Identification and validation of crotonylation-related diagnostic markers for lung adenocarcinoma via weighted correlation network analysis and machine learning
Highlight box
Key findings
• Genes associated with crotonylation may aid in the diagnosis of lung adenocarcinoma and hold promise as novel therapeutic targets.
What is known and what is new?
• Crotonylation plays a crucial role in the occurrence and progression of various tumors.
• We demonstrated that crotonylation has a notable impact on the prognosis of lung adenocarcinoma and the tumor immune microenvironment.
What is the implication, and what should change now?
• Crotonylation holds clinical value for the diagnosis and treatment of lung adenocarcinoma. We should thus clarify the clinical translational value of crotonylation-related targets, especially MMACHC.
Introduction
Lung cancer is one of the most common cancers worldwide in terms of incidence and mortality, with lung adenocarcinoma (LUAD) being the most common subtype (1,2). The mortality rate among patients with LUAD is high, partly because many of these patients are already at an advanced stage at the time of diagnosis when treatment options are limited and the prognosis poor (3-5). Therefore, there is an urgent need to discover new diagnostic markers and therapeutic targets for LUAD treatment.
In 2011, Tan et al. (6) were the first to identify crotonylation modification in the lysine residues of histones using a proteomic technique based on mass spectrometry. As research has progressed, histone and nonhistone crotonylation has been shown to play a significant role in human diseases such as cardiovascular disease (7), human immunodeficiency virus (HIV) infection (8) and malignant tumors such as cervical, prostate or colon cancer (9,10). Enhanced cellular crotonylation boosts transcription, more so than acetylation. The balance between histone crotonylation and acetylation impacts gene expression (11). Relevant experiments have proven that relative levels of acetylation and crotonylation are affected by concentrations of acetyl-CoA and crotonyl-CoA. Increasing crotonyl-CoA concentrations raise intracellular histone crotonylation and reduce acetylation. This shows acetylation and crotonylation have a competitive relationship (11). Xu et al. identified 2,696 lysine crotonylation sites in H1299 cells. Crotonylated non-histone proteins may be linked to multiple signaling pathways and cellular functions, such as ribosome biogenesis and Parkinson’s disease-related pathways (12).
DNA damage and repair have garnered considerable research interest in recent years, and a close relationship between crotonylation modification and DNA damage mechanisms has been demonstrated. Abu-Zhayia et al. found that upon treatment of human osteosarcoma cells with ionizing radiation, ultraviolet radiation, or the use of etoposide, there is a transient decrease in crotonylation modification levels on histone H3K9 (13). The progression of hepatocellular carcinoma (HCC) is closely associated with epigenetic regulatory mechanisms such as DNA methylation and posttranslational modifications. To investigate the role of crotonylation modification in HCC, Wan et al. knocked out HDAC1 and HDAC3 and treated Huh-7 with trichostatin A to increase the overall level of crotonylation modification (14). They confirmed that raising the modification level can inhibit the proliferation capabilities of HCC cells, indicating that crotonylation can serve as a suppressive factor to hinder the progression of HCC.
Interestingly, several studies found that lysine crotonylation expression is down-regulated in liver, stomach and renal cancer and up-regulated in thyroid, esophagus and colon cancer suggesting that lysine crotonylation may play an ambivalent role by regulating different cancer-related key proteins (14,15).
The role of crotonylation modification in LUAD has not yet been clarified. Therefore, our study primarily utilizes WGCNA and machine learning to identify key genes associated with crotonylation in LUAD and to analyze their impact on LUAD prognosis and the tumor immune microenvironment, thereby laying a foundation for subsequent research. We present this article in accordance with the MDAR reporting checklist (available at https://tlcr.amegroups.com/article/view/10.21037/tlcr-2025-204/rc).
Methods
Data processing
The Cancer Genome Atlas (TCGA) provides RNA-sequencing and corresponding clinical data for 33 cancer types, while the Genotype-Tissue Expression (GTEx) database provides expression data from normal tissues. The packages “ggord” and “DESeq2” in R (The R Foundation for Statistical Computing) were used to identify the threshold for messenger RNA (mRNA) differential expression screening and investigate gene expression differences. Furthermore, by employing a relevance threshold above 4 for filtering, we successfully identified 211 genes related to lysine crotonylation from the GeneCards database.
Enrichment of function
Gene Ontology (GO) is a widely used approach for attributing functions to genes, including molecular functions (MF), biological pathways (BP), and cellular components (CC). Meanwhile, Kyoto Encyclopedia of Genes and Genomes (KEGG) analysis can be employed to analyze gene functions. Disease Ontology (DO) analysis is used to describe diseases and their characteristics.
Development of coexpression networks
Samples were clustered to identify any significant outliers according to a method described in the literature (16). High module connection and clinical importance were indicated by a module membership (MM) >0.8 and a gene significance (GS) >0.2, respectively. Gene information for the corresponding module was selected for further investigation (17,18).
Construction of a protein–protein interaction (PPI) network and the identification of hub genes
PPIs were retrieved using the Search Tool for the Retrieval of Interacting Genes (STRING) database and visualized with Cytoscape software. The Core module in the PPI network was extracted via the Molecular Complex Detection plug-in (19). Genes within the core module were considered potential hub genes.
Identification of feature genes
The potential hub genes were used to isolate the feature genes that were used to diagnose LUAD. Support vector machine–recursive feature elimination (SVM-RFE) is a machine learning method that trains on a subset of features across various categories, with the aim of reducing the feature set and identifying the most predictive features (20,21). The “glmnet” package in R was used to conduct least absolute shrinkage and selection operator (LASSO) regression for the selection and computation of linear models. Subsequently, binomial distribution variables were integrated into the LASSO classification (22). The model was constructed using the one-standard-error lambda value for the minimum criterion (1-SE criterion), which ensures good performance with only 10 cross-validation folds. The random forest algorithm was employed to rank the genes, with a relative importance value above 0.25 being considered as indicative of a typical random occurrence (21,23). The intersection of gene lists was subsequently used to identify the most significant feature genes in this study via LASSO regression, SVM-RFE, and random forest.
