A prognostic nomogram of non-small cell lung cancer based on tumor marker inflammatory nutrition score
Highlight box
Key findings
• The tumor marker inflammatory nutrition (TMIN) score is a novel and effective clinical prognostic tool for overall survival (OS) and progression-free survival (PFS) in non-small cell lung cancer (NSCLC) patients.
What is known and what is new?
• Lung cancer is a major public health problem, and NSCLC is still the leading cause of cancer death in the world.
• TMIN is a promising predictor for PFS and OS in NSCLC patients.
What is the implication, and what should change now?
• The TMIN-nomogram prediction model can be used as an effective tool for the comprehensive prognosis evaluation of NSCLC patients.
Introduction
At present, lung cancer is a major public health problem, non-small cell lung cancer (NSCLC) is still the leading cause of cancer death in the world (1,2). Despite recent advances in the diagnosis and treatment of NSCLC, the majority of patients are still diagnosed with advanced disease, and the mortality rate of advanced patients is still high (3). Therefore, it is critical to identify and define reliable tumor prognostic biomarkers to predict risk and make appropriate treatment decisions.
There is an intricate network of relationships between inflammation and tumor (4). Inflammation can not only act as a catalyst for tumor initiation and expansion, but also be reacted by tumors in their microenvironment through diversified mechanisms (5). Therefore, it is necessary to further study the interaction mechanism between inflammation and tumor to provide new ideas and methods for tumor treatment (6,7). There is increasing evidence that a variety of nutritional/inflammation-related factors can be used as effective prognostic predictors of lung cancer. Systemic inflammatory/nutritional markers, such as platelet/lymphocyte ratio (PLR) (8), lymphocyte/monocyte ratio (LMR) (9), and hemoglobin, albumin, lymphocytes and platelets (HALP) (10) have been shown to play important roles in the progression and prognosis of lung cancer patients. Nomograms are intuitive visual prediction tools that are considered a reliable way to quantify cancer risk. Nomograms can make prediction models more targeted by integrating important pathological and clinical features and adding useful potential biomarkers, thereby enabling precise and personalized treatment (11,12). Therefore, in this study, we combined several clinical variables to construct and validate a nomogram to predict the prognosis of NSCLC patients, and compared its predictive performance with the traditional tumor lymph node metastasis (TNM) system-based nomogram. We present this article in accordance with the TRIPOD reporting checklist (available at https://tlcr.amegroups.com/article/view/10.21037/tlcr-24-708/rc).
Methods
Patient selection criteria
We retrospectively collected patients diagnosed with NSCLC in Qingdao Municipal Hospital from January 2009 to December 2019. Inclusion criteria were as follows: (I) NSCLC diagnosed by cytology or pathology in our hospital; (II) complete clinical, pathological and imaging data; (III) receiving first-line treatment, including surgery, chemotherapy, or targeted therapy. Exclusion criteria: (I) patients had obvious infection in the past month or received anti-inflammatory treatment within 1 month before enrollment; (II) complicated with serious diseases of the heart, liver, kidney and blood system; (III) complicated with other tumors. Finally, 486 patients were included in the study. All patients were pathologically staged according to the 8th edition of the American Joint Committee on Cancer (AJCC) TNM staging system. The study was conducted in accordance with the Declaration of Helsinki (as revised in 2013). The study was approved by the Ethics Committee of Qingdao Municipal Hospital (No. 2024-LW-008). All retrospective data including this study were anonymized; therefore, the requirement of informed consent for this retrospective study was waived.
Data collection
Clinical and laboratory data were collected from the electronic medical record of our hospital. The blood indexes of patients within 1 week before diagnosis were collected, including white blood cell (WBC), neutrophil (N), lymphocyte (LYM), monocyte (M), platelet (PLT), hemoglobin (Hb), albumin (ALB), globulin (GLB), prealbumin (PAB), fibrinogen (FIB), carcinoembryonic antigen (CEA) and cytokeratin fragment antigen 21-1 (CYFRA21-1). Neutrophil/lymphocyte count ratio (NLR), PLR, LMR and HALP were calculated.
Case follow-up
Follow-up began from the date of diagnosis, including outpatient or inpatient reexamination and telephone follow-up. Follow-up included subsequent treatment, efficacy, recurrence and time of death. Overall survival (OS) was defined as the time from diagnosis to death or last follow-up. Progression-free survival (PFS) was defined as the time from diagnosis to tumor progression or last follow-up.
Statistical analysis
Patients were randomly divided into training group and validation group using SPSS 26.0.1 software. Kaplan-Meier curve was used to evaluate OS and PFS. The least absolute shrinkage and selection operator (LASSO) model was performed using the R 4.2.1 software “glmnet” package. Transient receiver operating characteristic (ROC) curves and time-dependent ROC values were plotted and area under curve (AUC) values were calculated using the “timeROC” R package. Univariate and multivariate COX regression analyses were used to evaluate the relationship between clinical indicators and OS and PFS in patients with lung cancer, and the hazard ratio (HR) and 95% confidence interval (CI) were calculated. The nomogram was constructed using the “rms” R package and a calibration plot was drawn using 1000 bootstrap resampling to obtain the C-index for further analysis of the performance of the nomogram. Decision curve analysis (DCA) was used to compare the accuracy of the nomogram and the AJCC TNM staging system. Graph Pad Prism 8.1.0 and R were used to analyze and plot the data. When P<0.05, the difference was statistically significant.
