Integrating radiomics and deep learning for enhanced prediction of high-grade patterns in stage IA lung adenocarcinoma
Highlight box
Key findings
• The fusion model combing radiomics and deep learning can identify the presence of high-grade patterns (HGPs) in invasive lung adenocarcinoma from preoperative computed tomography (CT) images.
What is known and what is new?
• Previous studies have demonstrated the potential of radiomics and deep learning models in distinguishing between tumors with and without specific high-risk subsets.
• This study introduces a novel fusion model combining radiomics features and deep learning features, which significantly improves the predictive accuracy for diagnosing the presence of HGPs in lung adenocarcinoma using preoperative CT images.
What is the implication, and what should change now?
• The fusion model could potentially guide more personalized treatment plans and improve patient management.
• Clinicians should consider incorporating this fusion model into routine clinical practice for enhanced preoperative decision-making. Further validation in larger, diverse cohorts is needed to confirm its broader applicability and establish standardized guidelines for integrating this model into clinical workflows.
Introduction
Current epidemiological data show that lung cancer remains the leading cause of human mortality worldwide (1). Adenocarcinoma, the most common subtype of lung cancer, is characterized by significant heterogeneity in its histological features. In 2011, the International Association for the Study of Lung Cancer (IASLC), American Thoracic Society (ATS), and European Respiratory Society (ERS) categorized invasive pulmonary non-mucinous adenocarcinomas into five pathological subtypes: lepidic, acinar, papillary, micropapillary, and solid. These subtypes were histologically graded according to their predominant features and recorded at 5% of the incremental semi-quantitative recording (2). Micropapillary and solid subtypes were defined as high-grade patterns (HGPs) due to their poor prognosis (3). Subsequent studies later identified complex glandular patterns (cribriform and fused glands), which was associated with high mitotic grade, tumor necrosis, and lymph node metastasis. This growth pattern had a similar prognosis to the micropapillary and solid subtypes and was an independent prognostic factor for the poor recurrence-free survival and overall survival (4-7). In 2020, IASLC introduced a novel grading system that included complex glandular patterns in the HGP category and defined tumors with ≥20% HGPs (micropapillary, solid, complex gland) as poorly differentiated cancers (8). The new grading system was adopted by the World Health Organization (WHO) Classification of Thoracic tumors (5th edition) in 2021 (9), and its more favorable prognostic stratification value has been validated in several large cohorts (10-12).
Several recent studies addressing surgical options for smaller diameter peripheral lung cancers have favored segmentectomy over lobectomy (13,14). However, in patients undergoing limited resection, a micropapillary component of 5% or greater was correlated with recurrence (15). Even a small percentage of micropapillary, solid, and complex glandular patterns can adversely affect recurrence and prognosis (16-19). Therefore, for lung adenocarcinomas with HGPs, undergoing more extensive resection and systematic mediastinal lymph node dissection may be necessary. In addition, a grading system based on HGPs can also be effective in stratifying patients who need to receive adjuvant chemotherapy (10,20). Therefore, it is essential to accurately identify the presence of HGPs preoperatively. Currently, preoperative biopsies and frozen sections do not provide a complete response to the histological differentiation of lung adenocarcinoma due to the inadequacy of sampling and poor quality of frozen sections (21). The detection of HGPs mainly relies on paraffin-embedded histopathology after complete tumor resection (9), which, however, is invasive and lagging. Therefore, it is urgent to develop a new technique that can effectively identify the presence of HGPs before treatment to guide clinicians through the entire diagnostic and treatment process and predict recurrence survival of patients.
Radiomics is a technique that uses machine learning to integrate features for prediction after extracting high-throughput features from medical images. It has been widely used in the field of lung cancer for screening, staging, recurrence prognosis, and prediction of treatment response (22,23). While deep learning is different from traditional radiomics, it is a neural network with multiple hidden layers that can utilize complex multi-layer neural network architectures to create more intrinsically effective deep features. It has recently been shown that fusion models combining radiomics features and deep learning features can improve preoperative diagnosis of high-risk subtypes of lung cancers (24). So, radiomics features and deep learning features may be complementary at some levels. Although some studies predicted certain high-risk subtypes by radiomics or deep learning (24-27), there are currently no studies predicting the presence of complete HGPs (micropapillary, solid, complex gland) according to the novel IASLC classification. If the identification works, the clinician may favor a more extensive surgery or more aggressive adjuvant treatment for the tumor with HPGs to avoid the recurrence.
In this study, we aimed to predict the presence of HGPs in invasive pulmonary non-mucinous adenocarcinoma based on preoperative computed tomography (CT) images for better treatment development and monitoring strategies. In the meantime, we explored the integration of both radiomics features and deep learning features to enhance prediction performance. We present this article in accordance with the TRIPOD reporting checklist (available at https://tlcr.amegroups.com/article/view/10.21037/tlcr-24-995/rc).
