Radiomicsmetabolic signature profiles for advanced non-small cell lung cancer with chemoimmunotherapy by reflecting biological function and survival
Highlight box
Key findings
• Positron emission tomography (PET)/computed tomography (CT) could reveal survival and tumor heterogeneity in advanced non-small cell lung cancer (NSCLC) patients undergoing chemoimmunotherapy. For patients with similar total metabolic tumor volume (tMTV) (≤105), patients with smaller lesions seemed to have better long-term prognoses than those with larger lesions, even of fewer in number.
What is known and what is new?
• Prior investigations have effectively delineated the probable correlation between PET/CT radiomics or metabolic parameters and immune-monotherapy response in NSCLC.
• For advanced NSCLC receiving chemoimmunotherapy, the Radiomicsmetabolicos model encompassing radiomics score (Rad-score) >0.705, squamous cell subtype, liver metastases, bone marrow to liver ratio >0.94, and tMTV >105 exhibited reliable prognostic capacity. Patients with Rad-score ≤0.705 demonstrated upregulation of immune-related pathways and favorable survival. Distant metastases metabolic tumor volume (MTV) and total lesion glycolysis, as well as intrathoracic lymph nodes MTV were associated with survival independently. For patients with similar tMTV (≤105), those with smaller lesions seemed to have better long-term prognoses than those with larger lesions, even of fewer in number.
What is the implication, and what should change now?
• Our findings proved that PET/CT could reveal survival and tumor heterogeneity in advanced NSCLC patients undergoing chemoimmunotherapy, which might guide the selection of immune-monotherapy for low-risk patients and facilitate the advancement of precision treatment.
Introduction
The crucial discovery of immune checkpoint inhibitors (ICIs) has made immunotherapies widely applied in the routine management of non-small cell lung cancer (NSCLC) (1,2). The combination strategies for immunotherapy, such as chemoimmunotherapy, have remarkably diversified therapy options and prolonged overall survival (OS) in various clinical trials. Although programmed death-ligand 1 (PD-L1) tumor proportion score (TPS) has been approved as the predictive biomarker for immunotherapy, it is only modestly helpful in identifying potentially favorable patients receiving chemoimmunotherapy (1), which highlights the significance of discerning efficacious predictive tools.
Compared to computed tomography (CT), 18F-fluorodeoxyglucose (FDG) positron emission tomography (PET)/CT serves as a more compelling option offering pivotal metabolic alongside multimodal data. Prior investigations have effectively delineated the probable correlation between PET/CT radiomics (3) or metabolic parameters and immunotherapy response in NSCLC. It has been suggested that metabolic parameters (4-10) including total metabolic tumor volume (tMTV), total lesion glycolysis (TLG), and inflammatory parameters (11) such as bone marrow to liver ratio (BLR) or spleen to liver ratio (SLR) are putative predictive or prognostic indicators. Nevertheless, few explorations have addressed the usage of PET/CT for advanced NSCLC patients undergoing chemoimmunotherapy. Moreover, mounting evidence has suggested a potential linkage between imaging subtype and transcriptomic gene expression patterns (12-14). However, no existing studies have integrated PET/CT radiomics signatures with biological processes and tumor microenvironment (TME) for chemoimmunotherapy.
Herein, we constructed and validated a multimodal PET/CT-based radiomicsmetabolic signature to recognize the favorable advanced NSCLC patients for receiving chemoimmunotherapy. An in-depth analysis of gene expression patterns and TME verified our hypothesis that radiomics features with robust prognostic information were correlated with underlying biological functions. Additionally, we comprehensively analyzed metabolic burden and the interplay and combined impact of PD-L1 and metabolic parameters. Meanwhile, we innovatively probed into the prognostic disparities among patients with similar tMTV but different tumor distributions (lesion locations and numbers). We present this article in accordance with the STROBE reporting checklist (available at https://tlcr.amegroups.com/article/view/10.21037/tlcr-24-576/rc).
Methods
Study design
The study’s overview is presented in Figure 1. NSCLC patients receiving PET/CT examinations were retrospectively collected from two cohorts. Cohort A recruited unresectable stage IIIB–IV NSCLC patients receiving immunotherapy and pretreatment whole-body 18F-FDG PET/CT examination in Jinling Hospital from August 2018 to January 2023. Eligible patients were required to meet the following criteria: (I) histologically or cytologically confirmed unresectable stage IIIB–IV NSCLC; (II) less-than-1-month delay from 18F-FDG PET/CT examination to the initiation of immunotherapy; (III) without immunotherapy history; and (IV) ineligible for surgical resection after treatment. Those lost to follow-up within 1 month or with missing original images were excluded from the analysis. The last follow-up time was in July 2023. A public cohort (Cohort B) with early-stage NSCLC patients receiving preoperative 18F-FDG PET/CT scanning and RNA sequencing test was downloaded from The Cancer Imaging Archive (TCIA) dataset (15,16), which was used to validate and elucidate the underlying molecular pathways and TME of radiomics models. The study was conducted in accordance with the Declaration of Helsinki (as revised in 2013) and was approved by the Institutional Review Board of Jinling Hospital (No. 2024DZKY-049-01). Institutional Review Board waived the requirement for informed consent considering the retrospective design of this study.
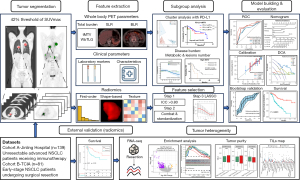
Whole-body PET-based and PET/CT radiomics feature extraction
Each patient was instructed to fast for at least 6 hours before the examination, and the blood glucose level was less than 8 mol/mL at the time of 18F-FDG injection (range, 3.7–6.6 MBq/kg). Three different PET/CT scanners were used in Cohort A (Appendix 1). All FDG-avid hypermetabolic tumor lesions on PET images were analyzed, with lesion segmentation and feature extraction performed using LIFEx 7.4.0 software (https://www.lifexsoft.org/) (17). Regions of interest (ROIs) in three-dimensional (3D) of each lesion were semiautomatically segmented with a threshold of 42% of the maximum standardized uptake value (SUVmax) (18) and were further reviewed by an experienced nuclear physician blinded to the clinical response. ROIs on CT were obtained by copying the ROIs of PET to CT if the two series matched well. Otherwise, the ROIs on CT were manually contoured layer by layer by the nuclear physician. Then, ROIs delineated from primary lesions containing more than 64 voxels were reserved for further radiomics analysis (17). Each ROI on PET and CT images were respectively resampled into 3×3×3 and 1×1×1 mm3. All the computed radiomics features were compliant with image biomarker standardization initiative (IBSI) guidelines (19). A total of 109 PET-based and 82 CT-based radiomics features were extracted (Table S1).
