Identification and validation of pyroptosis patterns with a novel quantification system for the prediction of prognosis in lung squamous cell carcinoma
Highlight box
Key findings
• Our study identified pyroptosis-related subtypes of lung squamous cell carcinoma (LUSC) on the basis of 51 pyroptosis-related genes (PRGs), investigated the biologic and clinical features of different subtypes.
What is known and what is new?
• Pyroptosis plays an essential role in innate immunity and antitumor effects.
• This study comprehensively examined the clinical and molecular characteristics PRGs in LUSC.
What is the implication, and what should change now?
• The quantification of pyroptosis patterns was found to be crucial in predicting prognosis and devising personalized treatment strategies in patients with LUSC.
Introduction
Lung cancer is a common malignant tumor worldwide. According to the 2020 global cancer statistics, the mortality and incidence rates of lung cancer rank first and second, respectively (1). Lung squamous cell carcinoma (LUSC), a subtype of non-small cell lung cancer (NSCLC), accounts for about 20–30% of lung cancer cases, second only to lung adenocarcinoma (2). Although immune checkpoint inhibitors (ICIs) have drastically improved the prognosis of localized LUSC (3-5), the median overall survival (OS) of patients with metastatic LUSC remains only 17.1 months even when treated with chemotherapy plus immunotherapy (6). Unfortunately, since LUSC develops insidiously, a considerable number of patients do not have the opportunity for surgery when diagnosed (7). In contrast to those with lung adenocarcinoma, patients with LUSC can rarely benefit from targeted therapies due to the low rate of mutation and high tumor heterogeneity (8). Traditional predictive approaches, including tumor pathologies and tumor staging system, cannot accurately assess the prognosis of patients with LUSC (9). Therefore, developing a practicable predictive model for the prognosis and treatment response of LUSC.
Pyroptosis is a mode of inflammatory programmed cell death. Pyroptosis, mediated by gasdermin D (GSDMD) manifests as cell swelling, cytomembrane rupture, and cellular content release, which leads to a strong inflammatory response (10,11). Pyroptosis is extensively involved in the pathophysiological process of infection, inflammatory diseases, and cancers (12). Pyroptosis exerts a dual effect on cancers. It creates an inflammatory microenvironment that can facilitate tumor development and spread. Conversely, pyroptosis can also directly suppress tumor growth, demonstrating its dual capacity in cancer dynamics (13,14). Recently, numerous studies have also found that promoting pyroptosis can inhibit tumor growth and reverse drug resistance (11,12,14). Pyroptosis plays a role in the adverse reactions related to tumor treatment. Evidence indicates that pyroptosis exhibits a dual nature in lung cancer and its treatment (15). It was reported that pyroptosis induced by p53 exerts antitumor effects in LUSC (16). Additionally, several studies have found that a pyroptosis-related signature is correlated with prognosis and immune infiltration in LUSC (17-19). Therefore, targeting the genes associated with tumor cell pyroptosis may be a promising antitumor strategy, and risk stratification of patients with LUSC based on pyroptosis patterns can perhaps contribute to precision treatment.
In this study, patients with LUSC from The Cancer Genome Atlas (TCGA) were divided into three pyroptosis clusters through the integration of pyroptosis-related genes (PRGs). Subsequently, based on the differentially expressed genes (DEGs) between the three pyroptosis clusters, two gene clusters were isolated that were associated with a distinct tumor microenvironment (TME) and clinical prognosis. Moreover, a novel scoring system was constructed to predict prognosis and immunotherapy response. Our findings may provide potential prognostic biomarkers and therapeutic targets for LUSC. We present this article in accordance with the TRIPOD reporting checklist (available at https://tlcr.amegroups.com/article/view/10.21037/tlcr-24-1003/rc).
Methods
The flowchart of the entire study is presented in Figure 1.
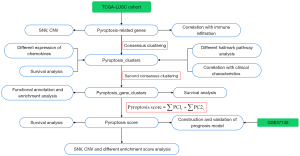
Data sources
The gene expression data, somatic mutation data, copy number variation (CNV) data, and corresponding clinical parameters of LUSC were obtained from TCGA (https://portal.gdc.cancer.gov/) database. Additionally, two datasets (GSE37745 and GSE135222) containing RNA-sequencing transcriptome information and survival data were downloaded from the Gene Expression Omnibus (GEO) (https://www.ncbi.nlm.nih.gov/geo/). A total of 51 genes involved in pyroptosis were retrieved from previous study (20) and the Molecular Signatures Database (MisgDB) database (https://www.gsea-msigdb.org/gsea/msigdb). All of these PRGs are listed in Table S1. The study was conducted in accordance with the Declaration of Helsinki (as revised in 2013).
Characteristics of the PRGs
First, we analyzed the genetic locus, somatic mutation prevalence, and CNV of PRGs. Moreover, principal component analysis (PCA) was performed on normal and tumor samples in the TCGA-LUSC dataset on the basis of the PRGs. The expression profile of 51 PRGs in normal and tumor samples was analyzed, as was the interaction relationship between the PRGs. Furthermore, the CIBERSORT algorithm was used to calculate the fractions of 22 immune infiltrating cells in each sample from the TCGA-LUSC dataset. The correlation between PRGs and immune infiltrating cells was determined and visualized in a correlation heatmap.
