Using human genetics to understand the epidemiological association between neuroticism and lung cancer
Highlight box
Key findings
• This study presents the first comprehensive investigation into the genetic link between neuroticism and lung cancer (LC), revealing significant genetic correlations and identifying shared loci. Additionally, it uncovers a potential causal relationship between neuroticism and both overall LC and its histological subtypes.
What is known and what is new?
• While previous observational studies suggested a potential link between neuroticism and LC, the underlying genetic mechanisms remained unclear, and no study had fully examined the association at the level of specific histological subtypes.
• This research fills that gap, providing genetic evidence of neuroticism’s association with various LC subtypes and identifying specific pleiotropic loci and genes that may underlie this association.
What is the implication, and what should change now?
• Our findings suggest that individuals with high neuroticism levels may have an elevated risk of developing LC, highlighting the potential benefit of managing neuroticism as part of preventive strategies.
Introduction
Neuroticism, a personality trait that reflects an individual’s emotional instability, is characterized by a heightened tendency to experience anxiety, fear, over-sensitivity, and other negative emotions in response to stress (1). As a core dimension of the Five-Factor Model of personality, neuroticism has been linked to a range of both psychological and physical health conditions, including mental disorders (2), cardiovascular diseases (3,4), chronic pulmonary diseases (5), irritable bowel syndrome, and atopic eczema (6). Furthermore, neuroticism has also been linked to an increased risk of developing malignancies. The mechanisms underlying these associations may involve the prolonged activation of stress responses, which can disrupt immune and endocrine functions (7) and lead to chronic inflammation (8).
Lung cancer (LC) remains the leading incidence and mortality among all malignancies, representing a significant public health concern (9). While numerous studies have established that smoking is a primary risk factor for LC (10,11), epidemiological research has also suggested a potential association between neuroticism and the risk of developing LC. For instance, two prospective cohort studies by Nakaya et al. (12) and Wei et al. (13) found that individuals with high neuroticism scores were at greater risk of developing LC. However, these findings are not supported by an individual-participant meta-analysis of six prospective cohorts (14). These conflicting results may stem from limitations inherent in traditional epidemiological studies, such as potential biases, insufficient adjustment for confounding factors, or reverse causality, meanwhile conducting randomized trials to explore this link would be ethically unfeasible.
Using genetic data for phenotypic correlation analysis offers greater accuracy than observational studies by avoiding reverse causality and minimizing confounding factors. With the increasing availability of high-quality genome-wide association study (GWAS) data (15), researchers can investigate the links between neuroticism and LC and explore underlying mechanisms. In this regard, two studies employing Mendelian randomization (MR) have uncovered a causal relationship between neuroticism and LC by using genetic variants as instrumental variables (IVs) (13,16). However, significant gaps remain: prior studies did not explore the causal link between neuroticism and detailed histological LC subtypes, adjust for limited confounders, and have not addressed the reverse causal effect of LC on neuroticism.
Thus, utilizing the large-scale GWAS data, this study conducted a comprehensive genome-wide cross-trait analysis to characterize the global and local genetic correlations, identify shared genetic loci, and uncover potential causality between neuroticism and LC. Figure 1 has outlined the design of this study. We present this article in accordance with the STREGA reporting checklist (17) (available at https://tlcr.amegroups.com/article/view/10.21037/tlcr-24-950/rc).
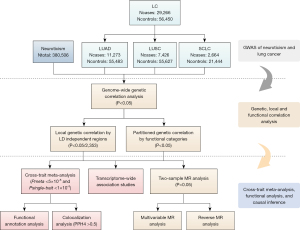
Methods
GWAS data sources
We obtained GWAS summary data for neuroticism from a study focusing on individuals of European ancestry. The phenotype was evaluated by summing up the neuroticism scores based on 12 dichotomous items of the Eysenck Personality Questionnaire Revised Short Form (EPQ-RS) (18), involving 380,060 participants from the UK Biobank, as detailed by Nagel et al. (19).
For LC, extensive GWAS data provided summary-level genetic associations for four distinct LC traits, sourced from a comprehensive analysis conducted by the International Lung Cancer Consortium (ILCCO) (20). This dataset comprised 85,716 participants, including 29,266 overall LC cases. When categorized by histological subtype, the data encompassed 11,273 cases for lung adenocarcinoma (LUAD), 7,426 for lung squamous cell carcinoma (LUSC), and 2,664 for small-cell LC (SCLC). Summary of data source of different traits is shown in Table 1. This study was conducted in accordance with the Declaration of Helsinki (as revised in 2013).
Table 1
Phenotype | Sample size† | Consortium | Population | Year | Data resources |
---|---|---|---|---|---|
Exposure and outcome | |||||
Neuroticism | 380,506 | UK Biobank | European | 2018 | (19) |
LC | 29,266/56,450 | ILCCO | European | 2017 | (20) |
LUAD | 11,273/55,483 | ILCCO | European | 2017 | (20) |
LUSC | 7,426/55,627 | ILCCO | European | 2017 | (20) |
SCLC | 2,664/21,444 | ILCCO | European | 2017 | (20) |
Confounding factors | |||||
BMI | 806,834 | UK Biobank and GIANT | European | 2019 | (21) |
Cigarettes smoked per day | 337,334 | GSCAN | European | 2019 | (22) |
Drinking per week | 941,280 | GSCAN | European | 2019 | (22) |
Physical activity | 90,667 | UK Biobank | European | 2018 | (23) |
Sleep duration | 446,118 | UK Biobank | European | 2019 | (24) |
†, continuous variables as total sample size, and categorical variables are shown as case/control. BMI, body mass index; GIANT, Genetic Investigation of ANthropometric Traits; GWAS, genome-wide association study; GSCAN, GWAS and Sequencing Consortium of Alcohol and Nicotine use; ILCCO, International Lung Cancer Consortium; LC, lung cancer; LUAD, lung adenocarcinoma; LUSC, lung squamous cell carcinoma; SCLC, small-cell lung cancer.
