Deep learning-based lung cancer risk assessment using chest computed tomography images without pulmonary nodules ≥8 mm
Highlight box
Key findings
• Deep learning-based, label-free lung cancer risk prediction models were developed using alternative low-dose chest computed tomography (LDCT) datasets, targeting individuals without pulmonary nodules larger than 8 mm.
What is known and what is new?
• The models showed high area under the receiver operating characteristic curve values and low Brier scores in validation using the alternative dataset, demonstrating strong accuracy in predicting lung cancer within 5 years.
• Tested on real-world LDCT datasets, the models maintained robust performance, highlighting their practical application and efficiency in screening.
What is the implication, and what should change now?
• These models can significantly improve LDCT lung cancer screening by identifying high-risk individuals early, enabling timely interventions and better outcomes.
Introduction
Lung cancer is the leading cause of cancer-related deaths in men and women worldwide (1). The United States Preventive Services Task Force recommends annual screening for lung cancer using low-dose chest computed tomography (LDCT) for high-risk individuals aged 50 to 80 years with a 20-pack-year smoking history who currently smoke or have quit within the past 15 years (2). However, LDCT screening carries potential harm, including false positives, overdiagnosis, and radiation exposure, which can lead to unnecessary procedures and patient anxiety (3). To mitigate these risks, the Fleischner Society Guidelines recommend several options for solid non-calcified nodules larger than 8 mm in diameter because they are suspected of malignancy and require further evaluation (4). These options include a 3-month follow-up, work-up with positron emission tomography and computed tomography (CT), tissue sampling, or a combination (5). However, for nodules measuring 8 mm or smaller, a consensus has not been reached on the criteria to differentiate between low- and high-risk nodules, leading to controversy regarding the appropriate follow-up strategy (6). Moreover, the decision to perform follow-up chest CT in individuals without detected pulmonary nodules on LDCT remains debatable, with varying guidelines, as limited evidence exists to support the routine use of follow-up CT scans in individuals without nodules. Thus, determining the appropriate follow-up strategy for patients with nodules measuring 8 mm or smaller, or for those without nodules, remains a critical issue. Efforts have been made to develop more accurate lung cancer prediction models that incorporate demographic information and biological characteristics to address these issues (7). However, these models have had varying performances, and their practicability has been limited (8).
One promising approach to developing lung cancer prediction models is the application of deep learning (DL) algorithms to analyze chest CT images (9). Previous DL-based models have primarily focused on the automatic detection and evaluation of pulmonary nodules to aid in the diagnosis of lung cancer (9,10). These algorithms are particularly adept at differentiating between benign and malignant tumors in individuals with pulmonary nodules larger than 8 mm in diameter (11). However, the predictive capabilities of DL algorithms in individuals with nodules measuring 8 mm or smaller, or those without any detected nodules, are less established. While some studies have reported that DL algorithms can detect important radiologic features by analyzing lung parenchyma on CT images—thus enabling lung cancer predictions beyond the currently identifiable features of pulmonary nodules (12)—research in this area remains limited. This limitation is partly due to the challenge of obtaining a large number of CT scans from high-risk individuals, considering the relatively low incidence rate (approximately 1–2%) of lung cancer among populations undergoing screening using LDCT images (13). To overcome the scarcity of high-risk LDCT scans needed for training DL algorithms, a label-free classification can be employed (14). This involves using easily obtainable training data that mimics high-risk conditions (15). In the context of lung cancer risk prediction, instead of using LDCT scans from high-risk individuals, the label-free approach permits the use of chest CT scans from patients already diagnosed with lung cancer—alternative training data. Specifically, this approach focuses on the lung opposite to the one with the tumor, which is expected to exhibit radiologic characteristics similar to those of high-risk individuals (16). This approach seeks to overcome the limitations of data scarcity and enhance the prediction model’s performance (14). Therefore, this study aims to develop a DL-based label-free lung cancer risk prediction model using alternative data and to validate it in individuals without non-calcified solid pulmonary nodules larger than 8 mm using real-world data. We present this article in accordance with the TRIPOD reporting checklist (available at https://tlcr.amegroups.com/article/view/10.21037/tlcr-24-882/rc).
Methods
Patient datasets
We included two groups of LDCT scans of individuals without non-calcified solid pulmonary nodules larger than 8 mm: one group for the training and validation datasets, and another for the real-world test dataset. The alternative dataset consisted of LDCT scans obtained from patients at Keimyung University Dongsan Hospital between January 2008 and December 2011 who underwent follow-up chest CT scans at least 5 years later. We excluded LDCT scans that showed single or multiple solid non-calcified nodules larger than 8 mm in diameter, active inflammation, lung metastasis, pleural effusion, pneumothorax, or those from patients with a history of lung surgery, as these factors could affect the reliability of the DL algorithm predictions. Within the alternative dataset, LDCT scans were classified into two subgroups: lung cancer and control. The lung cancer group, serving as an alternative for the high-risk group, included LDCT scans with pathologically proven lung cancer. The control group, representing the low-risk group, comprised LDCT scans that did not detect lung cancer and showed no evidence of lung cancer development over 5 years of follow-up. We randomly divided the alternative dataset into training and validation sets in a ratio of 3:1. In the lung cancer group, only the contralateral lung—the lung opposite to where cancer developed—was used for training and validation, whereas in the control group, both lungs were used. This approach enabled us to develop a DL-based, label-free lung cancer risk prediction model using this alternative dataset.