Results
Demographic characteristics
A total of 486 lung cancer patients were enrolled in this single-center study and randomly divided into training cohort (n=340) and validation cohort (n=146) in a ratio of 7:3. Table 1 provides an overview of the clinicopathological characteristics at baseline. A total of 486 patients were collected, including 302 males (62.14%) and 184 females (37.86%), with a median age of 62 [56, 67] years. There were 222 cases (45.68%) with smoking history and 264 cases (54.32%) without smoking history. The clinicopathological staging showed that 228 cases (46.91%) were in stage I–II and 258 cases (53.09%) were in stage III–IV. The correlation analysis of clinical baseline data and pathological characteristics in the training set and the validation set showed that the distribution was balanced between the two cohorts, and there was no statistically significant difference (P>0.05, Table 1).
Table 1
Characteristics | Training set (n=340) | Validation set (n=146) | Total set (n=486) | P value |
---|---|---|---|---|
Sex | 0.80 | |||
Male | 208 (61.18) | 94 (64.38) | 302 (62.14) | |
Female | 132 (38.82) | 52 (35.62) | 184 (37.86) | |
Age (years) | 62 (56, 67) | 62 (57, 68) | 62 (56, 67) | 0.85 |
Smoke | >0.99 | |||
No | 185 (54.41) | 79 (54.11) | 264 (54.32) | |
Yes | 155 (45.59) | 67 (45.89) | 222 (45.68) | |
Pathological classification | 0.61 | |||
Adenocarcinoma | 260 (76.47) | 121 (82.88) | 381 (78.40) | |
Squamous carcinoma | 70 (20.59) | 21 (14.38) | 91 (18.72) | |
Other | 10 (2.94) | 4 (2.74) | 14 (2.88) | |
Tumor location | 0.80 | |||
Upper | 193 (56.76) | 83 (56.85) | 276 (56.79) | |
Middle | 24 (7.06) | 15 (10.27) | 39 (8.02) | |
Lower | 123 (36.18) | 48 (32.88) | 171 (35.19) | |
Tumor diameter (cm) | 3.25 (1.8, 5) | 3.15 (2, 5) | 3.2 (1.9, 5) | |
AJCC stage | ||||
I | 109 (32.06) | 47 (32.19) | 156 (32.10) | |
II | 51 (15.00) | 21 (14.38) | 72 (14.81) | |
III | 73 (21.47) | 35 (23.97) | 108 (22.22) | |
IV | 107 (31.47) | 43 (29.45) | 150 (30.86) | |
Metastasis | 0.88 | |||
No | 232 (68.24) | 103 (70.55) | 335 (68.93) | |
Yes | 108 (31.76) | 43 (29.45) | 151 (31.07) | |
WBC (109/L) | 6.7 (5.4, 8.31) | 6.525 (5.45, 7.74) | 6.64 (5.4, 8.28) | 0.88 |
N (109/L) | 4.1 (3.05, 5.44) | 3.955 (2.99, 4.89) | 4.05 (3.02, 5.21) | 0.55 |
LYM (109/L) | 1.855 (1.45, 2.29) | 1.9 (1.48, 2.36) | 1.86 (1.45, 2.31) | 0.66 |
M (109/L) | 0.45 (0.34, 0.57) | 0.39 (0.31, 0.54) | 0.44 (0.33, 0.56) | 0.18 |
PLT (109/L) | 246.5 (200.75, 296) | 225.5 (188, 292) | 239 (194, 295.5) | 0.18 |
Hb (g/L) | 135 (123, 143.25) | 138 (124, 148.75) | 135 (123, 145) | 0.47 |
ALB (g/L) | 39.22 (35.91, 42.41) | 39.445 (36.68, 42.35) | 39.225 (36.28, 42.4) | 0.78 |
GLB (g/L) | 29.05 (25.97, 32.38) | 28.64 (26, 32.83) | 28.965 (25.97, 32.64) | 0.98 |
PAB (mg/L) | 241.73 (171.84, 311.63) | 251.55 (180.16, 322.94) | 244.68 (174.26, 315.1) | 0.37 |
CEA (ng/mL) | 3.555 (1.9, 7.91) | 4.155 (2.21, 9.41) | 3.575 (1.92, 8.75) | 0.52 |
CYFRA21-1 (ng/mL) | 3.285 (2.27, 6.69) | 3.275 (2.2, 5.65) | 3.28 (2.26, 6.46) | 0.87 |