PET-based inflammatory parameters, BLR and SLR, were calculated by dividing the bone marrow or spleen SUVmax by the liver SUVmax. Liver, spleen, and bone marrow SUVmax were acquired by contouring spherical ROIs with a diameter of 3, 2, and 1.5 cm respectively (20,21). Particularly, bone marrow SUVmax was calculated as the average SUVmax of four ROIs centered in lumbar 1–4 vertebral bodies. PET-based metabolic parameters were measured for each lesion, including SUVmax, mean standardized uptake value (SUVmean), metabolic tumor volume (MTV), and TLG. tMTV or whole-body TLG (wbTLG) was defined as the sum of MTV or TLG of all tumor lesions. Lesions were further divided into three categories according to the American Joint Committee on Cancer criteria TNM staging system (8th edition), including bilateral lung lesions, intrathoracic lymph nodes (N1, N2, N3), and distant metastases (except for lung metastases). The metabolic burden (MTV and TLG) of the above-mentioned categories was defined as the sum of the MTV or TLG of the lesions in each classification. The number of FDG-avid lesions was counted based on PET images (22). For the lesions closely located and inseparable macroscopically on PET images, such as central lung cancer, mediastinal or hilar lymph nodes lesions, a panel of experts including two nuclear physicians and one respiratory physician made joint confirmation by further checking the highest SUV (SUVmax) point within the supposed contour automatically selected by LIFEx software, and referring to its anatomical location via integrated PET/CT. Lesions too closely adherent to split would be counted as one. Additionally, the optimal cut-offs of PET/CT-based parameters were determined by the maximum Youden index discriminating the durable clinical benefit (DCB) and non-durable clinical benefit (NDB).
Clinical feature extraction
Baseline characteristics and peripheral blood inflammatory parameters before the immunotherapy were collected from electronic medical records. Baseline information was collected including age, gender, smoking status, histology (adenocarcinoma, squamous cell carcinoma), stage, line of immunotherapy, combination regimen, distant metastatic site, and PD-L1 expression. Laboratory inflammatory parameters contained derived neutrophil-to-lymphocyte ratio (dNLR), platelet-to-lymphocyte ratio (PLR), and systemic immune inflammation index (SII). Cut-offs were determined based on published methods (23-25), with three for dNLR, 150 for PLR, and 1,270 for SII (details in Appendix 2).
Follow-up and response assessment
Clinical response is defined as complete response (CR), partial response (PR), stable disease (SD), or progressive disease (PD) according to the modified Response Evaluation Criteria in Solid Tumors (RECIST) version 1.1 for immune-based therapeutics (26). Progression-free survival (PFS) and OS were defined as the time from the start of immunotherapy until progression and death. Patients with PFS >6 months were defined as DCB, and the others were regarded as NDB (3).
Feature selection
PET/CT radiomics features were selected based on a three-step method. First, a twenty-two-patient cohort was randomly selected and the features with intraclass correlation coefficient (ICC) >0.80 (27) were reserved for analysis. Secondly, we harmonized radiomics features, SUVmax, and SUVmean using the ComBat approach (28) as previously validated to correct for multi-scanner and multi-protocol effects. All radiomics features were standardized by the Z-score method, and features with P<0.1 by Cox regression analysis entered further feature selection. Finally, we identified the most predictive radiomics features using the least absolute shrinkage and selection operator (LASSO) with fivefold cross-validation (29). The radiomics score (Rad-score) was calculated based on a linear combination of selected features that were weighted by respective coefficients in the LASSO Cox model. Subsequently, the cohort was separated into high and low Rad-score groups with the cut-offs chosen by X-tile software (30). Specifically, the computation procedure of the Rad-score is as follows:
Model building, evaluation, and external validation
Three different models were separately established for PFS and OS. PET/CT radiomics models encompassed dichotomous Rad-score for PFS and OS, which were named Radiomics and Radiomicos. PET-based metabolic models were referred to as Metabolic (PFS) and Metabolicos (OS), including selected clinical and PET metabolic parameters (e.g., tMTV, wbTLG, BLR, or SLR). The combined models were established by integrating the parameters in the above-mentioned radiomics and metabolic models [Radiomicsmetabolic (PFS)/Radiomicsmetabolicos (OS)]. For the construction of metabolic models, parameters with P<0.05 by univariate analysis were reserved and entered multivariate Cox regression model using a backward step-wise selection with Akaike information criterion as the stopping rule. Nomograms and Kaplan-Meier survival curves based on the risk-scoring systems were plotted with cut-offs set by X-tile software. Model comparison was conducted by Delong test. Model performances were evaluated using time-dependent ROC (receiver operating characteristic) curves, decision curve analysis (DCA), and calibration curves. Internal validation was performed by 1,000 times bootstrap resampling (31). The public cohort with early-stage NSCLC patients from TCIA served as external validation.
Gene set enrichment analysis (GSEA) of different Rad-score groups and identification of differentially expressed genes (DEGs)
RNA-sequencing data from Cohort B were downloaded from Gene Expression Omnibus (GEO) under accession number GSE103584 (15). To elucidate the biological function of radiomics features, we conducted a pre-ranked GSEA between high and low Rad-score subgroups. Kyoto Encyclopedia of Genes and Genomes (KEGG) pathways and gene ontology (GO) functions were downloaded from the GSEA website (http://www.gsea-msigdb.org/gsea/downloads.jsp). False discovery rate (FDR) <0.1 and P<0.05 (32) were regarded as statistically significant. DEGs between the high and low Rad-score groups were identified using the ‘DESeq’ R package, with an absolute log2(fold change) >2 and adjusted P<0.05 set as the cut-off criteria.
Tumor purity and immune infiltration analysis between Rad-score subgroups
To validate the hypothesis that our Rad-score grouping could reflect the tumor heterogeneity, we used “Estimation of Stromal and Immune cells in malignant tumors using Expression data” (ESTIMATE) method (33) to infer tumor purity. Further, the infiltration profiling of immune cells was estimated via Timer2.0 (http://timer.cistrome.org/).
Clustering analysis
To reduce features’ redundancy, unsupervised consensus clustering was used for assignment based on similar radiomics feature patterns (34). Radiomics features with P<0.1 by the Cox regression analysis entered the cluster analyses. To identify the interaction between PD-L1 and metabolic features, unsupervised K-means clustering was performed with the number of clusters determined by the silhouette coefficient.