Unsupervised cluster and subtype analysis based on PRGs
Through the “ConsensusClusterPlus” package, consensus clustering, a method extensively used for subtype classification, was performed to sort patients into distinct PRG subtypes (21). The cumulative distribution function (CDF) curve was used to determine the best cluster number, and PCA was performed to confirm the distribution of the subtypes. To assess the clinical value of novel clustered subtypes, Kaplan-Meier survival analyses among subgroups were performed using the “survival” and “survminer” R packages (The R Foundation of Statistical Computing). Furthermore, the expression heatmap of PRGs combined with the clusters and clinicopathologic characteristics of patients, was generated via the “ggstatsplot” package.
Gene set variation analysis (GSVA)
As a nonparametric, unsupervised algorithm, GSVA is extensively used for gene enrichment based on RNA-sequencing data (22). GSVA was applied to determine the enrichment of 50 hallmark pathways at single-sample levels in TCGA-LUSC cohort.
Evaluation of the immune landscape
Single-sample gene set enrichment analysis (ssGSEA) was applied to evaluate and quantify the immune cell infiltration in each sample via the “GSVA” R package (22). Eighteen core gene signatures correlated with tumor were obtained from a previous study (23). Enrichment scores for each sample in the TCGA-LUSC dataset were calculated using the “GSVA” and “GSEABase” packages. Furthermore, 58 chemokines were obtained from the MsigDB database (https://www.gsea-msigdb.org/gsea/msigdb), 57 of which were contained in the TCGA-LUSC dataset.
Identification of pyroptosis gene clusters
The DEGs between pyroptosis clusters with |log fold change (FC)| >0.9 and P<0.05 were selected. Two pyroptosis gene clusters were obtained by consensus clustering of samples in TCGA-LUSC dataset with DEGs. To further explore the possible functions and pathways of DEGs, Gene Ontology (GO) analysis and Kyoto Encyclopedia of Genes and Genomes (KEGG) pathway enrichment analysis were conducted using the “clusterProfiler” package.
Construction of a prognostic pyroptosis score
To quantify the prognostic characteristics in LUSC, a pyroptosis score was calculated based on the DEGs between each pyroptosis cluster. First, univariate Cox regression was performed to screen DEGs correlated with prognosis. Second, PCA was conducted based on the DEGs significantly related to prognosis. The sums of principal components 1 and 2 were calculated as the pyroptosis score of each sample as follows:
where i is the expression of prognosis-related DEGs. The median value of the pyroptosis score was established as the cut-off point. Subsequently, patients were categorized into high-score (pyroptosis score > median value) and low-score (pyroptosis score < median value) groups using the “survminer” R package. TCGA-LUSC datasets were used as training sets, while GSE37745 and GSE135222 were used as validation sets. Kaplan-Meier survival analysis was applied to the training and validation cohorts, after which time-dependent receiver operating characteristic (ROC) curves were applied via the “timeROC” package.
Construction and validation of a nomogram scoring system
Univariate and multivariate Cox regression analyses were performed for clinical characteristics and pyroptosis score, the results of which were visualized in a forest map. A novel nomogram was established based on the significant variables in multivariate Cox regression analysis via the “rms” R package. Time-dependent ROC curves for 1, 2, and 3 years were plotted, and calibration plots were used to evaluate the predictive value of nomogram.
Correlation of immunotherapy response and pyroptosis score
We first compared the expressions of 62 immune checkpoints between the high- and low-score groups. Furthermore, samples from GSE135222 datasets were scored according to the pyroptosis score model and divided into high- and low-score groups. To investigate the evaluation value of pyroptosis score in immunotherapy response, survival analysis was performed. Additionally, we compared the pyroptosis score in durable clinical benefit (DCB) and no durable benefit (NDB) groups from GSE135222 datasets.
Statistical analysis
All statistical analyses were conducted using R software (version 4.0.5.). To assess correlation coefficients, we utilized Spearman’s rank correlation method. For comparisons between two groups, we applied independent sample t-tests for continuous variables that followed a normal distribution, and Mann-Whitney U tests for those that did not. When analyzing differences among three or more groups, we implemented one-way analysis of variance (ANOVA) or Kruskal-Wallis tests as appropriate. Survival analysis was performed using the Kaplan-Meier technique, with log-rank tests applied to determine the significance of observed differences. In all analyses, P<0.05 was considered statistically significant.
Results
Traits of PRGs in LUSC
A total of 51 PRGs were collected in our study. The positions of the PRGs on the chromosomes are shown in Figure 2A. Additionally, the somatic mutation prevalence of 51 PRGs in LUSC were investigated. CNV analysis indicated that there were more amplification cases than deletion cases in the PRGs (Figure 2B). The PRGs with the highest frequency of single-nucleotide variations (SNVs) was TP53 (up to 79%), followed by NLRP3 (up to 7%) (Figure 2C). Among the 51 PRGs, 42 were differentially expressed in normal versus LUSC samples (P<0.05) (Figure 2D). Moreover, PCA based on PRGs could clearly discriminate between normal and LUSC tissues (Figure 2E). To examine the association between PRGs and their prognostic value in LUSC in detail, a network diagram was generated (Figure 2F). Further analysis revealed that different PRGs were associated with different immune cell infiltration, with most PRGs being associated with macrophages, CD4 T cells, and CD8 T cells. GSDMD and the caspase family members that play important roles in pyroptosis were positively correlated with CD8 T cells and M1 macrophages (Figure 2G). The autocorrelation among 51 PRGs is displayed in Figure 2H.
