Effectiveness of artificial intelligence for detecting operable lung cancer on chest radiographs
Highlight box
Key findings
• Artificial intelligence (AI) could be effective for detecting operable lung cancer using chest radiographs.
• Lung nodules in the upper zone and peripheral region were more detectable by AI.
• For lesions with diameter >4 cm, lesion centrality did not affect AI detection.
What is known and what is new?
• Previous studies have shown that using AI in chest radiograph analysis improves the efficiency and accuracy of lung nodule detection.
• This study provides insights into the effectiveness of AI for nodule detection in chest radiographs specifically for surgically operable early-stage lung cancer, along with a detailed AI analysis based on lung nodule location and size.
What is the implication, and what should change now?
• The findings suggest that while AI can enhance detection rates in certain lung regions, further refinement of AI algorithms is needed to improve detection in central and lower lung zones; integrating AI into clinical practice may assist in earlier and more accurate lung cancer detection.
IntroductionOther Section
Lung cancer is the leading cause of cancer-related morbidity and mortality worldwide with 1.80 million deaths documented by the World Health Organization in 2020 (1). One of the main reasons for the high mortality rate associated with lung cancer is difficulty with early detection. Although large-scale clinical studies have demonstrated that screening of high-risk groups with low-dose chest computed tomography (CT) can enhance lung cancer detection and reduce mortality rates (2), the application of this screening method is somewhat restricted due to its high cost, need for specialized equipment, concerns about radiation exposure, and difficulty in generalization it to individuals with low risk of tobacco exposure. On the other hand, chest radiograph (CXR) is comparatively easily accessible, inexpensive, simple to perform; it involves low radiation exposure and remains the first-line investigation for lung lesions in primary care clinics. However, the detection of early-stage lung cancer using CXR is challenging. This difficulty primarily stems from the fact that early-stage tumors are often small and manifest as subtle, indistinct abnormalities that can easily blend with surrounding anatomical structures. Additionally, non-solid early lung cancers may not be clearly visible on standard CXR (3,4).
As artificial intelligence (AI)-based approaches for lesion detection on CXR have increased, attempts to utilize AI to detect lung cancer have attracted clinical interest (5). Several studies have demonstrated the benefits of AI for malignant lung nodule detection on CXR (6,7), in terms of enhancing the diagnostic performance of radiologists, reducing the workload of screening healthy populations with low prevalence of lung cancer (8), and reducing worries about missed lung cancer due to inexperience of early-career radiologists or unexpected situations (9-11). The detection of lung cancer at an early, surgically operable stage is clinically important because it is associated with favorable patient outcomes. However, there is lack of evidence regarding the detectability of operable lung cancers on CXR using AI. In this study, we aimed to investigate the effectiveness and capability of AI-based CXR analysis in detecting malignant lung nodules in patients who subsequently underwent lung cancer surgery. We present this article in accordance with the STARD reporting checklist (available at https://tlcr.amegroups.com/article/view/10.21037/tlcr-24-745/rc).
MethodsOther Section
Patients and clinical data
Patients who underwent surgery for lung cancer at two referral hospitals (Severance Hospital and Yongin Severance Hospital) between March 2020 and February 2021 were retrospectively included in the study (Figure 1). Detailed medical records of baseline characteristics, pathological diagnoses, and radiological results were retrospectively reviewed. Lung cancer stage was assessed according to the 8th edition of TNM classification (12).
