Short-term peri- and intra-tumoral CT radiomics to predict immunotherapy response in advanced non-small cell lung cancer
Highlight box
Key findings
• The delta-radiomics model of combined intratumoral and peritumoral region demonstrated optimal performance in predicting immunotherapy response of advanced non-small cell lung cancer (NSCLC) patients.
What is known and what is new?
• The dynamic changes in tumor radiomics features from pre- to post-treatment computed tomography scans can enhance model performance in predicting treatment response.
• A peri- and intra-tumoral radiomics-based explainable model was developed to predict immunotherapy response in advanced NSCLC patients.
What is the implication, and what should change now?
• The developed delta-radiomics models have the potential to assist physicians in improving treatment strategies for advanced NSCLC patients.
Introduction
Compared with chemotherapy, immune checkpoint inhibitors (ICIs) targeting programmed cell death protein-1 (PD-1)/programmed cell death-ligand 1 (PD-L1) significantly improve the overall survival (OS) of advanced non-small cell lung cancer (NSCLC) patients (1-4). Following the results of randomized, open, multicenter clinical trials (5-7), various immunotherapy drugs have been approved by the United States Food and Drug Administration (FDA) and China National Medical Products Administration (NMPA) for the treatment of NSCLC at different stages of the disease. Despite these advancements, a significant challenge remains in identifying which patients will respond to ICIs. Although biomarkers such as PD-L1 expression (8,9), tumor mutational burden (10,11), and tumor-infiltrating lymphocytes (12,13) have shown potential in predicting immunotherapy benefit, their predictive efficiency remains limited (14,15). Therefore, developing effective biomarkers to predict the immunotherapy response and decode the dynamic immune microenvironment and tumor heterogeneity in NSCLC patients is crucial.
Compared with invasive biopsy methods, which can obtain limited tumor tissue specimens, computed tomography (CT) provides a noninvasive approach for detecting, diagnosing, and monitoring lung cancer. In clinical practice, response evaluation criteria in solid tumors (RECIST) version 1.1 (16) remains the primary tool for assessing immunotherapy efficacy, with a focus on changes in tumor size on CT images. However, RECIST 1.1 does not account for tumor heterogeneity, which could offer additional insights into treatment response.
Radiomics techniques decode imaging phenotypes through the quantification of high-throughput features of tumor regions on standard-of-care medical images (17). Compared with conventional qualitative features, radiomics can capture undetectable tumor characteristics with the naked eye, offering important information associated with lung cancer diagnosis, prognosis and therapy prediction to support personalized decision making. Previous studies have shown that pre-treatment tumor radiomics features can reflect clinical efficacy in response to anti-PD-1/PD-L1 therapies in lung cancer (18,19). Recent research has focused on the dynamic changes in tumor radiomics features from pre- to post-treatment CT scans, which can enhance model performance in predicting treatment response (20-22). Thus, we hypothesized that the dynamic changes in CT-based radiomics features before and after treatment, which reveal tumor heterogeneity, could serve as imaging biomarkers for predicting immunotherapy response in advanced NSCLC patients.
In this study, we developed explainable machine learning models using pretreatment tumor radiomics features and delta-radiomics features to predict immunotherapy response in advanced NSCLC patients. Additionally, we compared these models with RECIST 1.1-based measurements of the maximal tumor diameter. We also evaluated progression-free survival (PFS) differences between the low- and high-risk groups defined by these predictive models. Figure 1 illustrates the overall workflow of the study. We present this article in accordance with the TRIPOD reporting checklist (available at https://tlcr.amegroups.com/article/view/10.21037/tlcr-24-973/rc).
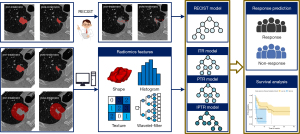
Methods
Study population
The study was conducted in accordance with the Declaration of Helsinki (as revised in 2013). This retrospective study was approved by the institutional review boards of Fudan University Shanghai Cancer Center (FUSCC) (No. 2203252-Exp4) and Shanghai Pulmonary Hospital (SPH) (No. L20-335-2), and the requirement for informed consent was waived due to the retrospective nature of the study. A total of 245 advanced NSCLC patients with clinical stage III or IV only treated with anti-PD-1/PD-L1 therapy between June 2015 and December 2020 at the FUSCC and SPH were included. The majority received immunotherapy alone as second-line or further lines treatment following chemotherapy. The entire treatment process for each patient was reviewed using the electronic medical records system at each center. Each patient underwent a baseline chest CT scan before immunotherapy and a post-treatment CT scan after one to three cycles of immunotherapy, which was downloaded from the Picture Archiving and Communication System (PACS). Patients with poor image quality or indistinguishable borders of the targeted lesions were excluded from the study.