For the real-world test dataset, we collected LDCT scans performed at Keimyung University Dongsan Hospital between January 2012 and December 2017. All LDCT scans were performed without contrast enhancement, following the imaging protocol for LDCT (17). Exclusion criteria were the same as for the alternative dataset: LDCT scans showing single or multiple solid non-calcified nodules larger than 8 mm, active inflammation, lung metastasis, pleural effusion, pneumothorax, or scans from patients with a history of lung surgery. LDCT scans that did not detect lung cancer at the time but where lung cancer developed within 5 years after the LDCT was classified into the high-risk group. LDCT scans that did not detect lung cancer and showed no evidence of lung cancer development over 5 years of follow-up were classified into the low-risk group. Recognizing that certain histopathologic types of lung cancer—such as small cell lung cancer (SCLC), carcinoid tumors, and other rare types—may exhibit different characteristics on LDCT images and possess different risk factors compared to adenocarcinoma and squamous cell carcinoma, we categorized the test dataset into two groups for subgroup analysis: Group A, comprising adenocarcinoma and squamous cell carcinoma, and Group B, including SCLC, carcinoid tumors, and rare types such as adenoid cystic carcinoma, sarcomatoid carcinoma, and pleomorphic carcinoma. This stratification allowed us to assess the performance of the DL model across different lung cancer histopathologic subtypes. Additionally, to evaluate the predictive ability for lung cancer development based on the presence or absence of pulmonary nodules, we further categorized the test dataset into two subgroups: the non-nodule group (LDCT scans without pulmonary nodules) and the nodule group (LDCT scans with pulmonary nodules smaller than 8 mm), enabling us to assess the DL model’s performance in predicting lung cancer risk in patients both with and without pulmonary nodules. The study was approved by the Institutional Review Board of Dongsan Hospital (No. 2023-01-067), and individual consent for this retrospective analysis was waived. The study was conducted in accordance with the Declaration of Helsinki (as revised in 2013).
Model development
For the training and validation of the DL-based lung cancer prediction model, only the lung parenchyma opposite the detected cancer was used for the lung cancer group, whereas both lungs were used for the control group. The model development process involves preprocessing LDCT images through intensity windowing, normalization, and segmentation using two-dimensional (2D) densely connected U-Net (DenseUNet) to isolate the lung parenchyma. Four three-dimensional (3D) convolutional neural networks (CNNs) are then trained on these segmented images to predict lung cancer risk. All deep networks were implemented with Python3 based on Keras with a TensorFlow backend using a single NVIDIA RTX A6000 GPU 48 Gb. The entire process of our proposed method is shown in Figure 1.
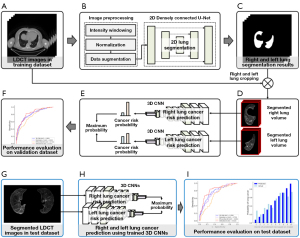
The Digital Imaging and Communications in Medicine (DICOM) files of LDCT images were loaded into Python using the Pydicom library. The LDCT images have a pixel spacing of 0.976 mm × 0.976 mm and a thickness ranging from 2.0 to 3.27 mm. The window width and level were set to 1,500 and −600 HU, respectively, with values ranging from −1,000 to 600 HU. Images were normalized to a scale of 0 to 1 using min-max normalization. Automatic lung segmentation was performed using 2D DenseUNet, which utilizes DenseNet121 as the backbone for efficient image segmentation (18). DenseNet121 consists of four densely connected blocks with transition blocks, and the decoder architecture is similar to that of U-Net for image resolution recovery (Figure 2A) (19). Input images of 512×512 pixels were normalized and fed to DenseUNet. The initial weights of DenseUNet were initialized using ImageNet for transfer learning. DenseUNet, with approximately 12.2 million trainable parameters, was used to segment the lung parenchyma, removing the mediastinum and chest wall. An input volume of 128×256×192 pixels for CNNs was extracted from the centroid of each segmented lung.