FIB (g/L) | 3.4 (2.83, 4.37) | 3.305 (2.66, 4.13) | 3.39 (2.8, 4.34) | 0.22 |
ECOG-PS | 0.77 | |||
<2 | 231 (67.94) | 104 (71.23) | 335 (68.93) | |
≥2 | 109 (32.06) | 42 (28.77) | 151 (31.07) | |
NLR | 0.72 | |||
≤2.11 | 168 (49.41) | 78 (53.42) | 246 (50.62) | |
>2.11 | 172 (50.59) | 68 (46.58) | 240 (49.38) | |
PLR | 0.62 | |||
≤129.68 | 170 (50) | 80 (54.79) | 250 (51.44) | |
>129.68 | 170 (50) | 66 (45.21) | 236 (48.56) | |
HALP | 0.52 | |||
≥35.95 | 193 (56.76) | 91 (62.33) | 284 (58.44) | |
<35.95 | 147 (43.24) | 55 (37.67) | 202 (41.56) | |
LMR | 0.62 | |||
≥3.74 | 203 (59.71) | 94 (64.38) | 297 (61.11) | |
<3.74 | 137 (40.29) | 52 (35.62) | 189 (38.89) |
Data are presented as n (%) or median (IQR). AJCC, American Joint Committee on Cancer; WBC, white blood cell; N, neutrophil; LYM, lymphocyte; M, monocyte; PLT, platelet; Hb, hemoglobin; ALB, albumin; GLB, globulin; PAB, prealbumin; CEA, carcinoembryonic antigen; CYFRA21-1, cytokeratins21-1; FIB, fibrinogen; ECOG-PS, Eastern Cooperative Oncology Group performance status; NLR, neutrophil to lymphocyte ratio; PLR, platelet to lymphocyte ratio; HALP, hemoglobin-albumin-lymphocytes-platelets; LMR, lymphocyte-to-monocyte ratio; IQR, interquartile range.
Construction of a new indicator tumor marker of inflammatory nutrition (TMIN)
Next, Kaplan-Meier survival analysis was performed on blood-related indicators, and the results showed that patients in WBC, N, M, PLT, GLB, CEA, CYFRA21-1, FIB, NLR, and PLR-High groups had significantly prolonged OS. However, patients in the LYM, Hb, ALB, PAB, HALP, and LMR-low groups had significantly longer OS (Figure 1). Similarly, PFS was significantly prolonged in the WBC, N, M, PLT, GLB, CEA, CYFRA21-1, FIB, NLR and PLR-high groups. However, patients in the LYM, Hb, ALB, PAB, HALP, and LMR-low groups had significantly prolonged PFS (Figure S1). Subsequently, we constructed a Cox regression clinical prognostic model based on Lasso after screening the variables, and constructed TMIN by selecting the 11 most valuable prognostic variables from 16 indicators, including WBC, N, PLT, ALB, GLB, PAB, CEA, CYFRA21-1, FIB, PLR, and LMR (Figure 2A,2B). TMIN is calculated according to the following formula: TMIN = 0.000449 × WBC + 0.131340 × N + 0.000396 × PLT-0.039098 × ALB + 0.007827 × GLB-0.002156 × PAB + 0.007235 × CEA + 0.076914 × CYFRA21-1 + 0.208253 × FIB + 0.000741 × PLR-0.040533 × LMR. According to the optimal cut-off value (TMIN =−0.35) determined by ROC curve, patients were divided into low-risk group (TMIN ≤−0.35) and high-risk group (TMIN >−0.35). We further analyzed the correlation between TMIN score and clinicopathological characteristics (Table 2). It can be seen that TMIN was associated with sex (P<0.001, P=0.02), smoke (P<0.001, P=0.04), pathological classification (P<0.001, P=0.005), AJCC stage (P<0.001, P<0.001), tumor diameter (P<0.001, P<0.001, respectively) and Eastern Cooperative Oncology Group-performance status (ECOG-PS) (P<0.001, P=0.001) in the training cohort and validation cohort. This indicates that TMIN score has a good correlation with clinicopathological features.