Statistical analyses
Global and local genetic correlation
We assessed the genetic basis shared between neuroticism and LC using linkage disequilibrium (LD) score regression (LDSC) (25), which calculates rg between traits utilizing GWAS summary-level data. It leverages the principle that a variant’s effect size includes effects of all variants in LD with it. LDSC regression of chi-square statistics on LD scores derives genetic correlation, considering sample sizes, overlapping single nucleotide polymorphisms (SNPs), genetic covariance, and phenotypic correlation. We used precomputed LD scores based on well-imputed HapMap3 SNPs from European populations, covering around 1.2 million variants. Subsequently, to address potential population overlap, we implemented LDSC with a constrained intercept.
To further examine local genetic correlations, we used the SUPERGNOVA algorithm (26), which divides the genome into approximately 2,353 LD-independent blocks. This approach allows us to quantify shared local genetic effects in specific regions, revealing local correlations that might be masked in global analyses. To adjusting for multiple comparisons, we applied a Bonferroni correction with a stringent significance threshold at P<0.05/2,353.
Partitioned LDSC
Subsequently, we explored the genetic correlation between neuroticism and LC across multiple functional genomic categories by using partitioned LDSC (27). Our analysis focused on 11 common functional categories: conserved regions, DNase I hypersensitive sites (DHS), DNase I digital genomic footprinting (DGF) region, fetal DHS, histone marks (namely H3K27ac, H3K9ac, H3K4me1, and H3K4me3), promoter regions, transcription factor binding sites (TFBS), and transcribed regions (27,28). For each functional category, we recalculated LD scores for SNPs within that category to estimate the genetic correlation between neuroticism and LC.
Cross-trait meta-analysis
Next, we used Cross-Phenotype Association (CPASSOC) analysis to uncover genetic variants influencing both neuroticism and LC (29). CPASSOC provides two key statistics: SHom, which adjusts for trait correlations in fixed-effect meta-analysis, and SHet, which improves power when genetic effect sizes differ across traits. Given potential genetic heterogeneity, we primarily relied on SHet. We further employed PLINK v1.9 clumping (parameters: -clump-p1 5E−8, -clump-p2 1E-5, -clump-r2 0.2, -clump-kb 500) to obtain shared independent variants (PCPASSOC<5×10−8 and Psingle-trait<1×10−3). Novel shared SNPs referred to those meeting these thresholds but not reaching genome-wide significance in single-trait analyses (1×10−3<Psingle-trait<5×10−8) and were independent (r2<0.2) of those previously reported genome-wide significant SNPs from both single-trait GWAS (30). We then annotated the genes located nearest to the SNPs using the Ensembl Variant Effect Predictor (VEP) (31).
Colocalization analysis
To further validate these pleiotropic loci, colocalization analysis was conducted utilizing the coloc package (32), which employs a Bayesian framework to estimate posterior probabilities for five possible hypotheses: H0 (absence of causal variants), H1/H2 (one unique causal variant for each trait), H3 (two independent causal variants, each linked to one trait), and H4 (a shared causal variant affecting both traits). Our focus was the posterior probability for H4 (PPH4), identifying genetic loci with PPH4 >0.5 located within 500 kb of the lead SNP as shared variants.
Transcriptome-wide association study (TWAS) analysis
To determine tissues most relevant to the shared genes, we employed FUSION software for the TWAS analysis (33). This analysis aimed to discover links between neuroticism and LC by analyzing gene expression across 49 tissue types from the Genotype-Tissue Expression (GTEx) project (version 8). The TWAS methodology integrates GWAS summary statistics with expression quantitative trait loci (eQTL) data, enabling inference of gene expression levels and their associations with complex traits. This approach effectively identifies tissues where gene expression changes are most likely to impact both neuroticism and LC. To mitigate false positives, we utilized the Benjamini-Hochberg correction for multiple comparisons.
MR analysis
Finally, we conducted a bidirectional two-sample MR analysis to investigate potential causal links between neuroticism and LC. For neuroticism, we selected genetic instruments with a significance threshold of P<5×10−8 and clumped them for independent IVs using parameters of r2=0.001 and a window size of 10 Mb. Similarly, we clumped SNPs for LC with the same significance threshold (P<5×10−8) but used r2=0.01 within the same window size. The strength of the selected IVs was assessed using the F-statistic, with values below 10 indicating weak instruments (34).
Our primary method was the inverse-variance weighted (IVW) approach, which combines Wald ratio estimates of each SNP by dividing the SNP-outcome estimate by the SNP-exposure estimate (35). If no heterogeneity was detected, the fixed-effect IVW method was performed. To test the robustness of the findings against the assumption of balanced pleiotropy, we also used MR-Egger regression (36) and weighted median (37). Sensitivity analyses were conducted by excluding palindromic SNPs, removing pleiotropic SNPs associated with confounding traits, performing leave-one-out analysis, and applying the MR-Pleiotropy Residual Sum and Outlier (MR-PRESSO) method (38) to detect and adjust for horizontal pleiotropy. To further address potential confounders, we employed a multivariable MR (MVMR) (39) approach accounting for body mass index (BMI) (21), smoking per day (22), drinking per week (22), physical activity (23), and sleep duration (24) (Table 1). Finally, we conducted a reverse-direction MR analysis to assess the potential reverse causality of genetically predicted LC on neuroticism.
Meta-analysis
To enhance statistical power, we conducted a meta-analysis combining our results with existing cohort studies on neuroticism and LC. A systematic PubMed search identified cohort studies before February 2, 2025, with additional references manually retrieved. Eligible studies were peer-reviewed, included European adults, and reported hazard ratio (HR) estimates with 95% confidence intervals (CIs). Studies with insufficient data or abstracts were excluded.