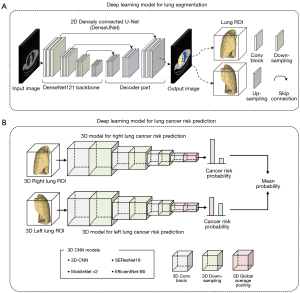
Following automatic lung segmentation, four 3D CNNs—3D-CNN, MobileNet v2, SEResNet18, and EfficientNet-B0—were employed to predict lung cancer risk from LDCT images (Figure 2B). The 3D-CNN included four convolutional blocks with 3×3×3 convolutions, batch normalization, rectified linear unit (ReLU) layers, a 3D max pooling layer, and a global average pooling (GAP) layer leading to a sigmoid-activated output layer, with approximately 1.2 million trainable parameters. MobileNet v2, optimized for low computing power with depth-wise separable convolutions, had about 2.4 million trainable parameters (20). SEResNet18, featuring squeeze-and-excitation blocks for dynamic feature recalibration, contained around 6.5 million trainable parameters (21). EfficientNet-B0, which uses a compound scaling method and neural architecture search framework, had approximately 33.3 million trainable parameters (22). Two 3D prediction networks were trained to output probabilities from 0.0 to 1.0 for left and right lung cancer risk, with the final probability being the average of the bilateral lung probabilities. These probabilities were then used to classify individuals as high- or low-risk for lung cancer development.
We trained the DenseUNet for 200 epochs with a mini-batch size of 1 and dice similarity loss. Data augmentation for the segmentation model was used with random rotation (−30° to 30°), zoom (−10% to 10%), and translation shift (−10% to 10%). For training, the Adam optimizer was applied with β1=0.9 and β1=0.999. An initial learning rate of 10−4 was reduced by half up to 10−6 each time the validation loss saturated for 25 epochs. We trained the 3D CNNs for 200 epochs with a mini-batch size of 1 and binary cross-entropy loss. Data augmentation methods were used with random rotation (−15° to 15°), zoom (−10% to 10%), and translation shift (−10% to 10%). The Adam optimizer was applied for network training with β1=0.9 and β1=0.999. An initial learning rate of 10−4 was reduced by half up to 10−6 each time the validation loss saturated for 10 epochs.
Statistical analysis
The prediction models were validated using the validation and test datasets to rigorously assess their performance, with a blind assessment of the predicted outcomes employed to minimize bias and enhance the reliability of the evaluation. The area under the receiver operating characteristic (ROC) curve (AUC) was calculated to determine discrimination. The prediction model is considered good when the AUC is over 0.70 and excellent when the AUC is over 0.8 (23). Sensitivity, specificity, positive predictive value, negative predictive value, and accuracy were also calculated by varying the threshold for the probability of lung cancer. The performances of our models were also evaluated with respect to calibration with bootstrap resampling (24). Calibration ability refers to how closely the predicted probabilities match the actual outcomes numerically. The risk of developing lung cancer for each participant was calculated, and the results were sorted in ascending order. In each decile, the average predicted probabilities were compared with the actual probabilities of developing lung cancer. The Brier score was calculated to determine the overall calibration of the predictions (25). A Brier score of 0 suggests perfect accuracy.
Results
Demographic characteristics
We included 1,064 LDCT scans from participants with an average age of 59.89±10.92 years and a male population of 489 (46.0%) in the alternative training and validation datasets. Among these participants, 684 were classified into the control group, whereas 380 were classified into the lung cancer group. Subsequently, the LDCT scans were randomly divided into training and validation sets in a 3:1 ratio (Figure 3). In the training dataset, within the low-risk group, 312 chest CT scans (68%) exhibited no pulmonary nodules, while 144 scans (32%) had nodules <8 mm. In the high-risk group, 160 chest CT scans (63%) showed no nodules, and 93 scans (37%) contained nodules <8 mm. Specifically, among the high-risk group, 34 chest CT scans (37%) had pulmonary nodules sized between 5 and 8 mm. In the low-risk group, 130 chest CT scans (90%) presented pulmonary nodules ranging from 1 to 4 mm. The real-world test dataset comprised 1,306 LDCT scans from participants with an average age of 60.05±11.85 years and a male population of 444 (34.0%). Among these participants, 1,254 were classified into the low-risk group, and 52 were classified into the high-risk group. Table 1 details the characteristics of the participants. In the test dataset, within the low-risk group, 575 chest CT scans (46%) exhibited no pulmonary nodules, while 679 scans (54%) had nodules. In the high-risk group, 23 chest CT scans (44%) showed no nodules, and 29 scans (56%) contained nodules <8 mm. Specifically, among the high-risk group, 9 chest CT scans (31%) had pulmonary nodules sized between 5 and 8 mm. In the low-risk group, 668 chest CT scans (98%) presented pulmonary nodules ranging from 1 to 4 mm.