Clinical endpoints
The primary efficacy endpoint was the response status defined by RECIST 1.1, which was classified as progressive disease (PD) or non-PD. Patients who developed at least a 20% relative increase in the sum of the diameters of the target lesions with an absolute increase of not less than 5 mm or any new lesion appearance after immunotherapy were assigned to the PD group and were considered nonresponders. Patients with complete response (CR), partial response (PR) or stable disease (SD) were assigned to the non-PD group and were considered responders. The secondary efficacy endpoint was PFS, which was defined as the time from immunotherapy initiation until progression or death and was censored at the date of the last follow-up for survivors without progression.
CT scanning
Pretreatment and post-treatment CT scans were acquired via multi-slice CT scanners from Siemens (Munich, Germany), Toshiba (Tokyo, Japan), General Electric Medical Systems (Waukesha, USA), Philips (Amsterdam, the Netherlands), or United Imaging Healthcare Company (Shanghai, China). The slice thickness of all the CT images with a matrix of 512×512 pixels was 0.77±0.14 mm (ranging from 0.3 to 1.0 mm), 0.82±0.19 mm (ranging from 0.3 to 1.5 mm), and 1.05±0.15 mm (ranging from 1.0 to 1.5 mm), and the pixel spacing was 0.74±0.07 mm (ranging from 0.59 to 0.98 mm), 0.76±0.07 mm (ranging from 0.62 to 0.96 mm), and 0.78±0.05 mm (ranging from 0.64 to 0.93 mm), respectively, in the training, internal validation, and external validation cohorts.
Tumor segmentation and measurement
The targeted lesion was defined as the largest tumor volume. A senior radiologist (Reader 1) with 10 years of experience annotated the three-dimensional (3D) intratumoral regions (ITR) on pre- and post-treatment CT images slice-by-slice via ITK-SNAP software (http://www.itksnap.org/, version 3.8.0), without access to the patients’ clinicopathological information. To further assess intra- and inter-observer reproducibility, 20 patients randomly selected from the training cohort were re-delineated twice by Reader 1 and another radiologist (Reader 2) with 5 years of experience after four weeks. Additionally, the longest diameter of the target tumor was measured on axial CT images via PACS measurement tools, and the percentage change in tumor diameter between baseline and follow-up CT scans was calculated.
The peritumoral regions (PTR) were obtained by expanding the ITR boundary by 15 mm (23,24), which is considered to be the surgically safe margin (25). The regions, including the air, normal tissue, chest or pericardial cavity, were manually excluded from each PTR by Reader 2. In the end, each target tumor had three regions of interest for subsequent analysis, namely, the ITR, PTR, and combined intratumoral and PTR (IPTR).
Radiomics feature extraction and selection
To ensure comparability, all images were resampled into 1 mm3 isotropic voxels to ensure comparability by applying a B-spline curve interpolation algorithm, which addresses variations in slice thickness and in-plane resolution. Radiomics features were extracted from both the original and wavelet-transformed images via the PyRadiomics package (version 3.0.1). A total of 851 radiomics features were extracted from both the original and wavelet images, including three groups, namely, 18 first-order features, 14 shape features, and 75 texture features, where shape features were only extracted from the original image. The texture features included 24 gray level co-occurrence matrix (GLCM) features, 16 gray level size zone matrix (GLSZM) features, 16 gray level run length matrix (GLRLM) features, 5 neighboring gray tone difference matrix (NGTDM) features, and 14 gray level dependence matrix (GLDM) features. Delta-radiomics features, reflecting the dynamic changes between pretreatment and post-treatment CT images, were computed by subtracting pretreatment CT features from post-treatment CT features and then dividing them by pretreatment CT features.
A two-step feature selection strategy was applied to select optimal radiomics features. First, the radiomics features with intra- and interclass correlation coefficients (ICC) lower than 0.8, indicating poor intra- and inter-observer agreement, were removed. To address scaling differences, all the radiomics features extracted were normalized via the z-score normalization method. Then, the least absolute shrinkage and selection operator (LASSO) with 10-fold cross-validation was employed to eliminate the redundant features and select the optimal features. In addition, the Wilcoxon rank sum test was used to evaluate the differences between the selected features in immunotherapy responders and non-responders.
Explainable prediction model development
The extreme gradient boosting (XGBoost) classifier was employed to develop machine learning models aimed at predicting immunotherapy response in advanced NSCLC patients. The development process included several key steps:
- Pretreatment prediction model: this model was developed using pretreatment radiomics features extracted from baseline CT images. This study aimed to predict immunotherapy response on the basis of tumor characteristics observed before treatment.
