Machine learning-based radiomics for guiding lymph node dissection in clinical stage I lung adenocarcinoma: a multicenter retrospective study
Introduction
Lung cancer is the most prevalent form of cancer and the primary cause of cancer-related fatalities worldwide, constituting approximately 18.0% of all cancer-related deaths (1,2). Non-small cell lung cancer (NSCLC) represents the most common subtype of lung cancer worldwide (3). Lymph node metastasis (LNM) of tumors plays a pivotal role in disease progression and significantly impacts treatment choices and prognosis. Roughly 40% of lung cancer patients develop metastatic disease (4). Regional NSCLC, according to statistics from the American Cancer Society, is characterized by the dissemination of cancerous cells from the lung to the neighboring lymph nodes, the five-year survival from lung cancer tends to be below 20% in most countries (3). Therefore, the importance of assessing lymph node status in NSCLC patients for prognostication and treatment decisions has been well-established in prior research (5). At present, lymph node puncture biopsy and intraoperative lymph node dissection are the gold standard to determine whether LNM occurs in early lung cancer (4,6). Extensive lymph node dissection significantly heightens the risk of physical trauma to patients, including unnecessary tissue damage, pneumothorax, lymph node leakage, and prolonged hospital stays (6,7).
The computed tomography (CT), positron emission tomography/CT (PET/CT), and other methods can assist in assessing the presence of LNM, but they are very dependent on the personal experience of the reviewers, especially for early-stage lung cancer, in which the judgement is more difficult (8,9). Spiral CT remains the preferred method for pre-treatment lymph node screening due to its lower cost and convenience. Only obvious lymph node enlargement is considered as metastasis in imaging, and most of the early lung cancer is occult LNM (10). In addition, the conventional CT's sensitivity in identifying metastatic lymph nodes is only 51% (11,12), lacking detection of micrometastasis and accurate lymph node status representation (13). Thus, there is a need for accurate, non-invasive preoperative prediction methods for LNM in NSCLC.
Recently, computer-aided diagnosis and artificial intelligence (AI) have gained increasing attention, offering novel strategies for disease diagnosis, treatment, and prognosis analysis (14). Radiomics is a technique for converting images into quantitatively identifiable information. It can comprehensively assess the heterogeneity of the tumor and provide more accurate information for diagnosis (15). Wang et al. (16) used radiomics methods to extract radiomics features from the peri-tumor 15 mm region to predict LNM in patients with stage T1 NSCLC. However, due to the limited sample size and lack of external data validation of the model, the reliability of the model needs to be further improved. We urgently need a LNM prediction model with good predictive performance, to provide strategic support for our lymph node dissection. Therefore, this study constructed various predictive models based on clinical data and imaging data from multicenter cohorts using radiomics technology. These models were tested on an external validation set to analyze and evaluate which predictive model has the best predictive value for assessing the risk of LNM in clinical stage I invasive LUAD. We present this article in accordance with the TRIPOD reporting checklist (available at https://tlcr.amegroups.com/article/view/10.21037/tlcr-24-668/rc).
Methods
Patient selection
Retrospective collection of clinical information and imaging data of clinical stage I invasive LUAD patients was conducted at three medical centers [Cancer Hospital, Chinese Academy of Medical Sciences (CCAM), the First Affiliated Hospital of Chongqing Medical University (1CMU), and Beijing Chao-Yang Hospital (BCYH)] from October 2017 to September 2020. CCAM and 1CMU cohorts were used for predictive model construction and were randomly divided into training and testing groups in a 2:1 ratio (17,18). The BCYH cohort served as an independent external validation group.
