Diagnostic accuracy of serum biomarkers MMP11 and SPP1 in non-small cell lung cancer: an analysis of sensitivity, specificity, and area under the curve
Highlight box
Key findings
• MMP11 and SPP1 are identified as novel serum biomarkers for non-small cell lung cancer (NSCLC), showing significant upregulation in tumor tissues compared to normal tissues across multiple datasets.
• In 200 NSCLC patients and 200 healthy controls, median serum MMP11 and SPP1 levels were 40.55 and 42.71 ng/mL, respectively, both significantly higher than in healthy individuals.
• Receiver operating characteristic analysis demonstrated robust diagnostic potential for both biomarkers, with MMP11 achieving an area under curve (AUC) of 0.9896 and SPP1 an AUC of 0.9053, significantly surpassing the conventional biomarker carcinoembryonic antigen (CEA), which had an AUC of 0.7109.
What is known and what is new?
• Current blood-based biomarkers for NSCLC, such as CEA and cytokeratin 19 fragment, often lack the requisite sensitivity and specificity for early detection, leading to potential false positives in benign conditions.
• This study introduces MMP11 and SPP1 as non-invasive serum biomarkers enhancing diagnostic accuracy in differentiating NSCLC from non-cancerous lung conditions.
What is the implication, and what should change now?
• The identification of MMP11 and SPP1 highlights their integration into standard diagnostic practices due to their superior sensitivity and specificity, which can improve early detection and patient outcomes. Therefore, NSCLC diagnosis should adopt various biomarkers rather than relying solely on the conventional marker CEA. Furthermore, validation studies in larger, diverse cohorts can confirm their applicability to lung cancer subtypes. Future research should explore upregulation mechanisms of MMP11 and SPP1 and enhance overall diagnostic accuracy alongside other existing biomarkers.
Introduction
Background
Roughly 80–85% of lung cancer diagnoses fall under the category of non-small cell lung cancer (NSCLC), which includes several distinct subtypes such as squamous cell carcinoma (SCC), adenocarcinoma, and large cell carcinoma (1,2). Despite advancements in treatment options, NSCLC remains a significant concern in oncology, largely due to its high incidence and mortality rates. Early detection and accurate diagnosis are critical for improving patient outcomes, as they enable timely intervention (3). Currently, standard diagnostic procedures primarily rely on imaging technologies, such as low-dose chest computed tomography (CT) scans, to locate lung nodules. When a nodule is identified, histological confirmation through tissue biopsy is performed, combined with molecular profiling for targeted therapies (4-6). However, these methodologies are often invasive, costly, and may not reliably detect the disease at an early stage, highlighting the pressing need for improved diagnostic techniques (3,7,8).
Rationale and knowledge gap
Although several blood-based biomarkers are available in clinical practice to assist in the detection of NSCLC, commonly used serum markers such as carcinoembryonic antigen (CEA), cytokeratin 19 fragment (CYFRA 21-1), and SCC antigen (SCC-Ag) exhibit significant limitations (9-11). For instance, elevated CEA levels can occur in various malignancies and some benign conditions, compromising its specificity for NSCLC (12). While CYFRA 21-1 demonstrates sensitivity for SCC, it lacks the ability to distinguish lung cancer from non-malignant lung diseases such as chronic obstructive pulmonary disease (COPD) (13,14). Similarly, SCC-Ag presence may not accurately indicate lung cancer due to potential elevations in benign inflammatory conditions (15). These shortcomings, particularly regarding sensitivity and specificity, create substantial barriers to the effective clinical utility of these biomarkers, especially in the detection of early-stage tumors or in differentiating subtypes of lung cancer.
Objective
This study aims to develop a novel blood-based biomarker that can enhance the health screening process for the early diagnosis of NSCLC. The primary objective is to improve both diagnostic accuracy and prognostic assessments by leveraging advancements in genomics and proteomics. Through this research, we aspire to establish a comprehensive diagnostic framework that not only addresses the current limitations of existing blood-based biomarkers but also offers a non-invasive and cost-effective solution for early detection of NSCLC.
Hypotheses
We propose two main hypotheses for this study: (I) the novel biomarkers identified through gene expression analysis will demonstrate superior sensitivity and specificity compared to existing serum biomarkers CEA in diagnosing NSCLC. (II) Utilizing these novel biomarkers in health screening contexts will significantly improve the early detection rates of NSCLC, leading to enhanced clinical management and improved patient outcomes. This research aims to address a potential need for innovative diagnostic tools that can better facilitate early detection and management of NSCLC, ultimately aiming to reduce cancer-related morbidity and mortality. We present this article in accordance with the STARD reporting checklist (available at https://tlcr.amegroups.com/article/view/10.21037/tlcr-2024-1068/rc).
Methods
Study design
This study employed a prospective cohort design, with data collection planned prior to performing the index tests (measurement of serum MMP11, SPP1, and CEA) and reference standards (diagnosis of NSCLC). By determining the study objectives and protocols in advance, we ensured systematic data collection processes.
Gene expression datasets
The Gene Expression Omnibus (GEO) (https://www.ncbi.nlm.nih.gov/geo/) and The Cancer Genome Atlas (TCGA) provided the gene expression data. The primary search terms included lung adenocarcinoma (LUAD) and lung squamous cell carcinoma (LUSC). Inclusion criteria for selected datasets were: (I) comparison of LUAD/LUSC with normal lung tissues; (II) use of human samples; (III) expression profile arrays, and (IV) each group containing at least five samples. Datasets were screened in accordance with ethical regulations, ensuring proper disclosure and consent obtained.