- Short-term prediction model: a separate model was developed on the basis of delta-radiomics features, which capture the dynamic changes in tumor characteristics between pre- and post-treatment CT scans. This approach aims to improve prediction accuracy by incorporating information on how the tumor evolves in response to treatment.
- Combined IPTR model: to exploit the complementary information from different tumor regions, we integrated radiomics features from both ITR and PTR into a single XGBoost model, referred to as the combined IPTR model. This approach aims to enhance prediction by leveraging the unique insights provided by each region.
- Comparison with the RECIST model: in addition to the radiomics-based models, we created an RECIST model that focused on tumor size changes according to the RECIST 1.1 criteria. This model was used to compare the performance of radiomics features with that of conventional methods for identifying immunotherapy responders and non-responders.
- Model explanation: to provide interpretability and insight into the decision-making process of the machine learning models, we utilized the SHapley additive exPlanations (SHAP) method (26). SHAP helps explain the contribution of each feature to the model’s predictions, enhancing our understanding of how the models make predictions and ensuring transparency.
These steps were taken to develop robust and interpretable models that not only predict immunotherapy response but also provide insights into the underlying factors driving those predictions.
Performance evaluation and survival analysis
The performance of the established prediction models was thoroughly assessed via several metrics to evaluate their effectiveness in predicting immunotherapy response. First, the discrimination performance was assessed through receiver operating characteristic (ROC) curve analysis and quantified by the area under the curve (AUC), accuracy, weighted average precision, weighted average recall and weighted average F1-score. The corresponding 95% confidence interval (CI) of the AUC was calculated by resampling 1,000 times via the bootstrap approach. In addition, the DeLong test was used to compare AUC values between different models to determine if there were statistically significant differences in their discrimination performance. Second, the calibration performance was assessed through a calibration curve evaluating the agreement between the predicted and actual outcomes. This approach provided insights into how well the model’s predicted probabilities aligned with the observed responses. The Brier score was calculated to evaluate the accuracy of the probabilistic predictions, with lower scores indicating better model calibration. Third, decision curve analysis (DCA) was used to assess the clinical utility of the prediction models by quantifying the net benefit of the models across a range of threshold probabilities. This analysis helps determine the practical value of the models in clinical decision-making.
Patients were categorized into high-risk and low-risk groups on the basis of the optimal cutoff value derived from the ROC curve via the Youden index method. Then, Kaplan-Meier (KM) curves were generated to compare PFS between the high-risk and low-risk groups. Log-rank tests were conducted to evaluate the statistical significance of differences in survival between these groups.
Statistical analysis
To evaluate differences in clinicopathological variables and ensure the robustness of the results, the following statistical methods were used. The Mann-Whitney U test was applied to compare continuous variables between responders and non-responders when the data did not meet the assumption of normality. Fisher’s exact test was used for categorical variables to determine if there were significant associations between clinicopathological features and response to immunotherapy. All the statistical analyses were performed via R software (version 3.5.2). Two-sided tests were conducted with a significance level set at 0.05 to determine statistical significance. Data processing and additional analyses were carried out via the Python programming language (version 3.9), which provides flexibility and advanced capabilities for handling and analyzing complex datasets.
Results
Patient characteristics
Table 1 summarizes the clinicopathological characteristics of the enrolled patients. Of the 245 patients from two medical centers, 115 patients from SPH, 63 patients from SPH, and 67 patients from FUSCC were allocated to the training, internal validation, and external validation cohorts, respectively. As the P values shown in Table 1, no statistically significant differences were observed between responders and non-responders in terms of age, sex, smoking status, histological type, or clinical stage across all cohorts. These findings indicate that the baseline characteristics were comparable between the two groups within each cohort.