Patient cohorts from three centers were identified based on established inclusion and exclusion criteria. The inclusion criteria were: (I) the diameter of the tumor on CT image was 10–30 mm, and the clinical stage was cT1N0; (II) the tumor underwent radical resection, with a pathological diagnosis of invasive adenocarcinoma; (III) systematic lymph node dissection was performed (this involved at least three groups of mediastinal lymph nodes and at least three groups of peripheral lymph nodes), and there was a definitive pathological diagnosis of whether LNM occurred; (IV) high-resolution contrast-enhanced CT (0.5–2.0 mm) of the chest was performed within 3 weeks before surgery. The exclusion criteria were: (I) the pathological diagnosis of the tumor was adenocarcinoma in situ (AIS) or minimally invasive adenocarcinoma (MIA); (II) incomplete clinical data information; (III) poor CT image quality; (IV) history of secondary thoracic surgery, radiotherapy, chemotherapy, targeted therapy, and other treatments; (V) history of other malignant tumors. According to the presence or absence of LNM, all patients were divided into the N0 group (without LNM) and the N+ group (with LNM). The workflow is shown in Figure 1. The study was approved by the Institutional Review Board of the Cancer Hospital, Chinese Academy of Medical Sciences (No. 22/492-3694). The other two participating hospitals were informed and agreed with the study. Since this is a retrospective study, the requirement for informed consent was waived. The study was conducted in accordance with the Declaration of Helsinki (as revised in 2013).
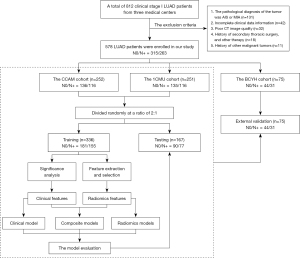
Image acquisition and clinical-radiological semantic features
The parameters of CT scanners in each medical center are detailed in Table S1. Two radiologists, each with 10 years of experience, evaluated the radiological semantic features in the lung window setting (window, −500 HU; width, 1,400 HU) and the mediastinal window setting (window, 40 HU; width, 350 HU) in a blinded manner. Any discrepancies in imaging findings were resolved through consultation between the two experts. The clinical-radiological semantic features included age, gender, nodules diameter, location, density (solid or mixed), cavity, margin status (clear & smooth or rough & blurred), lobulated sign, spiculated sign, and pleural indentation.
Extraction of radiomics features
The regions of interest (ROI) were manually contoured slice-wise on the axial projection to create a segmentation using 3D slicer software (version 5.1.0). Segmentation for all cases was performed by two thoracic surgeons with 10 years of experience. In case of disagreement, they reached an agreement through discussion and consultation. Finally, 1,316 radiomics features were extracted from each tumor using the PyRadiomics package in Python.
Radiomics feature selection
To continuously eliminate redundant radiomics features, we used the maximum correlation and minimum redundancy (mRMR) feature selection algorithm (from the mRMR selection package in Python) to filter out highly informative features. In addition, the least absolute shrinkage and selection operator (LASSO) method, suitable for high-dimensional data regression, was employed to select the most relevant predictive features from the dataset. The radiomics score (Rad-score) for each patient was computed as a linear combination of the selected features weighted by their coefficients. The optimal λ value was determined by minimizing the mean square error (MSE) on the model.
Development and clinical application of a personalized prediction model
In this study, we identified significant features of the training group associated with LNM to construct three logistic regression models (statsmodels package in Python), and tested these models on the testing group and the external validation group: (I) a clinical prediction model was built using significant clinical-radiological semantic features identified through significance testing; (II) a radiomics prediction model was created based on radiomics features selected by machine learning; (III) a composite model was developed by integrating the features from the first two parts. The predictive performance of each model was evaluated using the receiver operating characteristic (ROC) curve and the area under the curve (AUC). The Delong test was used to assess the significance of differences in predictive performance between models within each group. Decision curve analysis (DCA) was performed on the training group, testing group, and validation group to compare the net benefits at various threshold probabilities and to evaluate the clinical utility of the clinical, radiomics, and composite models (using the media package in R software). Based on the AUC, Delong test, and DCA results, the best predictive model with stable clinical net benefits was selected.
A nomogram of the best model was constructed by the radiomics features which were converted into a radiomics score (Radscore) and the possible clinical-radiological semantic features (using the rms package in R software). Calibration curves were drawn and the Hosmer-Lemeshow test was conducted to assess the goodness-of-fit, with P>0.05 indicating a good fit to the actual situation.
