Single cell RNA-seq and bulk RNA-seq analysis identifies MUC1 as a key gene for lung adenocarcinoma to neuroendocrine transformation
Highlight box
Key findings
• This research identifies mucin-1 (MUC1) as a key gene in the transition from lung adenocarcinoma (LUAD) to neuroendocrine carcinoma (NEC) and explores immune cell infiltration patterns.
What is known and what is new?
• Histologic transformation occurs in 2–15% of LUAD patients after epidermal growth factor receptor tyrosine kinase inhibitor (EGFR-TKI) failure and is now seen as an important resistance mechanism.
• Reduced expression of MUC1 may be associated with unfavorable prognoses and the progression of LUAD, playing a critical role in the histopathological transition from LUAD to NEC. Additionally, the notable correlation between MUC1 expression and the presence of CD8+ T cells suggests a potential connection between the immune microenvironment and cellular transformation.
What is the implication, and what should change now?
• These findings pave the way for future research on molecular mechanisms and therapies targeting MUC1 in the transition from LUAD to NEC.
Introduction
Lung cancer is the second most commonly diagnosed cancer and continues to be the primary cause of cancer-related deaths globally (1). Non-small cell lung cancer (NSCLC) and neuroendocrine carcinoma (NEC) are the predominant subtypes of this disease. Tyrosine kinase inhibitors (TKIs) targeting the epidermal growth factor receptor (EGFR) have shown notable advancements in the survival rates and overall prognosis of patients with EGFR-mutant lung cancer when used as initial treatment. Osimertinib, an EGFR-TKI, has been approved as a first-line treatment for patients with metastatic EGFR mutant lung cancers (2). In contrast, anti-programmed cell death protein 1 (PD-1) and programmed death-ligand 1 (PD-L1) immunotherapy has emerged as a promising option for patients with advanced driver gene-negative NSCLC. Despite advancements in targeted therapies and immunotherapy, acquired drug resistance inevitably occurs in almost all EGFR TKI-treated patients, resulting in poor prognosis (3).
Most EGFR-TKI resistance develops due to secondary EGFR mutations and bypass pathway activation (4). Histologic transformation, which happens in 2–15% of lung adenocarcinoma (LUAD) patients following EGFR-TKI failure, is becoming increasingly recognized as an additional mechanism of EGFR-TKI resistance (5). A study has demonstrated that epithelial-mesenchymal transition and phosphoinositide-3-kinase/Akt serine/threonine kinase (PI3K/AKT) pathway are implicated in this transition, suggesting a potential for targeted interventions (6). Furthermore, in addition to the histological transition from LUAD to small cell lung carcinoma (SCLC), several recent studies have documented transitions to uncommon histologic phenotypes, including large cell NECs and squamous cell carcinomas. Lung neuroendocrine tumor accounts for about 20% of all primary lung cancers and encompass carcinoid, large cell NECs, and SCLC. However, the molecular mechanisms and key drivers of the LUAD to NEC transition remain largely elusive.
Furthermore, the role of the tumor microenvironment and immune evasion strategies employed by these cancers has been increasingly recognized, offering new avenues for therapeutic strategies (7). Evidently, the histological transformation of NSCLC into SCLC is also related to immunotherapy resistance. Increasing evidence shows that tumor immune microenvironment plays a vital role in the clinical treatment of NSCLC (8,9). However, the role of immune microenvironment for histological transformation remains poorly unexplored.
This study utilized a thorough bioinformatics analysis to identify potential candidate genes linked to the transformation of SCLC, as well as to investigate the involvement of immunology in histological transformation. We present this article in accordance with the TRIPOD reporting checklist (available at https://tlcr.amegroups.com/article/view/10.21037/tlcr-24-806/rc).
Methods
Bulk RNA sequencing (RNA-seq) data acquisition and analysis
In preparation for Mendelian randomization (MR) analysis, candidate genes were screened using the GSE64322 dataset, which comprises patient-derived cell lines exhibiting transformation into SCLC. The GSE64322 dataset was obtained from the National Centre for Biotechnology Information (NCBI) Gene Expression Omnibus (GEO) repository. The platform utilized in GSE64322 was the GPL570 [HG-U133_Plus_2] Affymetrix Human Genome U133 Plus 2.0 Array.
Transcriptomic data of LUAD-to-SCLC transformation samples is from a published article, “Multiomic Analysis of Lung Tumors Defines Pathways Activated in Neuroendocrine Transformation” by Alvaro Q.V. and other researchers (6). The dataset contains control LUADs (n=11); pre-transformation or combined LUADs (n=11) and post-transformation or combined SCLCs (n=11); and control SCLCs (n=16).
Identification of differentially expressed genes (DEGs)
Initially, DEGs were identified from the GSE64322 dataset and LUAD-to-SCLC transformation samples using the R package “limma” with criteria of |log fold change (FC)| >2 and P value <0.05. Subsequently, an intersection of genes was performed between the DEGs from the two datasets to determine candidate genes. Volcano plots illustrating the DEGs were generated using the “ggplot2” package.