Table 1
Variables | Training cohort | Internal validation cohort | External validation cohort | ||||||||
---|---|---|---|---|---|---|---|---|---|---|---|
Responder (n=89) | Non-responder (n=26) | P value | Responder (n=47) | Non-responder (n=16) | P value | Responder (n=50) | Non-responder (n=17) | P value | |||
Age, years | 67.76±8.12 | 62.81±10.31 | 0.07 | 64.79±10.09 | 66.88±7.79 | 0.40 | 61.74±8.19 | 59.53±9.51 | 0.27 | ||
Gender | 0.15 | 0.13 | 0.02* | ||||||||
Female | 13 (14.6) | 7 (26.9) | 6 (12.8) | 5 (31.2) | 8 (16.0) | 8 (47.1) | |||||
Male | 76 (85.4) | 19 (73.1) | 41 (87.2) | 11 (68.8) | 42 (84.0) | 9 (52.9) | |||||
Smoking status | 0.18 | 0.35 | 0.23 | ||||||||
Absent | 47 (52.8) | 18 (69.2) | 31 (66.0) | 13 (81.3) | 14 (28.0) | 8 (47.1) | |||||
Presence | 42 (47.2) | 8 (30.8) | 16 (34.0) | 3 (18.7) | 36 (72.0) | 9 (52.9) | |||||
Histological type | 0.66 | 0.56 | >0.99 | ||||||||
Adenocarcinoma | 50 (56.2) | 16 (61.5) | 20 (42.6) | 5 (31.3) | 40 (80.0) | 14 (82.4) | |||||
Squamous cell carcinoma | 39 (43.8) | 10 (38.5) | 27 (57.4) | 11 (68.7) | 10 (20.0) | 3 (17.6) | |||||
Clinical stage | >0.99 | >0.99 | 0.57 | ||||||||
III | 14 (15.7) | 4 (15.4) | 14 (29.8) | 5 (31.3) | 4 (8.0) | 0 (0.0) | |||||
IV | 75 (84.3) | 22 (84.6) | 33 (70.2) | 11 (68.7) | 46 (92.0) | 17 (100.0) |
Data are presented as mean ± standard deviation or number (frequency). A P value less than 0.05 (marking with *) indicates statistically significant differences between the responder and non-responder groups.
Prediction model evaluation
The ROC curves of all the established models in the training, internal validation, and external validation cohorts are shown in Figure 2. Table 2 summarizes the AUC values for the RECIST model compared with those of the delta-radiomics models. Compared with the RECIST model, the ITR delta-radiomics model and IPTR delta-radiomics model significantly improved the AUC value to 0.85±0.05 (95% CI: 0.74–0.94) and 0.83±0.05 (95% CI: 0.71–0.93) in the internal validation cohort and 0.84±0.06 (95% CI: 0.71–0.94) and 0.86±0.04 (95% CI: 0.78–0.94) in the external validation cohort, respectively. These improvements in the AUC compared with those of the RECIST model were statistically significant, indicating enhanced predictive performance for the delta-radiomics models.
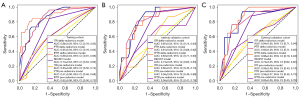
Table 2
Models | AUC (95% CI) | P value |
---|---|---|
Training cohort | ||
RECIST model | 0.73 (0.64–0.80) | – |
ITR delta-radiomics model | 0.86 (0.78–0.93) | <0.001* |
PTR delta-radiomics model | 0.85 (0.76–0.92) | <0.001* |
IPTR delta-radiomics model | 0.89 (0.81–0.96) | <0.001* |
Internal validation cohort | ||
RECIST model | 0.73 (0.63–0.83) | – |
ITR delta-radiomics model | 0.85 (0.74–0.94) | 0.006* |
PTR delta-radiomics model | 0.81 (0.68–0.92) | 0.12 |
IPTR delta-radiomics model | 0.83 (0.71–0.93) | 0.045* |
External validation cohort | ||
RECIST model | 0.73 (0.62–0.82) | – |
ITR delta-radiomics model | 0.84 (0.71–0.94) | 0.02* |
PTR delta-radiomics model | 0.83 (0.71–0.93) | 0.09 |
IPTR delta-radiomics model | 0.86 (0.78–0.94) | 0.02* |
A P value less than 0.05 (marking with *) indicates statistically significant AUC differences between the RECIST model and compared models. RECIST, response evaluation criteria in solid tumors version 1.1; ITR, intratumoral region; PTR, peritumoral region; IPTR, combined intratumoral and peritumoral region; AUC, area under the curve; CI, confidence interval.
The quantitative metrics detailed in Table 3 further support that delta-radiomics models outperform the RECIST model in distinguishing responders from non-responders. The comparison of the AUCs between the pre-radiomics and delta-radiomics models, as shown in Table 4, highlights that multiple-time series models offer superior performance compared with single-time point models. Specifically, delta-radiomics models for ITR, PTR, and IPTR all demonstrated statistically significant improvements in AUCs over pre-radiomics models in all cohorts.
Table 3
Models | Accuracy | Weighted precision | Weighted recall | Weighted F1-score |
---|---|---|---|---|
Training cohort | ||||
RECIST model | 0.77 | 0.60 | 0.77 | 0.68 |
ITR delta-radiomics model | 0.83 | 0.83 | 0.83 | 0.81 |
PTR delta-radiomics model | 0.79 | 0.81 | 0.79 | 0.80 |
IPTR delta-radiomics model | 0.90 | 0.90 | 0.90 | 0.90 |
Internal validation cohort | ||||
RECIST model | 0.75 | 0.56 | 0.75 | 0.64 |
ITR delta-radiomics model | 0.75 | 0.72 | 0.75 | 0.72 |
PTR delta-radiomics model | 0.76 | 0.82 | 0.76 | 0.78 |
IPTR delta-radiomics model | 0.79 | 0.79 | 0.79 | 0.79 |
External validation cohort | ||||
RECIST model | 0.75 | 0.56 | 0.75 | 0.64 |
ITR delta-radiomics model | 0.79 | 0.78 | 0.79 | 0.76 |
PTR delta-radiomics model | 0.82 | 0.84 | 0.82 | 0.83 |
IPTR delta-radiomics model | 0.84 | 0.83 | 0.84 | 0.83 |
RECIST, response evaluation criteria in solid tumors version 1.1; ITR, intratumoral region; PTR, peritumoral region; IPTR, combined intratumoral and peritumoral region.