Statistical analyses
Descriptive statistics of continuous parameters with or without normal distribution were given as the mean ± standard deviation or median (interquartile). Wilcoxon signed-rank test and Fisher’s exact test were used respectively for continuous and categorical variables. Predictors of survival were assessed using Cox proportional hazard (PH) models and log-rank tests. Variables incompliant with the PHs assumption were analyzed using the time-dependent Cox Regression Model and landmark analysis. A two-sided P less than 0.05 was regarded as significant. Statistical analyses were performed with R 4.3.0 and GraphPad Prism 9.3.1.
Results
Characteristics
Among the 1,089 patients initially, a sum of 220 patients met the inclusion criteria, including 139 unresectable advanced NSCLC patients receiving immunotherapy from Jinling Hospital (Cohort A) as the training cohort (Figure S1) and 81 early-stage NSCLC patients from TCIA (Cohort B) as the validation. Specifically, in Cohort A, 56 (40.3%) and 83 (59.7%) patients were with unresectable stage III and metastatic respectively. Among 56 stage III NSCLC patients, only 18 (32.1%) patients received concurrent or sequential chemo-radiotherapy followed by consolidation immunotherapy, while the rest underwent first-line chemoimmunotherapy. During the median follow-up period of 15.32 (interquartile, 8.66–25.03) months, 93 (66.9%) and 55 (39.6%) patients experienced disease progression and death. Objective response and DCB were respectively observed in 46 (33.1%) and 95 (68.3%) patients and the disease control rate reached 92.8%. No patients experienced CR, while 46 (33.1%), 85 (61.1%) and 8 (5.8%) patients were severally defined as PR, SD and PD for best response. The majority were treatment-naïve without prior treatment history (92.8%) and 117 (84.2%) patients received chemoimmunotherapy (Table 1).
Table 1
Characteristics | Data |
---|---|
Clinical parameters | |
Age ≥65 years | 79 (56.8) |
Gender (male/female) | 116 (83.5)/23 (16.5) |
Smoking status (current or ex/non-smoker) | 97 (69.8)/42 (30.2) |
Histology (adenocarcinoma/squamous cell) | 67 (48.2)/72 (51.8) |
Stage (III/IV) | 56 (40.3)/83 (59.7) |
Immunotherapy line (first/second or posterior) | 129 (92.8)/10 (7.2) |
Combination regimen (chemo/anti-angiogenesis/none) | 117 (84.2)/12 (8.6)/10 (7.2) |
Number of FDG-avid tumor lesions | 6 [3–11] |
Distant metastatic site (brain/liver/bone) | 11 (7.9)/13 (9.4)/38 (27.3) |
PD-L1 (<1%/1–49%/≥50%/unknown) | 18 (12.9)/17 (12.2)/8 (5.8)/96 (69.1) |
Best response (CR/PR/SD/PD) | 0 (0.0)/46 (33.1)/85 (61.1)/8 (5.8) |
Quantitative 18F-FDG PET/CT parameters | |
Total metabolic tumor volume (mL) | 48 [75] |
wbTLG (g) | 314 [603] |
SLR | 0.82 [0.17] |
BLR | 0.77 [0.31] |
Lung lesions metabolic tumor volume (mL) | 20 [36] |
Lung lesions TLG (g) | 164 [306] |
Intrathoracic lymph nodes metabolic tumor volume (mL) | 11 [17] |
Intrathoracic lymph nodes TLG (g) | 43 [110] |
Distant metastases metabolic tumor volume (mL) | 0 [13] |
Distant metastases TLG (g) | 0 [60] |
Primary lesion SUVmax (g/mL) | 13.17 [7.32] |
Primary lesion SUVmean (g/mL) | 7.97 [4.44] |
Primary lesion metabolic tumor volume (mL) | 15.97 [26.01] |
Primary lesion TLG (g) | 134.61 [280.31] |
Laboratory inflammatory parameters | |
SII | 749.68 [807.94] |
dNLR | 2.06 [1.18] |
PLR | 174.03 [114.70] |
Data are presented as n (%), median [interquartile], or mean [standard deviation]. FDG, fluorodeoxyglucose; PD-L1, programmed death-ligand 1; CR, complete response; PR, partial response; SD, stable disease; PD, progressive disease; PET, positron emission tomography; CT, computed tomography; wbTLG, whole-body total lesion glycolysis; SLR, spleen to liver ratio; BLR, bone marrow to liver ratio; TLG, total lesion glycolysis; SUVmax, maximum standardized uptake value; SUVmean, mean standardized uptake value; SII, systemic immune inflammation index; dNLR, derived neutrophil-to-lymphocyte ratio; PLR, platelet-to-lymphocyte ratio.
Construction and evaluation of the PET/CT radiomics, metabolic, and radiomics metabolic models
In Cohort A, a total of 130 patients with ROIs containing more than 64 voxels were reserved for radiomics analysis. Ten radiomics features were selected and contributed to the construction of the Rad-score in the Radiomics model for PFS (Figure 2A,2B). It was shown that patients with a Rad-score >0.093 had a significantly shorter PFS compared to those with a Rad-score ≤0.093 (P<0.001) (Figure 2C). Additionally, consensus clustering analysis based on the 26 PFS-related radiomics features with P<0.1 assigned patients into two clusters (Table S2, Figure 2D). Kaplan-Meier survival curves for the two clusters were intersected (P=0.02), leading to the selection of a 6-month time point as a landmark. Subsequent analysis revealed that at 6–40 months’ follow-up, cluster 1 exhibited inferior PFS compared to cluster 2 (P<0.001) (Figure 2E,2F). In the Metabolic model for PFS, four variables were retained through multivariate stepwise Cox regression. Patients with squamous cell subtype [hazard ratio (HR) =1.63; P=0.03], liver metastases (HR =2.18; P=0.01), BLR >0.94 (HR =2.01; P=0.003), and wbTLG >219 g (HR =2.00; P=0.002) tended to experience worse PFS outcomes (Table S3, Figure 2G).
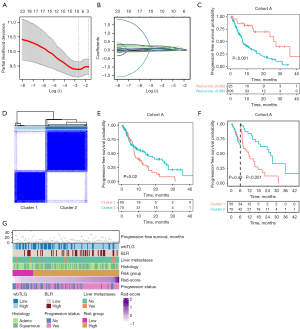
For the prediction for OS, three radiomics features constituted the Rad-score in the Radiomics model (Figure 3A,3B). Patients in the low Rad-score group exhibited more favorable OS compared to their counterparts (P=0.009) (Figure 3C). It was further validated in the external validation Cohort B, of which the landmark survival analysis revealed that the OS of the Rad-score ≤0.705 group was significantly higher than that of the Rad-score >0.705 group at 6–120 months’ follow-up (P=0.02) (Figure 3D,3E). Additionally, we tested the cut-off value of the Rad-Score using the “survminer” R package with the OS as the endpoint. The Kaplan-Meier survival curves were intersected (P=0.07); further landmark survival analysis revealed that the OS of the Rad-score ≤−0.111 group was significantly higher than that of the Rad-score>-0.111 group at 6–120 months’ follow-up (P=0.03) (Figure S2). In the Metabolicos model for OS, retained variables included squamous cell subtype (HR =1.75; P=0.046), liver metastases (HR =2.81; P=0.007), BLR >0.94 (HR =1.87; P=0.04), and tMTV >105 mL (HR =2.16; P=0.01) (Table S3, Figure 3F).
