A novel optimization of cone beam CT frequency for lung radiation therapy based on an image-guided radiation therapy protocol and patient classification method
Highlight box
Key findings
• We have proposed a new patient classification approach in the context of an image-guided radiation therapy (IGRT) protocol with optimized cone beam computed tomography (CBCT) frequency for conventionally fractionated lung cancer radiation therapy. The first three CBCT helps predict patient eligibility of this IGRT protocol.
What is known and what is new?
• Daily CBCT-guided radiation treatment is wildly applied in clinic, however, it carries certain drawbacks, for example, adding additional imaging dose to the patient, yielding increased workload and longer treatment duration.
• The optimal frequency for CBCT remains a topic of debate. Many studies have investigated if less frequent CBCT could achieve comparable clinical objectives to daily CBCT.
• In this study, we propose an in-house IGRT scheme, daily CBCT for the first 3 fractions following weekly CBCT, along with daily kilovoltage (kV)-orthogonal imaging.
• In the meanwhile, we develop a patient classification method to identify patients suitable for this scheme.
What is the implication, and what should change now?
• There are couple ways can be used to implement the proposed classification method. One method is to use the script programmed in treatment planning system (TPS) system. Second method is to build an in-house application by physicists.
Introduction
Lung cancer continues to rank among the leading contributors to cancer-related mortality in the United States (1). External beam radiation therapy for lung cancer has evolved with multiple techniques such as intensity modulated radiation therapy (IMRT), volumetric modulated arc therapy (VMAT) and stereotactic body radiation therapy (SBRT). These techniques combined with, image-guided radiation therapy (IGRT) have significantly improved patient outcomes by reducing uncertainties in target volumes and minimizing normal tissue doses (2,3). Of importance when using these advanced targeting modalities is the verification of the prescribed margin relative to the minimization of geometric uncertainties (2,3).
While the two-dimensional (2D) orthogonal kilovoltage (kV) pair or megavoltage (MV) images are still considered a form of image guidance for pretreatment IGRT for lung cancer radiation treatment, there is a growing trend of CBCT-guided radiation treatment, particularly in lung cancer radiation therapy (4). This trend is attributed to CBCT’s exceptional ability to enhance soft tissue contrast within the lung, leading to its widespread application in correcting patient positioning during lung SBRT treatment and conventional fractionated lung radiotherapy (5-7). However, employing daily CBCT-guided radiation treatment carries certain drawbacks. One is additional imaging dose to the patient [approximately 3–6 centigray (cGy) per scan to soft tissue, even higher to bone], which increases the chance of a second malignant cancer (8,9). Furthermore, daily CBCT yields increased workload and longer treatment duration, the latter consequently cause more intrafraction patient motion (10).
The optimal frequency for CBCT remains a topic of debate. Many studies have investigated if less frequent CBCT imaging could achieve comparable clinical objectives to daily CBCT, particularly in terms of minimizing setup error and enhancing target coverage. Santoro proposes an offline review protocol that employs the mean deviation from the first 5 CBCT scans for effective correction of gross tumor volume (GTV)-based systematic errors (11). Conversely, a study opposes the adoption of weekly CBCT. Hogue et al. (12) findings indicate that relying solely on weekly CBCT may not be a suitable substitute for daily CBCT in IMRT treatment, as it reveals that 25% of the prescribed dose may not reach the planning target volume (PTV) with weekly CBCT alone (12). Evidently, neither daily CBCT nor weekly CBCT might always be the ideal frequency for CBCT applications (4).
In this study we propose an in-house IGRT scheme and develop a patient classification method to identify patients suitable for this scheme. Our in-house IGRT includes daily CBCT for the first 3 fractions (F3F) following weekly CBCT, along with daily kV-orthogonal imaging. This IGRT scheme is designed to reach a balance between daily and weekly CBCT. Our patient classification method identifies patient eligibility of this IGRT scheme with high sensitivity, specificities and accuracies (Figure 1). We present this article in accordance with the TRIPOD reporting checklist (available at https://tlcr.amegroups.com/article/view/10.21037/tlcr-24-606/rc).
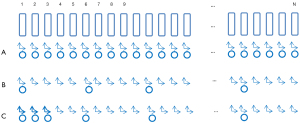
Methods
Patient cohort and treatment planning
This retrospective study was reviewed and approved by the Institutional Review Board (IRB) of University of Maryland School of Medicine (approval number: HP-00079915), and it was conducted in accordance with the Declaration of Helsinki (as revised in 2013). All patients’ individual consent for this retrospective analysis was waived. A total of 110 lung patients were involved for analysis. All patients were treated with conventionally fractionated radiation therapy for stage II–IV, non-small cell lung cancer (NSCLC) or small cell lung cancer (SCLC). Each patient underwent a four-dimensional computed tomography (4DCT) simulation (Big Bore, Philips Health Care, Best, Netherlands) with breathing recorded by Real-time Position Management System (RPM, Varian Medical Systems, Palo Alto, CA, USA). A conventional free breathing helical head first supine (HFS) CT scan for treatment planning purposes was also acquired. No special breathing control devices were applied for either scans. The 4DCT images were binned into 10 respiratory phases, with the phase at 0% labeled as the end-inspiration phase (EI), and the phase at 50% identified as the end-expiration phase (EE).
