNA analysis by targeted gene panel sequencing identified a considerably high ratio of variants detected in tumor tissues, although ctDNA sequencing in PD patients showed relatively low concordance rates. Repeated ctDNA analysis detected possible resistant mutations in patients with PD; notably, several PD mutations were found only in ctDNA but not in biopsied tumor tissue.
Owing to its minimal invasiveness, liquid biopsy has been extensively investigated to assess prognosis and guide treatment options for lung cancer patients. Liquid biopsy, particularly in ctDNA analysis or testing, can provide updated mutational and disease heterogeneity information and can be used to track molecular evolution (8). However, the main limitation of ctDNA diagnostics is the generation of false-positive results due to short DNA fragment length, low quantity, and a small fraction of tumor DNA (27). Therefore, pre-analytical steps, including experiments, should be standardized and optimized for accurate ctDNA analysis. During this study, we attempted to obtain sufficient blood samples and followed the experimental guidelines to obtain precise ctDNA analysis results.
The prognostic value of cfDNA concentration in lung cancer has been investigated extensively. Patients with higher cfDNA concentrations showed worse OS, metastatic characteristics, and disease recurrence than those with lower cfDNA concentrations (21,22). These results highlight the potential of cfDNA as a biomarker for assessing lung cancer prognosis. In the present study, patients with increased cfDNA concentration had relatively poorer OS and higher sensitivity to ctDNA variant calling from blood samples compared with those of tissue sequencing at diagnosis (Cohort A). However, in patients with PD (Cohort B), there was no significant difference in OS between the high and low cfDNA concentration groups; in addition, there was a weak correlation between cfDNA concentration and sensitivity of ctDNA variant calling. These results may indicate that pre-treatment cfDNA concentrations are highly correlated with tumor burden in the initial state at diagnosis. In contrast, disease progression after treatment is more related to the resistance mechanisms rather than tumor burden and increases with cfDNA amount.
Multiple previous studies have revealed high concordance between ctDNA analysis and tissue sequencing data in many types of cancer, reflecting its potential in profiling tumor mutations in pre-treatment baselines and monitoring disease progression (28-30). Nevertheless, the concordance between tumor-driven mutations in ctDNA and tumor tissue was relatively low in lung cancer compared with that in other cancers, possibly due to anatomical or tumor environmental conditions (31). Although our data showed slightly lower sensitivity (~60%) compared to other studies, detection sensitivity may vary depending on the sequencing panel, data analysis method, patient disease status, and comparison method between tumor tissue and ctDNA (32-34). Nevertheless, the sensitivity of our data for detecting lung cancer-related tumor mutations by ctDNA analysis indicated that more optimized experimental protocols and accurate data analysis algorithms are required. The most renowned genes related to lung cancer include TP53, EGFR, KRAS, and ALK, and the mutational frequencies of these genes are also prevalent in lung cancer patients. As expected, TP53 and EGFR were most frequently mutated in both Cohorts A and B. Despite lower concordance, these mutational patterns from ctDNA were highly similar to those from tumor tissue. As a result of cfDNA concentration analysis, the concordance between ctDNA and tumor tissue was lower in patients with PD after treatment (Cohort B) than that in patients at diagnosis (Cohort A). This was possibly due to the progression of tumor heterogeneity or the development of sub-clonal resistance mutations after treatment, which induced differences between systemic ctDNA and localized tumor tissue. On the other hand, disease progression under treatment without increasing ctDNA release to blood could be a reason for the low rate of variant detection from ctDNA in PD.
Despite insufficient concordance between tumor tissue and ctDNA for EGFR variants, the discovery of ctDNA-specific variants suggests that ctDNA analysis can be an additional approach for detecting resistance mutations and monitoring disease progression in patients. Because of tumor heterogeneity and the sub-clonal nature of resistant tumors, ctDNA, capable of representing systemic tumor status, is complementary to tissue biopsy to accurately detect treatment-resistant mutations. Considering that the EGFR T790M variant is the most notable signature of EGFR-targeted therapy resistance, our study showed that ctDNA-based sequencing could be beneficial for measuring acquired resistance during disease progression, even though sequencing from localized tumor tissue was performed but missed resistant mutations. In addition to the EGFR T790M variant, KRAS G12C, which was specifically found in the ctDNA of one patient, could influence changes in treatment with targeted therapy, demonstrating that ctDNA analysis has clinical utility in patients with PD after treatment.