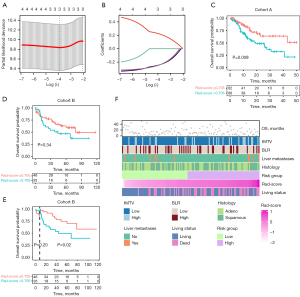
Moreover, we evaluated the predictive capacity of the Radiomicsmetabolic model for PFS, which encompassed squamous cell subtype [HR =1.596; 95% confidence interval (CI): 1.013–2.512], liver metastases (HR =3.060; 95% CI: 1.538–6.087), BLR >0.94 (HR =1.711; 95% CI: 1.061–2.761), wbTLG >219 g (HR =2.055; 95% CI: 1.280–3.299) and Rad-score for PFS >0.093 (HR =3.237; 95% CI: 1.589–6.594) (Table 2, Figure 4A). It exhibited reliable predictive capacity and was proven well-performed through bootstrap ROCs (Figure 4B,4C). Compared to the Radiomics (P<0.001) or Metabolic (P=0.03) model, the Radiomicsmetabolic outperformed distinctly with a 1-year area under the curve (AUC) (95% CI) of 0.814 (0.729–0.898) (Figure 4D, Table S4). The reliability was further bolstered by DCA and calibration curves (Figure 4E,4F). Furthermore, BLR >0.94 (P=0.02), wbTLG >219 g (P=0.002), and liver metastases (P=0.002) remained as independent prognostic factors for PFS after adjusting for confounders (Table S5). Analogously, Radiomicsmetabolicos model for OS included squamous cell subtype (HR =1.604; 95% CI: 0.897–2.868), liver metastases (HR =3.405; 95% CI: 1.466–7.908), BLR >0.94 (HR =1.781; 95% CI: 0.970–3.270), tMTV >105 mL (HR =2.070; 95% CI: 1.094–3.916) and Rad-score for OS >0.705 (HR =2.453; 95% CI: 1.324–4.544) (Figure 4G, Table 2). It was validated by bootstrap analysis, with a mean AUC of 0.649 (Figure 4H,4I). DeLong test, time-dependent ROC curves, DCA curves as well as calibration curves revealed the superior performance of the Radiomicsmetabolicos model with a notable 3-year AUC of 0.837 (95% CI: 0.735–0.939) (Figure 4J-4L, Table S6). Further analysis confirmed that BLR >0.94 (P=0.045), tMTV >105 mL (P=0.02) and liver metastases (P=0.006) remained independent prognostic factors after adjusting for confounders (Table S5).
Table 2
Parameters | Radiomicsmetabolic | Radiomicsmetabolicos | |||
---|---|---|---|---|---|
HR (95% CI) | P value | HR (95% CI) | P value | ||
Histology (squamous cell) | 1.596 (1.013–2.512) | 0.04* | 1.604 (0.897–2.868) | 0.11 | |
Liver metastases | 3.060 (1.538–6.087) | 0.001* | 3.405 (1.466–7.908) | 0.004* | |
BLR (>0.94) | 1.711 (1.061–2.761) | 0.03* | 1.781 (0.970–3.270) | 0.06 | |
wbTLG (>219 g) | 2.055 (1.280–3.299) | 0.003 | |||
tMTV (>105 mL) | 2.070 (1.094–3.916) | 0.03* | |||
Rad-score for PFS (>0.093) | 3.237 (1.589–6.594) | 0.001* | |||
Rad-score for OS (>0.705) | 2.453 (1.324–4.544) | 0.004* |
*, P<0.05. HR, hazard ratio; CI, confidence interval; BLR, bone marrow to liver ratio; wbTLG, whole-body total lesion glycolysis; tMTV, total metabolic tumor volume; Rad-score, radiomics score; PFS, progression-free survival; OS, overall survival.
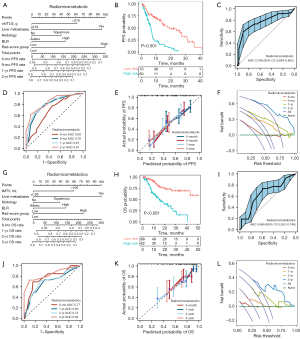
Correlation between the PET/CT parameters and PD-L1 or gene patterns
Gene patterns and immune infiltration analysis between two Rad-score groups
Patients in Cohort B were divided into high and low Rad-score groups for OS and PFS based on the same cut-offs of Cohort A. A total of 60 DEGs were identified for OS (Figure 5A, Table S7). GSEA analysis revealed the upregulation of immune-related pathways in the Rad-score ≤0.705 group (Figure 5B,5C). Furthermore, the ESTIMATE method found no significant difference in tumor purity between the two Rad-score groups (Figure 5D). However, immune infiltration analysis revealed a lower proportion of M2-type macrophages and a higher proportion of T regulatory in the low Rad-score group by Cibersort, with no disparities observed by any other methodologies (Figure 5E, Table S8). Regarding PFS, a total of 186 DEGs were identified, while only two immune-related pathways were significantly enriched in the low Rad-score group with favorable survival (Figure 6A,6B, Table S9). Estimation of immune cell infiltration demonstrated no significant disparities by Cibersort, but higher levels of B memory cells and CD4+ effector memory T cells were detected in the low Rad-score group via xCell method (Figure 6C, Table S8). No significant differences in tumor purity were observed between the two Rad-score groups (Figure 6D).