Data collected included sample size, follow-up, LC cases, and adjusted risk estimates. Study quality was assessed using the Newcastle-Ottawa scale, with all studies scoring ≥7. Pooled risk estimates were calculated, heterogeneity assessed with the Cochran Q test and I2 statistic, and a fixed-effects model was used. Sensitivity analysis tested result robustness by omitting one study at a time. Statistical analysis was performed using R4.4.2.
Results
Global and local genetic correlation
Using both unconstrained and constrained LDSC, the global genetic correlations between neuroticism and LC were analyzed (Figure 2, Table S1). The unconstrained analysis revealed significant correlations for neuroticism with overall LC (rg=0.15, P=2.24×10−5), LUSC (rg=0.21, P=3.39×10−6), and SCLC (rg=0.16, P=2.50×10−3). Under constrained conditions, these genetic correlations showed a slight decrease, along with reduced standard errors, enhancing the statistical robustness for overall LC (rg=0.10, P=6.20×10−7), and different histological subtypes (LUAD: rg=0.05, P=3.28×10−2; LUSC: rg=0.13, P=1.38×10−7; SCLC: rg=0.12, P=1.72×10−5).
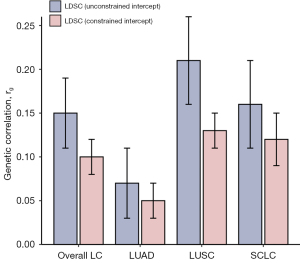
By analyzing local genetic correlations across 2,353 LD-independent regions, significant associations were identified between neuroticism and LC (Figure 3). In the analysis, chr6: 167178790–168548525 showed significant correlations with both overall LC and LUAD, while chr6: 100287245–101862923 was significantly associated with SCLC. Notably, chr6: 167178790–168548525 on 6q27 includes the gene CEP43 (20,40), and chr6: 100287245–101862923 on 6q16.2-q16.3 contains the gene ASCC3 (19,41)—both previously linked to neuroticism and LC.
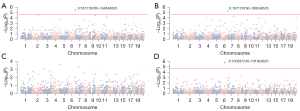
Partitioned LDSC
To explore the genetic overlap between neuroticism and LC across multiple functional genomic elements, we conducted partitioned LDSC analysis (Figure 4, Table S2). Significant genetic correlations were found between neuroticism and overall LC in 10 out of 11 functional categories, with rg values varying from 0.13 in transcribed regions to 0.26 in H3K27ac. For different histological LC subtypes, significant correlations were observed in seven out of 11 categories for LUAD, eight out of 11 for LUSC, and one out of 11 for SCLC. The strongest correlations were observed in TFBS for LUAD (rg=0.14), H3K9ac for LUSC (rg=0.26), and fetal DHS for SCLC (rg=0.13).
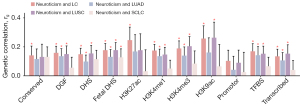
Cross-trait meta-analysis and colocalization analysis
Given the strong genetic association between neuroticism and LC, we conducted a genome-wide meta-analysis using CPASSOC to identify shared significant genetic loci. This analysis revealed 24 independent loci that achieved genome-wide significance (PCPASSOC<5×10−8 and Psingle-trait<1×10−3). Among these, we found nine loci shared between neuroticism and overall LC, four with LUAD, nine with LUSC, and two with SCLC (Table 2). These SNPs are predominantly located in genomic regions 6p21 (harboring SLC44A4, CYP21A2, HSPA1A, HSPA1L, TNXB, and HLA gene), 6p22 (harboring ZSCAN12, KRT18P1, SLC17A4, and ZNF322), 6q27 (harboring RNASET2), 15q21.1 (harboring SEMA6D), and 17q21.31 (harboring WNT3 and CRHR1). Excluding loci previously reported in single-trait GWAS or those in LD (r2≥0.2) with known loci, we identified four novel pleiotropic loci. Among these, two loci were shared between neuroticism and overall LC, and 2 between neuroticism and LUAD. The most significant SNP (rs2769345, PCASSOC=1.07×10−8) mapped to RNASET2, a tumor suppressor involved in multiple cancers (42). Another significant SNP, rs2854275 (PCASSOC=1.96×10−8), located within the HLA gene family, which plays a crucial role in immune regulation and is implicated in LC development and psychiatric disorders (43,44).