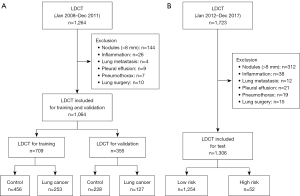
Table 1
Risk factors | Training set | Validation set | Test set | |||||
---|---|---|---|---|---|---|---|---|
Control (n=456) |
Lung cancer (n=253) | Control (n=228) |
Lung cancer (n=127) | Low risk (n=1,254) | High risk (n=52) | |||
Age (years) | 60.52 [11.17] | 60.16 [10.10] | 56.84 [11.46] | 62.33 [9.81] | 60.10 [12.0] | 58.98 [7.51] | ||
Male | 145 (31.8) | 179 (70.8) | 66 (28.9) | 99 (78.0) | 399 (31.8) | 45 (86.5) | ||
Body mass index (kg/m2) | 23.17 [3.56] | 23.26 [8.14] | 23.64 [3.91] | 23.01 [3.31] | 24.06 [4.07] | 21.03 [4.17] | ||
Current or past smoker | 93 (20.4) | 193 (76.3) | 45 (19.7) | 103 (81.1) | 340 (27.1) | 40 (76.9) | ||
Histologic type | ||||||||
Adenocarcinoma | N/A | 115 (45.5) | N/A | 57 (44.9) | N/A | 21 (40.4) | ||
Squamous carcinoma | N/A | 105 (41.5) | N/A | 54 (42.5) | N/A | 18 (34.6) | ||
Small cell carcinoma | N/A | 8 (3.2) | N/A | 3 (2.4) | N/A | 3 (5.8) | ||
Carcinoid carcinoma | N/A | 23 (9.1) | N/A | 12 (9.4) | N/A | 8 (15.4) | ||
Rare type | N/A | 2 (0.8) | N/A | 1 (0.8) | N/A | 2 (3.8) | ||
Number of pulmonary nodule | 0.6 [1.0] | 0.5 [0.8] | 0.5 [0.7] | 0.5 [0.8] | 0.7 [1.0] | 0.9 [1.0] | ||
Size of pulmonary nodule (mm) | 4.0 [1.8] | 3.3 [1.8] | 4.0 [1.5] | 3.5 [1.4] | 4.3 [1.7] | 4.5 [1.7] |
Data are presented as mean [SD] or n (%). The rare types of lung cancer include adenoid cystic carcinomas, sarcomatoid carcinoma, and pleomorphic carcinoma. N/A, not applicable; SD, standard deviation.
Lung cancer risk-prediction model validation
Lung cancer predictive models incorporating four CNNs (3D-CNN, MobileNet v2, SEResNet18, and EfficientNet-B0) were developed using the training set and were validated on the validation and test datasets. In the validation dataset, the AUC values were 0.801, 0.802, 0.755, and 0.833 for 3D-CNN, MobileNet v2, EfficientNet-B0, and SEResNet18, respectively (Table 2). The Brier scores, which are calibration measures, were 0.169, 0.175, 0.217, and 0.156 for the four CNNs, respectively (Figure 4). Table S1 presents the sensitivity, specificity, positive predictive value, negative predictive value, and accuracy of each prediction model using 10–90% probability thresholds in the validation dataset.
Table 2
Models | AUC (95% CI) | Brier score (95% CI) |
---|---|---|
3D-CNN | 0.801 (0.759–0.842) | 0.169 (0.130–0.208) |
MobileNet v2 | 0.802 (0.761–0.843) | 0.175 (0.135–0.215) |
EfficientNet-B0 | 0.755 (0.710–0.800) | 0.217 (0.174–0.260) |
SEResNet18 | 0.833 (0.794–0.872) | 0.156 (0.118–0.194) |
AUC, area under the ROC curve; ROC, receiver operating characteristic; CI, confidence interval; 3D, three-dimensional; CNN, convolutional neural network.
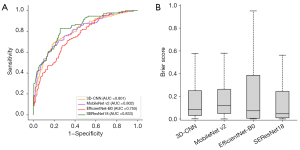
In the test dataset, regardless of histopathologic types and the presence of pulmonary nodules, the AUC values were 0.769, 0.753, 0.681, and 0.820 for 3D-CNN, MobileNet v2, EfficientNet-B0, and SEResNet18, respectively (Table 3). The Brier scores were 0.169, 0.180, 0.202, and 0.138, respectively (Figure 5). The calibration of the models shows that all four models effectively stratify lung cancer risk, with SEResNet18 and EfficientNet-B0 demonstrating the closest alignment between predicted and actual probabilities (Figure 6). Table S2 presents the sensitivity, specificity, positive predictive value, negative predictive value, and accuracy of each prediction model using 10–90% probability thresholds in the test dataset.
Table 3
Models | AUC (95% CI) | Brier score (95% CI) |
---|---|---|
3D-CNN | 0.769 (0.746–0.792) | 0.169 (0.130–0.208) |
MobileNet v2 | 0.753 (0.730–0.776) | 0.180 (0.140–0.220) |
EfficientNet-B0 | 0.681 (0.656–0.706) | 0.202 (0.160–0.244) |
SEResNet18 | 0.820 (0.799–0.841) | 0.138 (0.102–0.174) |
AUC, area under the ROC curve; ROC, receiver operating characteristic; CI, confidence interval; 3D, three-dimensional; CNN, convolutional neural network.