For the purpose of treatment planning, each patient 4DCT image was used to delineate the internal gross tumor volume (IGTV) throughout all 10 respiratory phases. Clinical target volume (CTV) expansions were based on histology and location. The PTV was then generated based on the CTV by adding setup margin (SM). VMAT technique was used for all 79 patients. The total dose is between 60–70 Gray (Gy), delivered by 30–35 fractions with a fractional dose of 1.8–2 Gy.
Among 110 patients, 31 were treated using daily CBCT, and the other 79 using our in-house IGRT scheme. These subsets are referred to as dataset31 and dataset79 throughout this work. In Table 1 the patient and tumor characteristics of the entire cohort are summarized, including whether subjects were enrolled in a protocol, and the CTV to PTV margin used in treatment planning. Patients number (No.) 1 to No. 79 on Table 1 are included in dataset79, and patients with No. 80 to No. 110 are included in dataset31.
Table 1
Patient No. | Age (years) | Sex | Stage | Tumor location | Initial PTV (cm3) | Treatment protocols | CTV to PTV margin (mm) |
---|---|---|---|---|---|---|---|
1 | 62 | M | T2bN2M0 | RLL | 719.7 | No | 5 |
2 | 65 | M | T1aN2M0 | LLL | 353.8 | No | 5 |
3 | 62 | M | T3N1M1b | RUL | 796.2 | No | 5 |
4 | 72 | F | N/A | RUL | 399.3 | No | 5 |
5 | 70 | M | N/A | Mediastinum | 642.5 | No | 5 |
6 | 63 | M | T1bN3M0 | LUL and mediastinum | 676.4 | NRG LU001 | 5 |
7 | 67 | M | T4N2M0 | LUL | 307.6 | No | 5 |
8 | 60 | M | T1aN2M0 | LUL and mediastinum | 383.7 | CALGB 30610 | 5 |
9 | 52 | F | T1b1N0M0 | RUL | 181.2 | No | 7 |
10 | 65 | F | T2N3M0 | LUL | 254.4 | No | 5 |
11 | 65 | F | T2N2M0 | RUL and mediastinal | 568.2 | NRG LU001 | 5 |
12 | 64 | M | T1aN2M0 | RLL and mediastinum | 241.8 | No | 5 |
13 | 55 | M | T4N2M1b | Rt lung and mediastinal | 1,824.0 | No | 5 |
14 | 78 | M | T4N2M0 | LUL | 539.9 | No | 5 |
15 | 57 | M | T1aN1 | RUL | 244.7 | No | 5 |
16 | 73 | F | T2bN2M0 | Lt lung | 363.1 | No | 5 |
17 | 65 | M | T4N0M0 | RUL | 887.2 | No | 5 |
18 | 61 | M | T4N0M0 | LUL | 392.4 | No | 5 |
19 | 76 | F | T3N2M0 | RLL | 344.2 | LU001 | 5 |
20 | 71 | F | N/A | LUL | 154.7 | No | 7 |
21 | 62 | M | T1aN2M0 | LUL | 343.9 | No | 5 |
22 | 52 | M | N/A | Lower media | 50.2 | No | 5 |
23 | 67 | M | T2bN2M0 | LLL | 784.6 | No | 5 |
24 | 57 | M | T2aN2Mx | RUL | 313.7 | No | 5 |
25 | 75 | F | T1bN3M0 | RLL | 1,005.0 | No | 5 |
26 | 49 | M | T3N2M0 | LLL | 192.1 | No | 5 |
27 | 49 | M | T3N2M0 | LLL | 192.1 | No | 5 |
28 | 70 | M | N/A | Rt hilar mass | 226.2 | No | 5 |
29 | 54 | M | T3N2M0 | Rt hilar mass | 832.0 | No | 7 |
30 | 67 | M | N/A | Rt hilum | 376.0 | No | 5 |
31 | 78 | M | T3N2M0 | LUL | 585.1 | No | 5 |
32 | 69 | M | T1aN0M0 | Lt lung | 116.3 | No | 5 |
33 | 66 | F | N/A | RLL | 116.3 | No | 5 |
34 | 67 | M | T3N1M0 | Rt lung | 587.3 | No | 5 |
35 | 78 | M | T3N2M0 | LUL | 585.1 | No | 5 |
36 | 78 | M | T1aN2M0 | Lt lung | 383.1 | No | 5 |
37 | 60 | F | T2aN2M0 | Rt lung | 228.9 | No | 5 |
38 | 66 | M | T2aN1M0 | RUL | 365.2 | No | 5 |
39 | 73 | M | T3N2M0 | Rt lung | 693.9 | No | 5 |
40 | 52 | M | T4N0M0 | RUL | 1,361.1 | No | 5 |
41 | 72 | M | T2N2M1 | RML | 256.4 | No | 5 |