Identification and integration of common differentially expressed genes (DEGs)
The GEO database provided the gene expression profiles, which were then analyzed using R statistical software (version 3.5.1). DEGs were identified through the limma package, employing the robust multi-array average (RMA) algorithm for data preprocessing. A classical t-test was performed, with cutoff values established at an adjusted P value <0.05 and log2 fold change >1. Venn analysis was utilized to integrate common DEGs across datasets.
Gene Ontology (GO) enrichment analyses of common DEGs
The biological characteristics of commonly identified DEGs were determined through GO analysis (http://www.geneontology.org). GO enrichment analysis was performed using the g:Profiler tool (https://biit.cs.ut.ee/gprofiler/), a free online resource for functional classification of genes. Specifically, GO terms related to biological processes, cellular component, and molecular function were analyzed, with a significance level set at P<0.05.
Samples
The investigation involved analyzing blood samples from an equal number of NSCLC patients and healthy individuals, with 200 participants in each group. The cohort included 120 adenocarcinoma (61.5%) and 80 SCC (38.5%) cases, with histological grading classified as 79 grade 1 (39.5%), 60 grade 2 (30.0%) and 61 grade 3 (30.5%). Tumor staging adhered to the 8th edition of the American Joint Committee on Cancer (AJCC) Cancer Staging Manual: 123 patients (61.5%) were stage I and II, 77 (38.5%) stage III and IV. Subgroup analyses were performed to assess biomarker performance across histology, grade, and stage (Table 1). The intended sample size of 400 was determined based on the goal of achieving statistically significant results with sufficient power to detect clinically relevant differences in biomarker concentrations between patients and healthy controls. To reduce institutional bias, samples were obtained from two distinct National Biobanks: 200 healthy controls were sourced from Seoul St. Mary’s Hospital Biobank, while 200 NSCLC patients were recruited from Ajou University Medical Center Biobank. The study was conducted in accordance with the Declaration of Helsinki (as revised in 2013), and the study was approved by the Institutional Review Board (IRB) of the Catholic University of Korea College of Medicine (No. MC15SISI0015) and Kangnam St Peter’s Hospital (No. SPH20-24-002). Samples were gathered from 2015 through 2020. The healthy control samples were sourced from the National Biobank of Korea (Seoul St. Mary’s Hospital), where individuals underwent comprehensive health evaluations. These evaluations included annual physical examinations, blood tests (complete blood count, liver/kidney function tests), chest X-rays, and abdominal ultrasounds, aligning with standard health screening practices in South Korea. Initially, healthy control samples were randomly selected from individuals without a known history of cancer, as confirmed by these routine health examinations. This selection process resulted in a final sample size of 200 NSCLC patients and 200 healthy controls. It’s important to note that while we use the term “healthy controls” in the context of cancer-free status, this group may include individuals with conditions that could potentially elevate CEA levels, such as hepatitis, moderate fatty liver disease, Helicobacter pylori-associated gastritis, and colonic polyps (n=87). Additionally, some individuals in this group were found to have suspected respiratory conditions such as fibrosis, atelectasis, or lung nodules (n=36). However, due to the lack of tissue biopsies, definitive diagnoses were not available for these conditions. This approach to sample selection aligns with the National Biobank of Korea’s categorization of control groups, which includes “normal” and “disease-specific” controls. In this case, our healthy control group may be considered a “disease-specific” control, as it consists of participants who do not have the particular disease being studied (NSCLC) but may have other health conditions. By including a diverse range of individuals without cancer, this selection method provides a more representative sample of the general population, potentially offering valuable insights into the relationship between various health conditions and NSCLC patients. Patients had no known familial cancer histories. The reference standard for diagnosing NSCLC was established through a comprehensive process involving clinical evaluations. NSCLC diagnoses were based on established medical criteria that included various imaging techniques, such as chest X-rays and CT scans, combined with histopathological examination of biopsy samples. Patients who displayed respiratory symptoms or exhibited imaging findings suggestive of lung cancer underwent further diagnostic procedures, including biopsies performed through methods such as bronchoscopy or needle aspiration. These biopsies provided histological confirmation of the presence and type of lung cancer, allowing for accurate classification of the disease. Only patients with a confirmed diagnosis of NSCLC were included in the analysis, ensuring the reliability of the study outcomes. All diagnostic procedures strictly adhered to IRB guidelines, and informed consent was obtained from each participant prior to their involvement in the study, thereby ensuring ethical compliance throughout the research process. Information on normal and patient samples is described in Table 1.
Table 1
Characteristics | Normal (N=200) | Tumor (N=200) |
---|---|---|
Age (years) | ||
<60 | 120 | 82 |
≥60 | 80 | 118 |
Gender | ||
Male | 114 | 104 |
Female | 86 | 96 |
Pathologic types | ||
ADC | – | 120 |
SCC | – | 80 |
TNM stage | ||
I–II | – | 123 |
III–IV | – | 77 |
Grades | ||
1 | – | 79 |
2 | – | 60 |
3 | – | 61 |
Smoking status | ||
Never | 87 | 92 |
Current | 113 | 108 |
ADC, adenocarcinoma; NSCLC, non-small cell lung cancer; SCC, squamous cell carcinoma; TNM, tumor-node-metastasis.