For TMB analysis, low concordance between blood and tissue TMB indicates that mutational analysis from liquid biopsies may not be sufficient to substitute tissue testing (35). Our results consistently illustrated that the calculated TMB for Cohorts A and B between tumor tissue and ctDNA was not exactly identical for most patients. Our sequencing data were based on an approximately 1.1-Mb targeted gene panel, which was insufficient to calculate TMB; therefore, it might be inappropriate to evaluate the validity of the TMB test from ctDNA in this study. For precise TMB calculation, whole exome sequencing or a specifically designed panel would be required for TMB analysis.
This study had several limitations regarding sample acquisition and data interpretation. Despite efforts to obtain appropriate samples and sequencing data, there were some loss of samples and inadequate data among Cohorts A and B patients (low concentration or total amount of ctDNA and genomic DNA contamination). In addition, matched normal tissue and tumor tissue purity was unavailable. The study was initially designed the study to collect serial blood samples during patient treatment to monitor disease course by ctDNA analysis, but time and cost were restricted; therefore, we could only analyze ctDNA sequencing at pre-treatment and after disease progression. The serial ctDNA analysis may be used in treatment monitoring along with tumor markers or imaging and in early detection of disease progression. This serial ctDNA sequencing analysis was left for future work. To improve the detection accuracy of somatic variant calling by ctDNA analysis, sequencing data from matched white blood cells are required to exclude germline mutations or variants derived from clonal hematopoiesis (36). Although this strategy could decrease the false-positive rates in ctDNA sequencing data, these samples were unavailable in the present study. Instead, we applied the population frequencies of the variants from public data to filter out germline mutations and selected lung cancer-related variants based on a database of cancer somatic mutations to reduce noise variants. However, additional experiments with white blood cells would be more effective for accurate ctDNA analysis.
Conclusions
Here, this study evaluated the clinical validity of NGS-based ctDNA analysis for lung cancer treatment. In conclusion, this study demonstrated the potential of cfDNA in blood samples as a biomarker for the prognosis of advanced lung cancer at diagnosis and the possibility of alternative or additional use of ctDNA sequencing as molecular tumor diagnosis before and after treatment; however, insufficient concordance in variant detection between tumor tissue and ctDNA sequencing results remains a limitation. The study suggests ctDNA analysis as a supplementary approach for tumor tissue biopsy at present, but improvement of the ctDNA analysis would promote more clinical utility and replace the tumor biopsy in the future. Therefore, NGS-based ctDNA analysis should be further evaluated using standardized experimental protocols and optimized analytic procedures with additional data supporting evidence of tumor mutations and filtering out germline or innate noise variants.
Acknowledgments
The authors would like to thank all patients who participated in this study and their caregivers for their cooperation.
Funding: This study was supported by a grant from the SNUBH Research Fund (grant No. 02-2017-0038 to JSL), the World Class 300 Project (S2638360) of the Ministry of Trade, Industry and Energy (MOTIE; https://english.motie.go.kr/www/main.do), and the Ministry of SMEs and Startups (MSS; https://www.mss.go.kr/site/eng/main.do) of the Republic of Korea.
Footnote
Reporting Checklist: The authors have completed the REMARK reporting checklist. Available at https://tlcr.amegroups.com/article/view/10.21037/tlcr-22-912/rc
Data Sharing Statement: Available at https://tlcr.amegroups.com/article/view/10.21037/tlcr-22-912/dss
Peer Review File: Available at https://tlcr.amegroups.com/article/view/10.21037/tlcr-22-912/prf
Conflicts of Interest: All authors have completed the ICMJE uniform disclosure form (available at https://tlcr.amegroups.com/article/view/10.21037/tlcr-22-912/coif). Sungjae Kim and NJK are employees of Macrogen Inc. The other authors have no conflicts of interest to declare.
Ethical Statement: The authors are accountable for all aspects of the work in ensuring that questions related to the accuracy or integrity of any part of the work are appropriately investigated and resolved. The study was conducted in accordance with the Declaration of Helsinki (as revised in 2013). The study was approved by Institutional Review Board (IRB) of Seoul National University Bundang Hospital (SNUBH) (IRB No. B-1703-387-301) and informed consent was obtained from all individual participants. The detailed study design and information are available from ClinicalTrials.gov (identifier: NCT03235765).