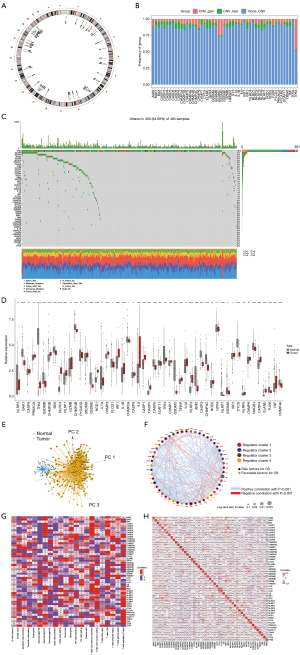
Identification of the pyroptosis cluster
To identify clusters related to pyroptosis, a consensus clustering algorithm was used. We observed that by varying the clustering variable (k) from 2 to 4, the highest intragroup correlations were obtained when k =3. Therefore, tumor samples in the TCGA-LUSC dataset were classified into three clusters based on 51 PRGs, termed “pyroptosis_cluster A”, “pyroptosis_cluster B”, and “pyroptosis_cluster C” (Figure 3A-3C). Thereafter, a heatmap was employed to visualize the relationship between clusters, clinical characteristics, and the expression of PRGs (Figure 3D). Notably, the expression of TP63 was higher in pyroptosis_cluster B than in pyroptosis_cluster A and pyroptosis_cluster C. The results of survival analysis revealed significant differences between the three pyroptosis clusters in TCGA-LUSC cohort (log-rank P=0.005). Patients with tumor samples in pyroptosis_cluster A had the lowest risk of mortality, while patients with tumor samples in pyroptosis_cluster B had the highest risk (Figure 3E). Additionally, GSVA of KEGG gene sets revealed 50 differentially enriched hallmark pathways across the three pyroptosis clusters, indicating their potential as prognostic factors (Figure 3F). We further examined the immune landscape associated with the pyroptosis patterns. The levels of immune cell infiltration were highest in pyroptosis_cluster B and included various subtypes of B cells, T cells, natural killer (NK) cells, and dendritic cells (Figure 3G). Pyroptosis_cluster B appeared to be enriched in CD8 T-effector, immune-checkpoint, and angiogenesis pathway, which are considered to be malignant pathways (23,24) (Figure 3H). Higher expressions of chemokines including CCL1, CCL4, CCL5, CCL19, CCL21, CCR1, CCR4, CCR5, CXCR3, CXCR4, CXCR8, CXCL9, CXCL13, and CXCL12 were observed in pyroptosis_cluster B than in pyroptosis_cluster A and pyroptosis_cluster C (Figure 3I). Moreover, Spearman correlation analysis revealed a strong correlation of pyroptosis cluster with gender, T stage [8th American Joint Committee on Cancer (AJCC) tumor-node-metastasis (TNM) staging system], and TCGA subtypes (secretory, primitive, classical, basal) (Figure 3J).
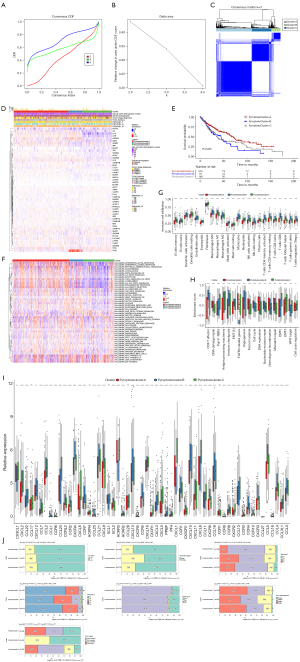
Identification of gene clusters based on pyroptosis cluster-related DEGs
To further investigate the potential biological behavior of each pyroptosis patterns, a total of 390 DEGs between the three pyroptosis clusters (Table S2) were identified after intersection analysis. Consensus clustering was leveraged to sort the samples in TCGA-LUSC into two clusters based on the 390 DEGs: pyroptosis_gene_cluster A and pyroptosis_gene_cluster B. A heatmap was constructed to visualize the complex pattern of the PRGs, indicating differential gene expressions between the two gene clusters (Figure 4A). A total of 417 pyroptosis gene cluster-related DEGs were obtained using “limma” package in R. To further investigate the functions and pathways that the 417 DEGs were involved in, GO terms and KEGG pathways were analyzed. GO analysis suggested that in biological processes, DEGs were mainly related to epidermis development, skin development, extracellular matrix organization, extracellular structure organization, and epidermal cell differentiation (Figure 4B). The most enriched cell components included collagen-containing extracellular matrix, endoplasmic reticulum lumen, apical plasma membrane, and apical part of the cell (Figure 4C). Additionally, KEGG pathway analysis revealed that DEGs were mainly enriched in metabolism xenobiotics by cytochrome P450 (Figure 4D). In terms of molecular function, the 417 DEGs were mainly enriched in extracellular matrix structural constituent, sulfur compound binding, peptidase regulator activity, carboxylic acid binding, and organic acid binding (Figure 4E). To evaluate the prognostic value of gene clusters, we performed survival analysis, which indicated that patients in pyroptosis_gene_cluster B had significantly better prognosis than did those in pyroptosis_gene_cluster A in TCGA database (P=0.005) (Figure 4F). Additionally, there were 39 PRGs differentially expressed between the two gene clusters, all of which showed higher expression in pyroptosis_gene_cluster A, except for TP63, TP53, CYCS, HMGB1, and CHMP4A (Figure 4G). With respect to immune infiltration, activated memory CD4 T cells, T regulatory cells (Tregs), activated NK cells, and neutrophils were more abundant in pyroptosis_gene_cluster A, while naïve B cells, CD8 T cells, and resting dendritic cells were markedly more abundant in pyroptosis_gene_cluster B (Figure 4H).