Statistical analysis
Statistical analysis was conducted using Python software (version 3.7.13), R software (version 4.3.2), and SPSS (version 21.0). For general clinical characteristics and imaging semantic features, continuous variables were expressed as mean ± standard deviation and categorical variables were expressed as frequency (percentage). Depending on whether the continuous variables follow a normal distribution, either the independent samples t-test or the Mann-Whitney U test was used to compare differences between groups. The χ2 test was used to compare differences between categorical variables. All statistical analyses in this study considered P<0.05 to indicate statistical significance.
Results
Clinical characteristics
The 503 patients from the CCAM and 1CMU cohorts were randomly divided into training and testing groups in a 2:1 ratio, while 75 patients from the BCYH cohort served as an independent external validation group. Characteristics of a total of 578 patients for the training, testing, and validation groups are presented in Table 1. The LNM status and pN stage for each group are presented in Table S2. The training group consisted of 336 patients (144 males and 192 females). The average age was 57.72±10.22 years in the N0 group and 56.93±10.62 years in the N+ group. The testing group comprised 167 patients (74 males and 93 females), while the validation group consisted of 75 patients (36 males and 39 females).
Table 1
Characteristics | Training group (n=336) | Testing group (n=167) | Validation group (n=75) | ||||||||
---|---|---|---|---|---|---|---|---|---|---|---|
N0 (n=181) | N+ (n=155) | P | N0 (n=90) | N+ (n=77) | P | N0 (n=44) | N+ (n=31) | P | |||
Gender | 0.43 | 0.006 | 0.32 | ||||||||
Male | 74 (40.9) | 70 (45.2) | 31 (34.4) | 43 (55.8) | 19 (43.2) | 17 (54.8) | |||||
Female | 107 (59.1) | 85 (54.8) | 59 (65.6) | 34 (44.2) | 25 (56.8) | 14 (45.2) | |||||
Age (years) | 57.72±10.22 | 56.93±10.62 | 0.49 | – | – | – | – | ||||
Diameter (mm) | 19.10±5.76 | 20.33±4.18 | 0.03 | 18.71±5.60 | 19.67±4.12 | 0.21 | 18.39±5.41 | 20.50±4.36 | 0.08 | ||
Location | 0.67 | 0.95 | 0.68 | ||||||||
Upper & middle lobe | 130 (71.8) | 108 (69.7) | 44 (48.9) | 38 (49.4) | 29 (65.9) | 19 (61.3) | |||||
Lower lobe | 51 (28.2) | 47 (30.3) | 46 (51.1) | 39 (50.6) | 15 (34.1) | 12 (38.7) | |||||
Tumor side | 0.78 | 0.67 | 0.60 | ||||||||
Right | 95 (52.5) | 79 (51.0) | 52 (57.8) | 47 (61.0) | 24 (54.5) | 15 (48.4) | |||||
Left | 86 (47.5) | 76 (49.0) | 38 (42.2) | 30 (39.0) | 20 (45.5) | 16 (51.6) | |||||
Density | <0.001 | <0.001 | 0.001 | ||||||||
Solid | 54 (29.8) | 132 (85.2) | 30 (33.3) | 68 (88.3) | 15 (34.1) | 23 (74.2) | |||||
Mixed ground-glass | 127 (70.2) | 23 (14.8) | 60 (66.7) | 9 (11.7) | 29 (65.9) | 8 (25.8) | |||||
Margin status | 0.48 | 0.26 | 0.15 | ||||||||
Clear & smooth | 77 (42.5) | 60 (38.7) | 38 (42.2) | 26 (33.8) | 20 (45.5) | 9 (29.0) | |||||
Rough & blurred | 104 (57.5) | 95 (61.3) | 52 (57.8) | 51 (66.2) | 24 (54.5) | 22 (71.0) | |||||
Cavity | <0.001 | <0.001 | 0.051 | ||||||||
Yes | 56 (30.9) | 90 (58.1) | 22 (24.4) | 50 (64.9) | 7 (15.9) | 11 (35.5) | |||||
No | 125 (69.1) | 65 (41.9) | 68 (75.6) | 27 (35.1) | 37 (84.1) | 20 (64.5) | |||||
Lobulated sign | <0.001 | <0.001 | 0.54 | ||||||||
Yes | 124 (68.5) | 139 (89.7) | 58 (64.4) | 69 (88.3) | 34 (77.3) | 22 (71.0) | |||||
No | 57 (31.5) | 16 (10.3) | 32 (35.6) | 9 (11.7) | 10 (22.7) | 9 (29.0) | |||||
Spiculated sign | 0.054 | 0.96 | 0.45 | ||||||||
Yes | 130 (71.8) | 126 (81.3) | 71 (78.9) | 61 (79.2) | 26 (59.1) | 21 (67.7) | |||||