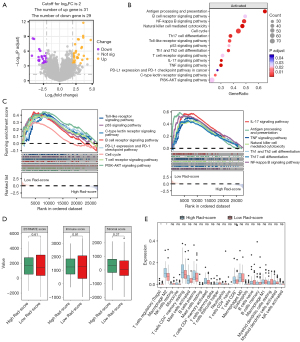
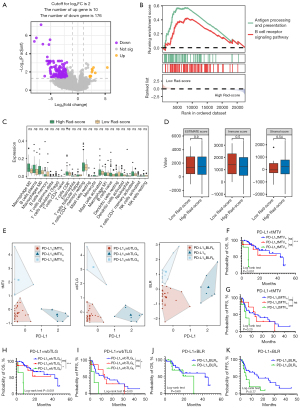
Clustering analysis according to metabolic parameters and PD-L1 expression
The log-rank test indicated that PD-L1 TPS was not associated with PFS (P=0.79) and OS (P=0.82) (Figure S3). According to unsupervised K-means clustering analysis, patients were assigned into three distinct clusters based on PD-L1 expression and metrics including tMTV, wbTLG, or BLR (Figure 6E). It was indicated that patients in PD-L1lotMTVhi and PD-L1lowbTLGhi groups acquired unfavorable OS compared to those in PD-L1lotMTVlo and PD-L1lowbTLGlo groups respectively, which was further validated by multivariate analysis (P=0.001; P<0.001). However, no distinct differences in OS and PFS were observed between PD-L1loBLRlo and PD-L1loBLRhi groups (P=0.60; P=0.77) (Figure 6F-6K, Table S10).
Prognostic disparities among patients with similar tMTV but different tumor distributions
Subgroup analysis according to different lesion locations in metastatic NSCLC
We further analyzed the correlation between the location-specific metabolic burden and survival. It was indicated that distant metastases’ MTV as well as TLG remained as independent risk factors for OS after adjusting for confounders [HR (95% CI): 2.62 (1.06–6.44); P=0.04; HR (95% CI): 2.28 (1.01–5.18); P=0.048]. Moreover, only MTV of intrathoracic lymph nodes impaired PFS independently [HR (95% CI): 2.31 (1.23–4.33); P=0.009] (Table S11).
The combined effect of total metabolic burden and the number of FDG-avid tumor lesions on survival
In the low-tMTV group (≤105 mL), Cox regression analysis between the number of lesions and OS unsatisfied with the PH assumption, therefore, a time-dependent Cox regression model was established with a time point of 12 months selected (Figure 7A). Multivariate analysis indicated that the baseline number of FDG-avid lesions was not associated with less-than-1-year OS [HR (95% CI): 1.01 (0.93–1.09); P=0.83], but was an independent protective factor for more-than-1-year OS [HR (95% CI): 0.79 (0.65–0.95); P=0.02] (Figure 7B,7C, Table S12). Furthermore, it was revealed that the number of FDG-avid lesions did not serve as a prognostic factor in the high-tMTV (>105 mL), low-wbTLG (≤219 g), and high-wbTLG (>219 g) groups (Table S13). Different cut-offs for tMTV were evaluated for their predictive capacity regarding treatment response and survival. It was suggested that cut-offs at 98, 105, or 144 mL demonstrated definite discriminative capacities in predicting DCB as well as survival, whereas the previously published cut-off at 75 mL did not (Table S14).
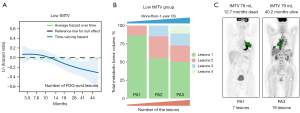
Discussion
To our knowledge, this is the largest retrospective study exploring the application of PET/CT for advanced NSCLC patients chiefly receiving chemoimmunotherapy. We initially established integrated models incorporating PET/CT radiomics and metabolic parameters simultaneously, in terms of predicting long-term survival as well as response among advanced NSCLC patients undergoing chemoimmunotherapy. Moreover, our study is the first to find the survival differences between patients with similar tMTV but different lesion numbers, which offers brand-new perspectives on the effect of MTV.
Herein, in terms of the predictive model for survival, we suggested that patients with heftier MTV, severer metabolic inflammatory status, higher intratumoral metabolic heterogeneity, of squamous cell subtype, and with liver metastases were inclined to present unfavorable survival outcomes. Additionally, to elucidate the PET/CT parameters, we found that our Rad-score grouping indicated distinct disparities in the enrichment of immune-related pathways and validated the prognostic potentials of metabolic burden for patients with PD-L1 ≤50%. Furthermore, we clarified the prognostic disparities among patients with similar tMTV but different tumor distributions. It was indicated that the metabolic burden of distant metastases and intrathoracic lymph nodes impaired survival distinctly and patients with smaller lesions seemed to have better long-term prognoses than those with larger lesions, even of fewer in number. These findings imply that PET/CT could significantly enhance the identification of favorable patients. Specifically, in clinical settings, it might be possible to consider immuno-monotherapy for advanced NSCLC patients in low-risk group to mitigate chemotherapy toxicities.
PET-based MTV parameters have been regarded as promising representatives of tumor burden, which have been proven effective for advanced NSCLC with immuno-monotherapy (35). In 2022, Kim et al. (36) explored the predictive merit of MTV and TLG in a 52-patient advanced NSCLC cohort receiving chemoimmunotherapy for the first time. Later, Silva et al. (11) further corroborated these findings by validating that high wbTLG was positively correlated with glucose transporter-1 expression, which suggested poor responses to chemoimmunotherapy. These two studies unveiled the potential of PET/CT metabolic parameters as biomarkers for chemoimmunotherapy. However, the limited sample sizes led to controversy regarding the optimal cut-offs of these metabolic parameters, hindering their application in clinical practice. Researchers have established diverse cut-offs for tMTV, ranging from 5 to 80 mL (5,7,37) for NSCLC patients with immuno-monotherapy, while for chemoimmunotherapy recipients, the cut-offs for tMTV and wbTLG fluctuated between 43.1–57.3 mL and 146.9–346.8 g respectively (11,36). However, these studies mainly focused on long-term endpoints and failed to check short-term clinical response simultaneously. Our set cut-offs for tMTV at 105 mL and for wbTLG at 219 g were more rational with capacity of predicting durable response and survival simultaneously.
In addition, the predictive value of BLR and SLR for immunotherapy warrants further clarification. We proved that BLR rather than SLR was an independent risk factor for survival, which is similar to the findings for advanced NSCLC receiving chemoimmunotherapy by Kim et al. (36). However, Seban et al. (38) demonstrated that SLR rather than BLR was an independent prognostic factor for 2-year OS in an immuno-monotherapy cohort. The divergences could be attributed to variations in endpoint events and treatment regimens. SLR or BLR, in proxy for hematopoietic tissue metabolism, respectively represent the destruction of tumor-antigen specific T-cells and sequestration of T-cells (39) and were both linked with concurrent inflammatory status (40). Therefore, they were biologically related to treatment response.