Methods
Inclusion and exclusion criteria
The study subjects were patients with lung adenocarcinoma who were admitted to Taizhou Hospital of Zhejiang Province from January 2023 to January 2024 for surgical treatment. The inclusion criteria were as follows: (I) patients with complete surgical resection and pathologically confirmed invasive adenocarcinoma (IAC) cancer; (II) Pathology reports containing complete percentages of each HGP; (III) chest CT scans performed 2 weeks prior to surgery, and digital image and communication in medicine (DICOM) files of the CT images were available; and (IV) patients with clinical staging of stage IA. Initially, 487 patients were included. The exclusion criteria were as follows: (I) pathologically confirmed variant adenocarcinoma, such as invasive mucinous adenocarcinoma, colloid carcinoma, etc., or mixed lung carcinoma containing other lung cancer types (n=24); (II) pathologically confirmed to contain multiple primary invasive lung adenocarcinoma (in order to avoid the clustering effect) (n=9); (III) poor image quality due to severe respiratory artefacts, etc. (n=19); (IV) CT image layer thickness >2.5 mm (n=27); and (V) preoperative history of radiotherapy and chemotherapy (n=5). Finally, 403 patients were enrolled in our study.
The study was conducted in accordance with the Declaration of Helsinki (as revised in 2013). This retrospective study was approved by the Ethics Review Committee of Taizhou Hospital, Zhejiang Province (No. KL20240438), and the requirement for informed consent has been waived due to the retrospective nature of the study.
Study cohort
The 403 patients were split into three cohorts using the inclusion and exclusion criteria. Patients admitted from January 2023 to October 2023 were defined as the training and internal validation cohort for improvement and evaluation of our models. The training and validation cohorts were randomly split in a 7:3 ratio. Patients admitted from November 2023 to January 2024 were defined as the test cohort. It should be mentioned that throughout the experiment, we transferred all patients with CT layer thickness of 2.5 mm (n=32) into the training and validation cohort to the test cohort for testing the generalization performance of the model under high automation. The specific study process is shown by the flowchart in Figure 1.
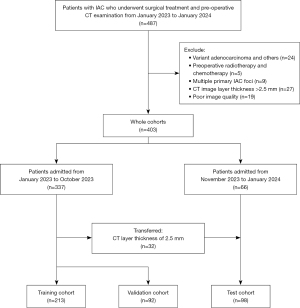
CT data acquisition
Chest CT images were obtained from two different scanners: the Discover CT750 HD (General Electric, Boston, MA, USA) and the UCT 530 (Shanghai United Imaging Medical Co., Ltd., Shanghai, China). All imaging data were taken with a layer thickness ≤2.5 mm. All CT scans were downloaded from the image archive and communication system as DICOM images. Patients’ personal information, including name and hospital number, were removed from the CT images.
Clinical and radiological characteristics
The electronic medical records of the patients were reviewed to collect clinical characteristics relevant to the study including age, gender, smoking history, tumor stage, site, and mode of surgery. Tumor staging was performed according to the IASLC tumor-nodes-metastasis (TNM) staging system version 8 (28). In addition, two radiologists with more than 10 years of experience and expertise related to CT diagnosis of lung adenocarcinoma, recorded each of the nine radiological features without the clinical and pathological information: max diameter, lobulation sign, spiculation sign, vacuole sign, air bronchogram sign, vascular convergence sign, pleural retraction sign, density and consolidation/tumor ratio (CTR). The controversial parts were discussed until a consensus was reached. The interpretation of CT radiological features is described in Table S1.
Histopathology evaluation
Pathological specimens were routinely fixed in 10% formalin and embedded in paraffin. Tissue sections of the maximum section of the tumor, 4 µm thick, were stained with hematoxylin and eosin (HE). Two pathologists with more than 10 years of experience in diagnostic lung pathology, without knowledge of clinical and radiological information, made pathological diagnoses of all 403 patients. Consensus on controversial diagnoses was reached through discussion. Diagnoses were described with reference to HGP in the novel IASLC grading system (8) and the percentage of HGPs was calculated in 5% increments. All patients were divided into two groups: the HGP+ group (percentage of HGP ≥5%) and the HGP− group (percentage of HGP <5%). The novel IASLC grading system of invasive lung adenocarcinoma is described in Table S2.
Region of interest (ROI) segmentation
ROIs within the training and validation cohorts were outlined by one radiologist with more than 10 years of experience, without the pathological and clinical information. Tumor contours were manually outlined in the horizontal cross-section of the CT images by ITK-SNAP software (version 3.8.0; http://www.itksnap.org/).
However, the use of manual delineation for such tasks significantly restricts its applicability in clinical settings due to its time-consuming nature. The study shows that the deep learning-based auto-segmentations has achieved acceptable accuracy in multi-site tumor analysis (29). So, we leveraged the annotated data from the training cohort to train our segmentation algorithm, subsequently applying it to delineate the ROI areas within the test cohort’s data. Detailed training methodologies are delineated in Appendix 1.
Feature extraction and selection
From the delineated ROI regions, we extracted a total of 256 deep learning features and 1,836 handcrafted features. The deep learning features were derived through transfer learning algorithms, specifically from the penultimate layer (Avgpool) of ResNet101, capturing a 2,048-dimensional feature space. To mitigate the risk of overfitting, we applied principal component analysis (PCA) to reduce this dimensionality to 256. The handcrafted features encompassed shape, first-order statistics, global texture, and local texture metrics. The training process for the deep learning model and the feature extraction methodology are further elaborated in Appendices 2,3, respectively.