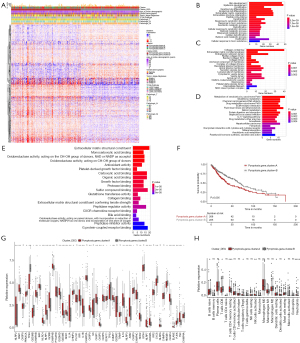
Development and validation of risk model based on pyroptosis score
To assess the risk in patients with LUSC, a prognostic model was constructed based on pyroptosis gene cluster-related prognostic DEGs in TCGA-LUSC cohort (n=493). The GSE37745 dataset (n=66) was used as a validation set. Univariate Cox regression analyses of 390 pyroptosis phenotype genes yielded 64 genes significantly correlated with prognosis (Table S3). Subsequently, PCA was applied based on the aforementioned 64 genes, and the pyroptosis score was calculated by summing the two principal components. Furthermore, patients with LUSC were divided into high- and low-pyroptosis score groups based on the median pyroptosis score. The Kaplan-Meier curves indicated that a lower pyroptosis score in TCGA cohort corresponded with better survival (P=0.002) (Figure 5A). Based on TCGA cohort, the 1-, 2-, 3-year area under the curve (AUC) of the time-dependent ROC curves for pyroptosis score was 0.573, 0.623, and 0.635, respectively (Figure 5B). Similar results were also observed in the GSE37745 dataset (P=0.005) (Figure 5C). Moreover, a Sankey diagram was plotted to visualize the pyroptosis clusters, gene clusters, pyroptosis scores, TCGA subtypes, and OS in each patient (Figure 5D). The patients with LUSC were grouped into three pyroptosis clusters and subsequently categorized into two gene clusters. Notably, patients in pyroptosis_gene_cluster A, who had unfavorable prognoses, were predominantly present in the high-pyroptosis score group. Similarly, the majority of patients in pyroptosis_gene_cluster B, who exhibited better prognoses, were categorized into the low-pyroptosis score group. Consistently, patients in pyroptosis_gene_cluster A had higher pyroptosis scores than did those in pyroptosis_gene_cluster B (P<0.001) (Figure 5E). As for the pyroptosis clusters, the pyroptosis scores in pyroptosis_cluster A were lower than those in pyroptosis_cluster B and C (P<0.001) (Figure 5F).
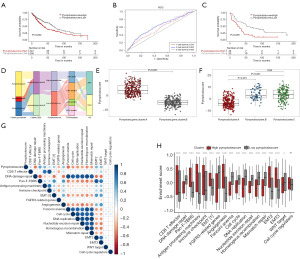
The correlation between pyroptosis score and malignant features of the tumor
To further clarify the underlying mechanism by which pyroptosis disorder affects LUSC prognosis, the correlation between pyroptosis score and gene signatures was analyzed. The results showed that the pyroptosis score was positively correlated with Pan-F-TBRS, EMT2, EMT3, antigen-processing machinery, and angiogenesis. In contrast, pyroptosis score was negatively correlated with DNA damage repair, homologous recombination, mismatch repair, cell cycle, and DNA replication (Figure 5G). The enrichment score of pathways was calculated for individuals based on GSVA. The results showed that most pathways were significantly different between the high- and low-score group, except cell cycle regulators. Concretely, higher enrichment scores of CD8 T effector, pan-F-TBRS, antigen-processing machinery, immune checkpoint, epithelial mesenchymal transition (EMT), and angiogenesis were observed in the high-pyroptosis score group. Meanwhile, pathways enriched in the low-pyroptosis score group were primarily related to reducing gene mutations (Figure 5H).