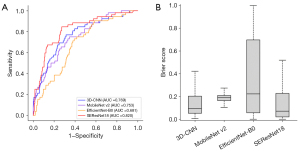
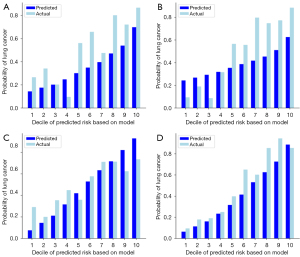
For the subgroup analysis based on the histopathologic types of lung cancer, we evaluated the model performance separately for Group A (adenocarcinoma and squamous cell carcinoma) and Group B (SCLC, carcinoid tumors, and rare types). In Group A of the test dataset, the AUC values were 0.762 for 3D-CNN, 0.776 for MobileNet v2, 0.667 for EfficientNet-B0, and 0.835 for SEResNet18. The Brier scores were 0.170 for 3D-CNN, 0.179 for MobileNet v2, 0.202 for EfficientNet-B0, and 0.137 for SEResNet18, respectively. In Group B, the AUC values were 0.793 for 3D-CNN, 0.686 for MobileNet v2, 0.724 for EfficientNet-B0, and 0.776 for SEResNet18 (Figure S1). The Brier scores were 0.169 for 3D-CNN, 0.177 for MobileNet v2, 0.201 for EfficientNet-B0, and 0.135 for SEResNet18, respectively. For the subgroup analysis based on the presence of pulmonary nodules on LDCT images, we assessed model performance separately for the non-nodule group and the nodule group. In the non-nodule group, the AUC values were 0.749 for 3D-CNN, 0.748 for MobileNet v2, 0.668 for EfficientNet-B0, and 0.786 for SEResNet18, with Brier scores of 0.169, 0.178, 0.202, and 0.137, respectively. In the nodule group, the AUC values improved to 0.786 for 3D-CNN, 0.758 for MobileNet v2, 0.691 for EfficientNet-B0, and 0.847 for SEResNet18, with Brier scores of 0.169, 0.178, 0.202, and 0.136, respectively (Figure S2).
Discussion
We developed a DL-based, label-free lung cancer risk prediction model using alternative LDCT images and validated it with a real-world dataset, focusing on individuals without solid non-calcified nodules larger than 8 mm in diameter. Our findings demonstrate that DL analysis of lung parenchyma in LDCT images can effectively predict lung cancer risk. Unlike traditional models that diagnose lung cancer using nodule-based risk assessments, our model predicts future lung cancer development by analyzing lung parenchyma independent of detectable nodules. To employ a label-free approach, we utilized LDCT scans from patients with lung cancer; these scans served as alternative training data representing high-risk individuals. This strategy enabled us to develop a clinically versatile and easily implementable lung cancer prediction model. Our model has the potential to enhance the efficiency of LDCT screening for lung cancer.
Several conventional models have been developed to predict the probability of lung cancer (8,26). Traditional epidemiological models, which rely on common risk factors, have limited predictive ability with an AUC of less than 0.75 (7), and require extensive data collection efforts while demonstrating limited effectiveness beyond their designated demographic groups. Furthermore, predictive models that incorporate molecular biology and genetic information necessitate significant data collection, including physician consultations, sample collection, and additional testing, resulting in increased healthcare costs, time, and effort, without significantly improving predictive performance over existing models, thereby limiting their clinical utility (27). To address these limitations, we developed a risk prediction model by analyzing the lung parenchyma on LDCT images, achieving an impressive AUC of 0.681–0.820 for predicting lung cancer development within 5 years. A comparable study, known as Sybil, utilized a DL-based prediction model with LDCT data from the NLST to predict lung cancer within 6 years, demonstrating similar performance with AUC values between 0.69 and 0.81 (12). The Sybil model trained its DL algorithm using chest CT images that included both scans without nodules and scans with pulmonary nodules larger than 8 mm. This approach enabled Sybil to predict lung cancer risk at 2, 3, and 4 years post-imaging. In contrast, our study focused on predicting the risk of lung cancer development after 5 years by training the DL algorithm on chest CT images without nodules or with pulmonary nodules smaller than 8 mm. In Sybil’s test dataset that included nodules, the AUC reached 0.81, comparable to the accuracy of our model. When Sybil analyzed chest CT scans without nodules separately, the AUC was 0.69, slightly lower than the results from the dataset including nodules. Similarly, our DL models generally showed slightly lower AUCs in test groups without nodules compared to those with nodules, demonstrating results consistent with Sybil’s findings. Based on these findings, both studies indicate that analyzing lung parenchyma on LDCT images can effectively predict lung cancer development. This DL-based lung cancer prediction model for analyzing chest CT images has the potential to enhance the efficiency of lung cancer screening and offer extensive public health and socioeconomic benefits (3). However, its specific role—such as recommending changes to screening intervals (more or less frequent) or integration into annual LDCT screening programs—would require further prospective studies to determine its clinical utility and impact on screening protocols.