Table 4
Models | Training cohort | Internal validation cohort | External validation cohort | |||||
---|---|---|---|---|---|---|---|---|
AUC (95% CI) | P value | AUC (95% CI) | P value | AUC (95% CI) | P value | |||
ITR pre-radiomics model | 0.63 (0.50–0.74) | – | 0.60 (0.45–0.73) | – | 0.59 (0.43–0.74) | – | ||
ITR delta-radiomics model | 0.86 (0.78–0.93) | 0.001* | 0.85 (0.74–0.94) | 0.01* | 0.84 (0.71–0.94) | 0.02* | ||
PTR pre-radiomics model | 0.57 (0.46–0.68) | – | 0.56 (0.46–0.68) | – | 0.59 (0.46–0.73) | – | ||
PTR delta-radiomics model | 0.85 (0.76–0.92) | <0.001* | 0.81 (0.68–0.92) | 0.002* | 0.83 (0.71–0.93) | 0.003* | ||
IPTR pre-radiomics model | 0.62 (0.50–0.73) | – | 0.59 (0.41–0.76) | – | 0.62 (0.45–0.78) | – | ||
IPTR delta-radiomics model | 0.89 (0.81–0.96) | <0.001* | 0.83 (0.71–0.93) | 0.048* | 0.86 (0.78–0.94) | 0.02* |
A P value less than 0.05 (marking with *) indicates statistically significant AUC differences between the pre-radiomics model and delta-radiomics model. ITR, intratumoral region; PTR, peritumoral region; IPTR, combined intratumoral and peritumoral region; AUC, area under the curve; CI, confidence interval.
Figure 3 shows the DCA of the established models in all cohorts. The delta-radiomics models consistently showed better clinical utility than both the RECIST model and the pre-radiomics models did. The calibration performance of the delta-radiomics models is further illustrated in Figure 4, where low Brier scores indicate good calibration with minimal prediction error.
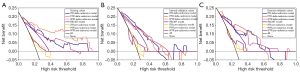
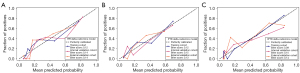
Figure 5 shows the KM survival curves for PFS from the delta-radiomics models. The high-risk groups identified by these models exhibited significantly worse PFS than the low-risk groups across all cohorts did, underscoring the models’ effectiveness in stratifying patients on the basis of their likelihood of responding to immunotherapy.
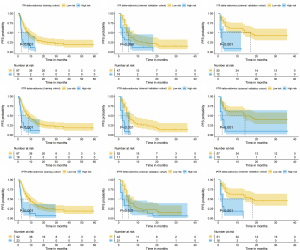
Interpretability of the prediction model
Figure 6A,6B illustrates the differential expression of delta-radiomics features between responders and non-responders for both ITR and PTR. The analysis revealed significant variations in feature values, highlighting the potential of these features in distinguishing between patient groups. Figure 6C displays the SHAP beeswarm summary plot for the IPTR delta-radiomics model. This plot offers a global view of the relationship between delta-radiomics feature values and the predicted probabilities. It visually demonstrates how variations in feature values correlate with the likelihood of predicting immunotherapy response, either positively or negatively. This interpretability enhances the understanding of how specific features impact model predictions and supports the clinical applicability of delta-radiomics models. The SHAP analysis, presented in Figure 6D, identified the importance of individual delta-radiomics features in the IPTR delta-radiomics model. Out of the six optimal features, five were found to have substantial influence on the model’s performance, providing insights into their contributions as input variables.
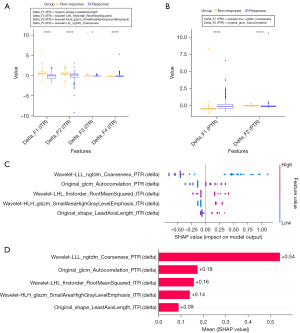
Discussion
Immunotherapy represents a significant advancement in the treatment of advanced NSCLC, particularly for patients without targetable genetic mutations (1). Despite its potential benefits, including improved survival and durable responses compared with those of chemotherapy, immunotherapy still yields low response rates and a risk of immunotoxicity. Consequently, identifying reliable, noninvasive biomarkers to predict treatment response is crucial for optimizing patient selection and therapeutic outcomes.