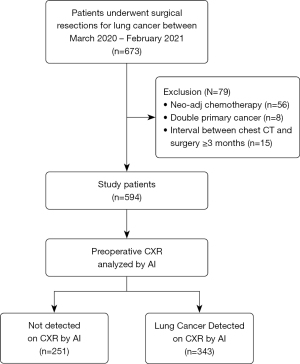
AI-based CXR analysis
CXRs obtained immediately before lung cancer surgery or biopsy were used for the AI analysis. Commercially available AI-based lesion detection software (Lunit INSIGHT for Chest Radiography, version 3.1.2, Lunit Inc., Republic of Korea) was used to analyze all CXRs in the posteroanterior or anteroposterior views. The software can detect nodules (Ndl), consolidation (Csn), pneumothorax (Ptx), pleural effusion (PEf), cardiomegaly (Cm), fibrosis (Fib), pneumoperitoneum (Ppm), and atelectasis (Atl) when it exceeds the vendor-recommended threshold of 15%, validated in previous research (13-15). The AI abnormality score represents the probability of the presence of suspicious areas for chest abnormalities, usually provided as a percentage. This software displays a contour map of the lesion location, abnormality score, and abbreviated name of the lesion detected on CXR (Figure 2A). If the score for any lesion type is below this threshold (less than 15%), the CXR is labeled as normal, with no region of interest (ROI) shown. We retrospectively extracted each abnormality score and lesion type from the AI server by uploading the digital imaging and communications in medicine (DICOM) images of the CXR to the server. AI detection of lung cancer was defined as AI-determined abnormality score above 15% at the location of known cancer lesion on CXR. We analyzed which lesion types were detected in the cancer region and the abnormality score of each. Total abnormality score for each lung cancer was defined as the highest score among the included lesions. To assess whether AI correctly detected the location of known lung cancer seen on CT and pathological findings, all CXRs and AI results were reviewed by four readers: two experienced radiologists (H.J.S., Y.J.S.) and two pulmonologists (S.H.K., E.H.L.) who were not blinded to the available information, such as the cancer’s presence and location. The location and type of lesions detected by the AI software were assessed accordingly by readers when known lung cancers were visible on CXR.
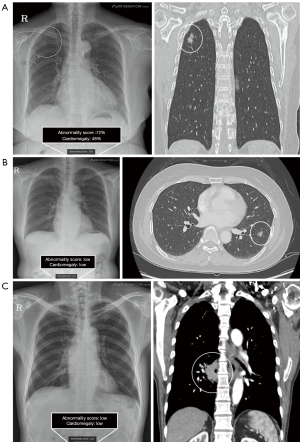
CT image acquisition and analysis
All patients underwent chest CT preoperatively using one of the following multidetector row scanners: Sensation 64, Somatom Definition Flash, Somatom Force, or Somatom Definition AS+ (Siemens Healthineers); Discovery CT750 HD, Revolution EVO, Revolution CT, or LightSpeed VCT (GE Healthcare); or iCT 256 (Philips Healthcare). Detailed imaging parameters are described in previous publications (16,17). For the assessment of lesion size, type, location, and centrality, preoperative chest CT images were analyzed as references. Six reviewers (above 4 readers and two additional thoracic radiologists (N.Y.K. and K.N.) participated in the CT image analysis with the assistance of AI-based computer-aided detection (CAD) software (CT AI-CAD) for the detection of lung nodules (AVIEW LCS, Coreline Soft, Seoul, Republic of Korea), with 70–150 cases per reader. The reviewers had information about the confirmed lung cancer when reviewing the CT AI-CAD results. They reviewed the AI-CAD results and corrected the size, type, and location of the CAD-detected malignant nodules if needed. When the CT AI-CAD could not detect the malignant nodule, the reviewers drew the contour of the nodule using semi-automated or manual methods. In addition, the centrality of the malignant nodule was assessed according to the definition proposed by a previous study (18). If multiple nodules were detected by the CT AI-CAD, the reviewers approved only one lesion per patient and deleted nodules other than those confirmed as lung cancer. The resulting data were considered the reference standard for nodule information on CT, including the size, type, and location of the lesions.
Statistical analysis
We used the R program (version 4.4.0, Foundation for Statistical Computing, Vienna, Austria; packages: survival, rms, compareC, and pec) for statistical analysis. Patients’ demographics were compared using t-test for continuous variables after the normality test, and Chi-squared test for categorical variables. To analyze the relationship between lung cancer size and AI abnormality score for peripheral and central lesions, we used the ggplot2 library, which facilitated the generation of plots to compare scoring trends across different nodule locations and sizes. Univariable and multivariable logistic regression analysis was conducted to investigate the factors that influenced the detection of lung cancer using AI. In the multivariable analysis, variables that had a P value less than 0.05 in the univariable analysis were included, along with variables of clinical importance related to cancer location. In the subgroup analysis, we utilized the forestploter library to generate forest plots. A P value <0.05 was considered significant for all analyses.
Ethical statement
This study was performed in accordance with the Declaration of Helsinki (as revised in 2013) and was approved by the institutional review board (IRB) of Yongin Severance hospital (approval No. 9-2021-0192). The requirement for informed consent was waived by the IRB of Yongin Severance Hospital because of the retrospective nature of the study and use of anonymized clinical data.