Single-cell data source and preprocessing
The scRNA-seq profile of LUAD was obtained from a published dataset by Philip Bischoff and colleagues (10), accessible as a Code Ocean capsule at https://doi.org/10.24433/CO.0 121060.v1. The scRNA analysis in this study included a total of six samples, comprising three LUAD tissues from Code Ocean and three lung carcinoid tissues from GSE196303. The Seurat package within R software (version 4.3.1) was utilized for processing the expression matrix of the scRNA-seq data. Quality control measures were implemented, requiring cells to have a minimum of 200 features and at least 3 cells. A total of 16,765 filtered cells were subsequently utilized for further bioinformatics analysis.
Functional annotation of DEGs
The Gene Ontology (GO) encompasses three key aspects: biological processes, molecular functions, and cellular components. The biological functional enrichment analysis of candidate genes was conducted utilizing the “clusterProfiler” R package and “org.Hs.eg.db” R package based on the GO and Kyoto Encyclopedia of Genes and Genomes (KEGG) databases, with a significance threshold set at P<0.05.
Construction of protein-protein interaction (PPI) network and hub-gene extraction
Utilizing the intersected DEGs obtained above, a protein network was constructed to illustrate the relationships among proteins through the utilization of the STRING database (https://string-db.org/). The Cytoscape software was employed to visually represent the protein network, and key modules within the PPI network were identified using CytoNCA.
MR analyses and colocalization
MR analyses were performed utilizing the TwoSampleMR R package, with single nucleotide polymorphisms (SNPs) filtered based on a P value <5×10-8, an r2 cut-off of 0.001, and a distance window threshold of 10 kb. Subsequently, harmonization was carried out to align each SNP of exposure and outcome with the same allele. Various MR methods, such as simple mode, weighted median, weighted mode, the inverse-variance-weighted (IVW), and Egger regression (MR-Egger), were chosen for the analysis.
To identify statistically significant MR results in two related cohorts, we conducted a Bayesian colocalization analysis using cis-expression quantitative trait loci (eQTL) and the coloc R package. A 100-kilobase region encompassing the relevant eQTL was extracted from genome-wide association study data for each gene of interest.
Immunohistochemistry (IHC)
Tissues were fixed using 4% paraformaldehyde and then embedded in paraffin (11). Following this, the slides underwent blocking with goat serum at 37 ℃ for 1 hour and were subsequently exposed to the primary antibody (Mouse anti-MUC1, cat. no. 4538; Cell Signaling Technology, Inc., Boston, USA) overnight at 4 ℃. A secondary antibody was then applied for 1 hour at room temperature. Finally, staining with diaminobenzidine (DAB, GK600510, Gene Tech, Shanghai, China) and hematoxylin was carried out. The slides were air-dried, sealed, and examined using a microscope.
Patients
A cohort of 377 patients diagnosed with LUAD was examined in this retrospective study conducted at the Department of Pathology of Guangdong Provincial People’s Hospital from 2003 to 2016. The clinical characteristics of the patients are detailed in Table S1. The two patients with LUAD-to-SCLC were recruited from Zhuzhou Hospital, which is affiliated with Xiangya School of Medicine. IHC analyses were performed on paraffin-embedded tissue sections. Prior to participation, patients provided informed written consent. The study was conducted in accordance with the Declaration of Helsinki (as revised in 2013). This study was approved by the Ethics Committee of Guangdong Provincial People’s Hospital (No. KY-Z-2021-177) and informed consent was taken from all the patients.
Weighted gene co-expression network analysis (WGCNA)
The WGCNA method was utilized to detect co-expression gene modules within immune cell data and to establish weighted co-expression networks. The optimal soft threshold power (β) was determined, and Pearson correlation coefficients were computed between the modules and immune cells. Subsequently, the expression matrix was transformed into an adjacency matrix and a topological overlap matrix (TOM). Gene modules were then clustered based on the eigengenes of each module, using the criteria of deepSplit =2 and minModuleSize =50.
Immune cell infiltration analysis
In this research, we incorporated the mRNA expression profiles of 21 distinct immune cell subsets and transcriptomic data from patients with LUAD and SCLC to assess the relative abundance of immune cells utilizing the cell-type identification by estimating relative subsets of RNA transcripts (CIBERSORT) algorithm. The ESTIMATE R package was employed to evaluate stromal cell scores, immune cell scores, and tumor purity. Subsequently, Pearson correlation coefficients and associated P values were computed. Additionally, the expression levels of immune-checkpoint related genes were investigated in groups with high and low gene expression.
Single-cell trajectory analyses
Monocle 3 was utilized to generate cell trajectories for various cell clusters, resulting in the establishment of a pseudotime ordering reflective of cell differentiation and transformation. Specifically, data from the Seurat object was extracted and single-cell differentiation trajectories were conducted utilizing variable genes identified by Monocle.