To address the model’s complexity and minimize the overfitting risk inherent in high-dimensional data, we initially standardized all features using z-score normalization. This was followed by a multifaceted feature screening process. The process began with t-test-based P value calculations, selecting features with P values less than 0.05 for further analysis. We then assessed feature redundancy through Pearson correlation coefficient, opting to retain a single feature from pairs with correlations above 0.9, thus implementing a greedy recursive elimination strategy to diminish redundancy. Further refinement of our feature set was achieved using the least absolute shrinkage and selection operator (LASSO) regression, which effectively minimized the impact of less relevant features. The optimal regularization parameter λ was identified via 10-fold cross-validation.
Development and assessment of models
For the adjustment of model hyperparameters, we employed 5-fold cross-validation on the training dataset, utilizing the Gridsearch algorithm for the optimization of hyperparameters. The parameters showing the best median performance were chosen for the final model’s training. After the LASSO-based feature selection, the deep learning radiomics (DLR) model was developed by both linear [logistic regression (LR) and support vector machine (SVM)] and tree-based models (RandomForest, ExtraTrees, XGBoost), in addition to a deep learning-based multi-layer perceptron (MLP) model. The deep learning model was represented by the output probabilities generated by the CNN. For the radiomics, we exclusively utilized handcrafted features for machine learning algorithm modeling, following a procedure analogous to the DLR model. In addition, the diagnostic accuracy of our models was gauged by receiver operating characteristic (ROC) curves. Calibration performance was evaluated through calibration curves and Hosmer-Lemeshow (HL) tests to assess the models’ calibration quality. Decision curve analysis (DCA) was employed to determine the clinical utility of our predictive models.
Statistical analysis
Our analyses were performed using Python version 3.7.12 and statsmodels version 0.13.2. Machine learning model development was facilitated by the scikit-learn version 1.0.2 interface. Deep learning training was conducted using an NVIDIA 4090 GPU, within the MONAI 0.8.1 and PyTorch 1.8.1 frameworks.
Results
Clinical and conventional radiographic characteristics
A total of 305 patients were randomly assigned to the training and validation sets in a 7:3 ratio. The training set consisted of 213 patients (129 in HGP− group, 84 in HGP+ group). The validation set totaled 92 patients (62 in HGP− group, 30 in HGP+ group). The test set comprised 98 patients (61 in HGP− group, 37 in HGP+ group). The clinical baseline characteristics of the 403 patients in the training, validation, and test sets are shown in Table 1. All clinical baseline characteristics did not differ statistically between the data sets.
Table 1
Clinical features | Training cohort (n=213) | Validation cohort (n=92) | Test cohort (n=98) | P value | ||||||||
---|---|---|---|---|---|---|---|---|---|---|---|---|
HGP− (n=129) | HGP+ (n=84) | P value | HGP− (n=62) | HGP+ (n=30) | P value | HGP− (n=61) | HGP+ (n=37) | P value | ||||
Age (years) | 61.19±10.35 | 63.95±9.50 | 0.11 | 64.08±8.90 | 62.27±10.46 | 0.39 | 63.428±9.18 | 63.95±9.90 | 0.81 | 0.41 | ||
Gender | 0.07 | 0.007 | <0.001 | 0.93 | ||||||||
Female | 82 (63.57) | 43 (51.19) | 43 (69.35) | 12 (40.00) | 44 (72.13) | 12 (32.43) | ||||||
Male | 47 (36.43) | 41 (48.81) | 19 (30.65) | 18 (60.00) | 17 (27.87) | 25 (67.57) | ||||||
Smoking | 0.17 | 0.02 | <0.001 | 0.97 | ||||||||
Never | 108 (83.72) | 64 (76.19) | 54 (87.10) | 20 (66.67) | 59 (96.72) | 19 (51.35) | ||||||
Ever | 21 (16.28) | 20 (23.81) | 8 (12.90) | 10 (33.33) | 2 (3.28) | 18 (48.65) | ||||||
Operation | 0.02 | 0.03 | 0.002 | 0.77 | ||||||||
Sublobectomy | 72 (55.81) | 33 (39.29) | 34 (54.84) | 9 (30.00) | 39 (63.93) | 12 (32.43) | ||||||
Lobectomy | 57 (44.19) | 51 (60.71) | 28 (45.16) | 21 (70.00) | 22 (36.07) | 25 (67.57) | ||||||
pT stage | 0.001 | 0.005 | <0.001 | 0.17 | ||||||||
1a | 26 (20.16) | 9 (10.71) | 11 (17.74) | 4 (13.33) | 8 (13.11) | 1 (2.70) | ||||||