ResultsOther Section
Study patients
Between March 2020 and February 2021, 673 patients underwent surgical resection of lung cancer in the two study centers. Among them, 79 patients were excluded because they had received neo-adjuvant chemotherapy (n=56), had primary cancers other than lung cancer (n=8), or had a chest CT scan conducted ≥3 months prior to surgery (n=15). Therefore, 594 patients were included in the final analysis. A flowchart of the patient inclusion process is shown in Figure 1.
AI detection of lung cancer on CXR
Table 1 shows a comparison of patients’ demographics according to AI detection status. Among the 594 cases of lung cancer [male:female =305:289; median age, 65 years; interquartile range (IQR), 58.8–72 years], adenocarcinoma was the predominant histological type, accounting for 87.9% (522/594) of the cases. In preoperative CXRs, AI detected 57.7% (343 out of 594) of lung cancers. The detection rates of AI varied with the lung cancer stage: 42.5% for stage IA, 86.3% for stage IB, and 90.9% for stages II–III. The AI-detected group had a higher median age (66 vs. 64 years, P=0.005) and higher proportions of male patients and ever-smokers. When we used CT results as a reference, the AI-detected lesions tended to be larger in total and solid diameters and to be of a solid nodule type than those that were not detected by AI (P<0.001). According to the lesion type on CT, 8.3%, 46.8%, and 77.3% of nonsolid, part-solid, and solid nodules, respectively, were detected by AI. Regarding cancer size, for stage IA1 (≤1 cm), the detection rate by AI was 13.4%. For IA2, where the size exceeded 1 cm, the detection rate increased to 60.3%, and for lesions exceeding 2 cm in stage IA2, the results showed greater than 80% detection using AI. The AI abnormality scores were all significantly higher in the AI-detected cases.
Table 1
Characteristics | Total (n=594) | AI-detection (n=343) | No AI-detection (n=251) | P value |
---|---|---|---|---|
Age, years | 65 (58.8–72) | 66 (60–73) | 64 (57.5–70) | 0.005 |
Gender | ||||
Male | 305 | 191 (62.6) | 114 (37.4) | 0.02 |
Female | 289 | 152 (52.6) | 137 (47.4) | |
BMI, (kg/m2) | 24.0±2.9 | 24.0±2.9 | 24.1±3.0 | 0.78 |
Smoking status | ||||
Never smoker | 338 | 177 (52.4) | 161 (47.6) | 0.003 |
Ever smoker | 256 | 166 (64.8) | 90 (35.2) | |
Subtype | ||||
Adenocarcinoma | 522 | 286 (54.8) | 236 (45.2) | 0.001 |
Squamous cell carcinoma | 54 | 44 (81.5) | 10 (18.5) | |
Others | 18 | 13 (72.2) | 5 (27.8) | |
Pathologic stage | ||||
IA | 400 | 170 (42.5) | 230 (57.5) | <0.001 |
IA1 (≤1 cm) | 187 | 25 (13.4) | 162 (86.6) | |
IA2 (>1 cm, ≤2 cm) | 131 | 79 (60.3) | 52 (39.7) | |
IA3 (>2 cm, ≤3 cm) | 82 | 66 (80.5) | 16 (19.5) | |
IB | 73 | 63 (86.3) | 10 (13.7) | |
II | 76 | 69 (90.8) | 7 (9.2) | |
III | 45 | 41 (91.1) | 4 (8.9) | |
Lesion type | ||||
Non-solid | 72 | 6 (8.3) | 66 (91.7) | 0.001 |
Part-solid | 218 | 102 (46.8) | 116 (53.2) | |
Solid | 304 | 235 (77.3) | 69 (22.7) | |
Lesion size | ||||
Lesion diameter (solid), mm | 20.5±16.3 | 28.7±15.6 | 9.2±8.6 | <0.001 |
Lesion diameter (total), mm | 26.6±13.8 | 32.4±14.3 | 18.6±7.7 | <0.001 |
Lesion volume (solid), mm3 | 7,770±20,696 | 12,859±26,049 | 816±1,907 | <0.001 |
Lesion volume (total), mm3 | 9,830±21,182 | 15,144±26,526 | 2,570±3,160 | <0.001 |
Location | ||||
R_Upper | 171 | 102 (59.6) | 69 (40.4) | 0.48 |
R_Middle | 48 | 30 (62.5) | 18 (37.5) | |
R_Lower | 164 | 86 (52.4) | 78 (47.6) | |
L_Upper | 130 | 80 (61.5) | 50 (38.5) | |
L_Lower | 81 | 45 (55.6) | 36 (44.4) | |
Centrality | ||||
Central | 71 | 44 (62.0) | 27 (38.0) | 0.52 |
Peripheral | 523 | 299 (57.2) | 224 (42.8) | |
AI abnormality score on CXR | ||||
Nodule score | 43.7±37.9 | 69.9±27.7 | 8.57±13.2 | <0.001 |