Table 2
Index SNP | Position | A1/A2 | Neuroticism | LC | PCPASSOC | Linear closest genes | |||
---|---|---|---|---|---|---|---|---|---|
Beta | P value | Beta | P value | ||||||
Neuroticism and LC | |||||||||
rs6545684 | 2p16.1 | T/G | 0.02 | 2.06×10−8 | −0.05 | 5.40×10−4 | 8.12×10−10 | LINC01122 | |
rs13213152 | 6p22.1 | A/G | 0.02 | 1.71×10−7 | 0.15 | 5.58×10−12 | 8.32×10−12 | ZSCAN12 | |
rs148696809 | 6p22.1 | T/C | 0.02 | 2.21×10−7 | 0.15 | 2.57×10−12 | 3.71×10−12 | KRT18P1 | |
rs2523573 | 6p21.33 | C/G | 0.01 | 1.06×10−5 | 0.10 | 3.18×10−17 | 2.70×10−17 | HLA-B, XXbac-BPG248L24.12 | |
rs501942 | 6p21.33 | T/C | −0.02 | 8.38×10−7 | 0.17 | 8.40×10−19 | 5.94×10−19 | SLC44A4, CYP21A2 | |
rs6456701 | 6p22.2 | T/C | 0.01 | 6.14×10−4 | 0.12 | 1.77×10−8 | 3.64×10−8 | SLC17A4 | |
rs1043618† | 6p21.33 | C/G | −0.01 | 9.88×10−7 | 0.05 | 9.38×10−5 | 3.55×10−8 | HSPA1A, HSPA1L | |
rs2769345† | 6q27 | T/C | 0.01 | 1.72×10−6 | 0.06 | 9.55×10−8 | 1.07×10−8 | RNASET2 | |
rs12903078 | 15q21.1 | A/G | 0.01 | 3.54×10−8 | 0.06 | 3.17×10−7 | 1.39×10−10 | SEMA6D | |
Neuroticism and LUAD | |||||||||
rs1150753† | 6p21.33 | A/G | 0.02 | 3.91×10−7 | 0.10 | 2.69×10−4 | 3.76×10−8 | TNXB | |
rs2854275† | 6p21.32 | A/C | −0.02 | 1.64×10−7 | 0.10 | 8.58×10−4 | 1.96×10−8 | HLA-DQA1, HLA-DQB1 | |
rs113661667 | 17q21.31 | T/C | −0.03 | 3.74×10−31 | −0.07 | 2.77×10−4 | 3.44×10−34 | CRHR1 | |
rs916888 | 17q21.31 | T/C | −0.03 | 1.37×10−22 | −0.07 | 3.50×10−4 | 5.86×10−25 | WNT3 | |
Neuroticism and LUSC | |||||||||
rs13201782 | 6p22.2 | A/T | −0.02 | 4.59×10−6 | 0.20 | 1.62×10−8 | 8.66×10−9 | ZNF322 | |
rs13213986 | 6p22.1 | A/T | −0.02 | 1.72×10−7 | 0.22 | 6.88×10−11 | 1.78×10−11 | ZSCAN12 | |
rs148696809 | 6p22.1 | T/C | 0.02 | 2.21×10−7 | 0.22 | 3.77×10−11 | 8.97×10−12 | KRT18P1 | |
rs2853999 | 6p21.33 | A/T | 0.01 | 1.06×10−5 | 0.25 | 3.51×10−16 | 1.64×10−17 | HLA-B, XXbac-BPG248L24.12 | |
rs36109883 | 6p22.2 | A/G | −0.02 | 1.39×10−5 | 0.25 | 6.16×10−12 | 1.14×10−12 | HIST1H2AC | |
rs4713570 | 6p21.32 | T/C | −0.01 | 1.44×10−5 | 0.14 | 2.04×10−8 | 1.12×10−8 | HLA-DQA1, HLA-DQB1 | |
rs501942 | 6p21.33 | T/C | −0.02 | 8.38×10−7 | 0.26 | 3.06×10−17 | 1.00×10−18 | SLC44A4, CYP21A2 | |
rs707938 | 6p21.33 | A/G | 0.01 | 3.34×10−8 | 0.07 | 3.35×10−4 | 6.56×10−10 | MSH5, SAPCD1, VWA7 | |
rs1327938 | 13q21.2 | T/C | −0.01 | 2.49×10−7 | −0.07 | 3.52×10−4 | 7.63×10−9 | RPP40P2 | |
Neuroticism and SCLC | |||||||||
rs4530683 | 4q28.3 | A/G | −0.01 | 3.43×10−8 | −0.10 | 5.60×10−4 | 1.59×10−8 | SLC7A11-AS1, LINC00616 | |
rs12903078 | 15q21.1 | A/G | 0.01 | 3.54×10−8 | 0.11 | 4.50×10−4 | 1.61×10−8 | SEMA6D |
†, novel SNPs are shared SNPs not driven by a single trait and not in LD (r2<0.2) with index SNPs from single-trait GWAS. CPASSOC, Cross-Phenotype Association; GWAS, genome-wide association study; LC, lung cancer; LD, linkage disequilibrium; LUAD, lung adenocarcinoma; LUSC, lung squamous cell carcinoma; SCLC, small-cell lung cancer; SNP, single nucleotide polymorphism.
For colocalization analysis of shared pleiotropic loci, five out of nine SNPs, three out of four SNPs, six out of nine SNPs, and two out of two SNPs were found to be shared between neuroticism and overall LC, LUAD, LUSC, and SCLC, respectively (Table S3).
TWAS analysis
Results from tissue-specific TWAS revealed gene-level genetic overlap (Tables S4-S7). After multiple corrections, we determined a total of 35 independent transcriptome-wide significant shared genes (including 20 genes shared by neuroticism and overall LC, 13 genes shared by neuroticism and LUAD, five genes shared by neuroticism and LUSC, and four genes shared by neuroticism and SCLC). These gene-level genetic overlap was largely enriched in nervous, digestive, respiratory, cardiovascular, and endocrine system. Of note, three genes were located at pleiotropic loci identified in cross-trait meta-analysis, including RNASET2 at 6q27 (enriched in brain, lung, adipose, whole blood, etc.), SEMA6D at 15q21.1 (enriched in brain), and CRHR1 at 17q21.31 (enriched in brain and kidney cortex).
Bidirectional MR analysis
To investigate the causal link between neuroticism and LC, we conducted bi-directional MR instrumental analysis utilizing 94 neuroticism-associated SNPs (Table S8). The analysis using the IVW method indicated a significant association between neuroticism and overall LC risk [odds ratio (OR) =1.48, P=5.53×10−4]. This association was further supported by the Weight median (OR =1.59, P=3.39×10−4) and MR-PRESSO (OR =1.42, P=1.24×10−3) methods (Figure 5). Although the MR-Egger regression provided estimates that were directionally consistent, the results were not statistically significant (OR =1.19, P=0.81). After excluding palindromic SNPs (OR =1.50, P=6.79×10−4) or pleiotropic (OR =1.41, P=7.02×10−3), the results remained consistent. No significant outliers were identified in the leave-one-out analysis (Figure S1). For specific LC subtypes, a significant causal link was found in LUSC (ORIVW =1.52, P=8.00×10−3), but not in LUAD (ORIVW =1.27, P=0.10) or SCLC (ORIVW =1.69, P=0.06), with these findings confirmed by sensitivity analyses. MVMR was conducted to control for potential confounding factors, resulting in consistent and statistically significant estimates. This indicates that the relationship between neuroticism with overall LC and LUSC remains unaffected by common confounders (Figure S2). In reverse-direction MR analysis using LC-associated SNPs (Table S9), no significant causal effect of LC on neuroticism was found: overall LC (ORIVW =0.99, P=0.33), LUAD (ORIVW =1.00, P=0.77), LUSC (ORIVW =0.98, P=0.18), and SCLC (ORIVW =1.00, P=0.85) (Figure S3).