In this two-center study, we investigated a CT-based radiomics method to predict immunotherapy response for the purpose of optimizing patient selection. We compared the predictive value of single-time point radiomics features with that of delta-radiomics features, which incorporate short-term radiomics feature changes during ICI treatment (21,22). Our results showed that models based on multiple time series (delta-radiomics models) significantly outperformed single-time point models. Specifically, in the internal and external validation cohorts, the ITR delta-radiomics model had AUCs of 0.85 and 0.84, the PTR delta-radiomics model had AUCs of 0.81 and 0.83, and the IPTR delta-radiomics model had AUCs of 0.83 and 0.86. These results highlight a marked improvement over the corresponding pre-radiomics models, which had AUC values ranging from 0.56–0.62 in the internal and external validation cohorts.
CT radiomics features, including tumor-infiltrating CD8+ T cells, somatic mutations, histopathologic grading, and metabolism, have been previously linked to clinical outcomes and tumor biology (27-29). Our study builds on these findings by showing that dynamic changes in radiomics features, captured through delta-radiomics, enhance prediction accuracy and provide valuable prognostic information. Compared with the RECIST model (30), which focuses solely on changes in tumor size, delta-radiomics models offer superior performance and clinical utility in predicting immunotherapy response. This is evident from the improved AUCs, quantitative metrics, and DCA, which collectively indicate superior prediction performance and clinical utility of delta-radiomics models. Among the three delta-radiomics models, the ITR and IPTR models showed nearly identical performance, which was slightly better than that of the PTR model. KM analysis further supported these findings, showing that high-risk groups defined by the delta-radiomics models had significantly worse PFS than low-risk groups did.
An important aspect of our study is the integration of explainability into the prediction models. By utilizing SHAP, we provided insight into how specific delta-radiomics features influence model predictions. SHAP analysis revealed that several key features had a significant impact on model performance, offering a deeper understanding of the relationship between radiomics features and immunotherapy response. The outcome indicates the robustness of the feature selection process in prioritizing biologically relevant and clinically meaningful predictors while minimizing confounding or irrelevant trends. This transparency not only validates the model’s predictions but also helps clinicians interpret the factors contributing to individual patient outcomes, thereby supporting more informed decision-making in clinical practice.
However, there are some limitations in this study. First, patient selection bias and variations in imaging parameters among the two centers could affect model performance. This variability could impact the robustness of the model. Future studies should validate the model via diverse and larger datasets with standardized CT scanning protocols. Second, manual slice-by-slice tumor segmentation was performed manually, relying on radiologists’ subjective experience. Although intra- and inter-observer reproducibility were assessed, there is a need for robust, accurate, and fully automated 3D tumor segmentation algorithms to increase efficiency and reduce labor costs. Third, clinical variables including PD-L1 expression levels and tumor stage was not incorporated into our model development and validation that might contribute to predicting immunotherapy response. Future work will focus on developing a clinical-radiomics fusion model by integrating CT radiomics features with clinical data, such as PD-L1 expression levels and tumor stage. Fourth, this study targeted the largest tumor lesion and overlooked potential impact from other tumor lesions on predicting immunotherapy response. Future studies should explore multi-lesion analyses to better account for intra-tumor heterogeneity and dissociated response. Fifth, while the model developed in this study demonstrates promising potential, it is currently in the research phase and not yet validated for clinical use. Future work is required to expand the dataset, perform prospective validation, and further optimize the model.
Conclusions
In conclusion, we developed an explainable machine learning model based on the dynamic changes of radiomics features between pre- and post-treatment CT scans to predict the response to immunotherapy in advanced NSCLC patients. Our findings indicate that delta-radiomics features, which capture changes over time, provide superior predictive value compared with static pretreatment radiomics features and traditional tumor size measurements. This approach offers a promising tool for enhancing the accuracy of immunotherapy response predictions, thereby potentially improving patient selection and treatment outcomes.
Acknowledgments
None.
Footnote
Reporting Checklist: The authors have completed the TRIPOD reporting checklist. Available at https://tlcr.amegroups.com/article/view/10.21037/tlcr-24-973/rc
Data Sharing Statement: Available at https://tlcr.amegroups.com/article/view/10.21037/tlcr-24-973/dss
Peer Review File: Available at https://tlcr.amegroups.com/article/view/10.21037/tlcr-24-973/prf
Funding: This work was supported by
Conflicts of Interest: All authors have completed the ICMJE uniform disclosure form (available at https://tlcr.amegroups.com/article/view/10.21037/tlcr-24-973/coif). The authors have no conflicts of interest to declare.