The nomogram based on pyroptosis score in LUSC
To gain an insight into the correlation between pyroptosis score and clinical features, further analyses based on TCGA-LUSC cohort were performed. We compared the pyroptosis scores in different clinical subgroups. Older adults appeared to have higher pyroptosis scores than did younger groups (Figure 6A). However, there were no differences in the subgroups of gender and TNM stage [8th edition of the Union for International Cancer Control (UICC)/AJCC TNM staging system) (Figure 6B-6F). To further investigate whether the pyroptosis score was an independent prognostic factor for LUSC, univariate and multivariate Cox regression analyses were applied in TCGA-LUSC cohort. The result of univariate Cox regression analyses showed that T3 stage [vs. T1 stage: hazard ratio (HR) =1.8; 95% confidence interval (CI): 1.15–2.82; P=0.01], T4 stage (vs. T1 stage: HR =2.32; 95% CI: 1.24–4.31; P=0.008), Mx stage (vs. M0 stage: HR =1.59; 95% CI: 1.07–2.36; P=0.02), M1 stage (vs. M0 stage: HR =3.2; 95% CI: 1.31–7.82; P=0.01), stage III (vs. stage I: HR =1.56; 95% CI: 1.09–2.23; P=0.02), stage IV (vs. stage I: HR =3.31; 95% CI: 1.34–8.18; P=0.009), and low pyroptosis score (vs. high pyroptosis score: HR =0.66; 95% CI: 0.5–0.86; P=0.003) were associated with OS (Figure 6G). In the multivariate Cox regression, low pyroptosis score was associated with a better prognosis (vs. high pyroptosis score: HR =0.65; 95% CI: 0.49–0.87; P=0.003) after adjustment for clinicopathological characteristics (Figure 6H). Furthermore, considering the strong association between risk score and the prognosis of patients with LUSC, we developed a nomogram integrating pyroptosis score, age, gender, and M stage to predict the survival rates over 1, 2, and 3 years in TCGA-LUSC cohort (Figure 6I). The pyroptosis score was found to be a more effective predictor of 1-year mortality compared with age, gender, and M stage as evidenced by the higher AUC value on the ROC curve (Figure 6J). Additionally, analysis of the calibration curves at 1, 2, and 3 years indicated good accuracy of the nomogram in the predictions of OS, with the predicted values closely matching the observed values (Figure 6K).
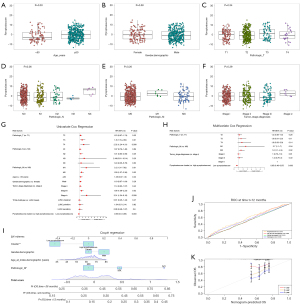
Gene variation and immunotherapy response
Cancer cells typically exhibit a high frequency of genetic mutations, ranging from gene sequence to more complex structural variants. Therefore, somatic mutations in patients with LUSC were analyzed, with particular attention paid to a comparison of the high- and low-pyroptosis score groups. The results showed significant differences in SNV mutations between the high- and low-pyroptosis score groups, with NFE2L2 and RASA1 being the top two mutated genes (Figure 7A,7B). We further analyzed the variation of 22 chromosomes in with a high and low pyroptosis score, respectively. The data showed that variation frequency of chromosomes was similar (Figure 7C). To determine whether pyroptosis score could be used to assess the effect of immunotherapy, we included an immunotherapy LUSC cohort (GSE135222). In GSE135222 dataset, survival analysis revealed that patients with lower pyroptosis scores exhibited better prognosis (log-rank test P=0.02) (Figure 7D). DCB and NDB were used to assess the efficacy of immunotherapy. However, there was no significant difference in pyroptosis score between the DCB and NDB related in the GSE135222 dataset (Figure 7E). Additionally, pyroptosis scores were applied to predict immunotherapy response, and the AUC was 0.706 (Figure 7F).
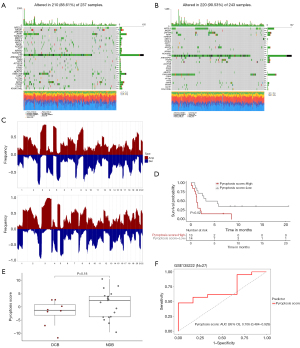
Discussion
Lung cancer is a potential fatal disease that poses a heavy burden on people worldwide. NSCLC accounts for about 85% of all lung cancer cases. Although there have been many studies LUSC, its clinical prognosis is worse compared to that of lung adenocarcinoma. Numerous studies (22-27) have shown that pyroptosis plays an essential role in innate immunity and antitumor effects. However, most studies have focused on a single PRG or single type of TME cell, and the overall effects mediated by the combination of multiple PRGs and TME infiltration characteristics have not been fully understood.
This study comprehensively examined the clinical and molecular characteristics of PRGs in LUSC by analyzing public datasets. We first analyzed the differential expression levels and gene mutation features of 51 PRGs in the TCGA-LUSC dataset and identified three pyroptosis clusters based on the consensus clustering of the 51 PRGs. OS, PRG, expression and TME characteristics showed significant differences between the three pyroptosis clusters. In comparison to pyroptosis_cluster A and pyroptosis_cluster C, pyroptosis_cluster B exhibited the highest mortality risk, coupled with the most pronounced immune infiltration. Subsequently, two distinct pyroptosis gene clusters were further identified using 390 DEGs between the three pyroptosis clusters. Patients in pyroptosis_gene_cluster B had a longer OS and higher CD8+ T-cell infiltration levels but lower Treg infiltration compared to pyroptosis_gene_cluster A. The above results indicated a more active immune-suppressive TME in pyroptosis_gene_cluster A. The immunosuppressive TME enables tumor cells to escape immune surveillance and contributes to cancer aggressiveness (28), which explains the shorter OS of patients in pyroptosis_gene_cluster A.