Kaplan-Meier curve
Kaplan-Meier plotter (24) was employed to evaluate the overall survival (OS) and progression-free interval (PFI) in LUAD for GAPDH, MMACHC, SLC25A13, and HDAC1 and to estimate hazard ratios (HR) and P values.
Immune cell analyses and immune-related features
To determine the correlation between immune cells and genes, the “corrplot” package in R was used to calculate the correlation coefficient. To characterize the immune cell abundance in LUAD tissues, the tumor immune estimation resource (TIMER), cell-type identification by estimating relative subsets of RNA transcripts (CIBERSORT), estimation of stromal and immune cells in malignant tumor tissues using expression data (ESTIMATE), microenvironment cell populations counter (MCPcounter), and xCell algorithms, along with a deconvolution algorithm, were employed to evaluate the proportions of immune cell types (25-29).
Patient samples
Collection and analysis of tumor tissue sections were obtained from 7 patients with LUAD who underwent surgery between 2023 and 2024 at Jiangsu Cancer Hospital. This study was approved by the Ethics Committee of Jiangsu Cancer Hospital (No. KY-2024-073). This study was conducted in accordance with the Declaration of Helsinki (as revised in 2013). All patients provided informed consent.
Cell culture, RNA interference, and cell transfection
Human LUAD cell lines A549 and H1299 were purchased from American Type Culture Collection (ATCC; Manassas, VI, USA). The A549 and H1299 LUAD cell lines were cultured in RPMI-1640 supplemented with 10% fetal bovine serum (FBS; Invigentech, USA) at 37 ℃ in a humidified incubator with 5% CO2. All cell lines underwent authentication through short tandem repeat (STR) profiling and were consistently screened for mycoplasma contamination. The negative control (NC) small interfering RNA (siRNA) and siRNAs targeting MMACHC were transfected into the cells using Lipofectamine 2000 (Invitrogen, USA). The sequences of siRNAs targeting MMACHC were as follows: MMACHC-si sense, 5'-GCAUAUCAGGUGUGUGCAUTT-3'; and MMACHC-si antisense, 5'-AUGCACACACCUGAUAUGCTT-3'. For transfection, the cells were seeded for 24 hours and then exposed to siRNA fragments and control siRNAs for 48 hours.
Cell viability assay
Cells were seeded at a density of 5,000 cells per well in 96-well plates. After addition of Cell Counting Kit-8 (CCK8; KeyGEN BioTECH, China), the plates were incubated at 37 ℃ for 100 min. Absorbance values were measured with a microplate reader (BioTek, USA) at a wavelength of 450 nm. Cell viability was determined using a colony formation assay, and 1×103 cells were plated in six-well plates and allowed to grow for 12 days before being stained with crystal violet.
Transwell assay
Cells were plated in the upper compartment of a Transwell insert, with and without Matrigel (BD Biosciences, Franklin Lakes, NJ, USA) coating. The lower compartment was filled with medium supplemented with 10% FBS. Following a 24-hour incubation period, cells that had migrated to the other side of the membrane were fixed using 4% paraformaldehyde and then stained with crystal violet.
Real-time quantitative polymerase chain reaction
RNA was isolated using TRIzol reagent (Invitrogen), and reverse transcription was performed with the RT SuperMix (cat. No. 11151ES60; Yeasen, Shanghai, China). The quantitative real-time polymerase chain reaction (qRT-PCR) was conducted with SYBR green assay. The primers were as follows: ACTIN forward, 5'-GGGAAATCGTGCGTGACATTAAGG-3'; ACTIN reverse, 5'-CAGGAAGGAAGGCTGGAAGAGTG-3'. GAPDH forward, 5'-GGAGCGAGATCCCTCCAAAAT-3'; GAPDH reverse, 5'-GGCTGTTGTCATACTTCTCATGG-3'. HDAC1 forward, 5'-TGACCAAGTACCACAGCGATGAC-3'; HDAC1 reverse, 5'-TTGCCACAGAACCACCAGTAGAC-3'. MMACHC forward, 5'-ACTGGCGTGATTGGACTTACCG-3'; MMACHC reverse, 5'-AATCGTTGGGCAGGTGGAGTG-3'; SLC25A13 forward, 5'-GGTCTGGGTTCTGTTGCTGGAG-3'; and SLC25A13 reverse, 5'-AGTTGATCGTTGGTTCTGCATTCG-3'.
Apoptosis assay
A total of 100,000 cells were seeded in each well of a six-well plate. Apoptotic cells were detected using an Annexin V-Fluorescein Isothiocyanate (FITC) and propidium iodide (PI) staining kit, followed by analysis with a flow cytometer according to the manufacturer’s protocol (KeyGen BioTECH, Nanjing, China).
T cell-mediated tumor cell-killing assays
Peripheral blood mononuclear cells (PBMCs) were isolated from heparinized venous blood of healthy volunteers via density gradient centrifugation. PBMCs were stimulated for 48 hours using CD3 antibody (HIT3a, 300302; BioLegend, San Diego, CA, USA) and CD28 antibody (CD28.2, 302902; BioLegend, USA), after which the cells were cultured in a serum-free medium supplemented with 20 ng/mL IL-2 protein (Sino Biological, Beijing, China). Following this, the collected PBMCs were cocultured with LUAD cells at a ratio of 8:1 for 48 hours to evaluate immune responses and induce tumor cell apoptosis. Tumor cells were washed with phosphate-buffered saline (PBS) and then with crystal violet. Finally a microplate reader was used to detect the optical density at a wavelength of 570 nm. PBMCs were washed twice with PBS, resuspended in 200 µL of staining buffer, and then stained with antibodies specific for CD3-PerCP-cyanine5.5 (OKT3, 45-0037-42; Invitrogen) and CD8a-PE (RPA-T8,12-0088-42; Invitrogen).