No | 51 (28.2) | 29 (18.7) | 19 (21.1) | 16 (20.8) | 18 (40.9) | 10 (32.3) | |||||
Pleural indentation | 0.12 | 0.19 | 0.39 | ||||||||
Yes | 124 (68.5) | 118 (76.1) | 62 (68.9) | 60 (77.9) | 27 (61.4) | 22 (71.0) | |||||
No | 57 (31.5) | 37 (23.9) | 28 (31.1) | 17 (22.1) | 17 (38.6) | 9 (29.0) |
Data are presented as mean ± standard deviation or n (%).
Regarding clinical features, there were no significant differences in clinical features of age or gender between the N0 and N+ groups. Radiological semantic features such as “diameter”, “density”, “margin status”, and “lobulated sign” were found to differentiate between N0 and N+. Table 1 provides a summary of patient details with final diagnoses of N0 and N+ across the training, testing, and validation groups. Considering that “tumor size” is a significant parameter affecting LNM, we further validated the differences in the number of patients included in each group with cT1b (10–20 mm), cT1c (20–30 mm) to avoid potential imbalance. There was no statistically significant difference in the results between the three groups (Table S3).
Feature selection of the radiomics signature
A total of 1,316 radiomics features were extracted. The predictive features most relevant to the categorical variables were implemented using mRMR, and 30 features were obtained by mRMR analysis (Figure 2A). After assessing reproducibility based on segmentation data, a LASSO logistic regression model with λ=0.00026 selected 8 features with non-zero coefficients to establish the Rad-score (Figure 2B). The 8 most relevant features were used to construct the radiomics model. Table S4 presented the Rad-score constructed based on these selected features.
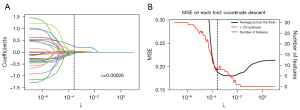
Performance of the predictive models
In the training group (Figure 3A), the AUC was 0.820 for the clinical model, 0.871 for the radiomics model, and 0.883 for the composite model. In the testing group (Figure 3B), the AUC was 0.897 for the clinical model, 0.915 for the radiomics model, and 0.934 for the composite model. In addition, the AUC of the external validation group was 0.710 for the clinical model, 0.870 for the radiomics model, and 0.841 for the composite model (Figure 3C). In general, the radiomics model and the composite model demonstrated better predictive performance across all data cohorts. It was noteworthy that the prediction performance of the clinical model markedly declined in the validation group. This suggested that constructing prediction models based on radiomics features was feasible, while clinical features as parameters for prediction models may not be reliable.
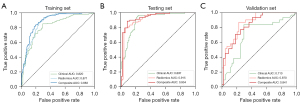
The results of the Delong test showed that the AUCs of the radiomics model were significantly higher than those of the clinical model in both the training (P=0.007) and validation (P=0.005) groups. Similarly, the composite models also significantly outperformed the clinical model in both datasets (P<0.05). Furthermore, in the external validation set, although there was no significant difference between the radiomics model and the composite model, the radiomics model still demonstrated the highest predictive performance (Table 2).
Table 2
Model 1 vs. model 2 | P value | ||
---|---|---|---|
Training group | Testing group | Validation group | |
Clinical vs. radiomics | 0.007 | 0.45 | 0.005 |
Clinical vs. composite | <0.001 | 0.08 | 0.01 |
Radiomics vs. composite | 0.11 | 0.06 | 0.10 |
AUC, area under the curve; clinical, clinical model; radiomics, radiomics model; composite, composite model.