Measurement of serum MMP11, SPP1, and CEA protein concentrations
Serum samples, pre-processed (centrifugation at 3,000 rpm for 10 min) and stored at −80 ℃ by biobanks, were thawed at 4 ℃ prior to analysis. Concentrations of MMP11 (Cat No. HUEB0498), SPP1 (Cat. No. HUEB0230), and CEA (Cat. No. HUFI00080) were determined using specific enzyme-linked immunosorbent assay (ELISA) kits from AssayGenie (Dublin, Ireland), following the manufacturer’s protocols. To minimize bias, serum samples were randomized, and analysts were blinded to group allocations during the assessment. Quality assurance measures included monitoring intra-assay and inter-assay coefficients of variation (CVs), which were maintained below 10% and 15%, respectively. All protein concentrations were measured in duplicate.
Defining MMP11, SPP1, and CEA cutoff value in ROC analysis
To assess the diagnostic performance of the chosen markers using binary classification, we employed receiver operating characteristic (ROC) curve analysis following the methodology described by Hanley and McNeil (16). The optimum cutoff values of MMP11, SPP1, and CEA for the diagnosis of NSCLC cancers were defined using the ROC curve and Youden index in sera obtained from healthy individuals and NSCLC cancer patients. Using the established cutoff points for serum levels of MMP11, SPP1, and CEA proteins, we computed the ranges of sensitivity and specificity. The serum levels of MMP11, SPP1, and CEA in NSCLC patients were analyzed to establish optimal cutoff values for NSCLC diagnosis in the study population. Various diagnostic parameters were calculated for these cutoff values, including sensitivity (true positive fraction, TPF), specificity (true negative fraction, TNF), false-negative fraction (FNF), false-positive fraction (FPF), positive predictive value (PPV), negative predictive value (NPV), accuracy, positive likelihood ratio (LR+), negative likelihood ratio (LR−), and diagnostic odds ratio (DOR), using previously described methodologies (17-19). We determined the cutoff values for serum MMP11, SPP1, and CEA concentrations by optimizing overall predictive performance using Youden’s J index [J(χ) = (sensitivity + specificity − 1)] derived from ROC analysis. Indeterminate results, which refer to those that cannot be definitively classified as positive or negative, were recorded during the analysis of serum protein concentrations for MMP11, SPP1, and CEA. Any indeterminate results from the index tests were categorized as false-positive or false-negative depending on the reference standard outcome. This conservative approach, known as the “worst-case scenario”, was implemented to account for potential bias in estimating diagnostic accuracy. The frequency of indeterminate results was diligently reported, along with the rationale for their classification, to provide transparency in the analysis and the impact of these results on overall findings.
Statistical analysis
Statistical analyses were conducted using MedCalc (MedCalc Software, Mariakerke, Belgium), Graphpad Prism (GraphPad Software, Inc., San Diego, CA, USA), and SAS (SAS Institute, Cary, NC, USA). The serum concentrations of MMP11, SPP1, and CEA were assessed in duplicates to validate reproducibility. Due to the left-skewed distribution of biomarker concentrations, data are reported in medians and interquartile ranges (IQRs). Group comparisons were performed using the Mann-Whitney U-test, with a P value <0.05 indicating statistical significance. Diagnostic performance was evaluated via the McNemar test.
Results
Identification of extracellular region and space genes as potential biomarkers for NSCLC
We conducted a comprehensive analysis of gene expression profiles across three independent datasets: GSE33479, GSE18842, and GSE32863, with the aim of identifying potential biomarkers for NSCLC. Applying rigorous selection criteria, including a P value cutoff of ≤0.05 and a log2 fold change of >1 or <−1, we identified DEGs in each dataset. This analysis yielded 1,403 DEGs in GSE33479, 2,042 DEGs in GSE18842, and 578 DEGs in GSE32863. Subsequently, we performed comprehensive GO analysis, identifying 30 genes residing within the extracellular region and space. Among these, seven key genes exhibited a predominant increase in abundance, indicating their potential role as biomarkers (Figure 1A). A heatmap illustrating the expression patterns across the three datasets is presented in Figure 1B-1D.
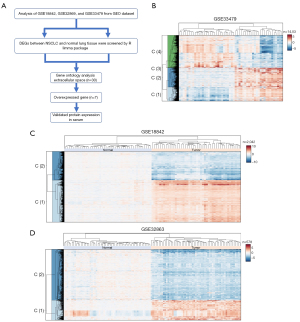
Differential expression and diagnostic potential of candidate biomarkers in NSCLC
To further investigate the clinical relevance and diagnostic potential of the identified seven candidate genes—CTHRC1, ERO1L, LAD1, MMP11, SFN, SPINK1, and SPP1—we conducted a detailed analysis of their expression profiles and ROC curves across the datasets (Table 2). In Figure 2A, we present the log2 expression levels of these genes in normal versus tumor samples from the GSE18842 dataset, revealing significant upregulation in tumor tissues compared to normal tissues, suggesting their involvement in NSCLC tumorigenesis. The ROC curves (Figure 2B) for each gene from the GSE18842 dataset demonstrate varying degrees of discriminative power, with area under the curve (AUC) values indicating their potential utility as biomarkers for distinguishing between normal and tumor tissues. This analysis showed consistent expression patterns across the GSE32863 and GSE33479 datasets (Figure 2C-2F).