Statistical analysis
All statistical analyses were conducted using R software version 4.2.1, with visualization of the results performed through the “ggplot2” package. The Student’s t-test was employed to ascertain the significance of differences between groups.
Results
Weighted gene coexpression network construction
For sample clustering, the TCGA-LUAD dataset was obtained and comprised 59 normal samples and 539 LUAD samples (Figure 1A). We determined the soft threshold as 7 based on the condition that R2 exceeded 0.9, and the connectivity was sufficiently high (Figure 1B). After combining the highly correlated modules using a clustering threshold of 0.25 (Figure 1C), we delineated a number of distinct modules for further analysis. These primed and consolidated PI modules were eventually displayed beneath the dendrogram (Figure 1D). Following this, an assessment of the correlations among the modules was conducted, with no significant correlations among them being found (Figure 1E). The reliability of module identification was confirmed through intramodular transcription correlation analysis, which indicated no significant intermodular connectivity. Correlations between module eigengenes (MEs) and clinical traits were then examined to clarify the modules’ relationship with clinical manifestations. Notably, the brown module showed a strong positive correlation with normal tissue (r=0.89; P<0.001) and a corresponding negative correlation with LUAD (r=–0.89; P<0.001) (Figure 1F). The blue and dark turquoise modules demonstrated a negative association with normal tissue (r<−0.5; P<0.001) and a positive correlation with LUAD (r>0.5; P<0.001) (Figure 1G,1H). Additionally, we explored the interactions among the elucidated modules by assessing their connectivity degree (Figure 1I).
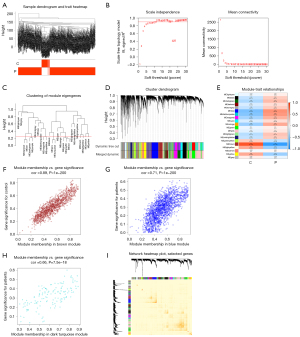
DEGs and functional analysis of hub genes
Data from 539 LUAD and 59 control samples from TCGA-LUAD were retrospectively analyzed in this study. A total of 9,485 DEGs were discovered (log |fold change| ≥0.5 and adjusted P value <0.05) (Figure S1). Upon intersecting the genes from critical modules, crotonylation, and DEGs using a Venn diagram, we identified 17 DEGs (Figure 2A). We conducted functional analyses to gain insights into the biological roles of the DEGs within the modules. The results of GO enrichment analysis revealed that these DEGs were linked to purine ribonucleotide metabolic process, ribonucleotide metabolic process, purine nucleotide metabolic process, purine-containing compound metabolic process, and nucleotide metabolic process, among others (Figure 2B). KEGG analysis was associated with the biosynthesis of amino acids, notch signaling pathway, peroxisome proliferator-activated receptor (PPAR) signaling pathway, and hypoxia-inducible factor-1 (HIF-1) signaling pathway (Figure 2C,2D). The results of DO enrichment revealed that these DEGs were linked to acute kidney failure, amino acid metabolic disorder, and diabetic retinopathy, among others (Figure 2E). As shown in Figure 2F, DEGs participate in regulating the notch signaling pathway.
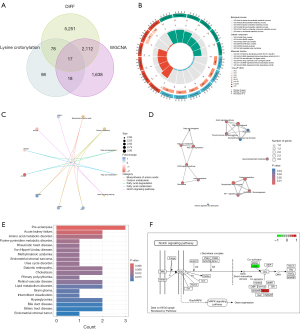
PPI network
PPI analysis was performed with the 17 DEGs via the STRING database (Figure S2A). The relationships among the key genes across the entire network were ascertained (Figure S2B).
Selection of feature genes
We employed three machine learning algorithms to identify feature genes: the SVM-RFE algorithm identified 7 genes (Figure 3A,3B), LASSO regression identified 11 genes from statistically significant univariate variables (Figure 3C), and random forest in conjunction with feature selection clarified the relationship between the error rate and the number of classification trees (Figure 3D,3E) and the 6 genes with relative importance. Via Venn diagram, we identified GAPDH, SLC25A13, MMACHC, and HDAC1 as the genes that overlapped after the above-mentioned procedures were completed (Figure 3F).
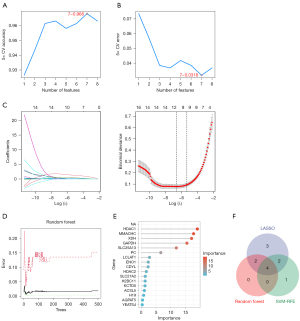
Immunological infiltration in LUAD
The results showed that the enrichment of G2M checkpoint, E2F targets, glycolysis, and DNA repair pathways in the LUAD group was higher than that in the control group (Figure 4A). We recognized that the four hub genes were linked to the G2M checkpoint, E2F targets, MYC targets, mammalian target of rapamycin complex-1 (mTORC1) signaling, and DNA repair pathways, and we used the “corrplot” package to calculate the positive correlation among the signature genes (Figure 4B). The four hub genes were found likely to regulate the immune response during the progression of LUAD.
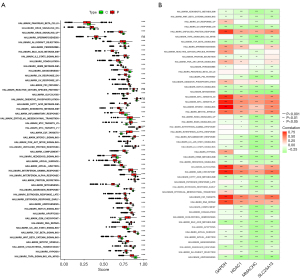
Expression status and prognostic value in pancancer
GAPDH, SLC25A13, MMACHC, and HDAC1 were found to be highly expressed in bladder urothelial carcinoma (BLCA), cervical squamous cell carcinoma and endocervical adenocarcinoma (CESC), LUAD, lung squamous cell carcinoma (LUSC), and uterine corpus endometrial carcinoma (UCEC) in TCGA (Figure 5A-5D). Next, the prognostic effect of GAPDH, MMACHC, SLC25A13, and HDAC1 for pancancer was further evaluated. Misregulation of GAPDH, MMACHC, SLC25A13, and HDAC1 were significantly associated with a poor prognosis. In LUAD, BLCA, CESC, and other cancers, a high level of GAPDH, MMACHC, SLC25A13, and HDAC1 was associated with a worse OS or PFI (Figure 5E-5L). These results indicate that GAPDH, MMACHC, SLC25A13, and HDAC1 are important factors that affect cancer prognosis.