Developing personalized prediction nomogram and clinical application
The clinical utility of the developed model was represented by the DCA. In all three cohorts, both the radiomics and composite models demonstrated substantial clinical benefits across broader ranges of risk thresholds, while the clinical model showed significantly lower clinical benefits in comparison (Figure 4A-4C). This suggested once again that predicting LNM risk in clinical stage I invasive LUAD solely through clinical-radiological semantic features was insufficient. Radiomics technology could complement and enhance the predictive ability of the model.
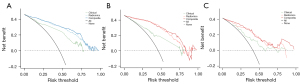
According to the above, the radiomics model’s ROC curve demonstrated higher and more consistent predictive performance across multiple datasets and showed better clinical net benefit at higher risk thresholds in the DCA. Therefore, this study identified the radiomics model as the best predictive model for assessing the risk of LNM in clinical stage I invasive LUAD, and a nomogram was constructed based on this model (Figure 5A). The calibration curves for the probability of LNM showed a good fit. (Hosmer-Lemeshow test, P=0.12) (Figure 5B).
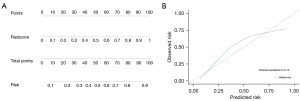
Discussion
Currently, many studies focus on the strategy of lymph node dissection in early-stage lung cancer. The results of NCT03216551 study (19) showed that for clinical T1N0 invasive lung cancer, patients with the consolidation tumor ratio (CTR) ≤0.5 did not require mediastinal lymph node dissection. In addition, the results of the JCOG0802 study (5) on pulmonary tumors with a diameter ≤2 cm showed that non-adjacent interlobar and mediastinal lymph node dissection had little impact on pathological nodal staging in patients with part-solid tumors. For low-risk, pure-solid tumors, lobe-selective lymph node dissection strategies may eventually replace systematic lymph node dissection. Similarly, the study by Mimae et al. (20) showed that omitting lymph node dissection may be acceptable with curative intent for small tumors with radiological ground glass opacity dominance. Appropriate surgical procedures, such as lung wedge resection, segmental resection, or lobectomy, can provide satisfactory results for patients with indolent tumors when the surgical margins are secured. However, how to effectively identify LNM in early-stage lung cancer patients and avoid excessive dissection are urgent problems that need to be solved.
This study provided a basis for formulating lymph node dissection strategies before surgery and helped to better identify the TNM stage of the early-stage LUAD. We developed three prediction models to predict LNM of clinical stage I LUAD using clinical-radiological semantic features, radiomics features, and a combination of both, respectively. Finally, the radiomics model demonstrated superior and more consistent predictive performance compared to the clinical model. An independent predictive nomogram was constructed using risk factors derived from radiomics features, and its robust predictive performance was validated. To reduce the error caused by selection bias, we included patients with stage I invasive LUAD ranging from 10 to 30 mm, as LNM is rare in 0–10 mm lung cancer, carcinoma in situ, and microinvasive adenocarcinoma in clinical practice (21).
Size-based conventional CT has limited predictive accuracy. Currently, PET/CT imaging is the standard noninvasive method for detecting lymph node metastases in clinical practice; however, it still has a high false-positive rate. Among patients without fluorodeoxyglucose uptake on PET/CT, the incidence of pathological mediastinal lymph node metastases can range from 13.2% to 24.1% (22). In comparison, radiomics offers a more accurate prediction of LNM. In a study on predicting occult LNM in stage IA lung adenocarcinoma (LUAD) patients, the clinical model constructed from tumor clinical features showed poor predictive performance in the external validation group (AUC =0.814) compared to the imaging model (AUC =0.917) and the combined model (AUC =0.933) (23). Our study indicated that in stage I (10–30 mm) patients, the marginal status and lobulation sign may be risk factors for LNM, reflecting active tumor cell proliferation and potential invasion of surrounding lymphatic vessels, leading to metastasis. Although the clinical semantic features of primary tumor lesions could reflect the risk of LNM to a certain extent, its predictive power was quite limited. In the external validation group, the clinical model (AUC =0.710) constructed solely from clinical-semantic features was not stable in their predictive power and did not have a good clinical net benefit.