42 | 72 | M | T4N1M0 | Lt lung | 784.5 | No | 5 |
43 | 56 | F | N/A | Rt lung | 377.2 | No | 5 |
44 | 71 | M | T3N2M1 | RUL | 364.0 | No | 5 |
45 | 51 | M | T3N2MX | Rt hilar mass | 524.1 | No | 5 |
46 | 54 | M | T1aN3M0 | RLL | 154.0 | No | 5 |
47 | 70 | M | T1aN1M0 | LUL | 226.0 | No | 5 |
48 | 65 | F | T1aN3M0 | Rt lung mass | 267.9 | No | 5 |
49 | 71 | F | T1bN2M0 | Rt lung mass | 189.2 | No | 3 |
50 | 66 | M | T1cN0M0 | RUL | 800.4 | No | 5 |
51 | 66 | F | N/A | RML | 63.3 | No | 3 |
52 | 68 | M | N/A | Rt lung | 280.4 | No | 6 |
53 | 76 | M | N/A | Lt hilum | 444.7 | No | 5 |
54 | 61 | F | T4N0M0 | Lung mass | 160.7 | No | 3 |
55 | 86 | M | T2N2M0 | RLL | 221.4 | No | 5 |
56 | 52 | M | T2N1M0 | Rt paratracheal LN | 131.1 | No | 5 |
57 | 50 | M | T3N0M0 | RUL | 334.2 | No | 5 |
58 | 48 | M | N/A | Media LN | 151.3 | No | 5 |
59 | 79 | M | T2aN2M0 | Rt lung | 264.0 | No | 5 |
60 | 65 | M | TXN2M0 | Lt lung tumor | 419.7 | No | 5 |
61 | 63 | M | N/A | Rt lung | 1,721.8 | No | 5 |
62 | 61 | F | T2aN2M0 | Lt lung | 434.5 | No | 5 |
63 | 59 | F | T1aN2M1b | Lt lung | 434.3 | No | 5 |
64 | 54 | F | N/A | Rt lung | 422.6 | No | 5 |
65 | 72 | M | N/A | Lt hilar mass and LN | 319.1 | No | 5 |
66 | 78 | M | N/A | Rt Lung | 153.3 | No | 4 |
67 | 66 | F | T2N3M1b | RLL | 589.0 | No | 4 |
68 | 75 | F | T2aN2M0 | Lt lung | 232.2 | No | 5 |
69 | 53 | F | N/A | Rt lung | 1,129.9 | No | 5 |
70 | 75 | M | N/A | Rt lung | 243.4 | No | 3 |
71 | 72 | M | T2N2M1 | RML, Rt hilum | 256.4 | No | 5 |
72 | 60 | M | N/A | Lt lung | 670.7 | No | 5 |
73 | 61 | M | N/A | LUL | 530.1 | No | 6 |
74 | 65 | F | N/A | Rt lung nodule | 234.1 | No | 5 |
75 | 53 | M | N/A | RLL | 825.2 | No | 4 |
76 | 39 | F | N/A | Rt lung mass | 900.0 | No | 3 |
77 | 71 | M | N/A | Rt mainstem bronchus | 397.7 | No | 7 |
78 | 61 | M | N/A | LUL | 1,107.4 | No | 6 |
79 | 56 | M | N/A | Lung tumor | 209.0 | No | 6 |
80 | 63 | M | T2aN2M0 | RUL | 153.5 | No | 5 |
81 | 58 | M | T3N2M1 | RUL | 965.7 | No | 5 |
82 | 60 | F | T1N3M0 | LLL | 71.5 | No | 5 |
83 | 66 | F | T1bN0M0 | Lt lung | 32.1 | No | 5 |
84 | 59 | F | T1N1 | RUL | 808.2 | BIO300 | 5 |
85 | 52 | M | N/A | Lower media | 50.2 | No | 5 |
86 | 64 | M | T1bN2M0 | Left hilum | 288.4 | No | 5 |
87 | 50 | F | T3N2M0 | LLL | 996.3 | LU001 | 6 |
88 | 71 | F | T1aN0M0 | LUL | 246.8 | No | 5 |
89 | 55 | M | T1N2 | RLL | 239.5 | No | 5 |
90 | 65 | F | T2N3M0 | LUL | 254.4 | No | 5 |
91 | 75 | F | T1N0M0 | RUL | 19.1 | No | 2 |
92 | 75 | M | T3N1M0 | RUL | 408.8 | No | 5 |
93 | 53 | M | T4N2M0 | RUL | 951.3 | No | 5 |
94 | 72 | M | T4N2M0 | Mediastinum | 496.3 | No | 5 |
95 | 70 | M | T2N0M0 | Rt lung | 1,139.7 | No | 5 |
96 | 70 | M | T2N1M0 | Rt bronchial stump | 240.2 | No | 5 |
97 | 70 | M | T1N0M0 | LUL | 412.9 | No | 5 |
98 | 64 | F | T3N2M0 | Rt lung | 546.5 | No | 5 |
99 | 65 | F | T4N2M0 | Lt lung | 977.4 | No | 5 |
100 | 79 | F | T1N3M0 | Mediastinum | 601.4 | No | 5 |
101 | 53 | F | T1N3M0 | LUL | 284.5 | No | 5 |
102 | 52 | M | T3N0M0 | LUL | 464.4 | No | 5 |
103 | 63 | M | T1bN3M0 | LUL | 676.4 | NRG LU001 | 5 |
104 | 65 | F | T2N2M0 | RUL | 568.2 | NRG LU001 | 5 |
105 | 63 | F | T3N3M1 | Rt lung | 736.9 | No | 5 |
106 | 75 | F | T2N2 | RUL | 378.8 | No | 4 |