Open Access Statement: This is an Open Access article distributed in accordance with the Creative Commons Attribution-NonCommercial-NoDerivs 4.0 International License (CC BY-NC-ND 4.0), which permits the non-commercial replication and distribution of the article with the strict proviso that no changes or edits are made and the original work is properly cited (including links to both the formal publication through the relevant DOI and the license). See: https://creativecommons.org/licenses/by-nc-nd/4.0/.
References
- Barta JA, Powell CA, Wisnivesky JP. Global Epidemiology of Lung Cancer. Ann Glob Health 2019;85:8. [Crossref] [PubMed]
- Cainap C, Pop LA, Balacescu O, et al. Early diagnosis and screening in lung cancer. Am J Cancer Res 2020;10:1993-2009. [PubMed]
- Jung KW, Won YJ, Kang MJ, et al. Prediction of Cancer Incidence and Mortality in Korea, 2022. Cancer Res Treat 2022;54:345-51. [Crossref] [PubMed]
- Kris MG, Johnson BE, Berry LD, et al. Using multiplexed assays of oncogenic drivers in lung cancers to select targeted drugs. JAMA 2014;311:1998-2006. [Crossref] [PubMed]
- Guibert N, Pradines A, Favre G, et al. Current and future applications of liquid biopsy in nonsmall cell lung cancer from early to advanced stages. Eur Respir Rev 2020;29:190052. [Crossref] [PubMed]
- Rolfo C, Russo A. Liquid biopsy for early stage lung cancer moves ever closer. Nat Rev Clin Oncol 2020;17:523-4. [Crossref] [PubMed]
- Freitas C, Sousa C, Machado F, et al. The Role of Liquid Biopsy in Early Diagnosis of Lung Cancer. Front Oncol 2021;11:634316. [Crossref] [PubMed]
- Corcoran RB, Chabner BA. Application of Cell-free DNA Analysis to Cancer Treatment. N Engl J Med 2018;379:1754-65. [Crossref] [PubMed]
- Esposito Abate R, Frezzetti D, Maiello MR, et al. Next Generation Sequencing-Based Profiling of Cell Free DNA in Patients with Advanced Non-Small Cell Lung Cancer: Advantages and Pitfalls. Cancers (Basel) 2020;12:3804. [Crossref] [PubMed]
- Kim S, Lim Y, Kang JK, et al. Dynamic changes in longitudinal circulating tumour DNA profile during metastatic colorectal cancer treatment. Br J Cancer 2022;127:898-907. [Crossref] [PubMed]
- Abbosh C, Birkbak NJ, Swanton C. Early stage NSCLC - challenges to implementing ctDNA-based screening and MRD detection. Nat Rev Clin Oncol 2018;15:577-86. [Crossref] [PubMed]
- Li H, Durbin R. Fast and accurate short read alignment with Burrows-Wheeler transform. Bioinformatics 2009;25:1754-60. [Crossref] [PubMed]
- DePristo MA, Banks E, Poplin R, et al. A framework for variation discovery and genotyping using next-generation DNA sequencing data. Nat Genet 2011;43:491-8. [Crossref] [PubMed]
- Cibulskis K, Lawrence MS, Carter SL, et al. Sensitive detection of somatic point mutations in impure and heterogeneous cancer samples. Nat Biotechnol 2013;31:213-9. [Crossref] [PubMed]
- Yoo SK, Kim CU, Kim HL, et al. NARD: whole-genome reference panel of 1779 Northeast Asians improves imputation accuracy of rare and low-frequency variants. Genome Med 2019;11:64. [Crossref] [PubMed]
- Wang K, Li M, Hakonarson H. ANNOVAR: functional annotation of genetic variants from high-throughput sequencing data. Nucleic Acids Res 2010;38:e164. [Crossref] [PubMed]
- Karczewski KJ, Francioli LC, Tiao G, et al. The mutational constraint spectrum quantified from variation in 141,456 humans. Nature 2020;581:434-43. [Crossref] [PubMed]
- Tate JG, Bamford S, Jubb HC, et al. COSMIC: the Catalogue Of Somatic Mutations In Cancer. Nucleic Acids Res 2019;47:D941-7. [Crossref] [PubMed]
- Adzhubei I, Jordan DM, Sunyaev SR. Predicting functional effect of human missense mutations using PolyPhen-2. Curr Protoc Hum Genet 2013;Chapter 7:Unit7.20.