Consolidation score | 13.0±22.3 | 20.1±26.0 | 3.38±9.6 | <0.001 |
Fibrosis score | 14.6±25.1 | 19.2±26.8 | 8.39±21.0 | <0.001 |
Atelectasis score | 5.89±15.4 | 6.94±16.7 | 4.46±13.5 | 0.046 |
Total abnormality score† | 45.0±37.8 | 71.8±25.6 | 8.57±13.2 | <0.001 |
Values are presented as medians with interquartile ranges, numbers, and numbers with percentages or mean ± SD. †, total abnormality score for each lung cancer was defined as the highest score among the included lesions. AI, artificial intelligence; CXR, chest radiograph; IQR, interquartile range; BMI, body mass index; SD, standard deviation; R, right; L, left.
A total of 399 lung cancers (67.2%) was considered visible on CXR, whereas 195 were not visible (Table S1). Among the CXR-visible lung cancers, 343 (86.0%) were detected correctly by AI, and the detected lesions were as follows: nodules (n=321), consolidations (n=97), fibrosis (n=52), and atelectasis (n=14). The remaining 56 cases were not detected by AI. Figure 2 shows examples of lung cancers detected and not detected by AI on CXR.
Factors that contribute to AI detection of lung cancer on CXRs
Table 2 presents the results of univariable and multivariable analysis of factors affecting the detection of lung cancer using AI on CXRs. In the univariable analysis, characteristics such as older age, male gender, ever-smoker, squamous histology, solid nodule rather than subsolid, as well as increased stage and size were shown to affect AI detection. However, in the multivariable analysis, significant effects on AI detection were observed when lung cancers were located in the upper zone [vs. mid & lower zone, odds ratio (OR): 2.78, 95% confidence interval (CI): 1.709–4.609, P<0.001], peripheral region (vs. central, OR: 4.59, 95% CI: 2.091–10.306, P<0.001), and as the nodule solid diameter (OR: 1.20, 95% CI: 1.167–1.247, P<0.001) increased. In the subgroup analysis using age (65 years) and solid diameter cutoff (16.3 mm), AI detection of lung cancer on CXR increased significantly in cases with solid compared to subsolid nodules, in addition to solid diameter, peripheral, and upper lung regions (Figure 3).
Table 2
Variable | Univariable analysis | Multivariable analysis | |||||
---|---|---|---|---|---|---|---|
OR | 95% CI | P value | Adjusted OR | 95% CI | P value | ||
Age | 1.02 | 1.005–1.039 | 0.009 | 1.00 | 0.975–1.023 | 0.93 | |
Gender (male) | 1.51 | 1.089–2.095 | 0.014 | 0.89 | 0.433–1.797 | 0.74 | |
Smoking (ever-smoker) | 1.68 | 1.202–2.342 | 0.002 | 0.94 | 0.445–1.964 | 0.86 | |
Histology | |||||||
Non-squamous | 1 | <0.001 | 1 | 0.69 | |||
Squamous | 3.55 | 1.748–7.194 | 1.23 | 0.452–3.554 | |||
Pathologic stage | |||||||
IA | 1 | – | – | – | |||
IB | 8.52 | 4.25–17.096 | <0.001 | – | – | – | |
II | 13.38 | 5.978–29.75 | <0.001 | – | – | – | |
III | 13.87 | 4.874–39.456 | <0.001 | – | – | – | |
Nodule type | |||||||
Subsolid (nonsolid + part-solid) | 1 | 1 | |||||
Solid | 5.74 | 4.027–8.258 | <0.001 | 1.13 | 0.643–1.97 | 0.67 | |
Nodule location† | |||||||
Mid & lower zone | 1 | 0.149 | 1 | <0.001 | |||
Upper zone | 1.27 | 0.918–1.763 | 2.78 | 1.709–4.609 | |||
Centrality | |||||||
Central | 1 | 0.443 | 1 | <0.001 | |||
Peripheral | 0.82 | 0.492–1.363 | 4.59 | 2.091–10.306 | |||
Nodule diameter (solid lesion, mm) | 1.18 | 1.146–1.207 | <0.001 | 1.20 | 1.167–1.247 | <0.001 | |
Nodule diameter (total lesion, mm) | 1.15 | 1.124–1.184 | <0.001 | – | – | – |
†, upper zone refers to the right upper lobe and left upper division, whereas the mid & lower zone refers to the remaining lobes (right middle lobe, left lingular division, right lower lobe, and left lower lobe). AI, artificial intelligence; CXR, chest radiograph; OR, odds ratio; CI, confidence interval.