107 | 69 | M | T4N0M0 | Rt lung | 160.4 | No | 4 |
108 | 81 | M | N/A | Rt lung | 869.8 | No | 5 |
109 | 70 | F | T2bN2M1 | Rt hilum | 212.8 | No | 5 |
110 | 59 | M | T2bN2M0 | LLL | 645.1 | No | 5 |
N/A, not applicable; PTV, planning target volume; CTV, clinical target volume; M, male; F, female; RLL, right lower lobe; LLL, left lower lobe; LML, left middle lobe; LUL, left upper lobe; RML, right middle lobe; RUL, right upper lobe; Rt, right; Lt, left.
Patient treatment setup and IGRT
A comprehensive representation of the procedural sequence on each treatment day, inclusive of CBCT imaging or not, is depicted in Figure 2. The patient is first aligned conventionally by skin-marks placed at simulation to room lasers. Subsequently, bony alignment is performed based on kV-orthogonal imaging. In cases where a CBCT scan is not scheduled, treatment is initiated following kV-based couch correction. Conversely, if a CBCT scan is scheduled, it follows the kV procedure. Alignment to soft tissue, including IGTV and PTV, is then executed through the CBCT scan. If necessary, an optional second CBCT scan is employed to validate any shifts identified in the initial CBCT scan. For dataset31 and dataset79, there are 984 and 632 CBCT scans (8 CBCTs per patient in dataset79) involved in this study.
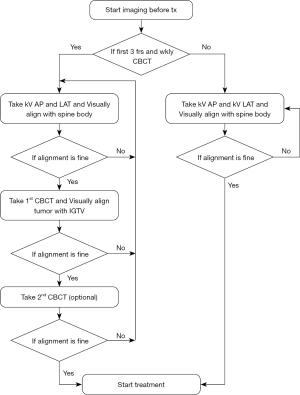
In order to show that the 8 fractions of CBCT can be used to create the same ground truth in the current study as that in the daily CBCT, the distribution of dataset79 and the distribution of dataset31 are compared using histograms on three directions, e.g., vertical [anterior-posterior (AP)], longitudinal [superior-inferior (SI)] and lateral [left-right (LR)] direction. These histograms overlap well visually; three-dimensional (3D) Gaussian mixture models are fitted and quantitatively compared so that we are confident to use dataset79 to create the ground truth of patient classification (Figure 3).
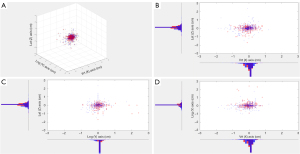
Patient classification
Our study recognizes that while the means of setup error from kV-CBCT remain relatively modest across all three dimensions for the majority of the patients, their standard deviations (SDs) are notably larger. This dispersion in distribution implies the potential existence of multiple distinct patient groups within the patient population. The 79 patients are classified into two distinct groups, iGroup and eGroup (Table 2). We define “kV-CBCT mismatch” and “kV-CBCT match” as follows: “kV-CBCT mismatch” happens when a CBCT based couch correction after kV-orthogonal is greater than or equal to a predetermined setup threshold ∆. “kV-CBCT match” happens when a CBCT based couch correction after kV-orthogonal is less than ∆. In this study, we evaluated three predetermined ∆ values (3, 4 and 5 mm) that are used in the clinic. The ground truth of eGroup (defined as class 0, or class negative) represents the subset of patients characterized by kV-CBCT matching for greater than or equal to a certain percentage of treatment fractions, named fraction matching threshold λ. Conversely, iGroup includes patients who do not meet the eGroup criteria (defined as class 1, or class positive).