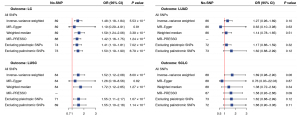
Phenotypic association analysis
Integrating data from four existing cohort studies on the association between neuroticism and LC incidence (12-14,45), the meta-analysis, which included over 490,000 participants, found a significant association (HR =1.06, 95% CI: 1.03–1.10), with no significant heterogeneity (P=0.48, I2=0%) (Figure S4).
Discussion
In this study, we explored the shared genetic basis between neuroticism and LC by investigating genetic correlations, pleiotropic loci, tissue-gene expression, and causal relationships. Our analysis revealed a significant genetic link between neuroticism and LC, with additional insights gained from partitioning the genome into specific regions (including chr6q27 and chr6q16.2–q16.3), and functional areas such as H3K27ac and H3K9ac. The genetic basis was further dissected through two key mechanisms: pleiotropy and causality. Pleiotropic loci identified through CPASSOC highlighted shared genetic influences, while bidirectional MR analysis provided evidence for causality. Collectively, these results provide valuable insights into the complex genetic interplay between neuroticism and LC, indicating potential benefit for assessing LC risk in individuals with high levels of neuroticism.
The global genetic correlation between neuroticism and LC, revealed by LDSC, was confirmed through additional LDSC with a constrained intercept, which improves statistical power by assuming no sample overlap (25). Although the genetic correlation between neuroticism and LUAD was initially non-significant, it became marginally significant when the intercept was constrained to zero. When the genome was divided into 2,353 distinct regions, two specific genomic regions, chr6q27 and chr6q16.2–q16.3, were found to be significantly associated with both neuroticism and LC. The gene ASCC3, located at chr6q16.2–q16.3, has been shown to promote malignant phenotypes in LC and is associated with neuroticism (41,46). Partitioned LDSC further identified strong genetic correlations in functional regions like H3K9ac and H3K27ac, consistent with previous studies on the role of epigenetic modifications in psychiatric disorders and cancer (47,48). These findings suggest that neuroticism and LC share common genetic and epigenetic pathways.
To explore the underlying mechanisms, we performed a cross-trait meta-analysis, identifying 24 shared loci between neuroticism and LC. Many of these loci were previously associated with psychiatric disorders (CRHR1, SLC17A4, SEMA6D) (49-51), inflammatory responses (HSPA1A, RNASET2) (52,53), and cancer progression (WNT3, TNXB) (54,55). Notably, several genes, including RNASET2 and the HLA gene family, showed strong associations, supported by significant colocalization evidence (PPH4 >0.5). Additionally, four novel loci were discovered, two linked to overall LC and two to LUAD, with RNASET2 and the HLA gene family emerging as prominent candidates.
RNASET2 is an RNase T2 enzyme present in humans and represents the only identified extracellular nuclease within its family (56). It has been recognized as a tumor suppressor gene, with reduced expression observed in primary ovarian tumors, melanoma, and non-Hodgkin’s lymphoma (57). Additionally, gene eQTL analysis of 1,425 lung tissue samples has identified RNASET2 as a potential susceptibility locus for LC (20). Beyond its role in cancer, RNASET2 has also been linked to neuroinflammation, a process involved in several mental health disorders (58). These findings highlight RNASET2 as a potential shared mechanism linking neuroticism and LC through its roles in both cancer progression and neuroinflammatory pathways.
The HLA gene family, a highly complex genetic system, plays a pivotal role in immune response and disease susceptibility, affecting various immuno-inflammatory disorders and cancers (59,60). Polymorphisms and expression of HLA molecules are associated with tumor occurrence and progression, as they regulate tumor cell proliferation and suppress antitumor immunity (59). Furthermore, recent studies have highlighted the critical role of HLA-related microglial expression in neurodegenerative disorders and aging (60,61). One potential mechanism by which HLA influences these conditions is through its effect on microglial function. Microglia are key players in the central nervous system, involved in neural circuitry development, brain blood vessel formation, and maintaining the blood-brain barrier architecture (62). Alterations in microglial function, such as microglial senescence and activation, have been linked to a wide range of psychiatric conditions, including psychosis, mania, depression, and anxiety (63). These results suggest the HLA gene family as another potential biological mechanism shared between neuroticism and LC. However, further fundamental research is required to validate these results and fully elucidate the underlying mechanisms.
By combining GTEx tissue expression data with GWAS findings at the gene-tissue level, TWAS analysis uncovered potential common mechanisms between neuroticism and LC. Both CPASSOC and TWAS pinpointed several genes—RNASET2, SEMA6D, CRHR1, and SLC17A4—as relevant across various tissues, particularly those involved in the nervous, respiratory, cardiovascular, and endocrine systems. Additionally, CEP43, located at 6q27, emerged in the local genetic correlation analysis. Other shared genes, such as SERPINA1 and IRF4, were also detected by TWAS, and have been previously associated—either directly or indirectly—with mental health disorders and LC (64-67). Overall, the biological targets that overlap between neuroticism and LC point to potential therapeutic strategies for individuals with comorbid conditions.
Our bidirectional MR analysis identified a significant causal link between neuroticism and LC, particularly in overall LC and LUSC. These results align with findings from large-scale cohort studies (12,13) and recent MR studies (13,16). Our study builds upon these previous MR analyses in several key ways. Our study extends these previous analyses by exploring causal effects across specific LC histological subtypes. Sensitivity analyses confirmed the robustness of our results, and reverse-direction MR analysis revealed no significant causal effect of LC on neuroticism. These findings suggest that neuroticism may play a causal role in LC risk, emphasizing the need for targeted preventive strategies for individuals with high neuroticism. This underscores the importance of assessing LC risk and developing preventive strategies for individuals with high levels of neuroticism.