Previous studies have suggested that highly heterogeneous tumors on PET/CT respond poorly to immunotherapy (3,41). Underlying, it could be explained by that the heterogeneity of FDG uptake distribution was notably correlated with progressed T cell exhaustion (42). Similarly, Park et al. (43) developed an FDG-PET score that could evaluate PD-L1 expression and immunotherapy response simultaneously. However, it was constructed based on a non-immunotherapy public dataset and was validated in an immunotherapy cohort with all the patients exhibiting positive PD-L1 expression, which was inapplicable for the majority of patients with negative PD-L1 expression in China. Our analysis mitigated its limitations by establishing a robust model on an immunotherapy cohort with a considerable number of PD-L1-negative patients. In our study, the predictive capacity of the Radiomicos model for OS was externally validated in a TCIA dataset, which could predict OS beyond a 6-month time-point. It must be admitted that an early-stage NSCLC validation cohort might not completely mimic the environment of advanced NSCLC, therefore, it merely provided a preliminary reference.
After the construction and validation of the Radiomics models, we deeply probed into whether radiomics could represent tumor biological functions. Previous studies were mainly enthralled by applying CT for the underlying association between radiomics and genomics (12,13) for NSCLC. Yang et al. (44) demonstrated that PET/CT Rad-score predicting neoadjuvant chemoimmunotherapy efficacy was associated with tumor proliferation pathways and immune infiltration. Likewise, we peculiarly focused on the immune-related pathways for the better elaboration of chemoimmunotherapy efficacy. It was found that in the low Rad-score group, Toll-like receptor signaling, PD-L1 expression, and PD-1 checkpoint, as well as B and T cell receptor signaling were upregulated. Unfortunately, the infiltration disparity of few immune cells was merely proved by a single algorithm, thereby we failed to identify a sufficient number of differentially expressed immune cells. Since ESTIMATE analysis indicated that the proportion of immune and stromal cells was comparable across the two groups, we preliminarily regarded that immune infiltration did not differ between Rad-score groups. These might be attributed to the early-stage NSCLC cohort and underscores the need for further research. It has to be pointed out that we explored the biological functions of our scoring system based on an early-stage NSCLC cohort, which could not completely mimic the advanced settings. However, we set the cut-off value in the early-stage validation cohort identical to its in our advanced model, to validate the association between the radiomics features and immune-related pathways rather than establish an integrated model encompassing radiomics and transcriptomics features.
Analogous to one previous study (1), PD-L1 TPS failed to predict survival for chemoimmunotherapy in our cohort. Nonetheless, we found that for patients with low PD-L1 expression, tMTV and wbTLG remained as alternative biomarkers for survival. It was rewarding since we could probably utilize immuno-monotherapy for patients with PD-L1 TPS ≤50% but with limited tMTV or wbTLG, thus eliminating the potential toxicities associated with chemotherapy.
The truth is that in clinical scenarios, there are likely patients with much the same tMTV but sharply different lesion numbers. We supposed that these groups might generate deviating prognoses. We proved that in the low-tMTV (≤105 mL) group, the patients with more small lesions seemed to have better long-term prognoses than those with larger lesions, even of fewer in number. It was indicated that we might not consider tMTV solely but neglect lesions’ numbers in prognosis judgment. Additionally, we assessed the significance hierarchy of MTV as well as TLG from different lesion locations on survival. It was suggested that the metastases’ metabolic burden might have a more pronounced influence on survival than that of the bilateral lung lesions. Recently, mediastinal lymph nodes have demonstrated potential implications for immunotherapy. Studies (45,46) have found that PET/CT N2 (+) could stratify survival for NSCLC patients receiving neoadjuvant chemoimmunotherapy. Pioneeringly, we found that intrathoracic lymph nodes’ metabolic burden remained predictive capacity in advanced settings, which portrays the pivotal role of lymph nodes for immunotherapy from an alternative perspective. This finding might be attributed to the fact that a large proportion of unresectable stage III patients were recruited in our cohort.
In contrast to previous studies which mainly enrolled monotherapy patients, our research is superior in its larger sample size, longer follow-up period, relatively homogeneous cohort with first-line chemoimmunotherapy, and auxiliary exploration of underlying immune-related biological functions between different Rad-score groups. We are also the first to explore survival differences between patients with similar tMTV but different tumor distribution and numbers. These comprehensively confirm the utility of PET/CT as a non-invasive prognostic tool for chemoimmunotherapy in real-world settings, which might promote the application of immuno-monotherapy for low-risk patients to alleviate chemotherapy toxicities. Concurrently, different scanners and semiautomated segmentation methods contributed to increased reproducibility and dependability. Regrettably, certain limitations remained in our research. First, the study retrospectively built a prognostic model with a relatively small sample size, and the external validation cohort was a non-immunotherapy group, which merely served as a supplementary reference. Moreover, a small proportion of the patients in the training cohort received immuno-monotherapy or combined anti-angiogenesis agents. In light of these, we conducted a revalidation of all predictors by adjusting for this confounder. Additionally, the limited accessibility of the PD-L1 expression test among patients might result in the omission of potential clusters, such as PD-L1himetabolic burdenhi. Finally, most participants received PET/CT merely at baseline, making it impossible to evaluate prompt therapy response following chemoimmunotherapy. Recently, some researchers have examined the predictive capacity of baseline or delta PET parameters for immunotherapy in neoadjuvant settings (44), which brought novel horizons with findings distinct from those observed in advanced settings. Future studies might compare the application of PET/CT in both scenarios and validate our findings prospectively.
Conclusions
PET/CT-based Radiomicsmetabolic model could be a beneficial tool of stratifying tumor heterogeneity and predicting survival in advanced NSCLC patients undergoing chemoimmunotherapy. Underlyingly, PET/CT parameters were embedded with gene patterns information and were still practical in patients with PD-L1 ≤50%. Moreover, it is recommended that we should not focus merely on the whole-body metabolic parameters but a comprehensive glimpse on their locations and numbers. Hopefully, this non-invasive prognostic risk scoring system for chemoimmunotherapy might facilitate the application of immuno-monotherapy for low-risk patients to alleviate additional chemotherapy toxicities.
Acknowledgments
Funding: This work was supported by
Footnote
Reporting Checklist: The authors have completed the STROBE reporting checklist. Available at https://tlcr.amegroups.com/article/view/10.21037/tlcr-24-576/rc
Data Sharing Statement: Available at https://tlcr.amegroups.com/article/view/10.21037/tlcr-24-576/dss
Peer Review File: Available at https://tlcr.amegroups.com/article/view/10.21037/tlcr-24-576/prf
Conflicts of Interest: All authors have completed the ICMJE uniform disclosure form (available at https://tlcr.amegroups.com/article/view/10.21037/tlcr-24-576/coif). Y.S. serves as an Editor-in-Chief of Translational Lung Cancer Research. The other authors have no conflicts of interest to declare.