Ethical Statement: The authors are accountable for all aspects of the work in ensuring that questions related to the accuracy or integrity of any part of the work are appropriately investigated and resolved. The study was conducted in accordance with the Declaration of Helsinki (as revised in 2013). This retrospective study was approved by the institutional review boards of Fudan University Shanghai Cancer Center (FUSCC) (No. 2203252-Exp4) and Shanghai Pulmonary Hospital (SPH) (No. L20-335-2), and the requirement for informed consent was waived due to the retrospective nature of the study.
Open Access Statement: This is an Open Access article distributed in accordance with the Creative Commons Attribution-NonCommercial-NoDerivs 4.0 International License (CC BY-NC-ND 4.0), which permits the non-commercial replication and distribution of the article with the strict proviso that no changes or edits are made and the original work is properly cited (including links to both the formal publication through the relevant DOI and the license). See: https://creativecommons.org/licenses/by-nc-nd/4.0/.
References
- Doroshow DB, Sanmamed MF, Hastings K, et al. Immunotherapy in Non-Small Cell Lung Cancer: Facts and Hopes. Clin Cancer Res 2019;25:4592-602. [Crossref] [PubMed]
- Rittmeyer A, Barlesi F, Waterkamp D, et al. Atezolizumab versus docetaxel in patients with previously treated non-small-cell lung cancer (OAK): a phase 3, open-label, multicentre randomised controlled trial. Lancet 2017;389:255-65. Erratum in: Lancet 2017;389:e5. [Crossref] [PubMed]
- Reck M, Rodríguez-Abreu D, Robinson AG, et al. Five-Year Outcomes With Pembrolizumab Versus Chemotherapy for Metastatic Non-Small-Cell Lung Cancer With PD-L1 Tumor Proportion Score ≥ 50. J Clin Oncol 2021;39:2339-49. [Crossref] [PubMed]
- Wu YL, Zhang L, Fan Y, et al. Randomized clinical trial of pembrolizumab vs chemotherapy for previously untreated Chinese patients with PD-L1-positive locally advanced or metastatic non-small-cell lung cancer: KEYNOTE-042 China Study. Int J Cancer 2021;148:2313-20. [Crossref] [PubMed]
- Zhou C, Huang D, Yu X, et al. Abstract CT039: Results from RATIONALE 303: A global phase 3 study of tislelizumab (TIS) vs docetaxel (TAX) as second-or third-line therapy for patients with locally advanced or metastatic NSCLC. Cancer Res 2021;81:CT039. [Crossref]
- Garassino MC, Gadgeel S, Esteban E, et al. Patient-reported outcomes following pembrolizumab or placebo plus pemetrexed and platinum in patients with previously untreated, metastatic, non-squamous non-small-cell lung cancer (KEYNOTE-189): a multicentre, double-blind, randomised, placebo-controlled, phase 3 trial. Lancet Oncol 2020;21:387-97. [Crossref] [PubMed]
- Mok TSK, Wu YL, Kudaba I, et al. Pembrolizumab versus chemotherapy for previously untreated, PD-L1-expressing, locally advanced or metastatic non-small-cell lung cancer (KEYNOTE-042): a randomised, open-label, controlled, phase 3 trial. Lancet 2019;393:1819-30. [Crossref] [PubMed]
- Tian P, He B, Mu W, et al. Assessing PD-L1 expression in non-small cell lung cancer and predicting responses to immune checkpoint inhibitors using deep learning on computed tomography images. Theranostics 2021;11:2098-107. [Crossref] [PubMed]
- Hinterleitner C, Strähle J, Malenke E, et al. Platelet PD-L1 reflects collective intratumoral PD-L1 expression and predicts immunotherapy response in non-small cell lung cancer. Nat Commun 2021;12:7005. [Crossref] [PubMed]
- Wang Z, Duan J, Cai S, et al. Assessment of Blood Tumor Mutational Burden as a Potential Biomarker for Immunotherapy in Patients With Non-Small Cell Lung Cancer With Use of a Next-Generation Sequencing Cancer Gene Panel. JAMA Oncol 2019;5:696-702. [Crossref] [PubMed]
- Negrao MV, Skoulidis F, Montesion M, et al. Oncogene-specific differences in tumor mutational burden, PD-L1 expression, and outcomes from immunotherapy in non-small cell lung cancer. J Immunother Cancer 2021;9:e002891. [Crossref] [PubMed]