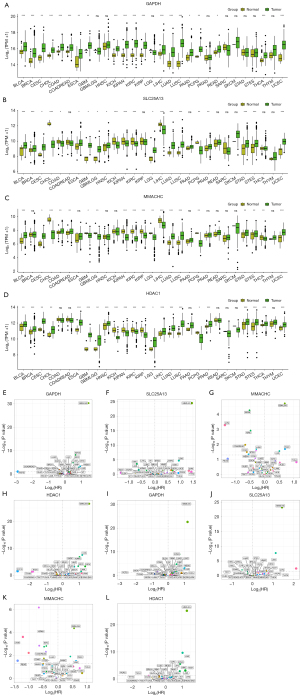
Analysis of immune infiltration
The association between the expression of GAPDH, SLC25A13, MMACHC, and HDAC1 and immune cell infiltration was determined using the TIMER, CIBERSORT, ESTIMATE, MCPcounter, and xCell algorithms. According to the results, the CIBERSORT algorithm revealed that CD8+ T cells in 10 tumors, CD4+ T cells in 4 tumors, neutrophils in 10 tumors, macrophages in 14 tumors, eosinophils in 6 tumors, and B cells in 28 tumors exhibited a strong correlation with GAPDH (Figure 6A). The MCPcounter algorithm indicated that CD8+ T cells in 20 tumors, neutrophils in 18 tumors, natural killer (NK) cells in 26 tumors, endothelial cells in 13 tumors, and native B cells in 15 malignancies had a strong association with SLC25A13 (Figure 6B). CD8+ T cells in 17 tumors, neutrophils in 20 tumors, dendritic cells (DCs) in 23 tumors, and B cells in 11 tumors were found to have a strong correlation with MMACHC, as indicated by the TIMER algorithm (Figure 6C). CD8+ T cells in 12 tumors, neutrophils in 6 tumors, DCs in 21 tumors, and B cells in 11 tumors were found to have a strong correlation with HDAC1, as revealed by the xCell algorithm (Figure 6D). The results of the ESTIMATE analysis indicate that in multiple tumors, including LUAD, the immune score and stromal score are significantly correlated with the expression levels of GAPDH, SLC25A13, MMACHC, and HDAC1 (Figure 6A-6D).
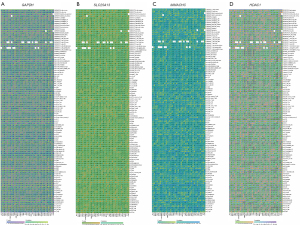
Immune checkpoints analysis
We first analyzed the correlation between GAPDH and 42 immune checkpoints, which indicated a significant correlation between GAPDH and most immune checkpoints in pancancer. In LUAD, GAPDH was positively correlated with cytotoxic T lymphocyte-associated antigen-4 (CTLA4) and programmed cell death protein-1 (PD-1) but and negatively correlated with programmed cell death ligand 1 (PD-L1) (Figure 7A). We then analyzed the correlations between SLC25A13, MMACHC, and HDAC1 and 42 immune checkpoints, identifying significant correlations between SLC25A13, MMACHC, and HDAC1 and most immune checkpoints in pancancer (Figure 7B-7D).
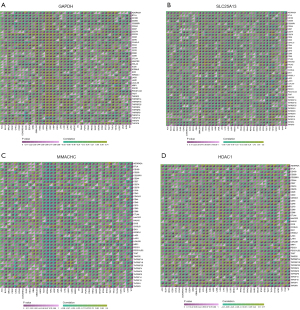
Immune inflammatory response pathway
We then analyzed the correlation between these four genes and immunoinflammatory response pathway in pancancer. The results indicated that these four genes exhibited significant correlations with nearly all immune-inflammatory pathways, suggesting that they may play a pivotal role in the immune-inflammatory response pathways associated with LUAD (Figure 8A-8D). HDAC1 is significantly negatively correlated with a variety of immunoinflammatory pathways in LUAD, including B-cell receptor signaling pathway, chemokine signaling pathway, FC epsilon RI signaling pathway, natural killer (NK) cell-mediated cytotoxicity, T-cell receptor signaling pathway, and toll-like receptor signaling pathway (Figure 8D). We observed similar results to in GAPDH, SLC25A13, and MMACHC, with a significant negative correlation with immunoinflammatory pathways (Figure 8A-8C). These results suggest that GAPDH, SLC25A13, MMACHC, and HDAC1 play a critical role in regulating immune-inflammatory pathways and influencing the occurrence and development of LUAD.