1b | 84 (65.12) | 48 (57.14) | 40 (64.52) | 17 (56.67) | 41 (67.21) | 13 (35.14) | ||||||
1c | 15 (11.63) | 12 (14.29) | 10 (16.13) | 2 (6.67) | 10 (16.39) | 10 (27.03) | ||||||
2a | 4 (3.10) | 15 (17.86) | 1 (1.61) | 7 (23.33) | 2 (3.28) | 13 (35.14) | ||||||
pN stage | 0.001 | 0.15 | 0.004 | 0.15 | ||||||||
0 | 128 (99.22) | 74 (88.10) | 62 (100.0) | 29 (96.67) | 60 (98.36) | 31 (83.78) | ||||||
1 | 0 (0.00) | 5 (5.95) | 0 (0.00) | 0 (0.00) | 1 (1.64) | 0 (0.00) | ||||||
2 | 1 (0.78) | 5 (5.95) | 0 (0.00) | 1 (3.33) | 0 (0.00) | 6 (16.22) | ||||||
Adjuvant therapy | <0.001 | <0.001 | 0.004 | 0.88 | ||||||||
No | 125 (96.90) | 70 (83.33) | 62(100.0) | 22 (73.33) | 59 (96.72) | 29 (78.38) | ||||||
Yes | 4 (3.10) | 14 (16.67) | 0 (0.00) | 8 (26.67) | 2 (3.28) | 8 (21.62) | ||||||
Tumor location | 0.39 | 0.61 | 0.41 | 0.82 | ||||||||
RUL | 52 (40.31) | 25 (29.76) | 22 (35.48) | 14 (46.67) | 21 (34.43) | 15 (40.54) | ||||||
RML | 7 (5.43) | 5 (5.95) | 7 (11.29) | 2 (6.67) | 7 (11.48) | 1 (2.70) | ||||||
RLL | 18 (13.95) | 13 (15.48) | 10 (16.13) | 2 (6.67) | 12 (19.67) | 5 (13.51) | ||||||
LUL | 36 (27.91) | 28 (33.33) | 15 (24.19) | 7 (23.33) | 13 (21.31) | 8 (21.62) | ||||||
LLL | 16 (12.40) | 13 (15.48) | 8 (12.90) | 5 (16.67) | 8 (13.11) | 8 (21.62) | ||||||
cT stage | 0.35 | 0.054 | 0.03 | 0.16 | ||||||||
1a | 25 (19.38) | 10 (11.90) | 8 (12.90) | 3 (10.00) | 6 (9.84) | 1 (2.70) | ||||||
1b | 78 (60.47) | 56 (66.67) | 45 (72.58) | 16 (53.33) | 43 (70.49) | 20 (54.05) | ||||||
1c | 26 (20.16) | 18 (21.43) | 9 (14.52) | 11 (36.67) | 12 (19.67) | 16 (43.24) |
Data are presented as mean ± standard deviation or n (%). P value represented the results of the univariate analysis of each clinical feature between HGP+ and HGP− groups in the training, validation, and test sets. A P value <0.05 indicates a significant difference. HGP+ group: percentage of HGP ≥5%; HGP− group: percentage of HGP <5%. cT, clinical T; HGP, high-grade pattern; LLL, left lower lobe; LUL, left upper lobe; pN, pathological N; pT, pathological T; RLL, right lower lobe; RML, right middle lobe; RUL, right upper lobe.
Multivariable analysis showed that density [odds ratio (OR) =2.27; 95% confidence interval (CI): 1.23–4.21; P=0.009] and CTR (OR =2.50; 95% CI: 1.54–4.04; P<0.001) were independent risk factors of HGP+. The detailed results of univariate and multivariable analysis are shown in Table S3. However, considering the subjectivity of decision-making for relevant radiological features and to improve model automation, clinical and radiological features were not included in the model analysis in this study.
Feature analysis and classifier selection
Before constructing the model, we need to screen for optimal features to improve the model’s accuracy and avoid over-fitting. So, we utilized the LassoCV method, incorporating 10-fold cross-validation, to select features. After LASSO feature selection, we finally extracted seven deep features and 10 radiomics features from 256 deep learning features and 1,836 radiomics features. These features have significantly higher weights. This process is graphically illustrated in Figure 2A-2C. For the optimization of hyperparameters within the model, we conducted 5-fold cross-validation on the training dataset and applied the Grid search algorithm as illustrated in Figure 2D.
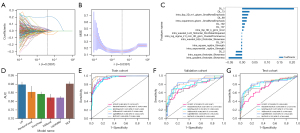
To select the most suitable classifier for the model construction, we applied six classifiers and compared their performances in each cohort. Finally, in the validation cohort, XGBoost outperformed other models with an area under the curve (AUC) of 0.862 (95% CI: 0.779–0.944), demonstrating its superior ability in the classification of tumors with and without HGPs. Random Forest, ExtraTrees, and LightGBM also presented competitive results, with AUCs of 0.837, 0.793, and 0.834. However, LR and MLP lagged slightly behind with AUCs of 0.752 and 0.748. The detailed results are shown in Figure 2E-2G. XGBoost’s performance in the validation cohort underscores its efficacy in predictive modeling. This suggests that XGBoost may be the most suitable classifier in the challenge of identifying whether the tumor contains HGPs. So, we chose it as the standard classifier to construct the fusion model in each cohort for further comparative analysis.