Cell-cell interaction analyses
The CellChat 2 package was employed to analyze the intercellular communication networks involving B cells, T cells, macrophages, natural killer (NK) cells, monocytes, neurons, endothelial cells, smooth muscle cells, and epithelial cells (12). Initially, the ‘CellChatDB.human’ function was utilized to assess the primary signaling interactions within all cell clusters. Subsequently, receptors and ligands expressed in over 10 cells within a given cell cluster were selected for further examination.
Statistical analysis
Statistical significance was assessed through the utilization of Student’s t-test or analysis of variance (ANOVA), with a significance threshold set at a P value of less than 0.05. Bioinformatics and MR analyses were conducted utilizing R software (version 4.1.3).
Results
Identification and analysis of DEGs
A total of 132 DEGs were identified in samples undergoing transformation from LUAD to SCLC in the GSE64322 dataset, based on the specified cut-off criteria. The Venn diagram illustrates that 132 genes were found to overlap between the two datasets (Figure 1A).
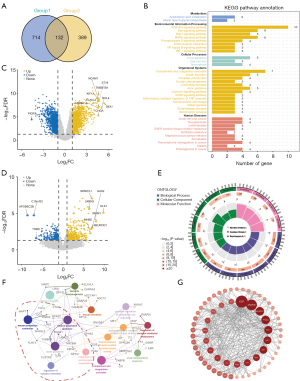
GO and KEGG pathway enrichment of DEGs
The DEGs were further investigated through GO and KEGG enrichment analyses. The KEGG pathway analysis revealed significant enrichment of DEGs in various pathways including Mucin type O-glycan biosynthesis, PI3K/Akt signaling, ECM-receptor interaction, focal adhesion, Axon guidance, EGFR-TKI resistance, transcriptional misregulation in cancer, and SCLC (Figure 1B). Additionally, volcano plots were generated to visually represent the DEGs in the LUAD-to-SCLC transformation samples and GSE64322 datasets (Figures 1C,1D).
GO analysis revealed enrichment of biological processes related to regulation of neuron projection development, axon development, trans-synaptic signaling, nervous system development, neurogenesis, and neurotransmitter secretion. Additionally, cellular components such as neuronal cell bodies, secretory granule membranes, and secretory granule lumen were significantly enriched (Figure 1E). Spearman correlation analysis was conducted on the top 10 up- or down-regulated DEGs. Notably, neurocrine-related genes exhibited significant enrichment and were highlighted in Figure 1F. In the PPI network, 132 DEGs were integrated from the STRING database. The PPI network was visualized using Cytoscape software, revealing the top 5 genes, including EGF, SNAP25, NEUROD1, PTGS2, mucin-1 (MUC1) (Figure 1G).
MR analysis
The cohort for SCLC comprised 2,664 patients and 21,444 controls sourced from ebi-a-GCST004746. Utilizing a two-sample MR analysis on 132 DEGs and SCLC, a negative correlation was observed between MUC1 and SCLC (Figure 2A,2B). Notably, both the weighted median and IVW methods demonstrated a significant relationship between MUC1 and SCLC (Figure 2C), thereby providing additional evidence for the causal association between MUC1 and SCLC.
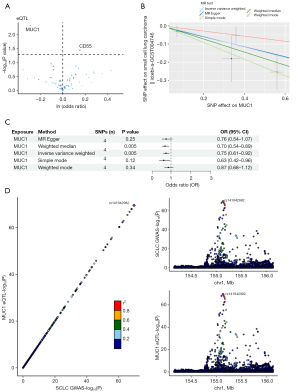
Bayesian colocalization analysis
A Bayesian colocalization analysis was performed to assess the likelihood of shared causal genetic variants between an SNP locus associated with cis-eQTL and SCLC. The findings indicated a probable shared causal variant within the rs141942982 locus between MUC1 and SCLC (Figure 2D). Additionally, MUC1 was identified as a key gene associated with the transformation from LUAD to SCLC through MR analysis.
The expression of MUC1 in clinical samples
In order to investigate the correlation between MUC1 expression and the transformation of LUAD to SCLC, immunohistochemical staining was conducted on tissue sections (Figure S1A). Subsequent to treatment, responsive patients exhibited a notable decrease in disease activity, accompanied by a significant downregulation of MUC1 expression in transformed SCLC in patient 2. Conversely, non-responsive patients displayed a modest downregulation of MUC1 after treatment in transformed SCLC in patient 1. The findings of this study validated the reduced expression of MUC1 in transformed SCLC, aligning with the outcomes of prior bioinformatics analyses.
Association between MUC1 expression and clinicopathologic characteristics in LUAD
The clinicopathologic characteristics of the 377 LUAD patients are summarized in Table S1. A Cox regression analysis of clinicopathologic variables revealed a statistically significant association between metastasis, age, clinical stage, N classification, and the differentiation of MUC1 expression levels (low versus high) in LUAD patients. Representative images depicting histopathological slides for MUC1 are presented in Figure S1B. The predictive capability of MUC1 was evaluated through receiver operating characteristic (ROC) analysis, yielding an AUC of 0.819 [95% confidence interval (CI): 0.775–0.864; Figure S1C].