Table 2
Group | Ground truth of kV-CBCT | Proposed method (F3F) |
---|---|---|
iGroup (class 1, class positive) | Match <λ of all fractions (λ is 40–70%) | ≤1 fraction match in F3F, or ≤2 fractions match in first 4 fractions* |
eGroup (class 0, class negative) | Match ≥λ of all fractions (λ is 40–70%) | All 3 fractions match in F3F, or ≥3 fractions match in first 4 fractions* |
iGroup, protocol-ineligible group; eGroup, protocol-eligible group; λ, fraction matching threshold in the ground truth of setup error of kV-CBCT. *, if only 2 fractions match in first 3 fractions, an additional CBCT was acquired. kV, kilovoltage; CBCT, cone beam computed tomography; F3F, first three fractions.
In pursuit of implementing this innovative concept into clinical practice, this paper proposed the F3F method to classify patients into two different groups, with distinct CBCT imaging frequencies. As Figure 4 shows, for the first three CBCT scans, if the count of kV-CBCT matches less than or equal to one, the patient is promptly classified as iGroup. Conversely, if the count of kV-CBCT matches is three, the patient is assigned to the eGroup. Should two mismatches in the first three fractions arise, an additional CBCT scan is scrutinized in the subsequent treatment fraction—i.e., fraction number 4. If there is a match on fraction 4, the patient is classified as eGroup. Otherwise, the patient is included in the iGroup category.
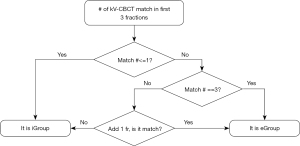
The fraction matching threshold λ concept is necessary, in principle, to parametrize what is the fraction of cases needed to determine that a patient has a total number of IGRT fractions that overall matched the test of the first three fractions—i.e., to establish a ground truth. Beyond this definition, however, we use this parameter to optimize the sensitivity and specificity of the F3F test.
Statistical analysis
As we mentioned above, the patient who is assigned to the iGroup is defined as class 1 (class positive), and the patient who is assigned to the eGroup is defined as class 0 (class negative). The predicted allocation to iGroup or eGroup, according to the F3F test, may correspond to the ground truth match—as defined in “Patient classification”—in four possible ways:
- iGroup(1,1): the outcome of the F3F test and ground truth are both positive, and the F3F classification operated a (true) positive identification.
- eGroup(1,0): the ground truth is positive, but the F3F classification returned a (false) negative identification.
- iGroup(0,1): the ground truth is negative, but the F3F classification returned a (false) positive identification.
- eGroup(0,0): F3F test and ground truth are both negative, and the case is a (true) negative identification.
The widely recognized concept of confusion matrix can be used to illustrate this classification scheme. For further details on the confusion matrix and its use we refer the reader to existing literature (13,14). For quick reference, in general sensitivity, specificity and accuracy are defined as follows:
The sensitivity, specificities and accuracies are calculated using Statistics and Machine Learning Toolbox in Matlab (MathWorks, Portola Valley, CA, USA).
The ground truth—i.e., the fraction of true eGroup and iGroup patients—depends on λ. The sensitivities, specificities and accuracies based on the ground truth will also change accordingly. In this report, λ ranging from 40% to 70% is applied to evaluate the changes in sensitivity and specificity. When λ is less than 40%, sensitivities and specificities reach a saturation level and won’t change with λ. When λ is greater than 70%, the specificities drop to less than 50%, and it cannot be used as a classification parameter. It’s recognized that sensitivity and specificity have an inverse relationship, where one increases as the other decreases (15). Combining sensitivity and specificity, by simple summation, one can select a best λ that maximizes both quantities simultaneously.
Results
We have analyzed 1,616 CBCT scans and the corresponding setup corrections based on the soft tissue alignment by CBCT after bony alignment using kV-orthogonal images. Among the 79 patients under study, the difference in the couch shifts between the kV and CBCT image guidance stages was calculated, and the results for all 632 CBCT scans are shown in Figure 5. The mean difference in setup error and their SD was −0.4±3.2 mm (AP), 0.1±8.9 mm (SI) and −0.2±1.6 mm (LR). While the means displayed relatively small magnitudes across all three dimensions, the observed larger SDs hint at the possibility of distinct patient groups within the overall population.

We calculated the overall means, SD of the means of the population systematic error, as well as the SD of the random error; this data is presented in Table 3.