Model construction and performance comparison
After selecting XGBoost as the standard classifier, the fusion model combining radiomics and deep learning features denoted as DLR, demonstrated superior predictive performance across all cohorts. In the training, validation, and test cohort, DLR achieved impressive AUCs of 0.983 (95% CI: 0.969–0.996), 0.862 (95% CI: 0.779–0.944), and 0.832 (95% CI: 0.744–0.920), which means the fusion model can effectively distinguish patients with or without HGPs. The ROC curves for different models across each cohort are shown in Figure 3A-3C. Details of the AUC, accuracy, sensitivity, and specificity of each model are presented in Table 2. For instance, in the test cohort, the DLR has a sensitivity of 0.649, a specificity of 0.902, a negative predictive value of 0.809, and a positive predictive value of 0.800. It means when the model identifies a tumor as HGP+ or HGP−, the probability that it matches the pathological result is more than 80%, which has exceeded any currently known model. These findings also underscore the potential of integrating radiomics features and deep learning features to enhance the predictive accuracy in this classification.

Table 2
Cohorts | Models | Accuracy | AUC (95% CI) | Sensitivity | Specificity | PPV | NPV |
---|---|---|---|---|---|---|---|
Training | Radiomics | 0.812 | 0.886 (0.842–0.930) | 0.875 | 0.770 | 0.713 | 0.904 |
Deep learning | 0.901 | 0.939 (0.908–0.971) | 0.875 | 0.919 | 0.875 | 0.919 | |
DLR | 0.924 | 0.983 (0.969–0.996) | 0.977 | 0.889 | 0.851 | 0.984 | |
Validation | Radiomics | 0.688 | 0.800 (0.701–0.899) | 0.871 | 0.600 | 0.509 | 0.907 |
Deep learning | 0.865 | 0.870 (0.772–0.967) | 0.774 | 0.908 | 0.800 | 0.894 | |
DLR | 0.802 | 0.862 (0.779–0.944) | 0.774 | 0.815 | 0.667 | 0.883 | |
Test | Radiomics | 0.653 | 0.787 (0.691–0.883) | 0.081 | 1.000 | 1.000 | 0.642 |
Deep learning | 0.776 | 0.814 (0.729–0.899) | 0.676 | 0.836 | 0.714 | 0.810 | |
DLR | 0.806 | 0.832 (0.744–0.920) | 0.649 | 0.902 | 0.800 | 0.809 |
AUC, area under curve; CI, confidence interval; DLR, deep learning radiomics; NPV, negative predictive value; PPV, positive predictive value.
For comparative analysis, we selected the best-performing classifiers based on their validation set performance to construct the other models. Detailed results for the radiomics model and deep learning model are provided in Appendices 4,5, respectively. In the training set, the discrimination performance of the fusion model outperformed the radiomics model (AUC: 0.983 vs. 0.886; DeLong’s test, P<0.001) and the deep learning model (AUC: 0.983 vs. 0.939; DeLong’s test, P=0.005). The fusion model was comparable to the deep learning model in the validation set (AUC: 0.862 vs. 0.870; P=0.79) and the test set (AUC: 0.832 vs. 0.814; DeLong’s test, P=0.59). Detailed results for DeLong’s test are shown in Figure S1.
The HL test is key for assessing a predictive model’s calibration. It compares predicted probabilities with actual outcomes. A higher HL statistic indicates better calibration, showing closer alignment between model predictions and observed outcomes. In our study, the DLR shows good coherence between model prediction and the actual observation, evidenced by HL test statistics of 0.886 and 0.845 in the validation and test cohorts, suggesting its high effectiveness in the prediction (Figure 3D-3F). Figure 3G-3I also illustrates the DCA for the training, validation, and test sets. These curves reveal that our fusion model provides a higher clinical benefit in terms of the identification between the tumor with and without HGPs.
Discussion
In this study, we attempted to develop an optimal model to predict HGPs in clinical stage IA invasive non-mucinous cancers. The results show that the fusion model, which utilizes the XGBoost classifier, exhibits stronger predictive performance in the training set (AUC: 0.983), validation set (AUC: 0.862), and test set (AUC: 0.832) compared to the single radiomics model or deep learning model. In addition, the good calibration curve and DCA curve also demonstrate its excellent consistency and clinical application value.
Although the novel IASLC grading system defines poorly differentiated invasive lung adenocarcinoma as HGPs ≥20% (8), studies by Nitadori et al., Wang et al., and Kadota et al. have all found that the smaller percentage of high-risk subtypes affects prognosis as well (15,18,19). It is the reason why we defined the presence of HGPs (≥5%) as the main predictive variable. Several studies have utilized frozen sections to diagnose high-risk subtypes, however, their sensitivity remained limited. For instance, Yeh et al. reported diagnostic sensitivities of 37% and 69% for micropapillary and solid patterns, respectively (30). Zhao et al. improved the sensitivity (from 56.8% to 81.1%) of diagnosis for micropapillary patterns by including the concept of a filigree pattern (31). In contrast, the radiology model we conducted not only provides more integrated sensitivity and specificity, but also avoids the invasiveness and latency, Moreover, it plays an important role in decision-making regarding the need for more extensive resection and more aggressive adjuvant therapy for patients.