Table 2
Genes | GSE18842 | GSE32863 | GSE33479 | ||||||||||||
---|---|---|---|---|---|---|---|---|---|---|---|---|---|---|---|
AUC | Std. error | 95% CI | P value | AUC | Std. error | 95% CI | P value | AUC | Std. error | 95% CI | P value | ||||
CTHRC1 | 0.982 | 0.010 | 0.962 to 1.00 | <0.001 | 0.907 | 0.028 | 0.852 to 0.961 | <0.001 | 0.834 | 0.056 | 0.725 to 0.943 | <0.001 | |||
ERO1L | 0.942 | 0.031 | 0.881 to 1.00 | <0.001 | 0.966 | 0.019 | 0.930 to 1.00 | <0.001 | 0.927 | 0.036 | 0.858 to 0.997 | <0.001 | |||
LAD1 | 0.930 | 0.032 | 0.868 to 0.993 | <0.001 | 0.943 | 0.023 | 0.898 to 0.989 | <0.001 | 0.911 | 0.041 | 0.830 to 0.991 | <0.001 | |||
MMP11 | 0.984 | 0.011 | 0.961 to 1.00 | <0.001 | 0.991 | 0.006 | 0.979 to 1.00 | <0.001 | 0.933 | 0.037 | 0.861 to 1.00 | <0.001 | |||
SFN | 0.882 | 0.035 | 0.813 to 0.952 | <0.001 | 0.963 | 0.0145 | 0.935 to 0.991 | <0.001 | 0.925 | 0.035 | 0.857 to 0.993 | <0.001 | |||
SPINK1 | 0.761 | 0.050 | 0.663 to 0.859 | <0.001 | 0.861 | 0.038 | 0.787 to 0.935 | <0.001 | 0.741 | 0.068 | 0.608 to 0.874 | 0.002 | |||
SPP1 | 0.961 | 0.021 | 0.920 to 1.00 | <0.001 | 0.950 | 0.020 | 0.910 to 0.990 | <0.001 | 0.910 | 0.038 | 0.835 to 0.984 | <0.001 |
AUC, area under the curve; CI, confidence interval; Std., standard.
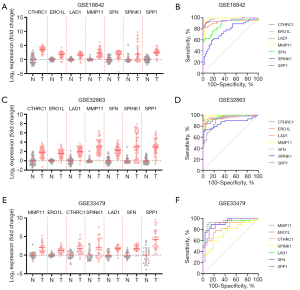
Additionally, we validated gene expression levels in TCGA datasets for LUAD and LUSC. Consistent with the previous findings, CTHRC1, ERO1L, LAD1, MMP11, SFN, SPINK1, and SPP1 were significantly elevated in tumor tissues compared to normal tissues across both datasets (Figure 3), reinforcing their potential role in lung cancer progression.
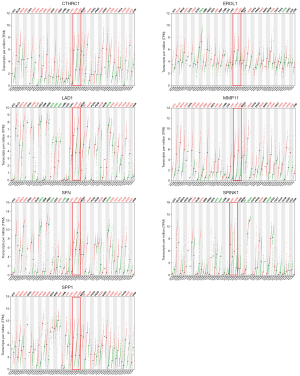
Based on their strong diagnostic potential, we selected MMP11 and SPP1 for further validation of their serum expression. Both genes exhibited robust discriminative power, supported by high AUC values, which underscores their effectiveness in distinguishing between normal and tumor tissues. MMP11 supports the processes of tumor invasion and metastasis by breaking down elements of the extracellular matrix, while SPP1 is critical for cell signaling and immune regulation, aiding tumor growth and invasiveness.
Tissue-level validation of MMP11 and SPP1
To complement serum biomarker analyses, we quantified MMP11 and SPP1 protein levels in NSCLC tissue lysates (n=20) using ELISA. NSCLC tissues exhibited significantly higher MMP11 (48.79±8.45 for SCC, P<0.001; 39.50±3.31 for ADC, P<0.001) and SPP1 (58.62±13.28 for SCC, P<0.001; 52.37±11.48 for ADC, P<0.001) concentrations compared to adjacent normal tissues (6.85±1.37 for MMP11; 6.54±2.17 for SPP1) (Figure 4A). These findings corroborate TCGA messenger RNA (mRNA) data (Figure 3) and confirm tissue-level dysregulation of both biomarkers in NSCLC.
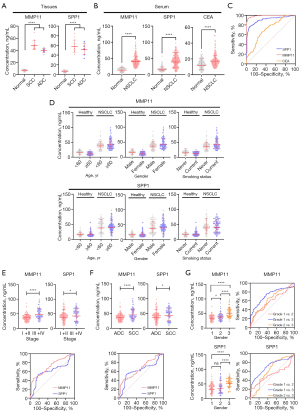
Analysis of protein concentration in serum samples from normal individuals and NSCLC patients
Focusing on MMP11 and SPP1, we conducted an investigation into their protein expression in serum, as these two biomarkers demonstrated consistently high diagnostic performance across the three GEO datasets, with AUC values exceeding 0.9 in all datasets. To assess serum protein concentrations and their potential as novel biomarkers, we studied 200 NSCLC patients and 200 healthy controls (Figure 4B).
The results indicate elevated serum levels of MMP11 (median: 40.55 ng/mL, IQR, 31.89–47.50 ng/mL) and SPP1 (median: 42.71 ng/mL, IQR, 29.12–54.29 ng/mL) in NSCLC patients compared to healthy individuals (median: 16.01 ng/mL, IQR, 10.63–18.46 ng/mL for MMP11; median: 16.11 ng/mL, IQR, 12.85–19.30 ng/mL for SPP1).
Comparatively, conventional markers such as CEA also showed elevation, but to a lesser extent (median: 16.91 ng/mL, IQR, 13.49–49.76 ng/mL in NSCLC; median: 12.81 ng/mL, IQR, 8.74–16.29 ng/mL in healthy controls). The ROC curve analysis (Figure 4C and Table 3) demonstrated strong discriminatory power, with MMP11 and SPP1 showing outstanding AUC values of 0.9896 and 0.9053, respectively. These values were notably superior to that of CEA, which had an AUC of 0.7109. Specifically, in this refined healthy control group, the median CEA level was 4.15 ng/mL with a standard deviation (SD) of 1.16 ng/mL (data not shown).