WGCNA analysis and related key module identification
In order to investigate the co-expression relationships among genes at a systems level, we conducted a WGCNA. Initially, a sample tree was generated through sample clustering, and no outlier samples were detected during this process (Figure 3A; Figure S1D,S1E). Following this, a soft threshold of 9 was identified as optimal for constructing a scale-free network (Figure S1F). It is noteworthy that the majority of the top 6 modules exhibited a decreasing trend, with the exception of the brown module, which showed an increase in expression levels post-transformation (Figure 3B). Additionally, the analysis of GO terms revealed a significant enrichment of terms within the top six modules (Figure 3C). A total of 21 modules were identified through weighted gene co-expression network analysis (Figure 3D).
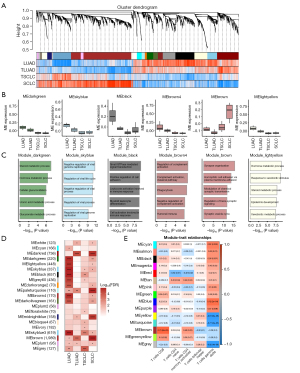
In order to elucidate the contribution of the immune microenvironment to the T cell-mediated trans-differentiation effect, we conducted WGCNA on pre-transformed LUAD and post-transformed SCLC samples. Our findings indicate a strong correlation between CD8+ T cells and the brown module compared to other immune cell types (Figure 3E). Consequently, we focused our subsequent analysis on CD8+ T cells to investigate their role in the initiation of transformation.
Correlation analysis of MUC1 with immune infiltration
In order to explore the association between MUC1 expression and the immune microenvironment, the CIBERSORT database was utilized to assess the infiltration of 21 distinct immune cell types in tissues undergoing LUAD-SCLC transformation. The analysis revealed a positive correlation between MUC1 expression and Macrophages M0 and Mast cells resting, while a negative correlation was observed with T cells CD8, T cells follicular helper, and Macrophages M1 (Figure 4A,4B). We conducted a comparative analysis of the infiltration levels of 21 immune cells between groups with low and high MUC1 expression using CIBERSORT (Figure 4C). Correlation analysis revealed that the abundance of CD8+ T cells was notably lower in the high MUC1 expression group (Figure 4C). Additionally, ImmuneScore, ESTIMATEScore, StromalScore, and TumorPurity were computed. Figure 4D demonstrates that patients in the MUC1 low-expression cohort exhibit decreased ImmuneScore, ESTIMATEScore, and StromalScore, as well as elevated TumorPurity. Additionally, an investigation into the relationship between immunological checkpoints and MUC1 expression was conducted (Figure 4E), revealing significant associations with CD247, HAVCR2, and PDCD1LG2. These findings indicate the potential regulatory effects of MUC1 on immune checkpoints in lung cancer.
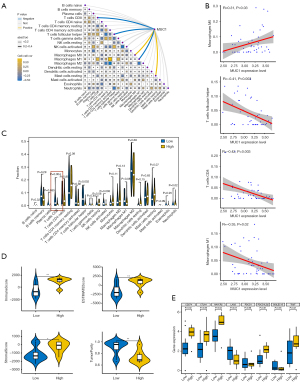
Cellular constitution of LUAD and NEC in scRNA-seq
In this study, a total of six single cell samples, consisting of three SCLC samples and three neuroendocrine tumor samples, were analyzed. The integrated data underwent PCA downscaling, retaining the top nine dimensions of cells. Figure 5 presents an overview of the single cell data for both LUAD samples and neuroendocrine tumors.
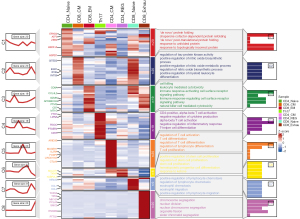
The visualization of Uniform Manifold Approximation and Projection (UMAP) clustering revealed the partitioning of 16,765 cells into 9 distinct subgroups, as depicted in Figure 6A. Identification of cell types was achieved through marker gene analysis, resulting in the classification of 9 cell types, namely B cells, endothelial cells, epithelial cells, macrophages, monocytes, neutrophils, NK cells, smooth muscle cells, and T cells. To further investigate the heterogeneity of T cells during this transformation, sub-clustering and annotation were performed, as illustrated in Figure 6B. The visualization of the UMAP cluster demonstrated the division of T cells into 8 distinct subgroups, with a significant reduction observed in the majority of T cell types.