Although some studies have predicted high-risk subtypes of invasive lung adenocarcinoma based on imaging, such as Zhou et al. who used radiomics to predict micropapillary/solid type based on 18-fluorine-fluorodeoxyglucose (18F-FDG) positron emission tomography (PET)/CT images (32), PET/CT is not as prevalent as CT for preoperative screening in the clinic, especially for early-stage lung cancers with small diameters. Secondly, the study did not limit the clinical staging to stage IA, and the guidance for the diagnosis and treatment of invasive lung adenocarcinoma at stage IA is more practical in clinical practice. He et al. used four machine learning algorithms: a generalized linear model (GLM), Naïve Bayes, SVM, and random forest classifiers, to predict the presence of micropapillary/solid phenotypes, which proved that a radiomics-based machine learning approach is a powerful prediction tool (33). However, the study’s AUC of each algorithm in the internal validation set was only 0.74, 0.75, 0.73, and 0.72, which is much lower than the AUC (0.862) in the validation set of our study. Therefore, we avoided the disadvantages mentioned above by limiting our prediction to lung cancers of clinical stage IA in preoperative CT images, which also demonstrated the potential value of our model for clinical applications.
It is worth mentioning that the test set in our study is a special dataset. The well-known human cost of outlining ROIs greatly restricts the clinical application of related techniques. To improve the clinical applicability and the degree of model automation, we used the developed auto-segmented VNet model in the test set. The average Dice coefficients in the training and validation sets are 0.900 and 0.836, respectively. The auto-segmentation algorithm not only avoids the burden of manual outlining but also demonstrates the automation of our predictive models. As we know, the more automated a model is, the more potential it will have for widespread clinical application.
In addition, the effect of different CT layer thicknesses on model efficacy is controversial. Park et al. concluded that there was no difference between different CT layer thicknesses in survival prediction of NSCLC (34), but the prediction performance of thick CT for lung adenocarcinoma with EGFR mutations was significantly lower than that of thin CT model in Li et al.’s study (35). In our study, there were more thick-layer CT images in the test set, which were transferred from the training and validation sets. The AUCs of the validation set vs. the test set for this were 0.862 vs. 0.832, with a slight but insignificant decrease in performance. This demonstrates that we still have the generalization ability on different thickness CT images when we achieve a high degree of automation by automatic segmentation.
Although we compared many algorithms, many of them are known as black box methods, which automatically select the data features that contribute most to high-performance outputs without providing easily interpretable implications of the results (36). Therefore, to investigate the deep learning models’ recognition abilities on various samples, we used the gradient-weighted class activation mapping (Grad-CAM) technique for visualization. Figure S2 illustrates Grad-CAM’s application, highlighting the activations in the final convolutional layer relevant to the results. This helps identify image regions that significantly impact the model’s decision-making, offering insights into its interpretability.
Limitation
Although we demonstrated the performance and clinical value of our prediction model, there are still some limitations in this study. Firstly, it is a single-center retrospective study with a limited sample size and unavoidable selection bias. A prospective multi-center study is needed for further validation before its application to clinical practice. Secondly, there may be inconsistency in the assessment of pathological subtypes by pathologists, especially when multiple subtypes coexist and HGPs account for a smaller proportion. Thirdly, early fusion is used in our study, it is the fusion of information from all modalities at the input stage, uniformly fed into the classifier. Whether other fusion modalities applied to our study would have superior results deserves more in-depth research in the future. Finally, while tools such as saliency and attention maps generated by algorithm may aid interpretation, the lack of true transparency and interpretability may have a negative impact on the prospects for clinical application.
Conclusions
In summary, we built a fusion model that combines radiomics features and deep learning features to predict the presence of HGPs in invasive lung adenocarcinomas. It demonstrates a more prominent discriminative ability compared to the radiomics model and deep learning model. Compared to previous studies, it not only improves the prediction performance but also enhances the automation and generalization capabilities, which make it more suitable for clinical application. Although more extensive prospective studies are deserved for further validation, the model is still worth generalizing.
Acknowledgments
None.
Footnote
Reporting Checklist: The authors have completed the TRIPOD reporting checklist. Available at https://tlcr.amegroups.com/article/view/10.21037/tlcr-24-995/rc
Data Sharing Statement: Available at https://tlcr.amegroups.com/article/view/10.21037/tlcr-24-995/dss
Peer Review File: Available at https://tlcr.amegroups.com/article/view/10.21037/tlcr-24-995/prf
Funding: The present study was supposed by
Conflicts of Interest: All authors have completed the ICMJE uniform disclosure form (available at https://tlcr.amegroups.com/article/view/10.21037/tlcr-24-995/coif). The authors have no conflicts of interest to declare.
Ethical Statement: The authors are accountable for all aspects of the work in ensuring that questions related to the accuracy or integrity of any part of the work are appropriately investigated and resolved. The study was conducted in accordance with the Declaration of Helsinki (as revised in 2013). This retrospective study was approved by the Ethics Review Committee of Taizhou Hospital, Zhejiang Province (No. KL20240438), and the requirement for informed consent has been waived due to the retrospective nature of the study.