Table 3
Genes | Variables | Values |
---|---|---|
MMP11 | AUC | 0.990 |
Std. error | 0.003 | |
95% CI | 0.983 to 0.996 | |
P value | <0.001 | |
SPP1 | AUC | 0.905 |
Std. error | 0.016 | |
95% CI | 0.874 to 0.937 | |
P value | <0.001 | |
CEA | AUC | 0.711 |
Std. error | 0.026 | |
95% CI | 0.661 to 0.761 | |
P value | <0.001 |
AUC, area under the curve; CI, confidence interval; NSCLC, non-small cell lung cancer; Std., standard.
Determining the optimal cutoff values for NSCLC diagnosis revealed 22.2 ng/mL for MMP11, 22.89 ng/mL for SPP1, and 14.95 ng/mL for CEA. MMP11 and SPP1 showed promising diagnostic accuracy with sensitivity values of 94.53% and 83.17%, and specificity values of 94.97% and 83.84%, respectively, compared to CEA’s sensitivity of 66.83% and specificity of 67.69% (Table 4).
Table 4
Variables | MMP11 | SPP1 | CEA |
---|---|---|---|
Cut-off (ng/mL) | 22.2 | 22.89 | 14.95 |
Sensitivity (TPF) | 0.945 | 0.832 | 0.668 |
Specificity (TNF) | 0.950 | 0.838 | 0.677 |
PPV | 0.95 | 0.84 | 0.685 |
NPV | 0.945 | 0.83 | 0.66 |
LR+ | 18.811 | 5.146 | 2.069 |
LR− | 0.058 | 0.201 | 0.490 |
Accuracy | 0.948 | 0.835 | 0.673 |
DOR | 326.455 | 25.632 | 4.221 |
Youden’s index | 0.895 | 0.670 | 0.345 |
False discovery rate | 0.055 | 0.168 | 0.332 |
False omission rate | 0.050 | 0.162 | 0.323 |
DOR, diagnostic odds ratio; LR+, positive likelihood ratio; LR−, negative likelihood ratio; NPV, negative predictive value; NSCLC, non-small cell lung cancer; PPV, positive predictive value; TNF, true negative fraction; TPF, true positive fraction.
Moreover, MMP11 and SPP1 expression levels demonstrated consistency across age, gender, and smoking status (Figure 4D). However, a robust correlation was observed between these biomarkers and key clinical parameters. MMP11 and SPP1 levels were significantly elevated in advanced disease stages (III/IV) compared to early stages (I/II), with a statistically found difference (P<0.001 for MMP11 and P=0.02 for SPP1; Figure 4E). Furthermore, SCC patients exhibited markedly higher expression levels than those with adenocarcinoma (P<0.001 for MMP11 and P=0.01 for SPP1; Figure 4F). Poorly differentiated tumors showed a 1.8-fold and 1.4-fold increase in MMP11 and SPP1 levels relative to well-differentiated tumors [P=0.045 (grade 1 vs. 2), P<0.001 (grade 1 vs. 3), and P<0.001 (grade 2 vs. 3) for MMP11; P=0.25 (grade 1 vs. 2), P<0.001 (grade 1 vs. 3), and P<0.001 (grade 2 vs. 3) for SPP1; Figure 4G], underscoring the prognostic relevance of histological grading. Subgroup analyses using ROC curves revealed non-significant diagnostic accuracy across all stages (MMP11 AUC: 0.7214; SPP1 AUC: 0.6142) and histological subtypes (MMP11 AUC: 0.6000–0.7281; SPP1 AUC: 0.5264–0.7107) (Figure 4E-4G and Table 5). These findings reinforce the clinical utility of MMP11 and SPP1 as reliable biomarkers. Consequently, we advocate for their incorporation into non-invasive diagnostic strategies to enhance NSCLC diagnosis accuracy and improve patient outcomes.
Table 5
Variables | MMP11 | SPP1 | |||||||
---|---|---|---|---|---|---|---|---|---|
AUC | Std. error | 95% CI | P value | AUC | Std. error | 95% CI | P value | ||
ADC vs. SCC | 0.6634 | 0.03974 | 0.5856 to 0.7413 | <0.001 | 0.6201 | 0.04318 | 0.5355 to 0.7047 | 0.004 | |
Stage I+II vs. III+IV | 0.7214 | 0.03766 | 0.6475 to 0.7952 | <0.001 | 0.6142 | 0.04397 | 0.5280 to 0.7004 | 0.007 | |
Grade 1 vs. 2 | 0.6000 | 0.04599 | 0.5099 to 0.6902 | 0.03 | 0.5264 | 0.05092 | 0.4266 to 0.6262 | 0.56 | |
Grade 1 vs. 3 | 0.7281 | 0.03497 | 0.6596 to 0.7967 | <0.001 | 0.7107 | 0.03541 | 0.6413 to 0.7801 | <0.001 | |
Grade 2 vs. 3 | 0.6335 | 0.04403 | 0.5472 to 0.7198 | 0.004 | 0.6202 | 0.04820 | 0.5257 to 0.7146 | 0.01 |
ADC, adenocarcinoma; AUC, area under the curve; CI, confidence interval; NSCLC, non-small cell lung cancer; SCC, squamous cell carcinoma; Std., standard.