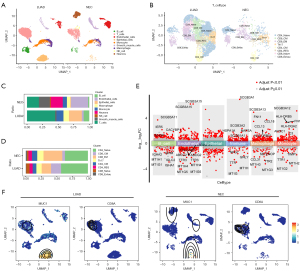
Subsequently, in order to analyze the cellular composition differences between the two groups, we quantified the proportion of each predominant cell type, as depicted in Figure 6C. Our findings indicated a notable decrease in T cells and endothelial cells and a marked increase in neuron cells in NEC compared to LUAD (Figure 6C). Following a notable decrease in T cell population, a detailed examination of T cell subtypes was conducted. The findings revealed that CD8_CM (central memory) and CD8_EM (effector memory) T cells exhibited complementary expression patterns between the two subtypes, as illustrated in Figure 6D. These results suggest a potential significance of T cells in the transformation from LUAD to SCLC. Volcano plot representations of the illustrated clusters are shown in Figure 6E. Consistent with previous RNAseq findings, a reduction in MUC1+ epithelial cells was observed in NEC in Figure 6F.
Pseudotime trajectory in scRNA-seq
To substantiate the developmental progression of distinct cell subsets, the Monocle3 package was employed for pseudo-time series analysis in NEC. The findings indicated that the nine clusters can be categorized into three distinct differentiation states (Figure 7A). Additionally, Figure 7B illustrates the temporal progression of MUC1-expressing cells, with darker colors representing earlier developmental stages. The findings indicated that MUC1 was highly expressed in epithelial cells and a limited number of T cells. Figure 7C illustrates the top 10 DEGs across pseudotime. The heatmap in Figure 7D demonstrates the evolving patterns of DEGs over pseudotime. The expression patterns of NEC along the pseudotime trajectory were hierarchically grouped into four subclusters. A comprehensive annotation was performed on a set of 21 genes, comprising the top 20 genes along with MUC1, as depicted on the left, and GO terms were provided for each cluster (right).
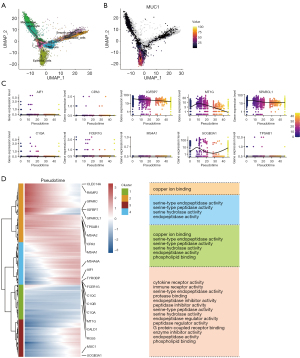
Cell-cell interaction analysis in scRNA-seq
In order to investigate potential cell-to-cell interactions, we utilized CellChat to examine alterations in intercellular communications (Figure 8A). Analysis of ligand-receptor interactions revealed associations between various cell types, including epithelial cells and neurons (Figure 8B). Furthermore, we constructed a cell-cell communication network by integrating specific signaling pathways and ligand-receptor interactions (Figure 8B). The findings indicated that interactions were predominantly enriched in several signaling pathways, including VEGF, TGFβ, MHC-II, and LAMININ (Figure 8C). Specifically, our analysis revealed that microphages and monocytes are pivotal in the TGFβ signaling pathway communication network. Additionally, the study plotted the outgoing communication patterns of secreting cells, demonstrating the relationship between key genes and cell groups (Figure 8D).
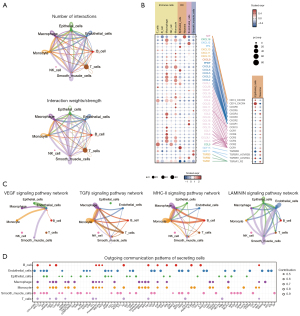
Discussion
Several recent studies have investigated the transcriptomic characteristics of LUAD-SCLC transformation (6,13,14). However, there is a gap in the literature regarding the integration of bulk RNA-seq data and single-cell RNA data for a comprehensive analysis of neuroendocrine transformation. In this study, we integrated the largest available RNA-seq dataset of pre/post-transformation clinical samples with single-cell RNA-seq data from LUAD and NEC tumors. Elucidating the phenotype-genotype correlations in this context has the potential to advance personalized medicine strategies, thereby improving prognosis (15,16).
LUAD and SCLC are different histological types of lung malignancy that have profound implications for patient well-being and quality of life. LUAD, a subtype of NSCLC, is distinguished by its molecular heterogeneity and propensity to progress into the more aggressive SCLC (17), which is characterized by rapid proliferation and early dissemination. This transition is linked to unfavorable prognostic outcomes and limited therapeutic interventions, emphasizing the critical necessity for further investigation into the underlying molecular mechanisms driving this phenomenon (17).
Through the utilization of bulk RNA-seq analysis, MR, and IHC staining techniques, our study elucidated that the decreased expression of MUC1 plays a significant role in the histological evolution from LUAD to NEC. MUC1, a transmembrane glycoprotein commonly overexpressed in various epithelial cancers, including LUAD, has been linked to tumor advancement and metastasis (18). Our findings of an inverse relationship between MUC1 levels and SCLC transformation are particularly intriguing. Furthermore, the MR analysis that identified MUC1 as a pivotal gene linked to the transition from LUAD to SCLC highlights its importance in the molecular pathways involved in this conversion (19). Consistent with our research, Takahashi et al. also found a significant correlation between MUC1 and TKI resistance (20). Their results illustrated that activation of the eIF4A3/MUC1/EGFR signaling pathway may enhance the efficacy of EGFR-TKIs in EGFR-mutant lung cancer. Our bioinformatics analysis highlights the potential of MUC1 as a target for the prediction and treatment of EGFR-mutant LUAD.