In our training set, we utilized segmented LDCT images of the contralateral lung—the lung opposite to where cancer developed. This approach differs from that of other models that use LDCT images of both lungs from high-risk cohorts prior to lung cancer development (12). Acquiring sufficient LDCT scans from high-risk cohorts for training DL algorithms is challenging because the probability of future lung cancer development in individuals with normal LDCT screening results is less than 1% to 5% (28). A recent study using LDCT scans from the National Lung Screening Trial (NLST) reported the efficiency of DL algorithms in predicting lung cancer development within 6 years in individuals without any detected pulmonary nodules. However, even in this large-scale study, only about 5% (1,400 LDCT scans) of the 28,000 patients whose CT scans comprised the training set were classified as high-risk individuals (12). To address this issue, a label-free approach, which leverages alternative data relevant for training DL models could be employed, even if it does not precisely match the target application data (14). In the context of lung cancer risk prediction, using LDCT scans from patients already diagnosed with lung cancer serves as an appropriate alternative. Although the contralateral lung does not possess all the same lung cancer development features as the affected lung, and therefore, cancer did not develop there, it exhibits lung cancer–related characteristics to a similar extent as the lung where cancer developed. This is because both lungs are exposed to the same systemic carcinogenic factors, such as smoking, environmental toxins, and genetic predispositions (29). These shared exposures can induce similar structural and molecular changes associated with increased cancer risk. The contralateral lung may exhibit radiologic features similar to those of high-risk individuals, including chronic obstructive pulmonary disease, emphysema, interstitial lung disease, and asbestosis (28,30). Moreover, patients with lung cancer have a higher likelihood of developing metachronous or synchronous primary lung cancers in the contralateral lung (30), indicating that both lungs are susceptible to malignancy due to systemic carcinogenic influences. By using LDCT images of the contralateral lung, we enable the DL model to learn from patterns associated with elevated lung cancer risk, even in the absence of overt malignancy.
In the present study, the DL-based lung cancer risk prediction model aims to measure the probability of lung cancer development within the next 5 years by analyzing features of the lung parenchyma without detecting pulmonary nodules larger than 8 mm. This approach is feasible because LDCT imaging can provide detailed structural information about the lungs with millimeter-thick sections, enabling the distinct presentation of structural changes associated with lung cancer (14). These changes include chronic obstructive pulmonary disease, pulmonary tuberculosis, asbestosis, silicosis, emphysema, and interstitial abnormalities, which can help identify individuals at higher risk of developing lung cancer (24,28). Supporting this approach, a Danish study involving 1,990 chest LDCT images from the Danish Lung Cancer Screening Trial revealed that patients with lung cancer had a higher occurrence and severity of emphysema and a greater frequency of interstitial abnormalities on visual analysis (14). However, these differences were not observed in the quantitative analysis of chest CT images. This study had some limitations that warrant discussion. The trial was a 4-year, 5-round screening study in which the interval between CT scans was significantly shorter for patients who developed cancer compared to those who did not, potentially introducing a bias in the detection of structural changes. Additionally, the effect of radation exposure from repeated LDCT scan was not explored, which could influence lung tissue characteristics. Moreover, some differences between cancer and non-cancer patients were significant only in the later scans and not in the baseline scans, suggesting that these structural changes may develop over time and may not be present at initial screening (16). Although the study emphasized that visual analysis was more effective than quantitative analysis of emphysema and interstitial abnormalities for predicting lung cancer risk, visual analysis alone might not be sufficient for predicting lung cancer occurrence due to its subjective nature and potential for observer variability. This finding underscores the challenge of using conventional quantitative or visual methods to evaluate numerous structural features related to lung cancer development, which can result in overlooking ambiguous areas on LDCT images. Consequently, both conventional quantitative and visual analysis methods have limitations in predicting lung cancer development (10). To overcome these limitations, we utilized a DL algorithm to objectively predict lung cancer development by extracting sub-level features, classifying, and quantifying features related to lung cancer development, and providing a quantitative risk score. By leveraging advanced DL techniques, our model aims to improve the prediction of lung cancer risk and contribute to its prevention.
Our study has some limitations. First, the study population was relatively small and consisted exclusively of individuals from South Korea, indicating that the performance of our lung cancer prediction model has only been validated in an Asian population. Second, we excluded LDCT scans of individuals with pulmonary nodules larger than 8 mm in diameter, which prevented us from assessing the risk associated with larger pulmonary nodules in lung cancer development. Moreover, although our study included chest CT scans with nodules measuring 8 mm or smaller, it was challenging to compare our lung cancer prediction model with existing nodule diagnosis models. This difficulty arises because existing models diagnose lung cancer by evaluating current nodules, whereas our model predicts lung cancer development over a 5-year period—a fundamentally different approach. In real-world clinical settings, pulmonary nodules are often detected during LDCT screening, highlighting the necessity of developing a comprehensive lung cancer diagnosis and prediction model that evaluates the risk of lung cancer based on both lung parenchyma and pulmonary nodules (8). Therefore, further large-scale cohort studies using a DL-based prediction model that incorporates both lung parenchyma and pulmonary nodules are necessary to assess the impact of such models on management and decision-making for individuals undergoing lung cancer screening in real-world settings.