Table 3
Variable | AP (cm) | SI (cm) | LR (cm) | 3D vector (cm) |
---|---|---|---|---|
Μ | −0.04 | 0.01 | −0.02 | 0.27 |
Σ | 0.15 | 0.10 | 0.07 | 0.50 |
σ | 0.31 | 0.94 | 0.15 | 0.82 |
Margin* | 0.58 | 0.91 | 0.28 | 1.83 |
*, margins calculated using van Herk margin recipe: 2.5 Σ+0.7 σ (15). kV, kilovoltage; CBCT, cone beam computed tomography; AP, anterior-posterior; SI, superior-inferior; LR, left-right; 3D, three dimensional; M, overall mean systematic setup error; Σ, standard deviation of the systematic setup error; σ, standard deviation of the random setup error.
It is also useful to know what is the distribution of shifts that exceed a given threshold Δ; we present this data in Figure 6. The shifts ≥5 mm occurred in 9.6% of the patients in the AP direction, 14.7% in the SI direction and 4.3% in the LR direction. In practice, for these patients the couch shifts from CBCT IGRT were always applied, so Figure 6 is a distribution of the residuals in the hypothetical case that only 2D-kV bony alignment is used to set up the patient.
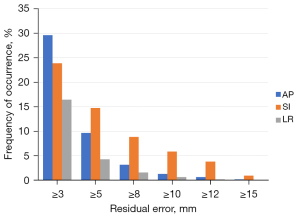
Figure 7A shows the sensitivity and specificity curves with λ between 40% to 70% with step size of 5%, as well as ∆ values of 3, 4, and 5 mm. The inversely-related sensitivity and specificity is illustrated in Figure 7A. The specificity curves increase slowly, while the sensitivity curves decrease fast, when λ changes from 40% to 70%.
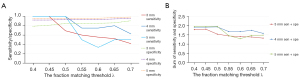
One criterion for evaluating the best λ is to maximize of sum of sensitivity and specificity (16). In Figure 7B, the best λ is determined to be 50% for ∆ values 4 and 5 mm, and 45% for ∆ values 3 mm. To simplify the comparison of sensitivities, specificities and accuracies with different setup thresholds, a fraction matching threshold of 50% will be used.
Table 4 displays the 2×2 confusion matrix for different ∆ values 3, 4, and 5 mm with λ equal to 50%. Only 16.5%, 3.8%, and 1.3% of cases fall under the classification of iGroup for the respective setup thresholds. On the other hand, 83.5%, 96.2%, and 98.7% of cases are categorized as eGroup.
Table 4
Ground truth of kV-CBCT | Proposed method (F3F) | |
---|---|---|
iGroup | eGroup | |
∆=3 mm, λ=50% | ||
iGroup | 9 | 4 |
eGroup | 10 | 56 |
∆=4 mm, λ=50% | ||
iGroup | 3 | 0 |
eGroup | 5 | 71 |
∆=5 mm, λ=50% | ||
iGroup | 1 | 0 |
eGroup | 3 | 75 |
iGroup, protocol-ineligible group; eGroup, protocol-eligible group; ∆, predetermined setup threshold of kV-CBCT shift; λ, fraction matching threshold in the ground truth of setup error of kV-CBCT. F3F, first three fractions; kV, kilovoltage; CBCT, cone beam computed tomography.
Sensitivities of determining an iGroup patient from the first three kV-orthogonal plus CBCT fractions are 0.69, 1.0, and 1.0. Specificities for identifying an eGroup patient are 0.85, 0.93 and 0.96. Accuracies were 0.82, 0.94 and 0.96, respectively (Table 5).
Table 5
Data | Sensitivity | Specificity | Accuracy |
---|---|---|---|
∆=3 mm | 0.69 | 0.85 | 0.82 |
∆=4 mm | 1.00 | 0.93 | 0.94 |
∆=5 mm | 1.00 | 0.96 | 0.96 |
F3F, first three fractions; ∆, predetermined setup threshold of kV-CBCT shift; λ, fraction matching threshold in the ground truth of setup error of kV-CBCT.
Discussion
In the present study we proposed a classification approach based on the F3F method to identify lung cancer patients eligible for an optimized IGRT protocol for conventionally fractionated radiation therapy. Based on our analysis, the majority of patients can benefit from our proposed IGRT protocol. Only a small amount of patients were classified as ineligible. More frequent than weekly CBCTs are recommended for these patients. This study also determines population-based kV-CBCT mismatch threshold parameters, which help make clinical decision when the F3F approach is used.
Prior published research contributed to the body of knowledge that is the foundation of our method. Santoro et al. (11) examined the differences in corrections between soft tissue and bony alignment. Specifically, corrections of 0.0±4.1, 1.1±3.8, 0.9±2.9, and 4.8±1.5 mm were observed in the AP, SI, LR directions and 3D vector, respectively (11). Similarly, Yeung et al. (10) found the differences of the corrections between soft tissue and bony alignments to be 0.3±3.1, −1.1±6.7, −0.6±2.4, and 5.4±5.7 mm respectively in the AP, SI and LR directions and 3D vector (10). Our mean differences in setup correction ± SD in the AP, SI, and LR directions and 3D vector are consistent with those reported by (10,11). The 2×2 cross tabulation we reported in Table 5 shows the percentage of cases classified as belonging to iGroup when the setup thresholds are 3, 4 and 5 mm, respectively, consistent with those reported by (10).