Our study conducted an enrichment analysis of DEGs to identify pathways crucial in the transition from LUAD to NEC. The Mucin type O-glycan biosynthesis pathway was found to be involved in post-translational modification of proteins, impacting cell signaling and interaction, aligning with the known role of glycosylation in tumor progression and metastasis (21). Alterations in glycosylation patterns have been linked to immune evasion and drug resistance (22), suggesting a potential mechanism by which LUAD cells may acquire aggressive features of SCLC.
The PI3K/Akt signaling pathway, which is also enriched in this study, is recognized for its role in cell survival, proliferation, and metabolism (23). Dysregulation of this pathway is a hallmark of several cancers, including lung cancer, and is linked to unfavorable prognosis and resistance to treatment (5,24,25). The enrichment of DEGs in this pathway highlights its potential as a therapeutic target in the transition from LUAD to SCLC.
Moreover, the axon guidance pathway, typically linked to neural development, is now being acknowledged for its involvement in cancer progression, specifically in influencing cell migration and invasion. The discovery of DEGs within the EGFR-TKI resistance pathway holds particular significance, posing a formidable obstacle of resistance to targeted therapies in the treatment of lung cancer (26). Furthermore, the enrichment of DEGs in pathways associated with ECM-receptor interaction, focal adhesion, and axon guidance indicates potential changes in cell-extracellular matrix interactions and cell motility, which are critical for cancer cell invasion and metastasis (27). In summary, the dysregulation of these pathways may contribute to the phenotypic changes observed during the aggressive transformation of LUAD to NEC.
Our findings of immune analysis, as revealed by WGCNA and CIBERSORT methods, have elucidated the complex relationship between the tumor microenvironment and the transition from LUAD to SCLC. This observation is significant in light of the growing recognition of the immune microenvironment’s role in cancer development and treatment response (28). Specifically, the expression of MUC1 was found to have a positive association with the presence of macrophages M0 and resting mast cells, while showing a negative correlation with CD8+ T cells and follicular helper T cells (29). This implies that MUC1 may have a significant impact on the immune microenvironment during neuroendocrine transformation, potentially influencing the effectiveness of immune checkpoint therapies. Previous studies have demonstrated a correlation between MUC1, CD8+ T cells, and the immune checkpoint PD-L1 in lung cancer (30,31). Furthermore, the combination of chimeric antigen receptor (CAR)-T therapy targeting MUC1 and anti-PD-1 antibodies has shown promising results in tumor cell eradication (32). Thus, MUC1 may offer valuable insights for advancing lung cancer diagnosis and immunotherapy strategies.
Single-cell RNA sequencing analysis provided further information on the immune contexture, indicating a notable decrease in CM CD8+ T cells and total T cells, alongside a corresponding rise in neuron-like cells EM CD8+ T cells in NEC samples relative to LUAD samples. Prior research has demonstrated that CM CD8+ T cells exhibit enhanced effector cell expansion and more enduring responses in vivo compared to EM CD8+ T cells (33). The present study has identified a reduction in CM CD8+ T cell populations, indicating the suppression of immune response in clinically more aggressive NEC. This underscores the necessity for additional research on therapeutic approaches that could potentially modulate the immune microenvironment to impede or postpone this transition. These results offer valuable insights into the immune microenvironment implicated in the progression from LUAD to SCLC, presenting potential targets for immunotherapeutic intervention and biomarkers for monitoring disease advancement.
Upon reflection of the limitations of this study, it is imperative to acknowledge several factors. Firstly, the sample size utilized in the analysis, particularly for the single-cell RNA-seq data, may not be adequate to fully capture the heterogeneity of the disease, thereby potentially impacting the reliability and applicability of the findings. Secondly, the utilization of multiple datasets introduces the risk of batch effects, which could have influenced the analysis of differential gene expression despite attempts to address these concerns through proper preprocessing techniques. Thirdly, the absence of clinical validation of MUC1 in driving LUAD-to-NEC transformation hinders our capacity to establish correlations between the identified genetic markers and patient outcomes as well as their response to therapy.
Reflecting on the limitations of this study, several factors must be acknowledged. Firstly, the sample size used in the analysis, particularly for the single-cell RNA-seq data, may not be sufficient to capture the full heterogeneity of the disease, potentially affecting the robustness and generalizability of the results. Secondly, the use of multiple datasets introduces the possibility of batch effects, which may have influenced the differential gene expression analysis despite efforts to mitigate such issues through appropriate preprocessing steps. Thirdly, the lack of clinical validation of MUC1 drives LUAD-to-NEC transformation limits our ability to correlate the identified genetic markers with patient outcomes and response to therapy.
Conclusions
In conclusion, this research has identified MUC1 as a key gene linked to the transition from LUAD to NEC and has examined the immune cell infiltration patterns in this context. The validation of MUC1 protein levels in patient tissue samples using IHC, MR, and colocalization analyses provided evidence for genetic impact on neuroendocrine transformation. The analysis of immune infiltration emphasized a noteworthy correlation between MUC1 expression and CD8+ T cells, indicating a potential connection between immune contexture and transformation. These results provide a foundation for future investigations aiming at elucidating the molecular mechanisms and therapeutic strategies involved in the transition from LUAD to NEC.