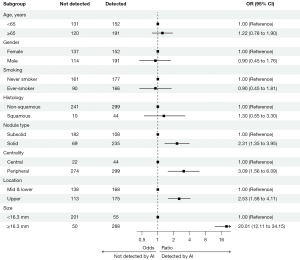
Differences in AI detection on CXR between central and peripheral regions by stage
Figure 4 indicates that malignant lesions located in the peripheral regions tended to have higher AI abnormality scores even when their sizes were small, suggesting a higher likelihood of detection by AI. Conversely, as lesion size approached or exceeded approximately 40 mm according to the stage, the disparity in the AI abnormality scores between the peripheral and central lesions diminished, indicating a convergence in nodule scores for larger lesions regardless of their location. When further analysis was conducted by dividing the stage into pathologic stage I and stage II or higher (using 4 cm tumor size cutoff), it was found that in stage I, AI detection of lung cancer in CXR was significantly higher in peripheral region at 50.7% compared to 31.4% in the central region (P=0.043) (Figure 5). On the other hand, in stage II or higher, there was no significant difference in AI detection between the central and peripheral regions, with both showing an AI detection rate of over 90%.
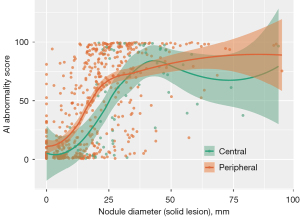
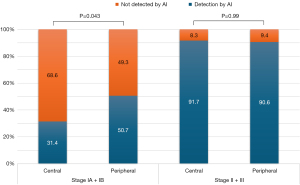
DiscussionOther Section
This study investigated the effectiveness of AI-based CXR analysis in detecting malignant lung nodules in patients who underwent lung cancer surgery. Our results showed that 57.7% (343 out of 594) of operable lung cancers were detected on CXR using AI, and 86% of CXR-visible lung cancers were detected with AI. Although AI identified only 42.5% of stage IA cancers, the detection rate increased to over 60% and 80% for stages IA2 and IA3, respectively. Interestingly, when the tumor size was less than 4 cm pathologic (stage IA and IB), AI detection rates on CXR were significantly higher in the peripheral region compared to central region cancers, demonstrating that AI tool in CXR is more effective in detecting peripheral lung cancer even when the tumors are smaller (Figures 4,5).
Recent meta-analyses have reported the sensitivity of lung cancer detection on CXR to be 77–80% (19). Literature suggests that CXR fails to identify lung cancer in approximately 20–25% of cases (3). However, these studies included patients with advanced lung cancer and those who were symptomatic. Studies on the sensitivity of CXR and its role in detecting surgically operable early-stage lung cancers are lacking; hence, we analyzed the utility of AI for the detection of malignant lesions in patients with operable lung cancer. As is already known, the AI-detectability of nodules on CXR is lower for smaller nodules, those at an early stage, and those with less solid portions, such as non-solid or part-solid nodules (20,21). Previous studies have reported that lung cancer is likely to be missed when located in areas with overlying ribs or in the sub-diaphragmatic space and hilar regions (19,22). Similarly, our study found that AI detection rate was significantly lower for tumors located in the central region and mid, lower lung zone (Table 2). Given that the incidence of lung cancer is significantly higher in the upper lobes (23), the fact that AI demonstrates strong detection capabilities in these regions is a significant advantage. On the other hand, the lower detection rates in the mid and lower lung regions can be explained by the anatomical and imaging challenges inherent to these areas. Structures such as the diaphragm and heart, along with the overlap of abdominal contents, can obscure smaller lesions, making detection more difficult (22). These findings underscore the importance of continued refinement of AI algorithms to improve detection rates across these more challenging regions.