We next developed a pyroptosis scoring system for comprehensively quantifying the pyroptosis state of patients with LUSC, which exhibited predictive capabilities. Notably, patients categorized as low risk or high risk based on their PRG scores exhibited significant differences in OS, clinical characteristics, mutations, and TME. This result was also validated using the GSE37745 and GSE135222 dataset of LUSC samples, and ROC curve analysis further confirmed the predictive ability of the pyroptosis score. The high-pyroptosis score group showed prominent enrichment of pyroptosis_cluster B and gene_cluster A, whereas the low-pyroptosis score group showed a predominant enrichment of pyroptosis_cluster A and gene_cluster B. Patients with a high pyroptosis score exhibited poor prognosis, consistent with the results in pyroptosis_cluster B and gene_cluster A. Subsequently, a novel nomogram incorporating pyroptosis scores and clinical characteristics was constructed, which further improved the performance and facilitated the use of pyroptosis score. This prognostic model can be used for the prognostic stratification of patients with lung cancer and can provide new insights into the molecular mechanisms of lung cancer for targeted therapy.
Pyroptosis is a programmed cell death regulated by a series of unique key inflammatory caspases. GSDMD is responsible for executing pyroptosis, which is characterized by continuous cell swelling until the cell membrane ruptures, leading to the release of cellular contents and activation of a strong inflammatory response (12,29). When it was first discovered in macrophages infected with Shigella flexneri, pyroptosis was initially misidentified as apoptosis due to the technological limitations of the time (30). The term “pyroptosis” was coined in 2001 to describe this specific form of cell death (31). The activation of pyroptosis can trigger a strong inflammatory response by releasing cellular contents. Pyroptosis-related pathways are generally classified into canonical, noncanonical, and other pathways on the basis of the type of caspase present (32). The canonical pathway is mediated by caspase-1, which assembles the inflammasome, cleaves GSDMD, and releases cytokines, mainly interleukin (IL)-1β and IL-18 (11,30). The activated caspase-1 cleaves the complete GSDMD into the N-terminal (GSDMD-NT) and C-terminal domains. Subsequently, GSDMD-NT binds to acidic phospholipids on the plasma membrane and forms oligomeric death-inducing pores, increasing intracellular osmolality and thus inducing cytolysis (33,34). The noncanonical pathway is simpler and more direct, triggered by the activation of caspase-4/5 in humans and caspase-11 in mice (35). The noncanonical inflammasome sensor can directly detect intracellular bacteria and lipopolysaccharide (LPS), activate caspase-4/5/11, cleave GSDMD, and activate the inflammasome (36). Recent research has also shown that caspase-3/8 and granule proteases can induce pyroptosis (37).
There is a growing body of evidence supporting the association between pyroptosis and LUSC. GSDMD protein levels have been reported to be notably elevated in LUSC specimens in comparison to adjacent tumor specimens (38). Moreover, a positive correlation between the expression of gasdermin E (GSDME) and postoperative survival rate in patients with lung cancer has been observed, implying that higher levels of GSDME may be associated with improved patient outcomes (39). In our study, we found the most frequently mutated pyroptosis gene was TP53. By orchestrating the activity of numerous target genes with diverse biological roles, TP53, the most crucial tumor suppressor in human cancers, effectively suppresses tumor growth (40). TP53 mutations, commonly found in NSCLC, are strongly linked to smoking, with a higher prevalence observed in patients with tobacco-associated lung cancer than in never-smokers (41,42). Several studies have indicated that TP53 mutations in lung cancer are linked to heightened resistance to cancer therapies and unfavorable prognosis in terms of survival outcomes (43-45). Therefore, TP53 might play an important role in LUSC.
There are several limitations to this study that need to be addressed. First, number of cohorts and sequencing data available for analysis might have been insufficiently abundant. Second, additional independent cohort studies focused on immunotherapy are necessary to confirm the predictive reliability and consistency of pyroptosis score in terms of both prognosis and response to immunotherapy. Finally, cigarette smoke extract induces pyroptosis in human bronchial epithelial cells, however, due to limitations of the database used, we were unable to ascertain the smoking status of the patients. All in all, further experiments are required to prove that PRGs exert an effect in LUSC.
Conclusions
In conclusion, our study identified pyroptosis-related subtypes of LUSC on the basis of 51 PRGs, investigated the biologic and clinical features of different subtypes, and found evidence for a close association between pyroptosis and LUSC. In addition, the pyroptosis score, based on the subtype-specific DEGs, effectively stratified patients into high- and low-risk groups, as demonstrated by significant survival differences in both the validation and immunotherapy datasets.
Acknowledgments
The authors are grateful for the multiple databases that provided the required data for this study.
Funding: This study was supported by
Footnote
Reporting Checklist: The authors have completed the TRIPOD reporting checklist. Available at https://tlcr.amegroups.com/article/view/10.21037/tlcr-24-1003/rc
Peer Review File: Available at https://tlcr.amegroups.com/article/view/10.21037/tlcr-24-1003/prf
Conflicts of Interest: All authors have completed the ICMJE uniform disclosure form (available at https://tlcr.amegroups.com/article/view/10.21037/tlcr-24-1003/coif). The authors have no conflicts of interest to declare.
Ethical Statement: The authors are accountable for all aspects of the work in ensuring that questions related to the accuracy or integrity of any part of the work are appropriately investigated and resolved. The study was conducted in accordance with the Declaration of Helsinki (as revised in 2013).