Conclusions
Our study demonstrated that DL-based, label-free lung cancer risk prediction models using alternative LDCT images can effectively predict lung cancer development in individuals without non-calcified solid pulmonary nodules larger than 8 mm. Lung cancer development can be successfully predicted by analyzing lung parenchyma on LDCT images without using additional nodule information. These models show potential to enhance the efficiency of lung cancer screening programs. However, their specific role requires further prospective studies to determine clinical utility and impact. Validation in larger, more diverse populations is necessary to ensure generalizability across different ethnic groups and geographic regions.
Acknowledgments
Funding: This research was funded by
Footnote
Reporting Checklist: The authors have completed the TRIPOD reporting checklist. Available at https://tlcr.amegroups.com/article/view/10.21037/tlcr-24-882/rc
Data Sharing Statement: Available at https://tlcr.amegroups.com/article/view/10.21037/tlcr-24-882/dss
Peer Review File: Available at https://tlcr.amegroups.com/article/view/10.21037/tlcr-24-882/prf
Conflicts of Interest: All authors have completed the ICMJE uniform disclosure form (available at https://tlcr.amegroups.com/article/view/10.21037/tlcr-24-882/coif). All authors report that this research was funded by a National Research Foundation of Korea (NRF) grant funded by the Korean government (MSIP) (No. RS-2023-00243836), and by a grant of the Korea Health Technology R&D Project through the Korea Health Industry Development Institute (KHIDI) funded by the Ministry of Health & Welfare, Republic of Korea (No. RS-2021-KH114109). J.H.H. is employed by Biolink Inc. The authors have no other conflicts of interest to declare.
Ethical Statement: The authors are accountable for all aspects of the work in ensuring that questions related to the accuracy or integrity of any part of the work are appropriately investigated and resolved. The Institutional Review Board of Dongsan Hospital approved this study (No. 2023-01-067) and individual consent for this retrospective analysis was waived. The study was conducted in accordance with the Declaration of Helsinki (as revised in 2013).
Open Access Statement: This is an Open Access article distributed in accordance with the Creative Commons Attribution-NonCommercial-NoDerivs 4.0 International License (CC BY-NC-ND 4.0), which permits the non-commercial replication and distribution of the article with the strict proviso that no changes or edits are made and the original work is properly cited (including links to both the formal publication through the relevant DOI and the license). See: https://creativecommons.org/licenses/by-nc-nd/4.0/.
References
- McGuire S. World Cancer Report 2014. Geneva, Switzerland: World Health Organization, International Agency for Research on Cancer, WHO Press, 2015. Adv Nutr 2016;7:418-9. [Crossref] [PubMed]
- Squires BS, Levitin R, Grills IS. The US Preventive Services Task Force Recommendation on Lung Cancer Screening. JAMA 2021;326:440-1. [Crossref] [PubMed]
- US Preventive Services Task Force. Screening for Lung Cancer: US Preventive Services Task Force Recommendation Statement. JAMA 2021;325:962-70. [Crossref] [PubMed]
- Callister ME, Baldwin DR, Akram AR, et al. British Thoracic Society guidelines for the investigation and management of pulmonary nodules. Thorax 2015;70:ii1-ii54. [Crossref] [PubMed]
- MacMahon H, Naidich DP, Goo JM, et al. Guidelines for Management of Incidental Pulmonary Nodules Detected on CT Images: From the Fleischner Society 2017. Radiology 2017;284:228-43. [Crossref] [PubMed]
- Zhu Y, Yang L, Li Q, et al. Factors associated with concurrent malignancy risk among patients with incidental solitary pulmonary nodule: A systematic review taskforce for developing rapid recommendations. J Evid Based Med 2022;15:106-22. [Crossref] [PubMed]
- Sakoda LC, Henderson LM, Caverly TJ, et al. Applying Risk Prediction Models to Optimize Lung Cancer Screening: Current Knowledge, Challenges, and Future Directions. Curr Epidemiol Rep 2017;4:307-20. [Crossref] [PubMed]