- Rakaee M, Adib E, Ricciuti B, et al. Association of Machine Learning-Based Assessment of Tumor-Infiltrating Lymphocytes on Standard Histologic Images With Outcomes of Immunotherapy in Patients With NSCLC. JAMA Oncol 2023;9:51-60. [Crossref] [PubMed]
- Gataa I, Mezquita L, Rossoni C, et al. Tumour-infiltrating lymphocyte density is associated with favourable outcome in patients with advanced non-small cell lung cancer treated with immunotherapy. Eur J Cancer 2021;145:221-9. [Crossref] [PubMed]
- Jardim DL, Goodman A, de Melo Gagliato D, et al. The Challenges of Tumor Mutational Burden as an Immunotherapy Biomarker. Cancer Cell 2021;39:154-73. [Crossref] [PubMed]
- Niu M, Yi M, Li N, et al. Predictive biomarkers of anti-PD-1/PD-L1 therapy in NSCLC. Exp Hematol Oncol 2021;10:18. [Crossref] [PubMed]
- Eisenhauer EA, Therasse P, Bogaerts J, et al. New response evaluation criteria in solid tumours: revised RECIST guideline (version 1.1). Eur J Cancer 2009;45:228-47. [Crossref] [PubMed]
- Mayerhoefer ME, Materka A, Langs G, et al. Introduction to Radiomics. J Nucl Med 2020;61:488-95. [Crossref] [PubMed]
- Lin Q, Wu HJ, Song QS, et al. CT-based radiomics in predicting pathological response in non-small cell lung cancer patients receiving neoadjuvant immunotherapy. Front Oncol 2022;12:937277. [Crossref] [PubMed]
- Zhu Z, Chen M, Hu G, et al. A pre-treatment CT-based weighted radiomic approach combined with clinical characteristics to predict durable clinical benefits of immunotherapy in advanced lung cancer. Eur Radiol 2023;33:3918-30. [Crossref] [PubMed]
- Khorrami M, Prasanna P, Gupta A, et al. Changes in CT Radiomic Features Associated with Lymphocyte Distribution Predict Overall Survival and Response to Immunotherapy in Non-Small Cell Lung Cancer. Cancer Immunol Res 2020;8:108-19. [Crossref] [PubMed]
- Farina B, Guerra ADR, Bermejo-Peláez D, et al. Integration of longitudinal deep-radiomics and clinical data improves the prediction of durable benefits to anti-PD-1/PD-L1 immunotherapy in advanced NSCLC patients. J Transl Med 2023;21:174. [Crossref] [PubMed]
- Cousin F, Louis T, Dheur S, et al. Radiomics and Delta-Radiomics Signatures to Predict Response and Survival in Patients with Non-Small-Cell Lung Cancer Treated with Immune Checkpoint Inhibitors. Cancers (Basel) 2023;15:1968. [Crossref] [PubMed]
- Wang T, She Y, Yang Y, et al. Radiomics for Survival Risk Stratification of Clinical and Pathologic Stage IA Pure-Solid Non-Small Cell Lung Cancer. Radiology 2022;302:425-34. [Crossref] [PubMed]
- Jiang Z, Li Q, Ruan J, et al. Machine Learning-Based Prediction of Pathological Responses and Prognosis After Neoadjuvant Chemotherapy for Non-Small-Cell Lung Cancer: A Retrospective Study. Clin Lung Cancer 2024;25:468-478.e3. [Crossref] [PubMed]
- Ettinger DS, Wood DE, Aisner DL, et al. Non-Small Cell Lung Cancer, Version 3.2022, NCCN Clinical Practice Guidelines in Oncology. J Natl Compr Canc Netw 2022;20:497-530. [Crossref] [PubMed]
- Lundberg SM, Nair B, Vavilala MS, et al. Explainable machine-learning predictions for the prevention of hypoxaemia during surgery. Nat Biomed Eng 2018;2:749-60. [Crossref] [PubMed]
- Sun R, Limkin EJ, Vakalopoulou M, et al. A radiomics approach to assess tumour-infiltrating CD8 cells and response to anti-PD-1 or anti-PD-L1 immunotherapy: an imaging biomarker, retrospective multicohort study. Lancet Oncol 2018;19:1180-91. [Crossref] [PubMed]
- Tomaszewski MR, Gillies RJ. The Biological Meaning of Radiomic Features. Radiology 2021;298:505-16. [Crossref] [PubMed]
- Chen Q, Zhang L, Mo X, et al. Current status and quality of radiomic studies for predicting immunotherapy response and outcome in patients with non-small cell lung cancer: a systematic review and meta-analysis. Eur J Nucl Med Mol Imaging 2021;49:345-60. [Crossref] [PubMed]
- Gong J, Bao X, Wang T, et al. A short-term follow-up CT based radiomics approach to predict response to immunotherapy in advanced non-small-cell lung cancer. Oncoimmunology 2022;11:2028962. [Crossref] [PubMed]