Open Access Statement: This is an Open Access article distributed in accordance with the Creative Commons Attribution-NonCommercial-NoDerivs 4.0 International License (CC BY-NC-ND 4.0), which permits the non-commercial replication and distribution of the article with the strict proviso that no changes or edits are made and the original work is properly cited (including links to both the formal publication through the relevant DOI and the license). See: https://creativecommons.org/licenses/by-nc-nd/4.0/.
References
- Bray F, Laversanne M, Sung H, et al. Global cancer statistics 2022: GLOBOCAN estimates of incidence and mortality worldwide for 36 cancers in 185 countries. CA Cancer J Clin 2024;74:229-63. [Crossref] [PubMed]
- Barta JA, Powell CA, Wisnivesky JP. Global Epidemiology of Lung Cancer. Ann Glob Health 2019;85:8. [Crossref] [PubMed]
- Forde PM, Spicer J, Lu S, et al. Neoadjuvant Nivolumab plus Chemotherapy in Resectable Lung Cancer. N Engl J Med 2022;386:1973-85. [Crossref] [PubMed]
- Antonia SJ, Villegas A, Daniel D, et al. Overall Survival with Durvalumab after Chemoradiotherapy in Stage III NSCLC. N Engl J Med 2018;379:2342-50. [Crossref] [PubMed]
- Felip E, Altorki N, Zhou C, et al. Adjuvant atezolizumab after adjuvant chemotherapy in resected stage IB-IIIA non-small-cell lung cancer (IMpower010): a randomised, multicentre, open-label, phase 3 trial. Lancet 2021;398:1344-57. [Crossref] [PubMed]
- Paz-Ares L, Vicente D, Tafreshi A, et al. A Randomized, Placebo-Controlled Trial of Pembrolizumab Plus Chemotherapy in Patients With Metastatic Squamous NSCLC: Protocol-Specified Final Analysis of KEYNOTE-407. J Thorac Oncol 2020;15:1657-69. [Crossref] [PubMed]
- Zhou H, Zhang H, Shi M, et al. A robust signature associated with patient prognosis and tumor immune microenvironment based on immune-related genes in lung squamous cell carcinoma. Int Immunopharmacol 2020;88:106856. [Crossref] [PubMed]
- Lau SCM, Pan Y, Velcheti V, et al. Squamous cell lung cancer: Current landscape and future therapeutic options. Cancer Cell 2022;40:1279-93. [Crossref] [PubMed]
- Yang CY, Yang JC, Yang PC. Precision Management of Advanced Non-Small Cell Lung Cancer. Annu Rev Med 2020;71:117-36. [Crossref] [PubMed]
- Shi J, Zhao Y, Wang K, et al. Cleavage of GSDMD by inflammatory caspases determines pyroptotic cell death. Nature 2015;526:660-5. [Crossref] [PubMed]
- Zhou Z, He H, Wang K, et al. Granzyme A from cytotoxic lymphocytes cleaves GSDMB to trigger pyroptosis in target cells. Science 2020;368:eaaz7548. [Crossref] [PubMed]
- Wei X, Xie F, Zhou X, et al. Role of pyroptosis in inflammation and cancer. Cell Mol Immunol 2022;19:971-92. [Crossref] [PubMed]
- Jiang X, Wang J, Deng X, et al. Role of the tumor microenvironment in PD-L1/PD-1-mediated tumor immune escape. Mol Cancer 2019;18:10. [Crossref] [PubMed]
- Fang Y, Tian S, Pan Y, et al. Pyroptosis: A new frontier in cancer. Biomed Pharmacother 2020;121:109595. [Crossref] [PubMed]
- Liang X, Qin Y, Wu D, et al. Pyroptosis: a double-edged sword in lung cancer and other respiratory diseases. Cell Commun Signal 2024;22:40. [Crossref] [PubMed]
- Zhang T, Li Y, Zhu R, et al. Transcription Factor p53 Suppresses Tumor Growth by Prompting Pyroptosis in Non-Small-Cell Lung Cancer. Oxid Med Cell Longev 2019;2019:8746895. [Crossref] [PubMed]
- Deng X, Wang Z, Luo Y, et al. Prediction of lung squamous cell carcinoma immune microenvironment and immunotherapy efficiency with pyroptosis-derived genes. Medicine (Baltimore) 2022;101:e30304. [Crossref] [PubMed]
- Li T, Liu H, Dong C, et al. Prognostic Implications of Pyroptosis-Related Gene Signatures in Lung Squamous Cell Carcinoma. Front Pharmacol 2022;13:806995. [Crossref] [PubMed]
- Pezzuto A, Cappuzzo F, D'Arcangelo M, et al. Prognostic Value of p16 Protein in Patients With Surgically Treated Non-small Cell Lung Cancer; Relationship With Ki-67 and PD-L1. Anticancer Res 2020;40:983-90. [Crossref] [PubMed]
- Pezzuto A, D'Ascanio M, Ricci A, et al. Expression and role of p16 and GLUT1 in malignant diseases and lung cancer: A review. Thorac Cancer 2020;11:3060-70. [Crossref] [PubMed]
- Luo P, Jiang Y, Ding S, et al. Integrative Analysis of Pyroptosis-Related Prognostic Signature and Immunological Infiltration in Lung Squamous Cell Carcinoma. Biomed Res Int 2022;2022:4944758. [Crossref] [PubMed]