- Gray EP, Teare MD, Stevens J, et al. Risk Prediction Models for Lung Cancer: A Systematic Review. Clin Lung Cancer 2016;17:95-106. [Crossref] [PubMed]
- Shimazaki A, Ueda D, Choppin A, et al. Deep learning-based algorithm for lung cancer detection on chest radiographs using the segmentation method. Sci Rep 2022;12:727. [Crossref] [PubMed]
- Venkadesh KV, Setio AAA, Schreuder A, et al. Deep Learning for Malignancy Risk Estimation of Pulmonary Nodules Detected at Low-Dose Screening CT. Radiology 2021;300:438-47. [Crossref] [PubMed]
- Wu Z, Li X, Zuo J. RAD-UNet: Research on an improved lung nodule semantic segmentation algorithm based on deep learning. Front Oncol 2023;13:1084096. [Crossref] [PubMed]
- Mikhael PG, Wohlwend J, Yala A, et al. Sybil: A Validated Deep Learning Model to Predict Future Lung Cancer Risk From a Single Low-Dose Chest Computed Tomography. J Clin Oncol 2023;41:2191-200. [Crossref] [PubMed]
- National Lung Screening Trial Research Team. Reduced lung-cancer mortality with low-dose computed tomographic screening. N Engl J Med 2011;365:395-409. [Crossref] [PubMed]
- Wang S, Zhou Y, Qin X, et al. Label-free detection of rare circulating tumor cells by image analysis and machine learning. Sci Rep 2020;10:12226. [Crossref] [PubMed]
- Yao Q, Xiao L, Liu P, et al. Label-Free Segmentation of COVID-19 Lesions in Lung CT. IEEE Trans Med Imaging 2021;40:2808-19. [Crossref] [PubMed]
- Wille MM, Thomsen LH, Petersen J, et al. Visual assessment of early emphysema and interstitial abnormalities on CT is useful in lung cancer risk analysis. Eur Radiol 2016;26:487-94. [Crossref] [PubMed]
- Baldwin DR, Duffy SW, Wald NJ, et al. UK Lung Screen (UKLS) nodule management protocol: modelling of a single screen randomised controlled trial of low-dose CT screening for lung cancer. Thorax 2011;66:308-13. [Crossref] [PubMed]
- Guan S, Khan AA, Sikdar S, et al. Fully Dense UNet for 2-D Sparse Photoacoustic Tomography Artifact Removal. IEEE J Biomed Health Inform 2020;24:568-76. [Crossref] [PubMed]
- Li G, Zhang M, Li J, et al. Efficient densely connected convolutional neural networks. Pattern Recognition 2021;109:107610. [Crossref]
- Akay M, Du Y, Sershen CL, et al. Deep learning classification of systemic sclerosis skin using the MobileNetV2 model. IEEE Open J Eng Med Biol 2021;2:104-10. [Crossref] [PubMed]
- Jin X, Xie Y, Wei XS, et al. Delving deep into spatial pooling for squeeze-and-excitation networks. Pattern Recognition 2022;121:108159. [Crossref]
- Duong LT, Nguyen PT, Di Sipio C, et al. Automated fruit recognition using EfficientNet and MixNet. Comput Electron Agric 2020;171:105326. [Crossref]
- White N, Parsons R, Collins G, et al. Evidence of questionable research practices in clinical prediction models. BMC Med 2023;21:339. [Crossref] [PubMed]
- Leoni MLG, Lombardelli L, Colombi D, et al. Prediction of 28-day mortality in critically ill patients with COVID-19: Development and internal validation of a clinical prediction model. PLoS One 2021;16:e0254550. [Crossref] [PubMed]
- Stehouwer N, Rowland-Seymour A, Gruppen L, et al. Validity and reliability of Brier scoring for assessment of probabilistic diagnostic reasoning. Diagnosis (Berl) 2024; Epub ahead of print. [Crossref] [PubMed]
- Muller DC, Johansson M, Brennan P. Lung Cancer Risk Prediction Model Incorporating Lung Function: Development and Validation in the UK Biobank Prospective Cohort Study. J Clin Oncol 2017;35:861-9. [Crossref] [PubMed]
- Integrative Analysis of Lung Cancer Etiology and Risk (INTEGRAL) Consortium for Early Detection of Lung Cancer. Assessment of Lung Cancer Risk on the Basis of a Biomarker Panel of Circulating Proteins. JAMA Oncol 2018;4:e182078. Erratum in: JAMA Oncol 2018;4:1439 Erratum in: JAMA Oncol 2019;5:1811. [Crossref] [PubMed]
- Han SS, Rivera GA, Tammemägi MC, et al. Risk Stratification for Second Primary Lung Cancer. J Clin Oncol 2017;35:2893-9. [Crossref] [PubMed]
- Pan SY, Huang CP, Chen WC. Synchronous/Metachronous Multiple Primary Malignancies: Review of Associated Risk Factors. Diagnostics (Basel) 2022;12:1940. [Crossref] [PubMed]
- Hu ZG, Li WX, Ruan YS, et al. Incidence trends and risk prediction nomogram of metachronous second primary lung cancer in lung cancer survivors. PLoS One 2018;13:e0209002. [Crossref] [PubMed]