Several limitations of this study should be acknowledged. The genetic data that we used was derived from GWAS involving participants of European background, which may limit the generalizability of the results to other populations. Additionally, the relatively small sample size for LC histological subtypes may have restricted the ability to detect subtype-specific causal relationships. Self-reported neuroticism data at baseline could have introduced misclassification, although this is likely non-differential and would underestimate the true association. Furthermore, while our study focused on LC, neuroticism has been linked to other health conditions (e.g., chronic bronchitis, asthma), and future studies should explore these associations further. Lastly, although we identified potential genetic mechanisms and causal pathways, further clinical validation is necessary to confirm their practical applications in cancer prevention.
Conclusions
In conclusion, this study provides robust evidence for a shared genetic basis between neuroticism and LC, supported by pleiotropic loci and causal associations. Our findings suggest that neuroticism may contribute to LC risk, highlighting the importance of considering personality traits in LC risk assessment and prevention. This study advances the understanding of the genetic architecture underlying the relationship between neuroticism and LC and suggests potential avenues for future research and cancer prevention.
Acknowledgments
None.
Footnote
Reporting Checklist: The authors have completed the STREGA reporting checklist. Available at https://tlcr.amegroups.com/article/view/10.21037/tlcr-24-950/rc
Peer Review File: Available at https://tlcr.amegroups.com/article/view/10.21037/tlcr-24-950/prf
Funding: This work was supported by the 1·3·5 Project for Disciplines of Excellence, West China Hospital, Sichuan University (to L.L.) (No. ZYJC21002), the National Natural Science Foundation of China (to J.Z.) (No. 82102968), and the Science and Technology Support Program of Sichuan Province (to S.W.) (No. 2023YFS0126).
Conflicts of Interest: All authors have completed the ICMJE uniform disclosure form (available at https://tlcr.amegroups.com/article/view/10.21037/tlcr-24-950/coif). The authors have no conflicts of interest to declare.
Ethical Statement: The authors are accountable for all aspects of the work in ensuring that questions related to the accuracy or integrity of any part of the work are appropriately investigated and resolved. The ethical approval for each summary-level data can be found from the corresponding studies. This study was conducted in accordance with the Declaration of Helsinki (as revised in 2013).
Open Access Statement: This is an Open Access article distributed in accordance with the Creative Commons Attribution-NonCommercial-NoDerivs 4.0 International License (CC BY-NC-ND 4.0), which permits the non-commercial replication and distribution of the article with the strict proviso that no changes or edits are made and the original work is properly cited (including links to both the formal publication through the relevant DOI and the license). See: https://creativecommons.org/licenses/by-nc-nd/4.0/.
References
- Lahey BB. Public health significance of neuroticism. Am Psychol 2009;64:241-56. [Crossref] [PubMed]
- Ormel J, Jeronimus BF, Kotov R, et al. Neuroticism and common mental disorders: meaning and utility of a complex relationship. Clin Psychol Rev 2013;33:686-97. [Crossref] [PubMed]
- Zhang F, Cao H, Baranova A. Genetic variation mediating neuroticism's influence on cardiovascular diseases. J Psychopathol Clin Sci 2022;131:278-86. [Crossref] [PubMed]
- Kang W, Malvaso A. Personality Traits Predict Self-Rated Health (SRH) in Coronary Heart Disease (CHD) Patients and Healthy Controls. Healthcare (Basel) 2023;11:1645. [Crossref] [PubMed]
- Kang W, Malvaso A. Can the Big Five personality traits predict ever chance and 7-year risk of clinically diagnosed chronic bronchitis in middle-aged and older adults? J Psychosom Res 2023;172:111423. [Crossref] [PubMed]
- Widiger TA, Oltmanns JR. Neuroticism is a fundamental domain of personality with enormous public health implications. World Psychiatry 2017;16:144-5. [Crossref] [PubMed]
- Antoni MH, Lutgendorf S. Psychosocial factors and disease progression in cancer. Curr Dir Psychol Sci 2007;16:42-6. [Crossref]
- Hänsel A, Hong S, Cámara RJ, et al. Inflammation as a psychophysiological biomarker in chronic psychosocial stress. Neurosci Biobehav Rev 2010;35:115-21. [Crossref] [PubMed]
- Siegel RL, Miller KD, Jemal A. Cancer statistics, 2019. CA Cancer J Clin 2019;69:7-34. [Crossref] [PubMed]
- He H, He MM, Wang H, et al. In Utero and Childhood/Adolescence Exposure to Tobacco Smoke, Genetic Risk, and Lung Cancer Incidence and Mortality in Adulthood. Am J Respir Crit Care Med 2023;207:173-82. [Crossref] [PubMed]
- Gredner T, Mons U, Niedermaier T, et al. Impact of tobacco control policies implementation on future lung cancer incidence in Europe: An international, population-based modeling study. Lancet Reg Health Eur 2021;4:100074. [Crossref] [PubMed]
- Nakaya N, Bidstrup PE, Saito-Nakaya K, et al. Personality traits and cancer risk and survival based on Finnish and Swedish registry data. Am J Epidemiol 2010;172:377-85. [Crossref] [PubMed]
