Development and validation of machine learning models based on molecular features for estimating the probability of multiple primary lung carcinoma versus intrapulmonary metastasis in patients presenting multiple non-small cell lung cancers
Highlight box
Key findings
• Machine learning (ML) models can accurately discriminate between multiple non-small cell lung cancers (NSCLCs).
• Multiple primary lung cancers (MPLCs) identified by ML models showed prolonged disease-free survival than that of intrapulmonary metastasis (IPM) identified by ML models.
What is known and what is new?
• Multiple NSCLCs have been recognized for over 50 years and show an increasing prevalence as the lung cancer screening developing. Distinguishing multiple NSCLCs as either MPLC or IPM is fundamental for tumor staging but remains a clinical dilemma.
• This study suggests that ML models could improve the accuracy of discrimination for multiple NSCLCs, particularly in challenging patients whose tumors had overlapped features in histology, histolohic morphologic appearence and driver mutations.
What is the implication, and what should change now?
• Current results warrant further validation in multicenter, prospective studies with large sample sizes.
Introduction
Lung cancer is one of the most frequent and deadly cancers in the world, with an annual incidence of approximately 2.2 million cases and almost 1.8 million deaths (1). Non-small cell lung cancer (NSCLC) accounts to almost 85% of lung cancer diagnoses and 70% of cases present with locally advanced or metastatic disease (2). Due to the recommendation of low-dose computed tomography (CT) scans for lung cancer screening, NSCLC with multiple pulmonary sites of involvement has become a growing concern (3-5). Multiple NSCLCs represent either separate independent primary tumors [multiple primary lung cancers (MPLCs)] or intrapulmonary metastases (IPMs). Identifying them is crucial for patient staging and adjuvant treatment decision. IPM tends to result in higher staging and worse prognosis than MPLC. The 8th edition of the tumor, node and metastasis (TNM) staging manual proposed by the International Association for the Study of Lung Cancer (IASLC) suggests T3 (same lobe), T4 (different lobes) and M1a (contralateral lung) for IPM and a separate cTNM and pTNM stage for each tumor of MPLC (6). Accordingly, the adjuvant treatment strategy for IPM should follow the principles for treating T3/T4/M1a NSCLC, whereas for MPLC with small tumors (no more than T2a staging) free of nodal or distant metastases, adjuvant treatment is unnecessary. However, the distinction of MPLC versus IPM remains challenging.
The Martini and Melamed (MM) criteria and the American College of Chest Physicians (ACCP) clinical guideline rely on clinicopathologic features to differentiate MPLC from IPM (7-10). Tumors with different histology, distinct locations, metachronous lesions diagnosed with more than 2 years interval, absence of lymph nodal or systemic metastasis are suggested to be treated as separated tumors. Comprehensive histologic assessment further improves the MPLC identification when tumors have same lung adenocarcinoma (ADC) histology but definitive distinct histological subtype (11). Recent advances in next-generation sequencing (NGS) technology offers a promising way to discriminate multiple NSCLCs by the analysis of molecular markers (4,12). Distinct patterns of driver mutations can differentiate MPLC from IPM. However, separate tumors could coincidentally harbor the same common driver mutation. IASLC recommends a multidisciplinary tumor board to consider all available information for a definitive diagnosis due to the complexity for the discrimination task (13). However, the discrimination of cases sharing the same histology with similar histologic appearance and common driver mutation patterns, without nodal or systemic metastases remains a clinical dilemma.
Cancer is, in essence, a genetic disease, and somatic mutations are present in most cancer genomes (14). NSCLC exhibits high-level genetic alterations, with multiple driver mutations commonly observed (15,16). Evolutionary view of cancer holds that metastases typically arise from the dissemination of subclones from the primary tumor, whereas separate primary tumors develop and evolve independently (17,18). Genetic heterogeneity and diversity evaluation among multiple NSCLCs provide an opportunity to delineate tumor clonal relationships (17,19). Different from traditional diagnostic methods, machine learning (ML) utilizes artificial intelligence to generate predictive and diagnosis models efficiently (20). Accumulated studies have applied ML methods to predict treatment responses and prognosis in lung cancers (21,22).
In this study, we performed whole-exome sequencing (WES) analysis for 157 resected tumor samples obtained from 72 multiple NSCLCs patients, and calculated four molecular features which were associated with multiple tumors relatedness. Based on the distinct molecular features between MPLC and IPM, we aimed to investigate and establish ML-based diagnostic models for the discrimination of MPLC and IPM. We hope to provide a new method to discriminate patients with multiple NSCLCs more accurately in clinical settings. We present this article in accordance with the TRIPOD reporting checklist (available at https://tlcr.amegroups.com/article/view/10.21037/tlcr-24-875/rc).