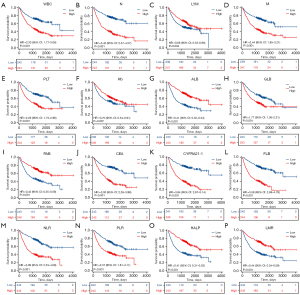
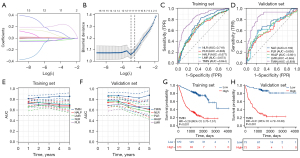
Table 2
Characteristics | Training set | Validation set | |||||
---|---|---|---|---|---|---|---|
Low risk (n=140) | High risk (n=200) | P value | Low risk (n=72) | High risk (n=74) | P value | ||
Sex | <0.001* | 0.02* | |||||
Female | 74 (52.86) | 58 (29.00) | 32 (44.44) | 20 (27.03) | |||
Male | 66 (47.14) | 142 (71.00) | 40 (55.56) | 54 (72.97) | |||
Age (years) | <0.001* | 0.18 | |||||
≤60 | 77 (55.00) | 63 (31.50) | 31 (43.06) | 24 (32.43) | |||
>60 | 63 (45.00) | 137 (68.50) | 41 (56.94) | 50 (67.57) | |||
Smoke | <0.001* | 0.04* | |||||
No | 96 (68.57) | 89 (44.50) | 45 (62.5) | 34 (45.95) | |||
Yes | 44 (31.43) | 111 (55.50) | 27 (37.5) | 40 (54.05) | |||
Pathological classification | <0.001* | 0.005* | |||||
Adenocarcinoma | 127 (90.71) | 133 (66.50) | 66 (91.67) | 55 (74.32) | |||
Non-adenocarcinoma | 13 (9.29) | 67 (33.50) | 6 (8.33) | 19 (25.68) | |||
Tumor location | 0.21 | 0.63 | |||||
M/L | 55 (39.29) | 92 (46.00) | 33 (45.83) | 31 (41.89) | |||
U | 85 (60.71) | 108 (54.00) | 39 (54.17) | 43 (58.11) | |||
Metastasis | 0.19 | <0.001* | |||||
No | 101 (72.14) | 131 (65.50) | 65 (90.28) | 38 (51.35) | |||
Yes | 39 (27.86) | 69 (34.50) | 7 (9.72) | 36 (48.65) | |||
AJCC stage | <0.001* | <0.001* | |||||
I/II | 105 (75.00) | 55 (27.50) | 53 (73.61) | 15 (20.27) | |||
III/IV | 35 (25.00) | 145 (72.50) | 19 (26.39) | 59 (79.73) | |||
Tumor diameter (cm) | <0.001* | <0.001* | |||||
<3.0 | 110 (78.57) | 55 (27.50) | 55 (76.39) | 16 (21.62) | |||
≥3.0 | 30 (21.43) | 145 (72.50) | 17 (23.61) | 58 (78.38) | |||
PLR | <0.001* | <0.001* | |||||
≤129.68 | 105 (75.00) | 65 (32.50) | 52 (72.22) | 28 (37.84) | |||
>129.68 | 35 (25.00) | 135 (67.50) | 20 (27.78) | 46 (62.16) | |||
LMR | <0.001* | <0.001* | |||||
≥3.74 | 124 (88.57) | 79 (39.50) | 66 (91.67) | 28 (37.84) | |||
<3.74 | 16 (11.43) | 121 (60.50) | 6 (8.33) | 46 (62.16) | |||
ECOG-PS | <0.001* | 0.001* | |||||
<2 | 115 (82.14) | 116 (58.00) | 60 (83.33) | 44 (59.46) | |||
≥2 | 25 (17.86) | 84 (42.00) | 12 (16.67) | 30 (40.54) |
Data are presented as n (%). *, P<0.05. TMIN, tumor marker inflammatory nutrition; M/L, middle/lower; U, upper; AJCC, American Joint Committee on Cancer; PLR, platelet/lymphocyte ratio; LMR, lymphocyte/monocyte ratio; ECOG-PS, Eastern Cooperative Oncology Group performance status.
Relationship between TMIN and patient prognosis
To compare the predictive ability of TMIN and other inflammatory nutritional markers for the prognosis of NSCLC patients, ROC curves were drawn and AUC was calculated. The ROC curve showed that the AUC of TMIN in the training set (AUC =0.844) and validation set (AUC =0.856) was higher than that of the other combined indicators (Figure 2C,2D), which indicated that TMIN had good diagnostic value for patients. The prognostic ROC curve showed the prediction of TMIN for patients at 1, 3, and 5 years. The AUC values of the training group were 0.814, 0.842, and 0.858, respectively, and the AUC values of the validation group were 0.863, 0.853, and 0.878, respectively (Figure 2E,2F). Kaplan-Meier curves showed that high-risk patients had significantly lower OS than low-risk patients in both the training and validation cohorts (Figure 2G,2H). At the same time, to better evaluate the prognostic power of the TMIN risk score, we performed a stratified analysis to confirm whether it retained the power to predict OS in different subgroups. We found that in both the training cohort and the validation cohort, the high-risk group had worse OS and PFS than the low TMIN group in both the younger (≤60 years) and older (>60 years) groups (Figure 3, Figure S2). Similarly, the same differences were observed between women and men, and between never-smokers and smokers (Figure 3, Figure S2).
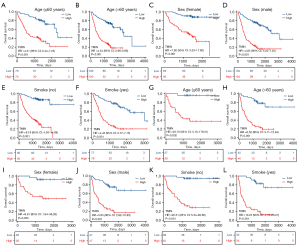
Subsequently, we performed Cox regression analysis on TMIN and clinical baseline data (Table 3). Univariate analysis showed that female, smoking, pathological type (non-adenocarcinoma), large tumor diameter (≥3.0 cm), advanced stage (III, IV), metastasis, ECOG-PS ≥2 and high TMIN level were associated with shorter OS. In multivariate analysis, sex (HR: 2.052, 95% CI: 1.297–3.246, P=0.002), AJCC stage (HR: 3.106, 95% CI: 1.887–5.115, P<0.001), metastasis (HR: 1.799, 95% CI: 1.227–2.637, P=0.003), ECOG-PS (HR: 1.377, 95% CI: 1.002–1.891, P=0.04); and TMIN (HR: 0.357, 95% CI: 0.217–0.588, P<0.001) were independent prognostic factors for NSCLC patients (Table 3). Similarly, univariate analysis showed that male, smoking, non-adenocarcinoma, large tumor diameter, advanced stage, metastasis, ECOG-PS ≥2 and high TMIN level were associated with shorter PFS. In multivariate analysis, sex (HR: 1.890, 95% CI: 1.194–2.992, P=0.007), tumor diameter (HR: 1.526, 95% CI: (1.017–2.290, P=0.04), AJCC stage (HR: 3.458, 95% CI: 2.087–5.73, P<0.001), metastasis (HR: 1.754, 95% CI: 1.192–2.580, P=0.004) and TMIN (HR: 2.782, 95% CI: 1.505–4.094, P<0.001) were independent prognostic factors for NSCLC patients (Table S1).