- Wei X, Jiang X, Zhang X, et al. Association Between Neuroticism and Risk of Lung Cancer: Results From Observational and Mendelian Randomization Analyses. Front Oncol 2022;12:836159. [Crossref] [PubMed]
- Jokela M, Batty GD, Hintsa T, et al. Is personality associated with cancer incidence and mortality? An individual-participant meta-analysis of 2156 incident cancer cases among 42,843 men and women. Br J Cancer 2014;110:1820-4. [Crossref] [PubMed]
- Sudlow C, Gallacher J, Allen N, et al. UK biobank: an open access resource for identifying the causes of a wide range of complex diseases of middle and old age. PLoS Med 2015;12:e1001779. [Crossref] [PubMed]
- Shi J, Wen W, Long J, et al. Genetic correlation and causal associations between psychiatric disorders and lung cancer risk. J Affect Disord 2024;356:647-56. [Crossref] [PubMed]
- Little J, Higgins JP, Ioannidis JP, et al. STrengthening the REporting of Genetic Association studies (STREGA): an extension of the STROBE Statement. Ann Intern Med 2009;150:206-15. [Crossref] [PubMed]
- Eysenck SBG, Eysenck HJ, Barrett P. A revised version of the psychoticism scale. Pers Individ Dif 1985;6:21-9. [Crossref]
- Nagel M, Watanabe K, Stringer S, et al. Item-level analyses reveal genetic heterogeneity in neuroticism. Nat Commun 2018;9:905. [Crossref] [PubMed]
- McKay JD, Hung RJ, Han Y, et al. Large-scale association analysis identifies new lung cancer susceptibility loci and heterogeneity in genetic susceptibility across histological subtypes. Nat Genet 2017;49:1126-32. [Crossref] [PubMed]
- Pulit SL, Stoneman C, Morris AP, et al. Meta-analysis of genome-wide association studies for body fat distribution in 694 649 individuals of European ancestry. Hum Mol Genet 2019;28:166-74. [Crossref] [PubMed]
- Liu M, Jiang Y, Wedow R, et al. Association studies of up to 1.2 million individuals yield new insights into the genetic etiology of tobacco and alcohol use. Nat Genet 2019;51:237-44. [Crossref] [PubMed]
- Klimentidis YC, Raichlen DA, Bea J, et al. Genome-wide association study of habitual physical activity in over 377,000 UK Biobank participants identifies multiple variants including CADM2 and APOE. Int J Obes (Lond) 2018;42:1161-76. [Crossref] [PubMed]
- Dashti HS, Jones SE, Wood AR, et al. Genome-wide association study identifies genetic loci for self-reported habitual sleep duration supported by accelerometer-derived estimates. Nat Commun 2019;10:1100. [Crossref] [PubMed]
- Bulik-Sullivan B, Finucane HK, Anttila V, et al. An atlas of genetic correlations across human diseases and traits. Nat Genet 2015;47:1236-41. [Crossref] [PubMed]
- Zhang Y, Lu Q, Ye Y, et al. SUPERGNOVA: local genetic correlation analysis reveals heterogeneous etiologic sharing of complex traits. Genome Biol 2021;22:262. [Crossref] [PubMed]
- Finucane HK, Bulik-Sullivan B, Gusev A, et al. Partitioning heritability by functional annotation using genome-wide association summary statistics. Nat Genet 2015;47:1228-35. [Crossref] [PubMed]
- Bernstein BE, Stamatoyannopoulos JA, Costello JFThe NIH Roadmap Epigenomics Mapping Consortium, et al. Nat Biotechnol 2010;28:1045-8. [Crossref] [PubMed]
- Li X, Zhu X. Cross-Phenotype Association Analysis Using Summary Statistics from GWAS. Methods Mol Biol 2017;1666:455-67. [Crossref] [PubMed]
- Qu Y, Xiao C, Wu X, et al. Genetic Correlation, Shared Loci, and Causal Association Between Sex Hormone-Binding Globulin and Bone Mineral Density: Insights From a Large-Scale Genomewide Cross-Trait Analysis. J Bone Miner Res 2023;38:1635-44. [Crossref] [PubMed]
- McLaren W, Gil L, Hunt SE, et al. The Ensembl Variant Effect Predictor. Genome Biol 2016;17:122. [Crossref] [PubMed]
- Giambartolomei C, Vukcevic D, Schadt EE, et al. Bayesian test for colocalisation between pairs of genetic association studies using summary statistics. PLoS Genet 2014;10:e1004383. [Crossref] [PubMed]
- Gusev A, Ko A, Shi H, et al. Integrative approaches for large-scale transcriptome-wide association studies. Nat Genet 2016;48:245-52. [Crossref] [PubMed]
- Burgess S, Thompson SG. Avoiding bias from weak instruments in Mendelian randomization studies. Int J Epidemiol 2011;40:755-64. [Crossref] [PubMed]
- Burgess S, Scott RA, Timpson NJ, et al. Using published data in Mendelian randomization: a blueprint for efficient identification of causal risk factors. Eur J Epidemiol 2015;30:543-52. [Crossref] [PubMed]
- Bowden J, Davey Smith G, Burgess S. Mendelian randomization with invalid instruments: effect estimation and bias detection through Egger regression. Int J Epidemiol 2015;44:512-25. [Crossref] [PubMed]
- Bowden J, Davey Smith G, Haycock PC, et al. Consistent Estimation in Mendelian Randomization with Some Invalid Instruments Using a Weighted Median Estimator. Genet Epidemiol 2016;40:304-14. [Crossref] [PubMed]
- Verbanck M, Chen CY, Neale B, et al. Detection of widespread horizontal pleiotropy in causal relationships inferred from Mendelian randomization between complex traits and diseases. Nat Genet 2018;50:693-8. [Crossref] [PubMed]
- Burgess S, Thompson SG. Multivariable Mendelian randomization: the use of pleiotropic genetic variants to estimate causal effects. Am J Epidemiol 2015;181:251-60. [Crossref] [PubMed]