Acknowledgments
None.
Footnote
Reporting Checklist: The authors have completed the TRIPOD reporting checklist. Available at https://tlcr.amegroups.com/article/view/10.21037/tlcr-24-806/rc
Data Sharing Statement: Available at https://tlcr.amegroups.com/article/view/10.21037/tlcr-24-806/dss
Peer Review File: Available at https://tlcr.amegroups.com/article/view/10.21037/tlcr-24-806/prf
Funding: The research was funded by
Conflicts of Interest: All authors have completed the ICMJE uniform disclosure form (available at https://tlcr.amegroups.com/article/view/10.21037/tlcr-24-806/coif). The authors have no conflicts of interest to declare.
Ethical Statement: The authors are accountable for all aspects of the work in ensuring that questions related to the accuracy or integrity of any part of the work are appropriately investigated and resolved. The study was conducted in accordance with the Declaration of Helsinki (as revised in 2013). This study was approved by the Ethics Committee of Guangdong Provincial People’s Hospital (No. KY-Z-2021-177) and informed consent was taken from all the patients.
Open Access Statement: This is an Open Access article distributed in accordance with the Creative Commons Attribution-NonCommercial-NoDerivs 4.0 International License (CC BY-NC-ND 4.0), which permits the non-commercial replication and distribution of the article with the strict proviso that no changes or edits are made and the original work is properly cited (including links to both the formal publication through the relevant DOI and the license). See: https://creativecommons.org/licenses/by-nc-nd/4.0/.
References
- Sung H, Ferlay J, Siegel RL, et al. Global Cancer Statistics 2020: GLOBOCAN Estimates of Incidence and Mortality Worldwide for 36 Cancers in 185 Countries. CA Cancer J Clin 2021;71:209-49. [Crossref] [PubMed]
- Soria JC, Ohe Y, Vansteenkiste J, et al. Osimertinib in Untreated EGFR-Mutated Advanced Non-Small-Cell Lung Cancer. N Engl J Med 2018;378:113-25. [Crossref] [PubMed]
- Benjamin DJ, Nagasaka M. Freeing the Competition: Will Aumolertinib (AENEAS) Have a Fighting Chance Against Osimertinib (FLAURA)? J Clin Oncol 2023;41:742-4. [Crossref] [PubMed]
- He J, Huang Z, Han L, et al. Mechanisms and management of 3rd-generation EGFR-TKI resistance in advanced non-small cell lung cancer Int J Oncol 2021;59:90. (Review). [Crossref] [PubMed]
- Xu J, Xu L, Wang B, et al. Outcomes in Patients With Lung Adenocarcinoma With Transformation to Small Cell Lung Cancer After EGFR Tyrosine Kinase Inhibitors Resistance: A Systematic Review and Pooled Analysis. Front Oncol 2021;11:766148. [Crossref] [PubMed]
- Quintanal-Villalonga A, Taniguchi H, Zhan YA, et al. Multiomic Analysis of Lung Tumors Defines Pathways Activated in Neuroendocrine Transformation. Cancer Discov 2021;11:3028-47. [Crossref] [PubMed]
- Chen Z, Wang Z, Ma H, et al. Immune cells mediated the causal relationship between the gut microbiota and lung cancer: a Mendelian randomization study. Front Microbiol 2024;15:1390722. [Crossref] [PubMed]
- McLaughlin M, Patin EC, Pedersen M, et al. Inflammatory microenvironment remodelling by tumour cells after radiotherapy. Nat Rev Cancer 2020;20:203-17. [Crossref] [PubMed]
- Jiang X, Wang J, Deng X, et al. Role of the tumor microenvironment in PD-L1/PD-1-mediated tumor immune escape. Mol Cancer 2019;18:10. [Crossref] [PubMed]
- Bischoff P, Trinks A, Obermayer B, et al. Single-cell RNA sequencing reveals distinct tumor microenvironmental patterns in lung adenocarcinoma. Oncogene 2021;40:6748-58. [Crossref] [PubMed]
- Sun Y, Luo J, Chen Y, et al. Combined evaluation of the expression status of CD155 and TIGIT plays an important role in the prognosis of LUAD (lung adenocarcinoma). Int Immunopharmacol 2020;80:106198. [Crossref] [PubMed]
- Jin S, Guerrero-Juarez CF, Zhang L, et al. Inference and analysis of cell-cell communication using CellChat. Nat Commun 2021;12:1088. [Crossref] [PubMed]
- Wang Z, Zhang L, Xu W, et al. The Multi-Omics Analysis of Key Genes Regulating EGFR-TKI Resistance, Immune Infiltration, SCLC Transformation in EGFR-Mutant NSCLC. J Inflamm Res 2022;15:649-67. [Crossref] [PubMed]