Ethical Statement: The authors are accountable for all aspects of the work in ensuring that questions related to the accuracy or integrity of any part of the work are appropriately investigated and resolved. The study was conducted in accordance with the Declaration of Helsinki (as revised in 2013) and was approved by the Institutional Review Board of Jinling Hospital (No. 2024DZKY-049-01). Institutional Review Board waived the requirement for informed consent considering the retrospective design of this study.
Open Access Statement: This is an Open Access article distributed in accordance with the Creative Commons Attribution-NonCommercial-NoDerivs 4.0 International License (CC BY-NC-ND 4.0), which permits the non-commercial replication and distribution of the article with the strict proviso that no changes or edits are made and the original work is properly cited (including links to both the formal publication through the relevant DOI and the license). See: https://creativecommons.org/licenses/by-nc-nd/4.0/.
References
- Paz-Ares L, Luft A, Vicente D, et al. Pembrolizumab plus Chemotherapy for Squamous Non-Small-Cell Lung Cancer. N Engl J Med 2018;379:2040-51. [Crossref] [PubMed]
- Garassino MC, Gadgeel S, Speranza G, et al. Pembrolizumab Plus Pemetrexed and Platinum in Nonsquamous Non-Small-Cell Lung Cancer: 5-Year Outcomes From the Phase 3 KEYNOTE-189 Study. J Clin Oncol 2023;41:1992-8. [Crossref] [PubMed]
- Mu W, Tunali I, Gray JE, et al. Radiomics of (18)F-FDG PET/CT images predicts clinical benefit of advanced NSCLC patients to checkpoint blockade immunotherapy. Eur J Nucl Med Mol Imaging 2020;47:1168-82. [Crossref] [PubMed]
- Dall'Olio FG, Calabrò D, Conci N, et al. Baseline total metabolic tumour volume on 2-deoxy-2-[18F]fluoro-d-glucose positron emission tomography-computed tomography as a promising biomarker in patients with advanced non-small cell lung cancer treated with first-line pembrolizumab. Eur J Cancer 2021;150:99-107.
- Seban RD, Mezquita L, Berenbaum A, et al. Baseline metabolic tumor burden on FDG PET/CT scans predicts outcome in advanced NSCLC patients treated with immune checkpoint inhibitors. Eur J Nucl Med Mol Imaging 2020;47:1147-57. [Crossref] [PubMed]
- Jreige M, Letovanec I, Chaba K, et al. (18)F-FDG PET metabolic-to-morphological volume ratio predicts PD-L1 tumour expression and response to PD-1 blockade in non-small-cell lung cancer. Eur J Nucl Med Mol Imaging 2019;46:1859-68. [Crossref] [PubMed]
- Chardin D, Paquet M, Schiappa R, et al. Baseline metabolic tumor volume as a strong predictive and prognostic biomarker in patients with non-small cell lung cancer treated with PD1 inhibitors: a prospective study. J Immunother Cancer 2020;8:e000645. [Crossref] [PubMed]
- Kudura K, Ritz N, Templeton AJ, et al. Predictive Value of Total Metabolic Tumor Burden Prior to Treatment in NSCLC Patients Treated with Immune Checkpoint Inhibition. J Clin Med 2023;12:3725. [Crossref] [PubMed]
- Kudura K, Ritz N, Kutzker T, et al. Predictive Value of Baseline FDG-PET/CT for the Durable Response to Immune Checkpoint Inhibition in NSCLC Patients Using the Morphological and Metabolic Features of Primary Tumors. Cancers (Basel) 2022;14:6095. [Crossref] [PubMed]
- Eude F, Guisier F, Salaün M, et al. Prognostic value of total tumour volume, adding necrosis to metabolic tumour volume, in advanced or metastatic non-small cell lung cancer treated with first-line pembrolizumab. Ann Nucl Med 2022;36:224-34. [Crossref] [PubMed]
- Silva SB, Wanderley CWS, Gomes Marin JF, et al. Tumor glycolytic profiling through (18)F-FDG PET/CT predicts immune checkpoint inhibitor efficacy in advanced NSCLC. Ther Adv Med Oncol 2022;14:17588359221138386. [Crossref] [PubMed]
- Guo QK, Yang HS, Shan SC, et al. A radiomics nomogram prediction for survival of patients with "driver gene-negative" lung adenocarcinomas (LUAD). Radiol Med 2023;128:714-25. [Crossref] [PubMed]
- Lin P, Lin YQ, Gao RZ, et al. Integrative radiomics and transcriptomics analyses reveal subtype characterization of non-small cell lung cancer. Eur Radiol 2023;33:6414-25. [Crossref] [PubMed]
- Dall'Olio FG, Garcia CA, Zrafi WS, et al. 4P FDG PET derived metabolic tumor volume (MTV) and its transcriptomic correlates as biomarker to predict efficacy of immune checkpoint inhibitors (ICB) alone or in combination with chemotherapy in advanced NSCLC: A multicentric study. Immuno-Oncology and Technology 2022;16:100109.