- Kim Y, Saunders GRB, Giannelis A, et al. Genetic and neural bases of the neuroticism general factor. Biol Psychol 2023;184:108692. [Crossref] [PubMed]
- Ao YQ, Gao J, Jin C, et al. ASCC3 promotes the immunosuppression and progression of non-small cell lung cancer by impairing the type I interferon response via CAND1-mediated ubiquitination inhibition of STAT3. J Immunother Cancer 2023;11:e007766. [Crossref] [PubMed]
- Bruno A, Noonan DM, Valli R, et al. Human RNASET2: A Highly Pleiotropic and Evolutionary Conserved Tumor Suppressor Gene Involved in the Control of Ovarian Cancer Pathogenesis. Int J Mol Sci 2022;23:9074. [Crossref] [PubMed]
- Nudel R, Benros ME, Krebs MD, et al. Immunity and mental illness: findings from a Danish population-based immunogenetic study of seven psychiatric and neurodevelopmental disorders. Eur J Hum Genet 2019;27:1445-55. [Crossref] [PubMed]
- Hematian Larki M, Ashouri E, Barani S, et al. KIR-HLA gene diversities and susceptibility to lung cancer. Sci Rep 2022;12:17237. [Crossref] [PubMed]
- Nakaya N, Tsubono Y, Hosokawa T, et al. Personality and the risk of cancer. J Natl Cancer Inst 2003;95:799-805. [Crossref] [PubMed]
- Hindley G, Shadrin AA, van der Meer D, et al. Multivariate genetic analysis of personality and cognitive traits reveals abundant pleiotropy. Nat Hum Behav 2023;7:1584-600. [Crossref] [PubMed]
- Sengupta D, Zeng L, Li Y, et al. NSD2 dimethylation at H3K36 promotes lung adenocarcinoma pathogenesis. Mol Cell 2021;81:4481-4492.e9. [Crossref] [PubMed]
- Tsankova N, Renthal W, Kumar A, et al. Epigenetic regulation in psychiatric disorders. Nat Rev Neurosci 2007;8:355-67. [Crossref] [PubMed]
- Ramoz N, Hoertel N, Nobile B, et al. Corticotropin releasing hormone receptor CRHR1 gene is associated with tianeptine antidepressant response in a large sample of outpatients from real-life settings. Transl Psychiatry 2020;10:378. [Crossref] [PubMed]
- Ikeda M, Takahashi A, Kamatani Y, et al. Genome-Wide Association Study Detected Novel Susceptibility Genes for Schizophrenia and Shared Trans-Populations/Diseases Genetic Effect. Schizophr Bull 2019;45:824-34. [Crossref] [PubMed]
- Nakanishi Y, Izumi M, Matsushita H, et al. Semaphorin 6D tunes amygdalar circuits for emotional, metabolic, and inflammatory outputs. Neuron 2024;112:2955-2972.e9. [Crossref] [PubMed]
- Ogbodo E, Michelangeli F, Williams JHH. Exogenous heat shock proteins HSPA1A and HSPB1 regulate TNF-α, IL-1β and IL-10 secretion from monocytic cells. FEBS Open Bio 2023;13:1922-40. [Crossref] [PubMed]
- Wu L, Xu Y, Zhao H, et al. RNase T2 in Inflammation and Cancer: Immunological and Biological Views. Front Immunol 2020;11:1554. [Crossref] [PubMed]
- Yu F, Yu C, Li F, et al. Wnt/β-catenin signaling in cancers and targeted therapies. Signal Transduct Target Ther 2021;6:307. [Crossref] [PubMed]
- Liot S, Aubert A, Hervieu V, et al. Loss of Tenascin-X expression during tumor progression: A new pan-cancer marker. Matrix Biol Plus 2020;6-7:100021. [Crossref] [PubMed]
- Luhtala N, Parker R. T2 Family ribonucleases: ancient enzymes with diverse roles. Trends Biochem Sci 2010;35:253-9. [Crossref] [PubMed]
- Ji M, Zhao Z, Li Y, et al. FBXO6-mediated RNASET2 ubiquitination and degradation governs the development of ovarian cancer. Cell Death Dis 2021;12:317. [Crossref] [PubMed]
- Sinkevicius KW, Morrison TR, Kulkarni P, et al. RNaseT2 knockout rats exhibit hippocampal neuropathology and deficits in memory. Dis Model Mech 2018;11:dmm032631. [Crossref] [PubMed]
- Liu DH, Mou FF, An M, et al. Human leukocyte antigen and tumor immunotherapy Int J Oncol 2023;62:68. (Review). [Crossref] [PubMed]
- Tamouza R, Krishnamoorthy R, Leboyer M. Understanding the genetic contribution of the human leukocyte antigen system to common major psychiatric disorders in a world pandemic context. Brain Behav Immun 2021;91:731-9. [Crossref] [PubMed]
- Malvaso A, Gatti A, Negro G, et al. Microglial Senescence and Activation in Healthy Aging and Alzheimer's Disease: Systematic Review and Neuropathological Scoring. Cells 2023;12:2824. [Crossref] [PubMed]
- Zhu H, Guan A, Liu J, et al. Noteworthy perspectives on microglia in neuropsychiatric disorders. J Neuroinflammation 2023;20:223. [Crossref] [PubMed]
- Mondelli V, Vernon AC, Turkheimer F, et al. Brain microglia in psychiatric disorders. Lancet Psychiatry 2017;4:563-72. [Crossref] [PubMed]
- Zhang LY, Sun XW, Ding YJ, et al. SERPINA1 Methylation Levels are Associated with Lung Cancer Development in Male Patients with Chronic Obstructive Pulmonary Disease. Int J Chron Obstruct Pulmon Dis 2022;17:2117-25. [Crossref] [PubMed]
- Grove J, Ripke S, Als TD, et al. Identification of common genetic risk variants for autism spectrum disorder. Nat Genet 2019;51:431-44. [Crossref] [PubMed]
- Qian Y, Du Z, Xing Y, et al. Interferon regulatory factor 4 (IRF4) is overexpressed in human non‑small cell lung cancer (NSCLC) and activates the Notch signaling pathway. Mol Med Rep 2017;16:6034-40. [Crossref] [PubMed]
- Levinson DF, Shi J, Wang K, et al. Genome-wide association study of multiplex schizophrenia pedigrees. Am J Psychiatry 2012;169:963-73. [Crossref] [PubMed]