In this study, experienced readers assessed that operable lung cancer was visible in 67.2% (399 out of 594) of patients from preoperative CXRs. Our retrospective analysis involved radiologists and pulmonologists who reviewed CXRs with prior knowledge of each lung cancer’s presence and location for the assessment of visibility on CXR, allowing for maximal sensitivity and resulting in a 67.2% of visibility. Of these visible cases, AI successfully detected 86% (343 of 399) at the precise cancer location. Previous studies have shown that the sensitivity of lung nodule detection on CXR by doctors varies from 36% to 84% (24-26). Studies analyzing AI performance on CXR have reported more consistent sensitivities, ranging from 0.79 to 0.91 (13,27). However, it should be noted that this study did not compare AI’s effectiveness to cases missed by radiologists in real-world settings without AI assistance, nor did it assess AI’s performance in a blinded manner. These limitations, along with the retrospective design, mean that our study cannot definitively conclude AI’s incremental benefit over traditional radiologist interpretation. In real-world clinical settings, CXRs are often obtained for various reasons across outpatient, emergency, and primary care settings, where immediate radiological interpretation is frequently unavailable, placing the responsibility of CXR assessment on the attending physician (20). A previous study has reported that approximately 20% of patients with lung cancer had previous positive CXR findings that were missed at that time (21). Additionally, a recent randomized controlled trial found that radiologists who used AI software on CXR had a significantly higher rate of malignant lung nodule detection than the group that did not use AI (28). Another study reported that AI assistance was particularly beneficial for junior radiologists with less experience, enhancing their detection accuracy (29).
Our study provides support for the potential of AI in identifying operable, CXR-visible lung cancers. By presenting detailed information on AI performance based on tumor characteristics—such as size, location, and nodule type—our findings offer foundational data that can serve as a reference for understanding AI’s capabilities in detecting operable lung cancer. Additionally, the findings emphasize the need for radiologists and clinicians to pay close attention to areas prone to missed detections, such as the mid and lower lung zones and central regions, where AI detection remains limited. However, it is also important to note that when the AI abnormality score exceeds the 15% cutoff, a considerable number of detected cases may represent benign diseases in addition to cancer (30). Furthermore, AI CXR has limitations in assessing lymph node involvement and metastasis to adjacent structures, underscoring its constraints as a definitive diagnostic tool for cancer. Therefore, an AI-detected abnormality on CXR should not be considered definitive for cancer diagnosis; rather, it serves as an indicator for further workup, such as CT, in a screening context.
This study has some limitations. First, it was a retrospective analysis conducted in only two institutions, which limits the sample size and generalizability of the findings. Additionally, while the AI demonstrated a relatively high detection rate for operable lung cancer, the study did not compare this with detection rates in real-life clinical settings without AI assistance, making it difficult to assess AI’s incremental contribution to reducing missed diagnoses. This ambiguity limits conclusions about potential clinical benefits for patient prognosis. Second, we used one commercial AI software to analyze the CXRs, which may impact generalizability. However, our choice was informed by the need to use commercial software that demonstrates high performance in order to facilitate repeatability of the study. Nonetheless, studies assessing the efficacy of AI in analyzing CXR, specifically for the detection of early lung cancer, are rare, which lends significance to this study. Our study demonstrated the detailed analysis of the characteristics of surgically treatable lung cancer detected through AI, using CT as a reference. To validate these findings, larger-scale, multi-institutional prospective studies are needed to further ascertain the effectiveness of AI in detecting early-stage lung cancer and improving patients’ outcomes.
ConclusionsOther Section
In conclusion, this study demonstrated that AI could detect a considerable number of patients with lung cancers at surgically operable stages on CXRs. It also showed that AI detection via CXR is more effective when the cancer lesion has a larger solid diameter and is located in the upper zone and peripheral region.