Table 3
Characteristics | Total (N) | Univariate analysis | Multivariate analysis | |||
---|---|---|---|---|---|---|
HR (95% CI) | P value | HR (95% CI) | P value | |||
Age (years) | 340 | |||||
>60 | 200 | Reference | ||||
≤60 | 140 | 0.914 (0.669–1.250) | 0.57 | |||
Sex | 340 | |||||
Male | 208 | Reference | Reference | |||
Female | 132 | 1.920 (1.361–2.709) | <0.001* | 2.052 (1.297–3.246) | 0.002* | |
Smoke | 340 | |||||
No | 185 | Reference | Reference | |||
Yes | 155 | 1.371 (1.010–1.863) | 0.04* | 0.717 (0.473–1.087) | 0.11 | |
Pathological classification | 340 | |||||
Adenocarcinoma | 260 | Reference | Reference | |||
Non-adenocarcinoma | 80 | 1.950 (1.406–2.703) | <0.001* | 1.160 (0.800–1.684) | 0.43 | |
Tumor location | 340 | |||||
M/L | 147 | Reference | ||||
U | 193 | 1.049 (0.770–1.429) | 0.76 | |||
Tumor diameter (cm) | 340 | |||||
<3.0 | 165 | Reference | Reference | |||
≥3.0 | 175 | 4.172 (2.917–5.968) | <0.001* | 1.377 (0.923–2.057) | 0.11 | |
AJCC stage | 340 | |||||
I/II | 160 | Reference | Reference | |||
III/IV | 180 | 7.316 (4.859–11.014) | <0.001* | 3.106 (1.887–5.115) | <0.001* | |
Metastasis | 340 | |||||
No | 232 | Reference | Reference | |||
Yes | 108 | 5.271 (3.848–7.220) | <0.001* | 1.799 (1.227–2.637) | 0.003* | |
ECGO-PS | 340 | |||||
<2 | 231 | Reference | Reference | |||
≥2 | 109 | 2.205 (1.620–3.001) | <0.001* | 1.377 (1.002–1.891) | 0.04* | |
TMIN score | 340 | |||||
>−0.35 | 200 | Reference | Reference | |||
≤−0.35 | 140 | 0.148 (0.096–0.229) | <0.001* | 0.357 (0.217–0.588) | <0.001* |
*, P<0.05. OS, overall survival; M/L, middle/lower; U, upper; AJCC, American Joint Committee on Cancer; ECOG-PS, Eastern Cooperative Oncology Group performance status; TMIN, tumor marker inflammatory nutrition; HR, hazard ratio; CI, confidence interval.
Construction and evaluation of the prognostic nomogram
Based on the above independent prognostic factors, the nomogram based on TMIN was constructed to predict the OS and PFS of patients (Figure 4A,4B). ROC curves were used to evaluate the performance of the nomogram. The AUCs for 1-, 3-, and 5-year OS were 0.821, 0.872, and 0.852, respectively, in the training cohort and 0.887, 0.935, and 0.954, respectively, in the validation cohort (Figure 4C). Similarly, the AUCs for PFS at 1, 3, and 5 years were 0.826, 0.905, and 0.868, respectively, in the training cohort and 0.918, 0.971, and 0.950, respectively, in the validation cohort (Figure 4D). The nomogram was discriminated and calibrated, and the C-index for OS was 0.804 in the training cohort and 0.860 in the validation cohort, respectively. Meanwhile, the C-index of PFS was 0.793 in the training cohort and 0.867 in the validation cohort (Table 4). Furthermore, calibration curves for OS and PFS at 1, 3, and 5 years showed excellent agreement between predicted and actual probabilities in the training and validation cohorts (Figure 4E,4F). These results indicate that the model is a very good predictor of patient outcomes.
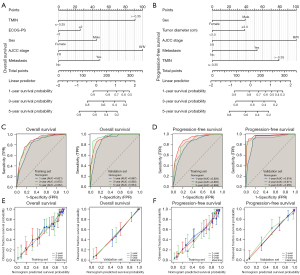
Table 4
Cohort | Cluster | C-index | 95% CI |
---|---|---|---|
Overall survival | Training set | 0.804 | 0.789–0.820 |
Validation set | 0.860 | 0.843–0.878 | |
Progression-free survival | Training set | 0.793 | 0.777–0.810 |
Validation set | 0.867 | 0.849–0.885 |
CI, confidence interval.