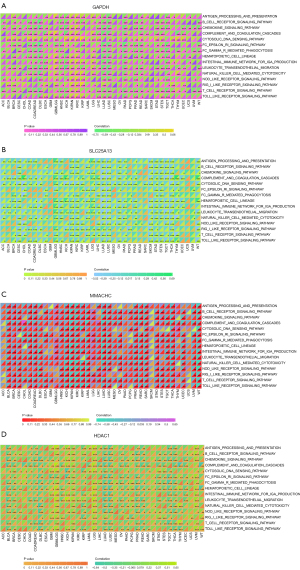
MMACHC knockdown inhibited proliferation and migration, induced apoptosis, and enhanced immunotherapy in LUAD
The findings indicated a substantial increase in HDAC1, GAPDH, MMACHC, and SLC25A13 mRNA expression levels in LUAD compared to paired normal tissues, which was consistent with the bioinformatics analysis outcomes mentioned above in this article (clinical sample size, n=7) (Figure 9A). MMACHC is considered a clinical marker that can be used to predict early prognosis for patients with esophageal squamous cell carcinoma (30). In our study, we examined MMACHC to validate its roles in LUAD cells. The expression level of MMACHC was significantly suppressed when transfected with siRNA-MMACHC (Figure 9B). Through CCK8 assay, it was observed that si-MMACHC had a remarkable inhibitory effect on the proliferation of LUAD (Figure 9C). Subsequently, colony formation assays were performed, which indicated a significant decrease in viability and colony-forming ability in cells with MMACHC knockdown (Figure 9D). Transwell experiments confirmed that the downregulation of MMACHC significantly reduced the migration and invasion rate of A549 and H1299 cells (Figure 9E). Apoptosis assays, complemented by flow cytometry, demonstrated that cells with the downregulation of MMACHC had significantly increased cell apoptosis as compared to control cells (Figure 9F). Ultimately, a T cell-mediated tumor cell-killing assay was conducted to evaluate the impact of MMACHC in modulating the effectiveness of immunotherapy (Figure 10A,10B). It indicated that MMACHC knockdown substantially enhanced the efficacy of immunotherapy. In comparison to that in NCs, the abundance of CD8+ T cells significantly increased following co-culture with si-MMACHC tumor cells (Figure 10C). All experiments were conducted independently in the laboratory three times. In the T cell-mediated tumor cell-killing assay, we performed biological replicates, while for the other experiments, we conducted technical replicates.
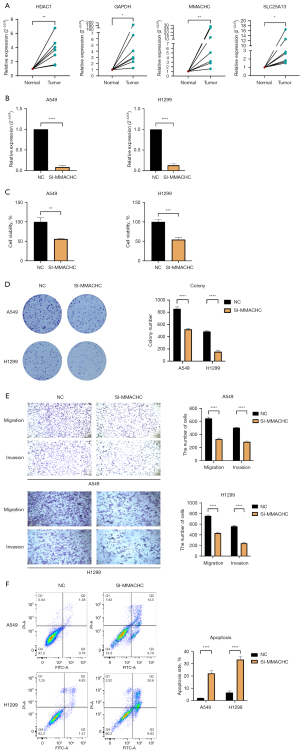
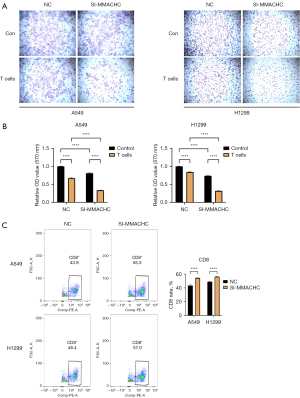
Discussion
As the predominant histological subtype of lung cancer globally, LUAD has exhibited a marked increase in incidence alongside a modest improvement in median survival rate in recent years, with its epidemiological characteristics demonstrating pronounced geographical heterogeneity (31,32). Geographically, East Asia (particularly China) displays a distinct epidemiological pattern characterized by an exceptionally high LUAD incidence among non-smokers, a predominant female patient population, and a progressive annual growth trend—strikingly contrasting with the smoking-associated LUAD predominance observed in Western populations (33). The advancement of precision medicine has further revealed molecular-level geographical disparities, notably the significantly higher epidermal growth factor receptor (EGFR) mutation rates in East Asian patients compared to Western cohorts, which has directly catalyzed the development of targeted therapies (34). Although recent improvements in LUAD survival rates can be partially attributed to rapid advancements in targeted therapies and immunotherapies, drug resistance and suboptimal response rates persist, maintaining LUAD as one of the leading causes of cancer-related mortality worldwide (35). There is an urgent need for novel biomarkers to improve the survival rates of patients with LUAD. In this study, we identified feature genes, including GAPDH, SLC25A13, MMACHC and HDAC1, as crotonylation-related diagnostic markers for LUAD and to analyze the relationship between immune microenvironmental status and these markers.
There is a growing body of evidence supporting the potential of crotonylation modification to play an important role in the regulation of a variety of biological functions from gene expression to telomere function maintenance. High concentrations of crotonic acid in the gastrointestinal tract can regulate transcription levels and protein activity through crotonylation modification, affecting the progression of malignant tumors. This suggests that targeting crotonic acid and crotonylation modification may be viable therapeutic strategies for cancer. Furthermore, with the development of high-throughput detection technologies, it is believed that more crotonylation-modifying enzymes will be identified and that the mechanism of action underlying crotonylation modification in several tumors will be revealed.
Through in vitro experiments, Liao et al. demonstrated that treatment with crotonic acid can suppress p53 activity and enhance the proliferation of colorectal cancer cells (36). Similar studies in prostate and cervical cancers have also demonstrated a correlation between crotonylation modification and the malignant phenotype of tumor cells (9,10).
More recently, Guo et al. study identified key changes in protein crotonylation under hypoxia, particularly in phosphoglycerate kinase 1 (PGK1), which affects glycolysis and mitochondrial metabolism in breast cancer and could serve as a diagnostic marker (37). Additionally, targeting general control non-depressible 5 (GCN5)-mediated crotonylation has been found to be a promising therapeutic strategy for improving the outcome of cancer radiotherapy (38). Higher lysine crotonylation expression has been identified as a predictive marker for lymph node metastasis in thyroid cancer (39).