Open Access Statement: This is an Open Access article distributed in accordance with the Creative Commons Attribution-NonCommercial-NoDerivs 4.0 International License (CC BY-NC-ND 4.0), which permits the non-commercial replication and distribution of the article with the strict proviso that no changes or edits are made and the original work is properly cited (including links to both the formal publication through the relevant DOI and the license). See: https://creativecommons.org/licenses/by-nc-nd/4.0/.
References
- Sung H, Ferlay J, Siegel RL, et al. Global Cancer Statistics 2020: GLOBOCAN Estimates of Incidence and Mortality Worldwide for 36 Cancers in 185 Countries. CA Cancer J Clin 2021;71:209-49. [Crossref] [PubMed]
- Travis WD, Brambilla E, Noguchi M, et al. International association for the study of lung cancer/american thoracic society/european respiratory society international multidisciplinary classification of lung adenocarcinoma. J Thorac Oncol 2011;6:244-85. [Crossref] [PubMed]
- Mikubo M, Tamagawa S, Kondo Y, et al. Micropapillary and solid components as high-grade patterns in IASLC grading system of lung adenocarcinoma: Clinical implications and management. Lung Cancer 2024;187:107445. [Crossref] [PubMed]
- Kadota K, Kushida Y, Kagawa S, et al. Cribriform Subtype is an Independent Predictor of Recurrence and Survival After Adjustment for the Eighth Edition of TNM Staging System in Patients With Resected Lung Adenocarcinoma. J Thorac Oncol 2019;14:245-54.
- Bai J, Deng C, Zheng Q, et al. Comprehensive analysis of mutational profile and prognostic significance of complex glandular pattern in lung adenocarcinoma. Transl Lung Cancer Res 2022;11:1337-47. [Crossref] [PubMed]
- Yang F, Dong Z, Shen Y, et al. Cribriform growth pattern in lung adenocarcinoma: More aggressive and poorer prognosis than acinar growth pattern. Lung Cancer 2020;147:187-92. [Crossref] [PubMed]
- Warth A, Muley T, Kossakowski C, et al. Prognostic impact and clinicopathological correlations of the cribriform pattern in pulmonary adenocarcinoma. J Thorac Oncol 2015;10:638-44. [Crossref] [PubMed]
- Moreira AL, Ocampo PSS, Xia Y, et al. A Grading System for Invasive Pulmonary Adenocarcinoma: A Proposal From the International Association for the Study of Lung Cancer Pathology Committee. J Thorac Oncol 2020;15:1599-610. [Crossref] [PubMed]
- Nicholson AG, Tsao MS, Beasley MB, et al. The 2021 WHO Classification of Lung Tumors: Impact of Advances Since 2015. J Thorac Oncol 2022;17:362-87. [Crossref] [PubMed]
- Deng C, Zheng Q, Zhang Y, et al. Validation of the Novel International Association for the Study of Lung Cancer Grading System for Invasive Pulmonary Adenocarcinoma and Association With Common Driver Mutations. J Thorac Oncol 2021;16:1684-93. [Crossref] [PubMed]
- Rokutan-Kurata M, Yoshizawa A, Ueno K, et al. Validation Study of the International Association for the Study of Lung Cancer Histologic Grading System of Invasive Lung Adenocarcinoma. J Thorac Oncol 2021;16:1753-8. [Crossref] [PubMed]
- Hou L, Wang T, Chen D, et al. Prognostic and predictive value of the newly proposed grading system of invasive pulmonary adenocarcinoma in Chinese patients: a retrospective multicohort study. Mod Pathol 2022;35:749-56. [Crossref] [PubMed]
- Saji H, Okada M, Tsuboi M, et al. Segmentectomy versus lobectomy in small-sized peripheral non-small-cell lung cancer (JCOG0802/WJOG4607L): a multicentre, open-label, phase 3, randomised, controlled, non-inferiority trial. Lancet 2022;399:1607-17. [Crossref] [PubMed]
- Hattori A, Suzuki K, Takamochi K, et al. Segmentectomy versus lobectomy in small-sized peripheral non-small-cell lung cancer with radiologically pure-solid appearance in Japan (JCOG0802/WJOG4607L): a post-hoc supplemental analysis of a multicentre, open-label, phase 3 trial. Lancet Respir Med 2024;12:105-16. [Crossref] [PubMed]
- Nitadori J, Bograd AJ, Kadota K, et al. Impact of micropapillary histologic subtype in selecting limited resection vs lobectomy for lung adenocarcinoma of 2cm or smaller. J Natl Cancer Inst 2013;105:1212-20. [Crossref] [PubMed]
- Cha MJ, Lee HY, Lee KS, et al. Micropapillary and solid subtypes of invasive lung adenocarcinoma: clinical predictors of histopathology and outcome. J Thorac Cardiovasc Surg 2014;147:921-928.e2. [Crossref] [PubMed]