- Zhang Y, Chen Q, Huang T, et al. Bioinformatics-based screening of key genes for transformation of tyrosine kinase inhibitor-resistant lung adenocarcinoma to small cell lung cancer. Front Med (Lausanne) 2023;10:1203461. [Crossref] [PubMed]
- Dernbach G, Kazdal D, Ruff L, et al. Dissecting AI-based mutation prediction in lung adenocarcinoma: A comprehensive real-world study. Eur J Cancer 2024;211:114292. [Crossref] [PubMed]
- Xia G, Huang J, Ni J, et al. Transformation of ALK-positive NSCLC to SCLC after alectinib resistance and response to combined atezolizumab: a case report. Transl Lung Cancer Res 2023;12:637-46. [Crossref] [PubMed]
- Li Y, Xie T, Wang S, et al. Mechanism exploration and model construction for small cell transformation in EGFR-mutant lung adenocarcinomas. Signal Transduct Target Ther 2024;9:261. [Crossref] [PubMed]
- Nath S, Mukherjee P. MUC1: a multifaceted oncoprotein with a key role in cancer progression. Trends Mol Med 2014;20:332-42. [Crossref] [PubMed]
- Movahedin M, Brooks TM, Supekar NT, et al. Glycosylation of MUC1 influences the binding of a therapeutic antibody by altering the conformational equilibrium of the antigen. Glycobiology 2017;27:677-87. [Crossref] [PubMed]
- Takahashi S, Noro R, Seike M, et al. Long Non-Coding RNA CRNDE Is Involved in Resistance to EGFR Tyrosine Kinase Inhibitor in EGFR-Mutant Lung Cancer via eIF4A3/MUC1/EGFR Signaling. Int J Mol Sci 2021;22:4005. [Crossref] [PubMed]
- Pinho SS, Reis CA. Glycosylation in cancer: mechanisms and clinical implications. Nat Rev Cancer 2015;15:540-55. [Crossref] [PubMed]
- Mereiter S, Balmaña M, Campos D, et al. Glycosylation in the Era of Cancer-Targeted Therapy: Where Are We Heading? Cancer Cell 2019;36:6-16. [Crossref] [PubMed]
- Su Y, Ma Y, Wang Y, et al. Downregulated CCND3 Is a Key Event Driving Lung Adenocarcinoma Metastasis during Acquired Cisplatin Resistance. Int J Biol Sci 2025;21:708-24. [Crossref] [PubMed]
- Xu H, Wang J, Al-Nusaif M, et al. CCL2 promotes metastasis and epithelial-mesenchymal transition of non-small cell lung cancer via PI3K/Akt/mTOR and autophagy pathways. Cell Prolif 2024;57:e13560. [Crossref] [PubMed]
- Deng H, Chen Y, Wang L, et al. PI3K/mTOR inhibitors promote G6PD autophagic degradation and exacerbate oxidative stress damage to radiosensitize small cell lung cancer. Cell Death Dis 2023;14:652. [Crossref] [PubMed]
- Dai Z, Lin B, Cao Y, et al. Melatonin reverses EGFR-TKI therapeutic resistance by modulating crosstalk between circadian-related gene signature and immune infiltration patterns in patients with COVID-19 and lung adenocarcinoma. Comput Biol Med 2024;180:108937. [Crossref] [PubMed]
- Hamidi H, Ivaska J. Every step of the way: integrins in cancer progression and metastasis. Nat Rev Cancer 2018;18:533-48. [Crossref] [PubMed]
- Daum S, Decristoforo L, Mousa M, et al. Unveiling the immunomodulatory dance: endothelial cells' function and their role in non-small cell lung cancer. Mol Cancer 2025;24:21. [Crossref] [PubMed]
- Svoboda M, Navrátil J, Slabý O. Immunotherapy for the Prevention and Treatment of Breast Cancer. Klin Onkol 2015;28:416-25. [Crossref] [PubMed]
- Pan J, Zeng W, Jia J, et al. A Novel Therapeutic Tumor Vaccine Targeting MUC1 in Combination with PD-L1 Elicits Specific Anti-Tumor Immunity in Mice. Vaccines (Basel) 2022;10:1092. [Crossref] [PubMed]
- Jiang ZB, Huang JM, Xie YJ, et al. Evodiamine suppresses non-small cell lung cancer by elevating CD8(+) T cells and downregulating the MUC1-C/PD-L1 axis. J Exp Clin Cancer Res 2020;39:249. [Crossref] [PubMed]
- Wang A, Lv T, Song Y. Tandem CAR-T cells targeting MUC1 and PSCA combined with anti-PD-1 antibody exhibit potent preclinical activity against non-small cell lung cancer. Cell Immunol 2023;391-392:104760. [Crossref] [PubMed]
- Larsen SE, Voss K, Laing ED, et al. Differential cytokine withdrawal-induced death sensitivity of effector T cells derived from distinct human CD8(+) memory subsets. Cell Death Discov 2017;3:17031. [Crossref] [PubMed]