Discussion
Key findings
The identification of MMP11 and SPP1 as potential biomarkers for NSCLC may represent an advancement in diagnostic methodologies. Detailed gene expression analysis from multiple datasets demonstrated that both MMP11 and SPP1 exhibited substantial upregulation in NSCLC tissues, reinforcing their potential as biomarkers. Notably, in ROC curve analyses, both biomarkers showed strong discriminatory capability with AUC values exceeding 0.9, significantly surpassing the conventional biomarker CEA, which had an AUC of 0.7109. Furthermore, MMP11’s sensitivity was found to be 94.53% with a specificity of 94.97%, while SPP1 showed a sensitivity of 83.17% and a specificity of 83.84%. These metrics highlight MMP11 and SPP1 as superior diagnostic tools, particularly critical for early detection of NSCLC.
Strengths and limitations
This study is strengthened by its comprehensive analysis using extensive datasets, which provides reliable evidence for the diagnostic performance of MMP11 and SPP1. The multi-dataset approach and rigorous statistical analyses lend credibility to our findings, offering a robust foundation for future research in NSCLC biomarker development. However, several limitations must be acknowledged. The findings were derived from a controlled setting, necessitating validation in larger and more diverse cohorts to ensure generalizability. The smaller sample sizes for specific subgroups constrained the robustness of the analyses, potentially limiting the applicability of our results to certain patient populations. It’s important to note that while our control group was cancer-free, it may include individuals with undiagnosed comorbidities that could influence biomarker levels. This highlights the need for more stringent screening protocols in future studies. Additionally, the specificity of MMP11 and SPP1 for NSCLC versus other cancers remains unclear and requires further investigation to establish their utility as NSCLC-specific biomarkers. The cross-sectional nature of our study precludes the assessment of biomarker dynamics over time. Longitudinal studies are needed to evaluate the prognostic utility of MMP11 and SPP1 and their potential for monitoring disease progression or treatment response. While we observed correlations between serum MMP11/SPP1 levels and tissue mRNA expression trends (Figures 3,4), direct comparisons between paired tissue and serum samples were not performed. This represents a limitation in our ability to directly link tissue-level expression with serum biomarker levels. Furthermore, the lack of immunohistochemical validation in NSCLC tissues is a critical gap that should be addressed in subsequent studies to confirm the cellular origin and distribution of these biomarkers within tumor tissues. The potential influence of external factors, such as genetic mutations or environmental conditions, on the expression levels of MMP11 and SPP1 requires further investigation. These factors could impact biomarker levels and affect their diagnostic accuracy in different patient populations. Lastly, while our study the lack of various non-malignant lung diseases samples limits direct comparison with this clinically relevant population. Addressing these limitations in future research will be crucial for validating the clinical utility of MMP11 and SPP1 as biomarkers for NSCLC and for developing more accurate and reliable diagnostic tools for this devastating disease.
Comparison with similar researches
Current blood-based biomarkers in NSCLC, including CEA, have demonstrated some utility in monitoring disease progression and treatment response; however, they often lack the specificity and sensitivity required for early diagnosis (20). CEA, while historically significant, has limitations in terms of false positives due to elevated levels in benign conditions, leading to potential misdiagnoses (21,22). While MMP11 and SPP1 demonstrate strong diagnostic performance in NSCLC, their roles in other malignancies, such as breast and ovarian cancers, suggest broader oncogenic functions (23-25). For example, MMP11 facilitates tumor-stroma interactions in pancreatic ductal adenocarcinoma, while SPP1 drives metastasis in hepatocellular carcinoma. This pan-cancer relevance underscores the need to evaluate their specificity for NSCLC in diverse cohorts. Moreover, previous studies have shown some utility in other biomarkers, such as CYFRA 21-1 and neuron-specific enolase (NSE), which also face limitations related to early-stage disease sensitivity (26-28). The performance of MMP11 and SPP1 as biomarkers appears to reflect a broader trend in research focusing on the extracellular region and space genes. Unlike conventional biomarkers, MMP11 and SPP1 are intricately linked to critical tumor microenvironment dynamics, suggesting that the emerging focus on these markers may address some limitations previously associated with established biomarkers.
Explanations of findings
The observed performance of MMP11 and SPP1 in serum analyses can be attributed to their roles in tumor microenvironment dynamics and extracellular matrix remodeling. The upregulation of these biomarkers in NSCLC indicates their potential involvement in cancer progression and metastasis. MMP11 and SPP1 contribute to NSCLC pathogenesis through distinct mechanisms. MMP11, a stromal enzyme, degrades collagen IV and laminin, enabling tumor invasion. SPP1 (osteopontin) interacts with CD44 and integrins to activate PI3K/Akt pathways, promoting cell survival and chemoresistance. Both biomarkers are overexpressed in tumor-associated fibroblasts and macrophages, highlighting their role in shaping the NSCLC microenvironment further underlying the potential utility of these markers in clinical practice. Also, the elevated MMP11/SPP1 levels in SCC and advanced-stage tumors (Figure 4E) may reflect subtype-specific microenvironmental interactions. Driver mutations (e.g., EGFR, KRAS) could further modulate these biomarkers’ expression, necessitating mutation-stratified analyses in future studies.