- Sim NL, Kumar P, Hu J, et al. SIFT web server: predicting effects of amino acid substitutions on proteins. Nucleic Acids Res 2012;40:W452-7. [Crossref] [PubMed]
- Mirtavoos-Mahyari H, Ghafouri-Fard S, Khosravi A, et al. Circulating free DNA concentration as a marker of disease recurrence and metastatic potential in lung cancer. Clin Transl Med 2019;8:14. [Crossref] [PubMed]
- Viller Tuxen I, Barlebo Ahlborn L, Mau-Soerensen M, et al. Plasma total cell-free DNA is a prognostic biomarker of overall survival in metastatic solid tumour patients. Br J Cancer 2019;121:125-30. [Crossref] [PubMed]
- Liu X, Lin XJ, Wang CP, et al. Association between smoking and p53 mutation in lung cancer: a meta-analysis. Clin Oncol (R Coll Radiol) 2014;26:18-24. [Crossref] [PubMed]
- Biton J, Mansuet-Lupo A, Pécuchet N, et al. TP53, STK11, and EGFR Mutations Predict Tumor Immune Profile and the Response to Anti-PD-1 in Lung Adenocarcinoma. Clin Cancer Res 2018;24:5710-23. [Crossref] [PubMed]
- Dong ZY, Zhong WZ, Zhang XC, et al. Potential Predictive Value of TP53 and KRAS Mutation Status for Response to PD-1 Blockade Immunotherapy in Lung Adenocarcinoma. Clin Cancer Res 2017;23:3012-24. [Crossref] [PubMed]
- Li Q, Zhou Q, Zhao S, et al. KRAS mutation predict response and outcome in advanced non-small cell lung carcinoma without driver alterations receiving PD-1 blockade immunotherapy combined with platinum-based chemotherapy: a retrospective cohort study from China. Transl Lung Cancer Res 2022;11:2136-47. [Crossref] [PubMed]
- Song P, Wu LR, Yan YH, et al. Limitations and opportunities of technologies for the analysis of cell-free DNA in cancer diagnostics. Nat Biomed Eng 2022;6:232-45. [Crossref] [PubMed]
- Jahangiri L, Hurst T. Assessing the Concordance of Genomic Alterations between Circulating-Free DNA and Tumour Tissue in Cancer Patients. Cancers (Basel) 2019;11:1938. [Crossref] [PubMed]
- Kang JK, Heo S, Kim HP, et al. Liquid biopsy-based tumor profiling for metastatic colorectal cancer patients with ultra-deep targeted sequencing. PLoS One 2020;15:e0232754. [Crossref] [PubMed]
- García-Foncillas J, Tabernero J, Élez E, et al. Prospective multicenter real-world RAS mutation comparison between OncoBEAM-based liquid biopsy and tissue analysis in metastatic colorectal cancer. Br J Cancer 2018;119:1464-70. [Crossref] [PubMed]
- Zhang Y, Yao Y, Xu Y, et al. Pan-cancer circulating tumor DNA detection in over 10,000 Chinese patients. Nat Commun 2021;12:11. [Crossref] [PubMed]
- Leighl NB, Page RD, Raymond VM, et al. Clinical Utility of Comprehensive Cell-free DNA Analysis to Identify Genomic Biomarkers in Patients with Newly Diagnosed Metastatic Non-small Cell Lung Cancer. Clin Cancer Res 2019;25:4691-700. [Crossref] [PubMed]
- Li BT, Janku F, Jung B, et al. Ultra-deep next-generation sequencing of plasma cell-free DNA in patients with advanced lung cancers: results from the Actionable Genome Consortium. Ann Oncol 2019;30:597-603. [Crossref] [PubMed]
- Park S, Olsen S, Ku BM, et al. High concordance of actionable genomic alterations identified between circulating tumor DNA-based and tissue-based next-generation sequencing testing in advanced non-small cell lung cancer: The Korean Lung Liquid Versus Invasive Biopsy Program. Cancer 2021;127:3019-28. [Crossref] [PubMed]
- Fridland S, Choi J, Nam M, et al. Assessing tumor heterogeneity: integrating tissue and circulating tumor DNA (ctDNA) analysis in the era of immuno-oncology - blood TMB is not the same as tissue TMB. J Immunother Cancer 2021;9:e002551. [Crossref] [PubMed]
- Razavi P, Li BT, Brown DN, et al. High-intensity sequencing reveals the sources of plasma circulating cell-free DNA variants. Nat Med 2019;25:1928-37. [Crossref] [PubMed]