Sensitivity quantifies the capability of the F3F method to accurately classify patients within the truly ineligible ground truth subset. Specificity gauges the F3F method’s competence in accurately categorizing patients within the truly eligible ground truth subset. The relatively high values of sensitivity and specificity emphasize the robustness of the F3F method in accurately classifying patients into iGroup or eGroup. This strong performance validates the method’s reliability for application to conventionally fractionated lung patient treatments.
The proposed F3F method well categorized cases for our kV-based IGRT protocol. Not surprisingly more patients are categorized as eGroup if a larger setup threshold ∆ is used. CBCT frequency in the daily kV-orthogonal plus weekly CBCT protocol may need to increase to more than one CBCTs per week for iGroup patients. After the first three fractions the IGRT CBCT frequency can be kept to no more frequently than weekly CBCT for eGroup patients.
The question of how the F3F frequentist approach may relate to the PTV margins may arise. Our clinical protocol for locally advanced lung cases is to use a CTV to PTV margin of 5 mm, as is the case with most of the cohort in this work. The underlying assumption in this study is that for a given action threshold (3, 4 or 5 mm), the IGRT correction, when applied, will produce a perfect PTV match, the measured shift being the residual setup error that the PTV must incorporate. With that in mind the margins derived using van Herk’s formula (17) for our patient population appear to be insufficient only in the SI direction; from Figure 6, however, we evince that mismatches larger than 5 mm in the SI direction are relatively infrequent, less than 15%, and unlikely to cause clinically significant dosimetric discrepancy with the treatment plan intent. Determining how significant such discrepancies from the treatment plan are, in relation to the frequency of imaging, was not the goal of the present work. Perhaps more modestly, this study intended to derive a relatively robust method to partition patient cohorts into IGRT frequency schedules that would be more patient-specific, avoiding the burden of increased imaging frequency for the majority of patients that may not need it. Yeung et al. (18) discussed the role of setup margins in different IGRT scenarios, finding that if daily image guidance is not possible there is not much benefit derived from imaging more frequently; this supports our approach, with the caveat that in our data the IGRT always included daily 2D kV alignment to bony anatomy, and the couch shifts are to be intended from an already existing bony anatomy registration. From a clinical standpoint the dosimetric impact of ‘missed mismatches’ plays a crucial role in determining just how many fractions with mismatch beyond threshold may be tolerable, for a given course of treatment. Specifically, the value of λ may be related to a dosimetric deficit to be quantified. This is a study that we plan to undertake as a follow-up to this work.
This method cannot give an indication of CBCT frequency for the iGroup patients in our study, which can be considered a limitation of this study. Secondly, the ground truth of patient classification in our study is based on dataset79, which has 8 fractions of CBCT per patient.
There are at least two ways that can be used to implement the proposed classification method. One method is to use a script within the TPS system that will automatically extract the kV/kV to CBCT shifts of F3F of CBCTs saved in the Record and Verify system and compare with the predefined threshold and classify the patient to either eGroup or iGroup. The second method is to build an in-house application which can be run by physicists to add the kV/kV to CBCT shifts of the F3F of CBCTs and determine if the patient belongs to the eGroup or iGroup.
Conclusions
We have proposed a new patient classification approach in the context of an IGRT protocol with optimized CBCT frequency for conventionally fractionated lung cancer radiation therapy. The first three CBCT helps predict patient eligibility of this IGRT protocol. We have evaluated different threshold criteria and found high sensitivity, specificities and accuracies are achievable. This study supports that weekly CBCT is sufficient for the most of the lung patients. The same method, proposed adaptive classification approach in this study, might also be applied for other anatomic sites.
Acknowledgments
We are deeply grateful to Dr. Karl Prado for his invaluable and constructive suggestions throughout the planning and development of this research. His generous dedication of time has been greatly appreciated and will be remembered with utmost gratitude.
Footnote
Reporting Checklist: The authors have completed the TRIPOD reporting checklist. Available at https://tlcr.amegroups.com/article/view/10.21037/tlcr-24-606/rc
Data Sharing Statement: Available at https://tlcr.amegroups.com/article/view/10.21037/tlcr-24-606/dss
Peer Review File: Available at https://tlcr.amegroups.com/article/view/10.21037/tlcr-24-606/prf
Funding: None.
Conflicts of Interest: All authors have completed the ICMJE uniform disclosure form (available at https://tlcr.amegroups.com/article/view/10.21037/tlcr-24-606/coif). All authors have no conflicts of interest to declare.