Implications and actions needed
The findings of this study underscore the urgent need to incorporate MMP11 and SPP1 into routine diagnostic and therapeutic strategies for NSCLC. Moving away from over-reliance on traditional markers like CEA, which often fail to provide adequate specificity, the integration of these novel biomarkers could substantially improve early detection and consequently enhance patient outcomes through timely interventions. Our ELISA-based tissue analysis bridges the gap between serum protein levels and tissue-level dysregulation, demonstrating concordant MMP11/SPP1 upregulation in NSCLC tissues. While immunohistochemistry (IHC) remains valuable for spatial profiling, ELISA quantification in tissue lysates provides a pragmatic alternative when specimen availability is limited. This approach offers a quantitative assessment of biomarker expression, complementing the spatial information provided by IHC. However, additional studies are necessary to validate these findings in diverse populations and to explore the regulatory mechanisms underlying the expression of MMP11 and SPP1. Future research should evaluate MMP11 and SPP1 in COPD cohorts to further validate their diagnostic utility, and focus on examining these biomarkers’ effectiveness in conjunction with existing biomarkers to improve overall diagnostic accuracy. This comprehensive approach will contribute to the evolution of non-invasive cancer diagnostics in NSCLC. While MMP11 and SPP1 may be elevated in other malignancies, their diagnostic performance in NSCLC remains superior to conventional biomarkers. Further studies comparing their expression across cancer types are warranted to establish their specificity for NSCLC. Additionally, future investigations should integrate IHC to resolve subcellular localization patterns and stromal contributions, providing a more complete picture of biomarker expression in the tumor microenvironment. By pursuing these research directions, we can refine the clinical application of MMP11 and SPP1 as biomarkers, potentially revolutionizing NSCLC diagnosis and monitoring. While our results are promising, further validation in larger, diverse cohorts is necessary to confirm the clinical utility of these biomarkers. This multifaceted approach to biomarker validation and implementation holds promise for improving patient care and outcomes in NSCLC.
Conclusions
In summary, the identification of MMP11 and SPP1 as novel serum biomarkers for NSCLC suggests a potential advancement in the landscape of cancer diagnostics. The findings from this study demonstrate that both biomarkers exhibit significant upregulation in NSCLC tissues and display superior sensitivity and specificity compared to traditional blood-based markers such as CEA. MMP11 and SPP1 not only show strong diagnostic performance in distinguishing NSCLC from non-cancerous conditions but also hold considerable potential for enhancing early detection efforts, which is vital for improving patient outcomes.
The comprehensive gene expression analysis conducted across multiple datasets has established MMP11 and SPP1 as reliable indicators of disease presence and progression. These biomarkers are linked to crucial biological processes within the tumor microenvironment, particularly in extracellular matrix remodeling and tumor invasion. Their ability to provide a more targeted and accurate assessment of NSCLC reinforces the necessity for their integration into routine diagnostic protocols, particularly for early-stage disease, where timely intervention is critical.
Despite the significant implications of this study, it is essential to recognize the limitations inherent in the research, such as the need for validation across larger and more diverse cohorts and the exploration of external factors that could influence biomarker expression. Future investigations are warranted to elucidate the underlying mechanisms of MMP11 and SPP1 upregulation and to assess their combined efficacy with other established biomarkers.
In light of these findings, the integration of MMP11 and SPP1 into clinical practice may contribute to improving NSCLC diagnostics, providing healthcare professionals with enhanced tools for early detection and personalized treatment planning. The ongoing development of non-invasive, blood-based diagnostic strategies is essential for addressing the public health challenge posed by NSCLC and could ultimately lead to improved prognostic outcomes for patients afflicted with this malignancy.
Acknowledgments
We would like to thank Ajou University Medical Center, a member of the National Biobank of Korea, which is supported by the Ministry of Health and Welfare, for providing the serum samples and clinical information on non-small cell lung cancer. We would like to thank Harrisco (www.harrisco.net) for assistance with English editing.
Footnote
Reporting Checklist: The authors have completed the STARD reporting checklist. Available at https://tlcr.amegroups.com/article/view/10.21037/tlcr-2024-1068/rc
Peer Review File: Available at https://tlcr.amegroups.com/article/view/10.21037/tlcr-2024-1068/prf
Funding: None.
Conflicts of Interest: All authors have completed the ICMJE uniform disclosure form (available at https://tlcr.amegroups.com/article/view/10.21037/tlcr-2024-1068/coif). The authors have no conflicts of interest to declare.
Ethical Statement: The authors are accountable for all aspects of the work in ensuring that questions related to the accuracy or integrity of any part of the work are appropriately investigated and resolved. The study was conducted in accordance with the Declaration of Helsinki (as revised in 2013), and the study was approved by the Institutional Review Board (IRB) of the Catholic University of Korea College of Medicine (No. MC15SISI0015) and Kangnam St Peter’s Hospital (No. SPH20-24-002). Anonymized serum, NSCLC cancer tissues, and clinical data were provided through the Korea Biobank network of Ajou University Medical Center and Catholic University Gangnam St Mary’s Hospital. Informed consent was obtained from each participant prior to participation in the study.
Open Access Statement: This is an Open Access article distributed in accordance with the Creative Commons Attribution-NonCommercial-NoDerivs 4.0 International License (CC BY-NC-ND 4.0), which permits the non-commercial replication and distribution of the article with the strict proviso that no changes or edits are made and the original work is properly cited (including links to both the formal publication through the relevant DOI and the license). See: https://creativecommons.org/licenses/by-nc-nd/4.0/.