In comparison, radiomics technology has the potential to address this deficiency and enhance the accuracy of predicting LNM in clinical stage I invasive LUAD. Radiomics, a quantitative method in medical imaging, extracts rich high-dimensional image features to reveal underlying pathophysiological heterogeneity (24,25). A previous study (26) reported a radiomics model can improve the prediction performance of LNM in cervical cancer patients, the radiomics signatures can be well distinguished between LNM and non-LNM groups, the AUC of the training group ROC was 0.753, and the AUC of the verification group was 0.754. Yang et al. (27) reported that a radiomics nomogram based on contrast-enhanced CT predicted LNM in LUAD patients, in the training group, the AUC for the radiomics model and the clinical model was 0.81 and 0.69, respectively. In the validation group, the AUC was 0.82 and 0.64, respectively. In addition, Wang et al. (16) found that peritumoral radiomics features, particularly wavelet features, enhanced LNM prediction in clinical T1 LUAD. The AUC values of radiomics signatures combined with the gross tumor volume and the peritumoral volume were 0.829 (95% CI: 0.745–0.913) and 0.825 (95% CI: 0.733–0.918), respectively. Furthermore, another study have explored the predictive capabilities of various machine learning algorithms, such as logistic regression and random forest, using radiomic features or a combination of clinical and radiological data to predict LNM, demonstrating improved accuracy and clinical utility (28). Although the radiomics model constructed by the above studies had better predictive performance than the clinical model, the sample size was small, and the data for external validation was insufficient in their studies.
In this study, we included patients from three medical centers, of which one medical center was used as the independent validation group. Selection bias was effectively reduced by reasonable inclusion and exclusion criteria, and the reliability of the model was further improved. The radiomics model constructed in this study demonstrated strong predictive ability across multiple datasets and showed the best performance in the external validation set. Additionally, in both the DCA and nomogram assessments, it exhibited advantages in clinical benefit.
However, there are several limitations in this study. First, this study was retrospective, and there may be patient selection bias or even radiomics feature selection bias. Prospective multicenter studies are needed to validate the preliminary results of the current study. Secondly, several studies have shown the correlation between the genetic characteristics of patients and LNM, which is an area currently lacking in this study (29,30). In the future, relevant prediction models combining radiomics and genetic characteristics should be established to improve the prediction performance of models. In addition, since the segmentation of ROI was mostly semi-automatic and not stable, the repeatability and objectivity still need to be improved. In the future, the ROI segmentation model based on the convolutional neural network could realize automatic contour delineation of deep learning.
Conclusions
This study suggests that the radiomics model exhibits superior and more consistent predictive performance compared to the clinical models. It proves valuable in predicting the risk of LNM in patients with clinical stage I LUAD. This model enables doctors to more accurately assess the likelihood of LNM in early-stage LUAD patients, facilitating a more personalized treatment strategy.
Acknowledgments
Funding: This study was supported by
Footnote
Reporting Checklist: The authors have completed the TRIPOD reporting checklist. Available at https://tlcr.amegroups.com/article/view/10.21037/tlcr-24-668/rc
Data Sharing Statement: Available at https://tlcr.amegroups.com/article/view/10.21037/tlcr-24-668/dss
Peer Review File: Available at https://tlcr.amegroups.com/article/view/10.21037/tlcr-24-668/prf
Conflicts of Interest: All authors have completed the ICMJE uniform disclosure form (available at https://tlcr.amegroups.com/article/view/10.21037/tlcr-24-668/coif). The authors have no conflicts of interest to declare.
Ethical Statement: The authors are accountable for all aspects of the work in ensuring that questions related to the accuracy or integrity of any part of the work are appropriately investigated and resolved. The study was approved by the Institutional Review Board of the Cancer Hospital, Chinese Academy of Medical Sciences (No. 22/492-3694). The other two participating hospitals were informed and agreed with the study. Since this is a retrospective study, the requirement for informed consent was waived.