- Lee G, Lee HY, Jeong JY, et al. Clinical impact of minimal micropapillary pattern in invasive lung adenocarcinoma: prognostic significance and survival outcomes. Am J Surg Pathol 2015;39:660-6. [Crossref] [PubMed]
- Wang Y, Zheng D, Zheng J, et al. Predictors of recurrence and survival of pathological T1N0M0 invasive adenocarcinoma following lobectomy. J Cancer Res Clin Oncol 2018;144:1015-23. [Crossref] [PubMed]
- Kadota K, Yeh YC, Sima CS, et al. The cribriform pattern identifies a subset of acinar predominant tumors with poor prognosis in patients with stage I lung adenocarcinoma: a conceptual proposal to classify cribriform predominant tumors as a distinct histologic subtype. Mod Pathol 2014;27:690-700. [Crossref] [PubMed]
- Sereno M, He Z, Smith CR, et al. Inclusion of multiple high-risk histopathological criteria improves the prediction of adjuvant chemotherapy efficacy in lung adenocarcinoma. Histopathology 2021;78:838-48. [Crossref] [PubMed]
- Trejo Bittar HE, Incharoen P, Althouse AD, et al. Accuracy of the IASLC/ATS/ERS histological subtyping of stage I lung adenocarcinoma on intraoperative frozen sections. Mod Pathol 2015;28:1058-63. [Crossref] [PubMed]
- Wu S, Zhan W, Liu L, et al. Pretreatment radiomic biomarker for immunotherapy responder prediction in stage IB-IV NSCLC (LCDigital-IO Study): a multicenter retrospective study. J Immunother Cancer 2023;11:e007369. [Crossref] [PubMed]
- Han Y, Ma Y, Wu Z, et al. Histologic subtype classification of non-small cell lung cancer using PET/CT images. Eur J Nucl Med Mol Imaging 2021;48:350-60. [Crossref] [PubMed]
- Wang F, Wang CL, Yi YQ, et al. Comparison and fusion prediction model for lung adenocarcinoma with micropapillary and solid pattern using clinicoradiographic, radiomics and deep learning features. Sci Rep 2023;13:9302. [Crossref] [PubMed]
- Choi Y, Aum J, Lee SH, et al. Deep Learning Analysis of CT Images Reveals High-Grade Pathological Features to Predict Survival in Lung Adenocarcinoma. Cancers (Basel) 2021;13:4077. [Crossref] [PubMed]
- Yang Z, Cai Y, Chen Y, et al. A CT-Based Radiomics Nomogram Combined with Clinic-Radiological Characteristics for Preoperative Prediction of the Novel IASLC Grading of Invasive Pulmonary Adenocarcinoma. Acad Radiol 2023;30:1946-61. [Crossref] [PubMed]
- Xu J, Liu L, Ji Y, et al. Enhanced CT-Based Intratumoral and Peritumoral Radiomics Nomograms Predict High-Grade Patterns of Invasive Lung Adenocarcinoma. Acad Radiol 2025;32:482-92. [Crossref] [PubMed]
- Goldstraw P, Chansky K, Crowley J, et al. The IASLC Lung Cancer Staging Project: Proposals for Revision of the TNM Stage Groupings in the Forthcoming (Eighth) Edition of the TNM Classification for Lung Cancer. J Thorac Oncol 2016;11:39-51. [Crossref] [PubMed]
- Hou Z, Gao S, Liu J, et al. Clinical evaluation of deep learning-based automatic clinical target volume segmentation: a single-institution multi-site tumor experience. Radiol Med 2023;128:1250-61. [Crossref] [PubMed]
- Yeh YC, Nitadori J, Kadota K, et al. Using frozen section to identify histological patterns in stage I lung adenocarcinoma of ≤ 3 cm: accuracy and interobserver agreement. Histopathology 2015;66:922-38. [Crossref] [PubMed]
- Zhao S, Xie H, Su H, et al. Identification of filigree pattern increases the diagnostic accuracy of micropapillary pattern on frozen section for lung adenocarcinoma. Histopathology 2022;81:119-27. [Crossref] [PubMed]
- Zhou L, Sun J, Long H, et al. Imaging phenotyping using (18)F-FDG PET/CT radiomics to predict micropapillary and solid pattern in lung adenocarcinoma. Insights Imaging 2024;15:5. [Crossref] [PubMed]
- He B, Song Y, Wang L, et al. A machine learning-based prediction of the micropapillary/solid growth pattern in invasive lung adenocarcinoma with radiomics. Transl Lung Cancer Res 2021;10:955-64. [Crossref] [PubMed]
- Park S, Lee SM, Kim S, et al. Performance of radiomics models for survival prediction in non-small-cell lung cancer: influence of CT slice thickness. Eur Radiol 2021;31:2856-65. [Crossref] [PubMed]
- Li Y, Lu L, Xiao M, et al. CT Slice Thickness and Convolution Kernel Affect Performance of a Radiomic Model for Predicting EGFR Status in Non-Small Cell Lung Cancer: A Preliminary Study. Sci Rep 2018;8:17913. [Crossref] [PubMed]
- Hashimoto DA, Varas J, Schwartz TA. Practical Guide to Machine Learning and Artificial Intelligence in Surgical Education Research. JAMA Surg 2024;159:455-6. [Crossref] [PubMed]