References
- Siegel RL, Miller KD, Jemal A. Cancer statistics, 2020. CA Cancer J Clin 2020;70:7-30. [Crossref] [PubMed]
- Duma N, Santana-Davila R, Molina JR. Non-Small Cell Lung Cancer: Epidemiology, Screening, Diagnosis, and Treatment. Mayo Clin Proc 2019;94:1623-40. [Crossref] [PubMed]
- Kratzer TB, Bandi P, Freedman ND, et al. Lung cancer statistics, 2023. Cancer 2024;130:1330-48. [Crossref] [PubMed]
- Peters AA, Wiescholek N, Müller M, et al. Impact of artificial intelligence assistance on pulmonary nodule detection and localization in chest CT: a comparative study among radiologists of varying experience levels. Sci Rep 2024;14:22447. [Crossref] [PubMed]
- Gazdar AF, Bunn PA, Minna JD. Small-cell lung cancer: what we know, what we need to know and the path forward. Nat Rev Cancer 2017;17:725-37. [Crossref] [PubMed]
- Zhu J, Wang W, Xiong Y, et al. Evolution of lung adenocarcinoma from preneoplasia to invasive adenocarcinoma. Cancer Med 2023;12:5545-57. [Crossref] [PubMed]
- Wistuba II, Gazdar AF. Lung cancer preneoplasia. Annu Rev Pathol 2006;1:331-48. [Crossref] [PubMed]
- Gazdar AF, Brambilla E. Preneoplasia of lung cancer. Cancer Biomark 2010;9:385-96. [Crossref] [PubMed]
- Travis WD, Brambilla E, Noguchi M, et al. International association for the study of lung cancer/american thoracic society/european respiratory society international multidisciplinary classification of lung adenocarcinoma. J Thorac Oncol 2011;6:244-85. [Crossref] [PubMed]
- Mamdani H, Ahmed S, Armstrong S, et al. Blood-based tumor biomarkers in lung cancer for detection and treatment. Transl Lung Cancer Res 2017;6:648-60. [Crossref] [PubMed]
- Babalola O, Muskrat J, Kanchustambham V. Diffuse Idiopathic Pulmonary Neuroendocrine Cell Hyperplasia (DIPNECH) Progressing to Carcinoid Tumor: A Case of Chronic Cough. Cureus 2023;15:e46659. [Crossref] [PubMed]
- Yang Y, Xu M, Huang H, et al. Serum carcinoembryonic antigen elevation in benign lung diseases. Sci Rep 2021;11:19044. [Crossref] [PubMed]
- Herbst RS, Morgensztern D, Boshoff C. The biology and management of non-small cell lung cancer. Nature 2018;553:446-54. [Crossref] [PubMed]
- Field JK, Oudkerk M, Pedersen JH, et al. Prospects for population screening and diagnosis of lung cancer. Lancet 2013;382:732-41. [Crossref] [PubMed]
- Tomasetti C, Marchionni L, Nowak MA, et al. Only three driver gene mutations are required for the development of lung and colorectal cancers. Proc Natl Acad Sci U S A 2015;112:118-23. [Crossref] [PubMed]
- Hanley JA, McNeil BJ. A method of comparing the areas under receiver operating characteristic curves derived from the same cases. Radiology 1983;148:839-43. [Crossref] [PubMed]
- Fedarko NS, Jain A, Karadag A, et al. Elevated serum bone sialoprotein and osteopontin in colon, breast, prostate, and lung cancer. Clin Cancer Res 2001;7:4060-6. [PubMed]
- Florkowski CM. Sensitivity, specificity, receiver-operating characteristic (ROC) curves and likelihood ratios: communicating the performance of diagnostic tests. Clin Biochem Rev 2008;29:S83-7. [PubMed]
- Sonego P, Kocsor A, Pongor S. ROC analysis: applications to the classification of biological sequences and 3D structures. Brief Bioinform 2008;9:198-209. [Crossref] [PubMed]
- Nagasaka M, Uddin MH, Al-Hallak MN, et al. Liquid biopsy for therapy monitoring in early-stage non-small cell lung cancer. Mol Cancer 2021;20:82. [Crossref] [PubMed]
- Niho S, Shinkai T. Tumor markers in lung cancer. Gan To Kagaku Ryoho 2001;28:2089-93. [PubMed]
- Sua LF, Serrano-Gomez SJ, Nuñez M, et al. Diagnostic potential of protein serum biomarkers for distinguishing small and non-small cell lung cancer in patients with suspicious lung lesions. Biomarkers 2024;29:315-23. [Crossref] [PubMed]
- Bai L, Huo R, Fang G, et al. MMP11 is associated with the immune response and immune microenvironment in EGFR-mutant lung adenocarcinoma. Front Oncol 2023;13:1055122. [Crossref] [PubMed]
- Zhang X, Huang S, Guo J, et al. Insights into the distinct roles of MMP-11 in tumor biology and future therapeutics Int J Oncol 2016;48:1783-93. (Review). [Crossref] [PubMed]
- Yuan Z, Li Y, Zhang S, et al. Extracellular matrix remodeling in tumor progression and immune escape: from mechanisms to treatments. Mol Cancer 2023;22:48. [Crossref] [PubMed]
- Dal Bello MG, Filiberti RA, Alama A, et al. The role of CEA, CYFRA21-1 and NSE in monitoring tumor response to Nivolumab in advanced non-small cell lung cancer (NSCLC) patients. J Transl Med 2019;17:74. [Crossref] [PubMed]
- Holdenrieder S, von Pawel J, Dankelmann E, et al. Nucleosomes, ProGRP, NSE, CYFRA 21-1, and CEA in monitoring first-line chemotherapy of small cell lung cancer. Clin Cancer Res 2008;14:7813-21. [Crossref] [PubMed]
- Yuan J, Sun Y, Wang K, et al. Development and validation of reassigned CEA, CYFRA21-1 and NSE-based models for lung cancer diagnosis and prognosis prediction. BMC Cancer 2022;22:686. [Crossref] [PubMed]