AcknowledgmentsOther Section
Funding: This study was supported by a faculty research grant of
FootnoteOther Section
Reporting Checklist: The authors have completed the STARD reporting checklist. Available at https://tlcr.amegroups.com/article/view/10.21037/tlcr-24-745/rc
Peer Review File: Available at https://tlcr.amegroups.com/article/view/10.21037/tlcr-24-745/prf
Data Sharing Statement: Available at https://tlcr.amegroups.com/article/view/10.21037/tlcr-24-745/dss
Conflicts of Interest: All authors have completed the ICMJE uniform disclosure form (available at https://tlcr.amegroups.com/article/view/10.21037/tlcr-24-745/coif). The authors have no conflicts of interest to declare.
Ethical Statement: The authors are accountable for all aspects of the work in ensuring that questions related to the accuracy or integrity of any part of the work are appropriately investigated and resolved. This study was performed in accordance with the Declaration of Helsinki (as revised in 2013) and was approved by the institutional review board (IRB) of Yongin Severance hospital (approval No: 9-2021-0192). The requirement for informed consent was waived by the IRB of Yongin Severance Hospital because of the retrospective nature of the study and use of anonymized clinical data.
Open Access Statement: This is an Open Access article distributed in accordance with the Creative Commons Attribution-NonCommercial-NoDerivs 4.0 International License (CC BY-NC-ND 4.0), which permits the non-commercial replication and distribution of the article with the strict proviso that no changes or edits are made and the original work is properly cited (including links to both the formal publication through the relevant DOI and the license). See: https://creativecommons.org/licenses/by-nc-nd/4.0/.
ReferencesOther Section
- WHO. WHO Fact Sheet—Cancer. 2021; Available online: https://www.who.int/news-room/fact-sheets/detail/cancer
- Sadate A, Occean BV, Beregi JP, et al. Systematic review and meta-analysis on the impact of lung cancer screening by low-dose computed tomography. Eur J Cancer 2020;134:107-14. [Crossref] [PubMed]
- Bradley SH, Bhartia BS, Callister ME, et al. Chest X-ray sensitivity and lung cancer outcomes: a retrospective observational study. Br J Gen Pract 2021;71:e862-8. [Crossref] [PubMed]
- Rampinelli C, Calloni SF, Minotti M, et al. Spectrum of early lung cancer presentation in low-dose screening CT: a pictorial review. Insights Imaging 2016;7:449-59. [Crossref] [PubMed]
- Armato SG 3rd. Deep Learning Demonstrates Potential for Lung Cancer Detection in Chest Radiography. Radiology 2020;297:697-8. [Crossref] [PubMed]
- Hwang SH, Shin HJ, Kim EK, et al. Clinical outcomes and actual consequence of lung nodules incidentally detected on chest radiographs by artificial intelligence. Sci Rep 2023;13:19732. [Crossref] [PubMed]
- Kwak SH, Kim EK, Kim MH, et al. Incidentally found resectable lung cancer with the usage of artificial intelligence on chest radiographs. PLoS One 2023;18:e0281690. [Crossref] [PubMed]
- Lee JH, Sun HY, Park S, et al. Performance of a Deep Learning Algorithm Compared with Radiologic Interpretation for Lung Cancer Detection on Chest Radiographs in a Health Screening Population. Radiology 2020;297:687-96. [Crossref] [PubMed]
- Yoo H, Lee SH, Arru CD, et al. AI-based improvement in lung cancer detection on chest radiographs: results of a multi-reader study in NLST dataset. Eur Radiol 2021;31:9664-74. [Crossref] [PubMed]
- Nam JG, Hwang EJ, Kim DS, et al. Undetected Lung Cancer at Posteroanterior Chest Radiography: Potential Role of a Deep Learning-based Detection Algorithm. Radiol Cardiothorac Imaging 2020;2:e190222. [Crossref] [PubMed]
- Ueda D, Yamamoto A, Shimazaki A, et al. Artificial intelligence-supported lung cancer detection by multi-institutional readers with multi-vendor chest radiographs: a retrospective clinical validation study. BMC Cancer 2021;21:1120. [Crossref] [PubMed]