In addition, it has been reported that the deacetylase-independent function of HDAC1 may be related to the expression of the cell cycle inhibitor protein p21 (40). Moreover, research has confirmed that the gene expression levels of HDAC1 are closely associated with the progression rate of lung cancer and that high expression of the HDAC1 and HDAC3 genes often predicts poor prognosis in LUAD (41). GAPDH is a glycolytic enzyme expressed in almost all tissues. It is commonly used as a housekeeping gene in biological experiments, such as RNA extraction and Western blotting. However, under certain special conditions, such as hypoxia or cell division, GAPDH may consume an abnormally large amount of adenosine triphosphate (ATP), leading to changes in its expression levels (42). Therefore, GAPDH is not considered suitable for use as an internal reference gene for gene expression in tumor cells. Our result also suggests that in LUAD-related research, such as in RNA quantification assays, GAPDH is not suitable as an internal reference gene, as it may affect the accuracy of the results. Research indicates that hypoxia can regulate multiple key genes related to the Warburg effect, inducing a reprogramming of cellular energy metabolism and promoting a shift in glucose metabolism (43). GAPDH may be an important gene involved in the growth of lung cancer cells under hypoxic conditions. Recently, Guéant et al. proposed a mechanism that underlies methionine dependence in the MeWo LC1 cutaneous melanoma cell line, suggesting the involvement of MMACHC, which encodes a crucial protein in cobalamin (vitamin B12) metabolism and its inactivation (44). Our study indicated that MMACHC has a significant impact on the biological characteristics of LUAD. Knocking down MMACHC not only inhibited the proliferative, invasive, and migratory abilities of LUAD but also significantly promoted apoptosis in LUAD. More importantly, knockdown of MMACHC could enhance the efficacy of immunotherapy in LUAD, and thus MMACHC may be a productive research target for immunotherapy in LUAD. SLC25A13, a calcium-regulated mitochondrial inner membrane carrier protein, is highly expressed in most human tumors (45). Previous studies have found that inhibiting the expression levels of SLC25A12/13 significantly suppresses the synthesis of aspartate in tumor cells, thereby inhibiting tumor proliferation, invasion, and migration (46,47).
CD8+ T lymphocytes kill cancer cells by releasing cytotoxic molecules and cytokines, and are major anti-tumor effector cells (48). Currently, a study indicates that the number of tumor-infiltrating CD8+ T cells (TILs) in the tumor microenvironment is a critical prognostic biomarker for cancer (49). Similarly, a meta-analysis studied peripheral blood and CD8+ TILs in solid tumor patients on immunotherapy, and it showed that high-level CD8+ TILs are a good prognostic indicator for patients receiving immune checkpoint inhibitor (ICI) therapy (50). Recently, a study has found that glioblastoma stem cells undergo lysine metabolic reprogramming to produce crotonyl-CoA, increasing the overall crotonylation level, affecting interferon signaling and CD8+ T-cell infiltration, and promoting tumor growth (51). This suggests crotonylation’s potential role in influencing tumor immune evasion and its implications for developing new immunotherapeutic strategies aimed at modulating crotonylation to enhance anti-tumor immunity and improve patient responses to immunotherapy.
We analyzed the expression and prognostic status of GAPDH, SLC25A13, MMACHC, and HDAC1 in pancancer and found that these four genes were significantly overexpressed in various tumors and associated with poor prognosis and a tumor microenvironment. Furthermore, we examined the relationship between the four genes and immune cell infiltration, immune-inflammatory pathways, and immune checkpoints in pancancer and found that all four genes were closely associated with multiple components of the immune microenvironment. Ultimately, our findings suggest that MMACHC plays a role in LUAD and is significantly associated with the malignant phenotype of LUAD and thus holds promise as a target for modulating the efficacy of immunotherapy in LUAD. Bioinformatics analyses have linked MMACHC to several immune cells and pathways. In our co-culture model, MMACHC knockdown was found to enhance CD8+ T-lymphocyte proliferation and immune-mediated tumor cell killing. This makes it a novel target for LUAD immunotherapy and further research.
Despite these encouraging findings, our study had certain limitations. To begin, we did not verify the expression of the four genes in terms of protein level in LUAD and we did not conduct further mechanistic exploration of MMACHC. Moreover, we did not validate the role of MMACHC in LUAD in vivo. These issues will be addressed in our subsequent research.
Conclusions
Our study lays an essential foundation for future clinical treatment and immunotherapy research in LUAD. Crotonylation-related enzymes are expected to become novel targets for LUAD diagnosis and treatment. Moreover, MMACHC could potentially be a diagnostic and therapeutic marker for LUAD.
Acknowledgments
This manuscript was written on behalf of AME Academic Collaborative Group. The authors would like to thank all the reviewers who participated in the review.
Footnote
Reporting Checklist: The authors have completed the MDAR reporting checklist. Available at https://tlcr.amegroups.com/article/view/10.21037/tlcr-2025-204/rc
Data Sharing Statement: Available at https://tlcr.amegroups.com/article/view/10.21037/tlcr-2025-204/dss
Peer Review File: Available at https://tlcr.amegroups.com/article/view/10.21037/tlcr-2025-204/prf
Funding: This research was funded by
Conflicts of Interest: All authors have completed the ICMJE uniform disclosure form (available at https://tlcr.amegroups.com/article/view/10.21037/tlcr-2025-204/coif). L.K. receives unrestricted research grant (NCT05027165) from AstraZeneca, grant from AMGEN, and Art Tempi (MasterClass LucaNext 2023/2024); payment from German Cancer Society (for lecturing), AstraZeneca (for lecturing), and Art Tempi; and gets support for attending meetings from AstraZeneca and ELCC. The other authors have no conflicts of interest to declare.
Ethical Statement: The authors are accountable for all aspects of the work in ensuring that questions related to the accuracy or integrity of any part of the work are appropriately investigated and resolved. This study was conducted in accordance with the Declaration of Helsinki (as revised in 2013), and approved by the Ethics Committee of Jiangsu Cancer Hospital (No. KY-2024-073). All patients provided informed consent.
Open Access Statement: This is an Open Access article distributed in accordance with the Creative Commons Attribution-NonCommercial-NoDerivs 4.0 International License (CC BY-NC-ND 4.0), which permits the non-commercial replication and distribution of the article with the strict proviso that no changes or edits are made and the original work is properly cited (including links to both the formal publication through the relevant DOI and the license). See: https://creativecommons.org/licenses/by-nc-nd/4.0/.