To evaluate the prognostic value of the TMIN-based nomogram and AJCC TNM staging for patients, ROC curves were used for further analysis. In the training cohort, the AUC in the OS nomogram was 0.888 and the AJCC TNM stage was 0.865 (Figure 5A). Similarly, in the validation cohort, the AUC in the OS nomogram was 0.941, whereas in the validation cohort, the AUC for AJCC TNM stage was 0.880 (Figure 5B). Consistently, in the training cohort, the AUC in the PFS nomogram was 0.890 and the AJCC TNM stage was 0.865 (Figure 5C). Similarly, in the validation cohort, the AUC in the PFS nomogram was 0.955, whereas in the validation cohort, the AUC for AJCC TNM stage was 0.880 (Figure 5D). Time-dependent ROC curve analysis showed that the AUC of the 1-, 3-, and 5-year survival prediction nomograms in the training and validation cohorts were higher than those in the AJCC TNM stage (Figure 5E-5H). We believe that the predictive performance of the nomogram is better than that of the AJCC TNM stage. In addition, DCA showed that the nomogram had better clinical utility than the AJCC TNM staging system at 1 year (Figure 5I-5L). These results confirm that the TMIN-based nomogram can accurately and effectively predict the survival of NSCLC patients.
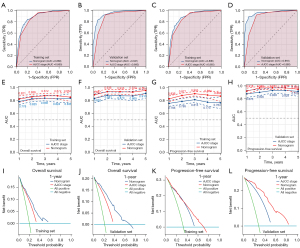
Discussion
Lung cancer is one of the most common human malignancies. About 85% of these lung cancer patients have NSCLC. It is estimated that more than 60% of patients with NSCLC have locally advanced or metastatic cancer (13), and despite significant advances in treatment, early diagnosis, and management of NSCLC in recent years, the overall prognosis of patients with advanced NSCLC remains unsatisfactory (1). Therefore, there is an urgent need to find a better prognostic indicator to improve outcomes. Multiple studies have shown that TNM stage (14), inflammatory markers (15), nutritional status (16), tumor markers (17) and clinicopathological type (18) are associated with the survival and prognosis of patients with advanced NSCLC. However, individual nutritional markers of inflammation or tumor markers usually do not reflect systemic conditions. TNM stage and clinicopathological type Because of the heterogeneity of prognosis, some patients in the same pathological type and clinical stage have different outcomes. Therefore, it is essential to find a simpler and reliable biomarker with lower cost.
The establishment of relevant prediction models is crucial for prognosis evaluation, and reliable prediction models can provide important reference for the development of individualized treatment and clinical decision-making for cancer patients (19). In recent years, nomogram model has been a hot topic in the biomedical field because of its accurate prediction of certain disease outcomes and its simplicity of clinical application, and it has been rapidly popularized in clinical research of tumor-related diseases (20). In this retrospective study, we constructed the new prognostic index TMIN, which shows the potential for risk stratification and prognostic prediction in NSCLC patients. Eleven prognostic OS related indicators were screened out by LASSO regression to construct the prognostic 11 index risk model, which was identified as the independent prognostic factors for OS and PFS for the first time. To our knowledge, this study is the first to demonstrate the prognostic significance of TMIN in NSCLC patients.
It is well known that peripheral-blood studies at the time of diagnosis or before treatment may reflect the inflammatory condition within the tumor. These blood components also undergo interesting alterations in the presence of tumors, providing valuable insight into potential differences in distinguishing benign from malignant lesions. Various measures of systemic inflammatory response, such as WBC count, PLR, and LMR, have been reported in multiple studies to be associated with increased risk and progression of a variety of cancers, including NSCLC (21), gastric cancer (22), and breast cancer (23). This suggests a broader applicability of these markers in cancer diagnosis and prognosis. Nutrition is related to many aspects of tumor development and prognosis, and plays an important role throughout the treatment process (24). Malnutrition can suppress immune function and reduce resistance to disease, leading to poor clinical outcomes (25). ALB, GLB and PAB are important serum biochemical indicators that affect the nutritional status of patients, and are widely used to assess the nutritional status and disease severity of cancer patients. In many types of cancer, the cumulative effect of ALB and GLB can provide effective prognostic value for cancer patients (26). In addition, some classical tumor markers such as CEA, CA125 combined with multiple biomarkers such as SII, lymphocytes, LMR have also been included in several studies to improve the potential of diagnostic accuracy and have been shown to be effective tumor markers (27,28).