Methods
Study design and participants
This retrospective study included multiple NSCLCs patients from January 2012 to January 2018 from two Chinese hospitals (West China Hospital of Sichuan University; Sichuan Cancer Hospital). Patients were eligible if they had synchronous or metachronous multiple NSCLC lesions, and underwent surgical resection of multiple lesions. Patients whose paired multiple tumors were unavailable for WES, with evidence of non-ADC and non-squamous cell carcinoma (SCC) histology, extra-thoracic metastases, suspected lung metastasis of cancers other than lung cancer and those who received neoadjuvant therapies were excluded. All samples had to pass standard quality control measures.
A total of 157 surgically resected tumors from 72 multiple NSCLC patients underwent surgical resections were finally analyzed. We divided these patients into model developing and test cohort. To define the tumors, we followed the clinical and pathologic criteria proposed by IASLC (13). Tumors have different histologic types [e.g., SCC and ADC], arise from carcinoma in situ (e.g., atypical adenomatous hyperplasia (AAH), adenocarcinoma in situ (AIS), or minimally invasive adenocarcinoma (MIA) were considered definitive separate tumors. Additionally, recent studies have illustrate that tumors with distinct driver mutation patterns based on broad-panel NGS refer to the definitive separate tumors (4). When tumors meet all the following criteria simultaneously: matching histologic type [invasive adenocarcinoma (IAC), or SCC] and histologic subtyping appearance, same driver mutations pattern, with significant nodal or systemic metastases, they are considered definitive IPM. Therefore, patients with definitive MPLC (n=36) or IPM (n=10) were assigned for model developing.
On the other hand, for tumors that shared the same histologic type (e.g., multiple SCC or ADC) and similar histologic appearence, exhibited an uninformative pattern of driver mutations (e.g., absent driver mutations across all tumors, or common driver alterations shared by all lesions), and lacked nodal or systemic metastases, distinguishing them was challenging. These patients (n=26) were assigned to the test cohort. The participant flow diagram is illustrated in Figure 1, and the study design is showed in Figure 2.

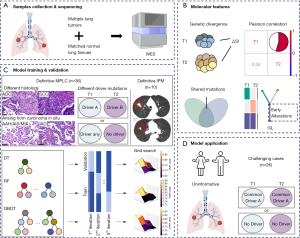
The study was conducted in accordance with the Declaration of Helsinki and its subsequent amendments. The study was approved by the Ethics Committee of West China Hospital of Sichuan University (No. 2017-SHEN-399), the leading center. As all data were de-identified and no additional interventions were involved, our collaborating institution, Sichuan Cancer Hospital, accepted this ethical review, and separate Institutional Review Board approval was deemed unnecessary. Individual consent for this retrospective analysis was waived.
Clinical data collection
Demographic information and clinical data for all cases were obtained by reviewing patient medical records. Selected epidemiologic characteristics included age and sex of the patients. smoking data, family history of tumor, dual tumors (except for lung cancer), number of lung lesions, tumor lesion’s location (contralateral, ipsilateral but different lobe, same lobe), histology, adjuvant treatment received were also reviewed. Radiological manifestation was recorded as pure ground-glass nodule (GGN), part-solid nodule (PSN), and pure solid nodule (SN) as previously reported. We also included information regarding the occurrence of multiple pulmonary lesions, specifying whether they were synchronous or metachronous. Additionally, the surgical resecrtion procedure preformed on each lesion, including lobectomy, segmentectomy, or wedge resection, were also recorded. Patients was followed up with chest and abdominal CT every 4–6 months and brain magnetic resonance imaging (MRI) every 6–12 months after surgery. Disease-free survival (DFS) was defined as the time from the last surgery to the first recurrence/metastasis or death from any cause, which was obtained as of November 2021 by using radiological follow-up and hospital visit data.
Histopathologic evaluation
In accordance with the initial pathological diagnosis, two expert pulmonary pathologists performed a blind review of pathological slides containing tumors. The histology and subtyping of lung adenocarcinoma (LUAD) in each tumor resection specimen were carried out following the guidelines provided by the IASLC and World Health Organization (WHO) (13,23).