References
- Siegel RL, Giaquinto AN, Jemal A. Cancer statistics, 2024. CA Cancer J Clin 2024;74:12-49. [Crossref] [PubMed]
- Wu A, Zhang A, Wang T, et al. Inhibition of miR-9-3p facilitates ferroptosis by activating SAT1/p53 pathway in lung adenocarcinoma. Transl Lung Cancer Res 2024;13:3426-42. [Crossref] [PubMed]
- Riely GJ, Wood DE, Ettinger DS, et al. Non-Small Cell Lung Cancer, Version 4.2024, NCCN Clinical Practice Guidelines in Oncology. J Natl Compr Canc Netw 2024;22:249-74. [Crossref] [PubMed]
- Meyer ML, Peters S, Mok TS, et al. Lung cancer research and treatment: global perspectives and strategic calls to action. Ann Oncol 2024;35:1088-104. [Crossref] [PubMed]
- Xiao Y, Wang H, Lu J, et al. Characteristics of the immune microenvironment and their clinical significance in lung adenocarcinoma patients with different ALK fusion variants. Transl Lung Cancer Res 2024;13:3538-54. [Crossref] [PubMed]
- Tan M, Luo H, Lee S, et al. Identification of 67 histone marks and histone lysine crotonylation as a new type of histone modification. Cell 2011;146:1016-28. [Crossref] [PubMed]
- Tang X, Chen XF, Sun X, et al. Short-Chain Enoyl-CoA Hydratase Mediates Histone Crotonylation and Contributes to Cardiac Homeostasis. Circulation 2021;143:1066-9. [Crossref] [PubMed]
- Jiang G, Nguyen D, Archin NM, et al. HIV latency is reversed by ACSS2-driven histone crotonylation. J Clin Invest 2018;128:1190-8. [Crossref] [PubMed]
- Xu X, Zhu X, Liu F, et al. The effects of histone crotonylation and bromodomain protein 4 on prostate cancer cell lines. Transl Androl Urol 2021;10:900-14. [Crossref] [PubMed]
- Han X, Xiang X, Yang H, et al. p300-Catalyzed Lysine Crotonylation Promotes the Proliferation, Invasion, and Migration of HeLa Cells via Heterogeneous Nuclear Ribonucleoprotein A1. Anal Cell Pathol (Amst) 2020;2020:5632342. [Crossref] [PubMed]
- Sabari BR, Tang Z, Huang H, et al. Intracellular crotonyl-CoA stimulates transcription through p300-catalyzed histone crotonylation. Mol Cell 2015;58:203-15. [Crossref] [PubMed]
- Xu W, Wan J, Zhan J, et al. Global profiling of crotonylation on non-histone proteins. Cell Res 2017;27:946-9. [Crossref] [PubMed]
- Abu-Zhayia ER, Machour FE, Ayoub N. HDAC-dependent decrease in histone crotonylation during DNA damage. J Mol Cell Biol 2019;11:804-6. [Crossref] [PubMed]
- Wan J, Liu H, Ming L. Lysine crotonylation is involved in hepatocellular carcinoma progression. Biomed Pharmacother 2019;111:976-82. [Crossref] [PubMed]
- Yang P, Qin Y, Zeng L, et al. Crotonylation and disease: Current progress and future perspectives. Biomed Pharmacother 2023;165:115108. [Crossref] [PubMed]
- Niu X, Xu C, Cheuk YC, et al. Characterizing hub biomarkers for post-transplant renal fibrosis and unveiling their immunological functions through RNA sequencing and advanced machine learning techniques. J Transl Med 2024;22:186. [Crossref] [PubMed]
- Langfelder P, Horvath S. WGCNA: an R package for weighted correlation network analysis. BMC Bioinformatics 2008;9:559. [Crossref] [PubMed]
- Liu X, Ren B, Fang Y, et al. Comprehensive analysis of bulk and single-cell transcriptomic data reveals a novel signature associated with endoplasmic reticulum stress, lipid metabolism, and liver metastasis in pancreatic cancer. J Transl Med 2024;22:393. [Crossref] [PubMed]
- Liu Y, Jin J, Chen Y, et al. Integrative analyses of biomarkers and pathways for adipose tissue after bariatric surgery. Adipocyte 2020;9:384-400. [Crossref] [PubMed]
- Huang ML, Hung YH, Lee WM, et al. SVM-RFE based feature selection and Taguchi parameters optimization for multiclass SVM classifier. ScientificWorldJournal 2014;2014:795624. [Crossref] [PubMed]
- Wang H, Cheng W, Hu P, et al. Integrative analysis identifies oxidative stress biomarkers in non-alcoholic fatty liver disease via machine learning and weighted gene co-expression network analysis. Front Immunol 2024;15:1335112. [Crossref] [PubMed]
- Sun D, Lu J, Zhao W, et al. Construction and validation of a prognostic model based on oxidative stress-related genes in non-small cell lung cancer (NSCLC): predicting patient outcomes and therapy responses. Transl Lung Cancer Res 2024;13:3152-74. [Crossref] [PubMed]
- Ishwaran H, Kogalur UB. Consistency of Random Survival Forests. Stat Probab Lett 2010;80:1056-64. [Crossref] [PubMed]
- Wang Y, Liang S, Hong Q, et al. Construction of a neutrophil extracellular trap formation-related gene model for predicting the survival of lung adenocarcinoma patients and their response to immunotherapy. Transl Lung Cancer Res 2024;13:3407-25. [Crossref] [PubMed]
- Newman AM, Liu CL, Green MR, et al. Robust enumeration of cell subsets from tissue expression profiles. Nat Methods 2015;12:453-7. [Crossref] [PubMed]
- Yoshihara K, Shahmoradgoli M, Martínez E, et al. Inferring tumour purity and stromal and immune cell admixture from expression data. Nat Commun 2013;4:2612. [Crossref] [PubMed]
- Li A, Zhang K, Zhou J, et al. Bioinformatics and experimental approach identify lipocalin 2 as a diagnostic and prognostic indicator for lung adenocarcinoma. Int J Biol Macromol 2024;272:132797. [Crossref] [PubMed]
- Shi K, Zhou J, Li M, et al. Pan-cancer analysis of PLAU indicates its potential prognostic value and correlation with neutrophil infiltration in BLCA. Biochim Biophys Acta Mol Basis Dis 2024;1870:166965. [Crossref] [PubMed]
- Huang H, Li Q, Tu X, et al. DNA hypomethylation patterns and their impact on the tumor microenvironment in colorectal cancer. Cell Oncol (Dordr) 2024;47:1375-89. [Crossref] [PubMed]
- Chen K, Lin Z, Shen Y, et al. A novel amino acid metabolism-related gene signature to predict the overall survival of esophageal squamous cell carcinoma patients. J Thorac Dis 2024;16:3967-89. [Crossref] [PubMed]
- Luo G, Zhang Y, Rumgay H, et al. Estimated worldwide variation and trends in incidence of lung cancer by histological subtype in 2022 and over time: a population-based study. Lancet Respir Med 2025;S2213-2600(24)00428-4.