Ethical Statement: The authors are accountable for all aspects of the work in ensuring that questions related to the accuracy or integrity of any part of the work are appropriately investigated and resolved. This retrospective study was reviewed and approved by the Institutional Review Board of University of Maryland School of Medicine (approval number: HP-00079915), and it was conducted in accordance with the Declaration of Helsinki (as revised in 2013). All patients’ individual consent for this retrospective analysis was waived.
Open Access Statement: This is an Open Access article distributed in accordance with the Creative Commons Attribution-NonCommercial-NoDerivs 4.0 International License (CC BY-NC-ND 4.0), which permits the non-commercial replication and distribution of the article with the strict proviso that no changes or edits are made and the original work is properly cited (including links to both the formal publication through the relevant DOI and the license). See: https://creativecommons.org/licenses/by-nc-nd/4.0/.
References
- Galetta D. Advances in Lung Cancer Therapy. Cancers (Basel) 2023;15:2671. [Crossref] [PubMed]
- Gholami L, Ivari JR, Nasab NK, et al. Recent Advances in Lung Cancer Therapy Based on Nanomaterials: A Review. Curr Med Chem 2023;30:335-55. [Crossref] [PubMed]
- De Ruysscher D, Belderbos J, Reymen B, et al. State of the art radiation therapy for lung cancer 2012: a glimpse of the future. Clin Lung Cancer 2013;14:89-95. [Crossref] [PubMed]
- Luh JY, Albuquerque KV, Cheng C, et al. ACR-ASTRO Practice Parameter for Image-guided Radiation Therapy (IGRT). Am J Clin Oncol 2020;43:459-68. [Crossref] [PubMed]
- Garibaldi C, Piperno G, Ferrari A, et al. Translational and rotational localization errors in cone-beam CT based image-guided lung stereotactic radiotherapy. Phys Med 2016;32:859-65. [Crossref] [PubMed]
- Lozano Ruiz FJ, Ileana Pérez Álvarez S, Poitevin Chacón MA, et al. The importance of image guided radiotherapy in small cell lung cancer: Case report and review of literature. Rep Pract Oncol Radiother 2020;25:146-9. [Crossref] [PubMed]
- Mossahebi S, Langner U, Prado K, et al. A study for the dosimetric evaluation of rotational setup error for lung stereotactic body radiation therapy. Journal of Radiotherapy in Practice 2018;17:420-4. [Crossref]
- Ding GX, Coffey CW. Radiation dose from kilovoltage cone beam computed tomography in an image-guided radiotherapy procedure. Int J Radiat Oncol Biol Phys 2009;73:610-7. [Crossref] [PubMed]
- Brenner DJ, Hall EJ. Computed tomography--an increasing source of radiation exposure. N Engl J Med 2007;357:2277-84. [Crossref] [PubMed]
- Yeung AR, Li JG, Shi W, et al. Tumor localization using cone-beam CT reduces setup margins in conventionally fractionated radiotherapy for lung tumors. Int J Radiat Oncol Biol Phys 2009;74:1100-7. [Crossref] [PubMed]
- Santoro JP, McNamara J, Yorke E, et al. A study of respiration-correlated cone-beam CT scans to correct target positioning errors in radiotherapy of thoracic cancer. Med Phys 2012;39:5825-34. [Crossref] [PubMed]
- Hogue C, Blackburn M, Swanson C, et al. Optimum Frequency of Cone Beam Computed Tomography (CBCT) for Intact Prostate IMRT. International Journal of Radiation Oncology, Biology, Physics 2014;90:S438. [Crossref]
- Fawcett T. An introduction to ROC analysis. Pattern Recognition Letters 2006;27:861-74. [Crossref]
- Tharwat A. Classification assessment methods. Applied Computing and Informatics 2021;17:168-92. [Crossref]
- Monaghan TF, Rahman SN, Agudelo CW, et al. Foundational Statistical Principles in Medical Research: Sensitivity, Specificity, Positive Predictive Value, and Negative Predictive Value. Medicina (Kaunas) 2021;57:503. [Crossref] [PubMed]
- Kaivanto K. Maximization of the sum of sensitivity and specificity as a diagnostic cutpoint criterion. J Clin Epidemiol 2008;61:517-8. [Crossref] [PubMed]
- van Herk M, Remeijer P, Rasch C, et al. The probability of correct target dosage: dose-population histograms for deriving treatment margins in radiotherapy. Int J Radiat Oncol Biol Phys 2000;47:1121-35. [Crossref] [PubMed]
- Yeung AR, Li J, Shi W, et al. Optimal image-guidance scenario with cone-beam computed tomography in conventionally fractionated radiotherapy for lung tumors. Am J Clin Oncol 2010;33:276-80. [Crossref] [PubMed]