Open Access Statement: This is an Open Access article distributed in accordance with the Creative Commons Attribution-NonCommercial-NoDerivs 4.0 International License (CC BY-NC-ND 4.0), which permits the non-commercial replication and distribution of the article with the strict proviso that no changes or edits are made and the original work is properly cited (including links to both the formal publication through the relevant DOI and the license). See: https://creativecommons.org/licenses/by-nc-nd/4.0/.
References
- Siegel RL, Miller KD, Fuchs HE, et al. Cancer Statistics, 2021. CA Cancer J Clin 2021;71:7-33. [Crossref] [PubMed]
- Duma N, Santana-Davila R, Molina JR. Non-Small Cell Lung Cancer: Epidemiology, Screening, Diagnosis, and Treatment. Mayo Clin Proc 2019;94:1623-40. [Crossref] [PubMed]
- Bray F, Laversanne M, Sung H, et al. Global cancer statistics 2022: GLOBOCAN estimates of incidence and mortality worldwide for 36 cancers in 185 countries. CA Cancer J Clin 2024;74:229-63. [Crossref] [PubMed]
- Goldstraw P, Chansky K, Crowley J, et al. The IASLC Lung Cancer Staging Project: Proposals for Revision of the TNM Stage Groupings in the Forthcoming (Eighth) Edition of the TNM Classification for Lung Cancer. J Thorac Oncol 2016;11:39-51. [Crossref] [PubMed]
- Maniwa T, Okami J, Miyoshi T, et al. Lymph node dissection in small peripheral lung cancer: Supplemental analysis of JCOG0802/WJOG4607L. J Thorac Cardiovasc Surg 2024;168:674-683.e1. [Crossref] [PubMed]
- National Lung Screening Trial Research Team. Results of initial low-dose computed tomographic screening for lung cancer. N Engl J Med 2013;368:1980-91. [Crossref] [PubMed]
- Jiang C, Zhang Y, Fu F, et al. A Shift in Paradigm: Selective Lymph Node Dissection for Minimizing Oversurgery in Early Stage Lung Cancer. J Thorac Oncol 2024;19:25-35. [Crossref] [PubMed]
- Qiao J, Zhang X, Du M, et al. (18)F-FDG PET/CT radiomics nomogram for predicting occult lymph node metastasis of non-small cell lung cancer. Front Oncol 2022;12:974934. [Crossref] [PubMed]
- Li Z, Pan C, Xu W, et al. Distinct impacts of radiological appearance on lymph node metastasis and prognosis based on solid size in clinical T1 non-small cell lung cancer. Respir Res 2024;25:96. [Crossref] [PubMed]
- Deng J, Zhong Y, Wang T, et al. Lung cancer with PET/CT-defined occult nodal metastasis yields favourable prognosis and benefits from adjuvant therapy: a multicentre study. Eur J Nucl Med Mol Imaging 2022;49:2414-24. [Crossref] [PubMed]
- He H, Hu W, Yi C, et al. Impact of imaging features on selecting limited lymph node resection for cT1N0M0 lung cancer. J Thorac Dis 2024;16:5138-51. [Crossref] [PubMed]
- Rogasch JM, Apostolova I, Steffen IG, et al. Standardized visual reading of F18-FDG-PET in patients with non-small cell lung cancer scheduled for preoperative thoracic lymph node staging. Eur J Radiol 2016;85:1345-50. [Crossref] [PubMed]
- Schmidt-Hansen M, Baldwin DR, Hasler E, et al. PET-CT for assessing mediastinal lymph node involvement in patients with suspected resectable non-small cell lung cancer. Cochrane Database Syst Rev 2014;2014:CD009519. [Crossref] [PubMed]
- Lambin P, Leijenaar RTH, Deist TM, et al. Radiomics: the bridge between medical imaging and personalized medicine. Nat Rev Clin Oncol 2017;14:749-62. [Crossref] [PubMed]