- Corby G, Barclay NL, Tan EH, et al. Incidence, prevalence, and survival of lung cancer in the United Kingdom from 2000-2021: a population-based cohort study. Transl Lung Cancer Res 2024;13:2187-201. [Crossref] [PubMed]
- Chen YJ, Roumeliotis TI, Chang YH, et al. Proteogenomics of Non-smoking Lung Cancer in East Asia Delineates Molecular Signatures of Pathogenesis and Progression. Cell 2020;182:226-244.e17. [Crossref] [PubMed]
- Li S, Wang A, Wu Y, et al. Targeted therapy for non-small-cell lung cancer: New insights into regulated cell death combined with immunotherapy. Immunol Rev 2024;321:300-34. [Crossref] [PubMed]
- Oliver AL. Lung Cancer: Epidemiology and Screening. Surg Clin North Am 2022;102:335-44. [Crossref] [PubMed]
- Liao P, Bhattarai N, Cao B, et al. Crotonylation at serine 46 impairs p53 activity. Biochem Biophys Res Commun 2020;524:730-5. [Crossref] [PubMed]
- Guo Z, Zhang Y, Wang H, et al. Hypoxia-induced downregulation of PGK1 crotonylation promotes tumorigenesis by coordinating glycolysis and the TCA cycle. Nat Commun 2024;15:6915. [Crossref] [PubMed]
- Han Y, Zhao H, Li G, et al. GCN5 mediates DNA-PKcs crotonylation for DNA double-strand break repair and determining cancer radiosensitivity. Br J Cancer 2024;130:1621-34. [Crossref] [PubMed]
- Li Z, Li J, Li F, et al. Potential functions and mechanisms of lysine crotonylation modification (Kcr) in tumorigenesis and lymphatic metastasis of papillary thyroid cancer (PTC). J Transl Med 2024;22:874. [Crossref] [PubMed]
- Liu PY, Chan JY, Lin HC, et al. Modulation of the cyclin-dependent kinase inhibitor p21(WAF1/Cip1) gene by Zac1 through the antagonistic regulators p53 and histone deacetylase 1 in HeLa Cells. Mol Cancer Res 2008;6:1204-14. [Crossref] [PubMed]
- Minamiya Y, Ono T, Saito H, et al. Expression of histone deacetylase 1 correlates with a poor prognosis in patients with adenocarcinoma of the lung. Lung Cancer 2011;74:300-4. [Crossref] [PubMed]
- Zhang JY, Zhang F, Hong CQ, et al. Critical protein GAPDH and its regulatory mechanisms in cancer cells. Cancer Biol Med 2015;12:10-22. [Crossref] [PubMed]
- Loo JM, Scherl A, Nguyen A, et al. Extracellular metabolic energetics can promote cancer progression. Cell 2015;160:393-406. [Crossref] [PubMed]
- Guéant JL, Chéry C, Oussalah A, et al. APRDX1 mutant allele causes a MMACHC secondary epimutation in cblC patients. Nat Commun 2018;9:67. [Crossref] [PubMed]
- Amoedo ND, Punzi G, Obre E, et al. AGC1/2, the mitochondrial aspartate-glutamate carriers. Biochim Biophys Acta 2016;1863:2394-412. [Crossref] [PubMed]
- Rabinovich S, Silberman A, Adler L, et al. The mitochondrial carrier Citrin plays a role in regulating cellular energy during carcinogenesis. Oncogene 2020;39:164-75. [Crossref] [PubMed]
- Infantino V, Dituri F, Convertini P, et al. Epigenetic upregulation and functional role of the mitochondrial aspartate/glutamate carrier isoform 1 in hepatocellular carcinoma. Biochim Biophys Acta Mol Basis Dis 2019;1865:38-47. [Crossref] [PubMed]
- Farhood B, Najafi M, Mortezaee K. CD8(+) cytotoxic T lymphocytes in cancer immunotherapy: A review. J Cell Physiol 2019;234:8509-21. [Crossref] [PubMed]
- Jansen CS, Prokhnevska N, Master VA, et al. An intra-tumoral niche maintains and differentiates stem-like CD8 T cells. Nature 2019;576:465-70. [Crossref] [PubMed]
- Li F, Li C, Cai X, et al. The association between CD8+ tumor-infiltrating lymphocytes and the clinical outcome of cancer immunotherapy: A systematic review and meta-analysis. EClinicalMedicine 2021;41:101134. [Crossref] [PubMed]
- Yuan H, Wu X, Wu Q, et al. Lysine catabolism reprograms tumour immunity through histone crotonylation. Nature 2023;617:818-26. [Crossref] [PubMed]