- Bakr S, Gevaert O, Echegaray S, et al. A radiogenomic dataset of non-small cell lung cancer. Sci Data 2018;5:180202. [Crossref] [PubMed]
- Clark K, Vendt B, Smith K, et al. The Cancer Imaging Archive (TCIA): maintaining and operating a public information repository. J Digit Imaging 2013;26:1045-57. [Crossref] [PubMed]
- Nioche C, Orlhac F, Boughdad S, et al. LIFEx: A Freeware for Radiomic Feature Calculation in Multimodality Imaging to Accelerate Advances in the Characterization of Tumor Heterogeneity. Cancer Res 2018;78:4786-9. [Crossref] [PubMed]
- Meignan M, Sasanelli M, Casasnovas RO, et al. Metabolic tumour volumes measured at staging in lymphoma: methodological evaluation on phantom experiments and patients. Eur J Nucl Med Mol Imaging 2014;41:1113-22. [Crossref] [PubMed]
- Zwanenburg A, Vallières M, Abdalah MA, et al. The Image Biomarker Standardization Initiative: Standardized Quantitative Radiomics for High-Throughput Image-based Phenotyping. Radiology 2020;295:328-38. [Crossref] [PubMed]
- Seban RD, Moya-Plana A, Antonios L, et al. Prognostic 18F-FDG PET biomarkers in metastatic mucosal and cutaneous melanoma treated with immune checkpoint inhibitors targeting PD-1 and CTLA-4. Eur J Nucl Med Mol Imaging 2020;47:2301-12. [Crossref] [PubMed]
- Tankyevych O, Trousset F, Latappy C, et al. Development of Radiomic-Based Model to Predict Clinical Outcomes in Non-Small Cell Lung Cancer Patients Treated with Immunotherapy. Cancers (Basel) 2022;14:5931. [Crossref] [PubMed]
- Masson-Deshayes S, Schvartz C, Dalban C, et al. Prognostic value of (18)F-FDG PET/CT metabolic parameters in metastatic differentiated thyroid cancers. Clin Nucl Med 2015;40:469-75. [Crossref] [PubMed]
- Berardi R, Santoni M, Rinaldi S, et al. Pre-treatment systemic immune-inflammation represents a prognostic factor in patients with advanced non-small cell lung cancer. Ann Transl Med 2019;7:572. [Crossref] [PubMed]
- Ferrucci PF, Ascierto PA, Pigozzo J, et al. Baseline neutrophils and derived neutrophil-to-lymphocyte ratio: prognostic relevance in metastatic melanoma patients receiving ipilimumab. Ann Oncol 2016;27:732-8. [Crossref] [PubMed]
- Arrieta O, Michel Ortega RM, Villanueva-Rodríguez G, et al. Association of nutritional status and serum albumin levels with development of toxicity in patients with advanced non-small cell lung cancer treated with paclitaxel-cisplatin chemotherapy: a prospective study. BMC Cancer 2010;10:50. [Crossref] [PubMed]
- Seymour L, Bogaerts J, Perrone A, et al. iRECIST: guidelines for response criteria for use in trials testing immunotherapeutics. Lancet Oncol 2017;18:e143-52. [Crossref] [PubMed]
- Leijenaar RT, Carvalho S, Velazquez ER, et al. Stability of FDG-PET Radiomics features: an integrated analysis of test-retest and inter-observer variability. Acta Oncol 2013;52:1391-7. [Crossref] [PubMed]
- Orlhac F, Boughdad S, Philippe C, et al. A Postreconstruction Harmonization Method for Multicenter Radiomic Studies in PET. J Nucl Med 2018;59:1321-8. [Crossref] [PubMed]
- Tibshirani R. The lasso method for variable selection in the Cox model. Stat Med 1997;16:385-95. [Crossref] [PubMed]
- Camp RL, Dolled-Filhart M, Rimm DL. X-tile: a new bio-informatics tool for biomarker assessment and outcome-based cut-point optimization. Clin Cancer Res 2004;10:7252-9. [Crossref] [PubMed]
- Miao X, Guo Y, Ding L, et al. A dynamic online nomogram for predicting the heterogeneity trajectories of frailty among elderly gastric cancer survivors. Int J Nurs Stud 2024;153:104716. [Crossref] [PubMed]
- Cacciani N, Skärlén Å, Wen Y, et al. A prospective clinical study on the mechanisms underlying critical illness myopathy-A time-course approach. J Cachexia Sarcopenia Muscle 2022;13:2669-82. [Crossref] [PubMed]
- Yoshihara K, Shahmoradgoli M, Martínez E, et al. Inferring tumour purity and stromal and immune cell admixture from expression data. Nat Commun 2013;4:2612. [Crossref] [PubMed]
- Wilkerson MD, Hayes DN. ConsensusClusterPlus: a class discovery tool with confidence assessments and item tracking. Bioinformatics 2010;26:1572-3. [Crossref] [PubMed]
- Ling T, Zhang L, Peng R, et al. Prognostic value of (18)F-FDG PET/CT in patients with advanced or metastatic non-small-cell lung cancer treated with immune checkpoint inhibitors: A systematic review and meta-analysis. Front Immunol 2022;13:1014063. [Crossref] [PubMed]
- Kim CG, Hwang SH, Kim KH, et al. Predicting treatment outcomes using (18)F-FDG PET biomarkers in patients with non-small-cell lung cancer receiving chemoimmunotherapy. Ther Adv Med Oncol 2022;14:17588359211068732. [Crossref] [PubMed]
- Hashimoto K, Kaira K, Yamaguchi O, et al. Potential of FDG-PET as Prognostic Significance after anti-PD-1 Antibody against Patients with Previously Treated Non-Small Cell Lung Cancer. J Clin Med 2020;9:725. [Crossref] [PubMed]
- Seban RD, Assié JB, Giroux-Leprieur E, et al. Prognostic value of inflammatory response biomarkers using peripheral blood and 18F-FDG PET/CT in advanced NSCLC patients treated with first-line chemo- or immunotherapy. Lung Cancer 2021;159:45-55. [Crossref] [PubMed]
- Seban RD, Nemer JS, Marabelle A, et al. Prognostic and theranostic 18F-FDG PET biomarkers for anti-PD1 immunotherapy in metastatic melanoma: association with outcome and transcriptomics. Eur J Nucl Med Mol Imaging 2019;46:2298-310. [Crossref] [PubMed]
- Salaun PY, Gastinne T, Bodet-Milin C, et al. Analysis of 18F-FDG PET diffuse bone marrow uptake and splenic uptake in staging of Hodgkin's lymphoma: a reflection of disease infiltration or just inflammation? Eur J Nucl Med Mol Imaging 2009;36:1813-21. [Crossref] [PubMed]
- Mu W, Jiang L, Shi Y, et al. Non-invasive measurement of PD-L1 status and prediction of immunotherapy response using deep learning of PET/CT images. J Immunother Cancer 2021;9:e002118. [Crossref] [PubMed]
- Zhang Y, Hu HH, Zhou SH, et al. PET-based radiomics visualizes tumor-infiltrating CD8 T cell exhaustion to optimize radiotherapy/immunotherapy combination in mouse models of lung cancer. Biomark Res 2023;11:10. [Crossref] [PubMed]
- Park C, Na KJ, Choi H, et al. Tumor immune profiles noninvasively estimated by FDG PET with deep learning correlate with immunotherapy response in lung adenocarcinoma. Theranostics 2020;10:10838-48. [Crossref] [PubMed]
- Yang M, Li X, Cai C, et al. [18F]FDG PET-CT radiomics signature to predict pathological complete response to neoadjuvant chemoimmunotherapy in non-small cell lung cancer: a multicenter study. Eur Radiol 2024;34:4352-63.
- Deng H, Xiong S, Zhong R, et al. "Major pathologic response" in lymph nodes: a modified nodal classification for non-small cell lung cancer patients treated with neoadjuvant immunochemotherapy. Exp Hematol Oncol 2023;12:40. [Crossref] [PubMed]
- Zhang L, E H, Huang J, et al. Clinical utility of [18F]FDG PET/CT in the assessment of mediastinal lymph node disease after neoadjuvant chemoimmunotherapy for non-small cell lung cancer. Eur Radiol 2023;33:8564-72.