In this study, we first performed LASSO Cox regression analysis on 16 inflammatory trophic factors to screen 11 prognostic OS related indicators of NSCLC patients, including WBC, N, PLT, ALB, GLB, PAB, CEA, CYFRA21-1, FIB, PLR and LMR. The prognostic index risk model TMIN was then constructed. Furthermore, TMIN has a good diagnostic value for OS and PFS. The ROC curve showed that the AUC of TMIN in the training set and validation set was higher than that of the other combined indicators. The prognostic ROC curve showed that TMIN had a good predictive value for patients at 1, 3, and 5 years. Kaplan-Meier curves showed that OS and PFS of patients with high TMIN were significantly lower than those with low TMIN in both the training cohort and the validation cohort. Subsequently, we evaluated the prognostic power of the TMIN index for different subgroups, and it was found that TMIN showed favorable prognostic value across gender, age, and smoking history. Multivariate regression analysis showed that sex, AJCC stage, metastasis, ECOG-PS and TMIN were independent prognostic factors for OS in NSCLC patients. Interestingly, sex, tumor diameter, AJCC stage, metastasis, and TMIN were independent prognostic factors for PFS in NSCLC patients. Next, the nomogram based on TMIN was constructed according to the independent prognostic factors, which showed good diagnostic efficacy for OS and PFS of NSCLC patients. In the validation cohort, the AUC values of the OS and PFS nomograms were 0.941 and 0.955, respectively. Meanwhile, the C-index of OS in the validation cohort was 0.860. In the validation cohort, the C-index of PFS was 0.867. In addition, calibration curves at 1, 3, and 5 years showed excellent agreement between predicted OS and PFS and actually observed OS and PFS in the training and validation cohorts. More importantly, we used time-dependent AUC curve and clinical DCA to evaluate the performance of the prognostic nomogram compared with the AJCC TNM staging system, and the results showed that the accuracy of the prognostic nomogram was better than that of the AJCC TNM staging system in both the training and validation cohorts. Considering that small changes in biomarkers can lead to large changes in the diagnostic efficacy of predictive biomarkers, TMIN adds multiple factors to the prediction, which is more comprehensive and meaningful than the AJCC TNM staging system. However, there are still some limitations in this study: this is a single-center retrospective study, which may have fewer subjects and selection bias. Moreover, the prediction model was not validated in an external cohort, only in an internal cohort. Therefore, prospective, large-sample, multi-center randomized controlled trials are needed to further verify the clinical predictive value of TMIN in NSCLC.
Conclusions
This study found that TMIN is a new prognostic predictor, and TMIN has a good predictive diagnostic value for NSCLC patients. Prediction models tailor interventions to individual risk profiles and improve outcomes for NSCLC patients, enhancing the clinical decision-making process.
Acknowledgments
Funding: This study was supported by
Footnote
Reporting Checklist: The authors have completed the TRIPOD reporting checklist. Available at https://tlcr.amegroups.com/article/view/10.21037/tlcr-24-708/rc
Data Sharing Statement: Available at https://tlcr.amegroups.com/article/view/10.21037/tlcr-24-708/dss
Peer Review File: Available at https://tlcr.amegroups.com/article/view/10.21037/tlcr-24-708/prf
Conflicts of Interest: All authors have completed the ICMJE uniform disclosure form (available at https://tlcr.amegroups.com/article/view/10.21037/tlcr-24-708/coif). The authors have no conflicts of interest to declare.
Ethical Statement: The authors are accountable for all aspects of the work in ensuring that questions related to the accuracy or integrity of any part of the work are appropriately investigated and resolved. The study was conducted in accordance with the Declaration of Helsinki (as revised in 2013). The study was approved by the Ethics Committee of Qingdao Municipal Hospital (No. 2024-LW-008). All retrospective data including this study were anonymized; therefore, the requirement of informed consent for this retrospective study was waived.
Open Access Statement: This is an Open Access article distributed in accordance with the Creative Commons Attribution-NonCommercial-NoDerivs 4.0 International License (CC BY-NC-ND 4.0), which permits the non-commercial replication and distribution of the article with the strict proviso that no changes or edits are made and the original work is properly cited (including links to both the formal publication through the relevant DOI and the license). See: https://creativecommons.org/licenses/by-nc-nd/4.0/.