- Lubner MG, Smith AD, Sandrasegaran K, et al. CT Texture Analysis: Definitions, Applications, Biologic Correlates, and Challenges. Radiographics 2017;37:1483-503. [Crossref] [PubMed]
- Wang X, Zhao X, Li Q, et al. Can peritumoral radiomics increase the efficiency of the prediction for lymph node metastasis in clinical stage T1 lung adenocarcinoma on CT? Eur Radiol 2019;29:6049-58. [Crossref] [PubMed]
- Zhang J, Hao L, Qi M, et al. Radiomics nomogram for preoperative differentiation of pulmonary mucinous adenocarcinoma from tuberculoma in solitary pulmonary solid nodules. BMC Cancer 2023;23:261. [Crossref] [PubMed]
- Wu G, Woodruff HC, Shen J, et al. Diagnosis of Invasive Lung Adenocarcinoma Based on Chest CT Radiomic Features of Part-Solid Pulmonary Nodules: A Multicenter Study. Radiology 2020;297:451-8. [Crossref] [PubMed]
- Zhang Y, Deng C, Zheng Q, et al. Selective Mediastinal Lymph Node Dissection Strategy for Clinical T1N0 Invasive Lung Cancer: A Prospective, Multicenter, Clinical Trial. J Thorac Oncol 2023;18:931-9. [Crossref] [PubMed]
- Mimae T, Miyata Y, Tsubokawa N, et al. Omitting Lymph Node Dissection for Small Ground-Glass Opacity-Dominant Tumors. Ann Thorac Surg 2025;119:192-8. [Crossref] [PubMed]
- Yotsukura M, Asamura H, Motoi N, et al. Long-Term Prognosis of Patients With Resected Adenocarcinoma In Situ and Minimally Invasive Adenocarcinoma of the Lung. J Thorac Oncol 2021;16:1312-20. [Crossref] [PubMed]
- Guo W, Lu T, Song Y, et al. Lymph node metastasis in early invasive lung adenocarcinoma: Prediction model establishment and validation based on genomic profiling and clinicopathologic characteristics. Cancer Med 2024;13:e70039. [Crossref] [PubMed]
- Liu MW, Zhang X, Wang YM, et al. A comparison of machine learning methods for radiomics modeling in prediction of occult lymph node metastasis in clinical stage IA lung adenocarcinoma patients. J Thorac Dis 2024;16:1765-76. [Crossref] [PubMed]
- van Timmeren JE, Cester D, Tanadini-Lang S, et al. Radiomics in medical imaging—“how-to” guide and critical reflection. Insights into Imaging 2020;11. [Crossref] [PubMed]
- Lambin P, Rios-Velazquez E, Leijenaar R, et al. Radiomics: extracting more information from medical images using advanced feature analysis. Eur J Cancer 2012;48:441-6. [Crossref] [PubMed]
- Kan Y, Dong D, Zhang Y, et al. Radiomic signature as a predictive factor for lymph node metastasis in early-stage cervical cancer. Journal of Magnetic Resonance Imaging 2018;49:304-10. [Crossref] [PubMed]
- Yang M, She Y, Deng J, et al. CT-based radiomics signature for the stratification of N2 disease risk in clinical stage I lung adenocarcinoma. Transl Lung Cancer Res 2019;8:876-85. [Crossref] [PubMed]
- Zhong Y, Yuan M, Zhang T, et al. Radiomics Approach to Prediction of Occult Mediastinal Lymph Node Metastasis of Lung Adenocarcinoma. AJR Am J Roentgenol 2018;211:109-13. [Crossref] [PubMed]
- Seto K, Kuroda H, Yoshida T, et al. Higher frequency of occult lymph node metastasis in clinical N0 pulmonary adenocarcinoma with <em>ALK</em> rearrangement. Cancer Management and Research 2018;10:2117-24. [Crossref] [PubMed]
- Meng W, Ye Z, Cui R, et al. MicroRNA-31 predicts the presence of lymph node metastases and survival in patients with lung adenocarcinoma. Clin Cancer Res 2013;19:5423-33. [Crossref] [PubMed]