- Ye Y, Dai Q, Qi H. A novel defined pyroptosis-related gene signature for predicting the prognosis of ovarian cancer. Cell Death Discov 2021;7:71. [Crossref] [PubMed]
- Wilkerson MD, Hayes DN. ConsensusClusterPlus: a class discovery tool with confidence assessments and item tracking. Bioinformatics 2010;26:1572-3. [Crossref] [PubMed]
- Hänzelmann S, Castelo R, Guinney J. GSVA: gene set variation analysis for microarray and RNA-seq data. BMC Bioinformatics 2013;14:7. [Crossref] [PubMed]
- Mariathasan S, Turley SJ, Nickles D, et al. TGFβ attenuates tumour response to PD-L1 blockade by contributing to exclusion of T cells. Nature 2018;554:544-8. [Crossref] [PubMed]
- Raskov H, Orhan A, Christensen JP, et al. Cytotoxic CD8(+) T cells in cancer and cancer immunotherapy. Br J Cancer 2021;124:359-67. [Crossref] [PubMed]
- Viallard C, Larrivée B. Tumor angiogenesis and vascular normalization: alternative therapeutic targets. Angiogenesis 2017;20:409-26. [Crossref] [PubMed]
- Lei X, Lei Y, Li JK, et al. Immune cells within the tumor microenvironment: Biological functions and roles in cancer immunotherapy. Cancer Lett 2020;470:126-33. [Crossref] [PubMed]
- Shi J, Gao W, Shao F. Pyroptosis: Gasdermin-Mediated Programmed Necrotic Cell Death. Trends Biochem Sci 2017;42:245-54. [Crossref] [PubMed]
- Zychlinsky A, Prevost MC, Sansonetti PJ. Shigella flexneri induces apoptosis in infected macrophages. Nature 1992;358:167-9. [Crossref] [PubMed]
- Cookson BT, Brennan MA. Pro-inflammatory programmed cell death. Trends Microbiol 2001;9:113-4. [Crossref] [PubMed]
- Yu P, Zhang X, Liu N, et al. Pyroptosis: mechanisms and diseases. Signal Transduct Target Ther 2021;6:128. [Crossref] [PubMed]
- Frank D, Vince JE. Pyroptosis versus necroptosis: similarities, differences, and crosstalk. Cell Death Differ 2019;26:99-114. [Crossref] [PubMed]
- Liu X, Zhang Z, Ruan J, et al. Inflammasome-activated gasdermin D causes pyroptosis by forming membrane pores. Nature 2016;535:153-8. [Crossref] [PubMed]
- Sborgi L, Rühl S, Mulvihill E, et al. GSDMD membrane pore formation constitutes the mechanism of pyroptotic cell death. EMBO J 2016;35:1766-78. [Crossref] [PubMed]
- An S, Hu H, Li Y, et al. Pyroptosis Plays a Role in Osteoarthritis. Aging Dis 2020;11:1146-57. [Crossref] [PubMed]
- Wang H, Zhou X, Li C, et al. The emerging role of pyroptosis in pediatric cancers: from mechanism to therapy. J Hematol Oncol 2022;15:140. [Crossref] [PubMed]
- Gao J, Qiu X, Xi G, et al. Downregulation of GSDMD attenuates tumor proliferation via the intrinsic mitochondrial apoptotic pathway and inhibition of EGFR/Akt signaling and predicts a good prognosis in non small cell lung cancer. Oncol Rep 2018;40:1971-84. [Crossref] [PubMed]
- Huang YL, Zhang GH, Zhu Q, et al. Expression levels of caspase-3 and gasdermin E and their involvement in the occurrence and prognosis of lung cancer. Cancer Rep (Hoboken) 2022;5:e1561. [Crossref] [PubMed]
- Hu J, Cao J, Topatana W, et al. Targeting mutant p53 for cancer therapy: direct and indirect strategies. J Hematol Oncol 2021;14:157. [Crossref] [PubMed]
- Vähäkangas KH, Bennett WP, Castrén K, et al. p53 and K-ras mutations in lung cancers from former and never-smoking women. Cancer Res 2001;61:4350-6.
- Le Calvez F, Mukeria A, Hunt JD, et al. TP53 and KRAS mutation load and types in lung cancers in relation to tobacco smoke: distinct patterns in never, former, and current smokers. Cancer Res 2005;65:5076-83. [Crossref] [PubMed]
- Viktorsson K, De Petris L, Lewensohn R. The role of p53 in treatment responses of lung cancer. Biochem Biophys Res Commun 2005;331:868-80. [Crossref] [PubMed]
- Ma X, Le Teuff G, Lacas B, et al. Prognostic and Predictive Effect of TP53 Mutations in Patients with Non-Small Cell Lung Cancer from Adjuvant Cisplatin-Based Therapy Randomized Trials: A LACE-Bio Pooled Analysis. J Thorac Oncol 2016;11:850-61. [Crossref] [PubMed]
- Gu J, Zhou Y, Huang L, et al. TP53 mutation is associated with a poor clinical outcome for non-small cell lung cancer: Evidence from a meta-analysis. Mol Clin Oncol 2016;5:705-13. [Crossref] [PubMed]
(English Language Editor: J. Gray)