- Goldstraw P, Chansky K, Crowley J, et al. The IASLC Lung Cancer Staging Project: Proposals for Revision of the TNM Stage Groupings in the Forthcoming (Eighth) Edition of the TNM Classification for Lung Cancer. J Thorac Oncol 2016;11:39-51. [Crossref] [PubMed]
- Nam JG, Park S, Hwang EJ, et al. Development and Validation of Deep Learning-based Automatic Detection Algorithm for Malignant Pulmonary Nodules on Chest Radiographs. Radiology 2019;290:218-28. [Crossref] [PubMed]
- Kim EY, Kim YJ, Choi WJ, et al. Concordance rate of radiologists and a commercialized deep-learning solution for chest X-ray: Real-world experience with a multicenter health screening cohort. PLoS One 2022;17:e0264383. [Crossref] [PubMed]
- Lee S, Shin HJ, Kim S, et al. Successful Implementation of an Artificial Intelligence-Based Computer-Aided Detection System for Chest Radiography in Daily Clinical Practice. Korean J Radiol 2022;23:847-52. [Crossref] [PubMed]
- Suh YJ, Han K, Kwon Y, et al. Computed Tomography Radiomics for Preoperative Prediction of Spread Through Air Spaces in the Early Stage of Surgically Resected Lung Adenocarcinomas. Yonsei Med J 2024;65:163-73. [Crossref] [PubMed]
- Kim SY, Suh YJ, Kim NY, et al. A Modified Length-Based Grading Method for Assessing Coronary Artery Calcium Severity on Non-Electrocardiogram-Gated Chest Computed Tomography: A Multiple-Observer Study. Korean J Radiol 2023;24:284-93. [Crossref] [PubMed]
- Casal RF, Sepesi B, Sagar AS, et al. Centrally located lung cancer and risk of occult nodal disease: an objective evaluation of multiple definitions of tumour centrality with dedicated imaging software. Eur Respir J 2019;53:1802220. [Crossref] [PubMed]
- Bradley SH, Abraham S, Callister ME, et al. Sensitivity of chest X-ray for detecting lung cancer in people presenting with symptoms: a systematic review. Br J Gen Pract 2019;69:e827-35. [Crossref] [PubMed]
- Hamanaka R, Oda M. Can Artificial Intelligence Replace Humans for Detecting Lung Tumors on Radiographs? An Examination of Resected Malignant Lung Tumors. J Pers Med 2024;14:164. [Crossref] [PubMed]
- Quekel LG, Kessels AG, Goei R, et al. Miss rate of lung cancer on the chest radiograph in clinical practice. Chest 1999;115:720-4. [Crossref] [PubMed]
- Shimazaki A, Ueda D, Choppin A, et al. Deep learning-based algorithm for lung cancer detection on chest radiographs using the segmentation method. Sci Rep 2022;12:727. [Crossref] [PubMed]
- Nilssen Y, Brustugun OT, Fjellbirkeland L, et al. Distribution and characteristics of malignant tumours by lung lobe. BMC Pulm Med 2024;24:106. [Crossref] [PubMed]
- Quekel LG, Kessels AG, Goei R, et al. Detection of lung cancer on the chest radiograph: a study on observer performance. Eur J Radiol 2001;39:111-6. [Crossref] [PubMed]
- Aberle DR, DeMello S, Berg CD, et al. Results of the two incidence screenings in the National Lung Screening Trial. N Engl J Med 2013;369:920-31. [Crossref] [PubMed]
- Lee KH, Goo JM, Park CM, et al. Computer-aided detection of malignant lung nodules on chest radiographs: effect on observers' performance. Korean J Radiol 2012;13:564-71. [Crossref] [PubMed]
- Takamatsu A, Ueno M, Yoshida K, et al. Performance of artificial intelligence-based software for the automatic detection of lung lesions on chest radiographs of patients with suspected lung cancer. Jpn J Radiol 2024;42:291-9. [Crossref] [PubMed]
- Nam JG, Hwang EJ, Kim J, et al. AI Improves Nodule Detection on Chest Radiographs in a Health Screening Population: A Randomized Controlled Trial. Radiology 2023;307:e221894. [Crossref] [PubMed]
- Homayounieh F, Digumarthy S, Ebrahimian S, et al. An Artificial Intelligence-Based Chest X-ray Model on Human Nodule Detection Accuracy From a Multicenter Study. JAMA Netw Open 2021;4:e2141096. [Crossref] [PubMed]
- Kwak SH, Kim KY, Choi JS, et al. Impact of AI-assisted CXR analysis in detecting incidental lung nodules and lung cancers in non-respiratory outpatient clinics. Front Med (Lausanne) 2024;11:1449537. [Crossref] [PubMed]