References
- Siegel RL, Miller KD, Wagle NS, et al. Cancer statistics, 2023. CA Cancer J Clin 2023;73:17-48. [Crossref] [PubMed]
- Roy-Chowdhuri S. Molecular Pathology of Lung Cancer. Clin Lab Med 2024;44:137-47. [Crossref] [PubMed]
- Hendriks LEL, Remon J, Faivre-Finn C, et al. Non-small-cell lung cancer. Nat Rev Dis Primers 2024;10:71. [Crossref] [PubMed]
- Klapp V, Álvarez-Abril B, Leuzzi G, et al. The DNA Damage Response and Inflammation in Cancer. Cancer Discov 2023;13:1521-45. [Crossref] [PubMed]
- Yang L, Li A, Wang Y, et al. Intratumoral microbiota: roles in cancer initiation, development and therapeutic efficacy. Signal Transduct Target Ther 2023;8:35. [Crossref] [PubMed]
- Antonucci L, Karin M. The Past and Future of Inflammation as a Target to Cancer Prevention. Cancer Prev Res (Phila) 2024;17:141-55. [Crossref] [PubMed]
- Kennel KB, Bozlar M, De Valk AF, et al. Cancer-Associated Fibroblasts in Inflammation and Antitumor Immunity. Clin Cancer Res 2023;29:1009-16. [Crossref] [PubMed]
- Nguyen CTT, Van TNK, Huong PT. Predictability of Neutrophile to Lymphocyte Ratio and Platelet to Lymphocyte Ratio on the Effectiveness of Immune Checkpoint Inhibitors in Non-small Cell Lung Cancer patients: A Meta-Analysis. Cancer Control 2024;31:10732748241285474. [Crossref] [PubMed]
- Kurt HG, Akinci Özyürek B, Erdoğan Y, et al. Association of mean platelet volume (MPV), MPV/PLATELET (PLT) ratio, and lymphocyte/monocyte ratio (LMR) as poor prognostic factor in EGFR-mutant lung adenocarcinoma treated with EGFR tyrosine kinase inhibitor. Tuberk Toraks 2024;72:197-207. [Crossref] [PubMed]
- Zhao B, Guo H, Wu W, et al. Hemoglobin, albumin, lymphocyte and platelet (HALP) score can predict the prognosis of patients with non-small cell lung cancer (NSCLC). Asian J Surg 2023;46:4891-2. [Crossref] [PubMed]
- Panaiyadiyan S, Kumar R. Prostate cancer nomograms and their application in Asian men: a review. Prostate Int 2024;12:1-9. [Crossref] [PubMed]
- Zheng P, Wang B, Luo Y, et al. Research progress on predictive models for malnutrition in cancer patients. Front Nutr 2024;11:1438941. [Crossref] [PubMed]
- Yu L, Yang R, Long Z, et al. Targeted therapy of non-small cell lung cancer: mechanisms and clinical trials. Front Oncol 2024;14:1451230. [Crossref] [PubMed]
- Fuorivia V, Attili I, Corvaja C, et al. Management of Non-Metastatic Non-Small Cell Lung Cancer (NSCLC) with Driver Gene Alterations: An Evolving Scenario. Curr Oncol 2024;31:5121-39. [Crossref] [PubMed]
- Chen T, Wang M, Chen Y, et al. Advances in predictive biomarkers associated with immunotherapy in extensive-stage small cell lung cancer. Cell Biosci 2024;14:117. [Crossref] [PubMed]
- Chen J, Xie C, Yang Y, et al. Association between albumin-to-globulin ratio and the risk of overall survival in advanced non-small cell lung cancer patients with anlotinib treatment: a retrospective cohort study. BMC Pulm Med 2023;23:275. [Crossref] [PubMed]
- Mezentsev A, Durymanov M, Makarov VA. A Comprehensive Review of Protein Biomarkers for Invasive Lung Cancer. Curr Oncol 2024;31:4818-54. [Crossref] [PubMed]
- Piórek A, Płużański A, Teterycz P, et al. Clinicopathological characteristics of patients with primary tracheal tumors: Analysis of eighty-nine cases. Thorac Cancer 2024;15:878-83. [Crossref] [PubMed]
- Maher NG, Vergara IA, Long GV, et al. Prognostic and predictive biomarkers in melanoma. Pathology 2024;56:259-73. [Crossref] [PubMed]
- Huang S, Lai H, Pan X, et al. Development and Validation of a Nomogram for Predicting Survival Based on Ferritin and Transferrin Ratio in Breast Cancer Patients. Cancer Control 2024;31:10732748241261553. [Crossref] [PubMed]
- Gao J, Nan Y, Liu G, et al. Nomogram for Predicting Efficacy and Prognosis After Chemotherapy for Advanced NSCLC. Clin Respir J 2024;18:e13815. [Crossref] [PubMed]
- Liu J, Sun R, Cai K, et al. A nomogram combining neutrophil to lymphocyte ratio (NLR) and prognostic nutritional index (PNI) to predict distant metastasis in gastric cancer. Sci Rep 2024;14:15391. [Crossref] [PubMed]
- Zhang Y, Wu J, Chen W, et al. Pretreatment System Inflammation Response Index (SIRI) is a Valuable Marker for Evaluating the Efficacy of Neoadjuvant Therapy in Breast Cancer Patients. Int J Gen Med 2024;17:4359-68. [Crossref] [PubMed]
- Ahmedah HT, Basheer HA, Almazari I, et al. Introduction to Nutrition and Cancer. Cancer Treat Res 2024;191:1-32. [Crossref] [PubMed]
- Firouzabadi D, Ahmadi H. Cancer-Related Malnutrition and the Role of Parenteral Nutrition in Cancer; A Narrative Review. Nutr Cancer 2024;76:870-84. [Crossref] [PubMed]
- Pan C, Gu Y, Ni Q. The Prognostic Value of Serum Albumin to Globulin Ratio in Patients with Breast Cancer: A Retrospective Study. Breast Cancer (Dove Med Press) 2024;16:403-11. [Crossref] [PubMed]
- Liu Q, Wang H, Chen Q, et al. Nomogram incorporating preoperative pan-immune-inflammation value and monocyte to high-density lipoprotein ratio for survival prediction in patients with colorectal cancer: a retrospective study. BMC Cancer 2024;24:740. [Crossref] [PubMed]
- Wang T, Zhang C, Gao Y, et al. Predictive value of CRP combined with peripheral blood cell ratio for the prognosis of advanced NSCLC. Am J Cancer Res 2023;13:5667-83. [PubMed]