Sample selection, DNA extraction and sequencing
Hematoxylin and eosin (H&E)-stained slides were reviewed by two pathologists to select the optimal formalin-fixed, paraffin embedded (FFEP) blocks for WES. Tumors with scant tissue, <10% tumor purity, or extensive necrosis were excluded. When multiple sections of the tumor were available, the section with the greatest viable tumor cellularity was selected. The matched normal lung tissues were used as the source for germline DNA control. Genomic DNA was extracted from all included tumor samples and paired normal tissues using blackPREP FFPE DNA Kit (Analytik Jena, Jena, Germany) according to the manufacturer’s instructions. Briefly, sequencing libraries were prepared using the KAPA Hyper Prep Kit (Illumina platforms) (KAPA Biosystems, Massachusetts, USA). Adapter ligated DNA was hybridized to SeqCap EZ MedExome Probes (Roche, Pleasanton, USA) covering 47 Mb of the genome using a SeqCap EZ MedExome Target Enrichment kit (Roche) according to the manufacturers’ protocols. The captured DNA libraries were sequenced on an Illumina Xten sequencing system with a paired-end 150 bp read length.
Variants calling and filtering
Sequencing reads were processed following GATK best practices (24) using an in-house pipeline that contained Trimmomatic (v0.36) for reads adapter trimming and quality filtering, BWA (0.7.12) for mapping reads to the hg19 reference genome, and Genome Analysis ToolKit (version 4.0) for sorting, marking duplicates and base quality score recalibration. For somatic variants calling of each tumor sample, we used MuTect2 to detect single nucleotide variants (SNVs) and small InDels with matched tumor-normal sample pairs. HaplotypeCaller was used to call germline SNVs and InDels for each sample. After annotation of these variants by ANNOVAR (Annotate Variation) (25), we filtered out variants either in introns or synonymous SNVs. To obtain the pathogenic germline variants, we used CharGer (26) to evaluate exonic nonsynonymous and selected variants labeled pathogenic or likely pathogenic (P/LP).
Genetic divergence analysis
We utilized the Shannon Index (SI) (27) as a measure of somatic mutational diversity. As follows:
in which pi is the max variant allele frequency (VAF) of somatic mutated genes i in the tumor sample. We firstly calculated the SI of each tumor using the max VAF of mutated genes by R vegan package. Then, we merged somatic SNV/InDels of each pair of tumors as a mixed tumor sample to calculate its SI for one patient. Next, the difference in SI between the mixed sample and each of its component tumors were calculated. The average SI difference was used to estimate the relationship between two component tumors. The lower the average SI difference was, the greater the probability that they were from a single tumor source. The minimum SI difference of tumor pairs in one patient, marked as ΔSI, was treated as the final inter-tumor genetic divergence feature. Mathematically, the minimum pairwise genetic diversity index (ΔSI) is defined as follows:
in which is the SI of merged sample i and j. and represented the SI of sample i and sample j, respectively.
Shared mutations analysis
Based on the filtered somatic variants, variants with VAF ≥8% were selected for sharing variants counting. Somatic variants sharing between tumor pairs of patients were counted. The greatest number in one patient was treated as the final sharing variants count. For each tumor pair in one patient, union variants were counted as well as the sharing variants.
Inter-sample correlation analysis
Genomic aberrations in each sample were used to construct a similarity matrix for calculating the inter-sample Pearson correlation coefficient for each patient. The maximum correlation in individual was selected as the correlation score of the patient.
Phylogenetic and clonality analysis
Based on the filtered nonsynonymous somatic SNVs and their associated VAFs within each sample, we applied the LICHeE (28) method for the reconstruction of multi-sample tumor phylogenies and tumor subclone decomposition. The early mutations number was selected as the trunk count of the patient.
ML models establishment
We employed three distinct supervised ML algorithms, decision trees (DT), random forests (RF), and gradient boosting decision trees (GBDT), to develop the discrimination model for multiple NSCLCs. The model was constructed using the Python package scikit-learn version 0.17.2. To ensure robust model training and evaluate the model’s generalizability, patients in developing cohort were spilt into training and validation sets using stratified random sampling, with 80% of the data allocated to training and 20% to validation. Additionally, we implemented stratified 5-fold cross-validation on these sets. The input features for the ML model comprised selected molecular markers relevant to multiple NSCLCs diagnosis: genetic divergence, shared mutation number, Pearson correlation coefficient and early mutation number. We utilized grid search to identify the optimal settings for hyperparameters such as max_depth, n_estimators, and learning_rate, aiming for the best model performance. Models performance were assessed by areas under the curve (AUCs), accuracy, precision, recall, and F1 score in validation set. Finally, the established ML models were applied to illustrate the relationships among undetermined multiple NSCLC tumors. Tumors relationship in test cohort were also assessed according to ACCP criteria. DFS comparsions between groups were used to evaluate the performance of ML models and ACCP criteria.
Statistical analysis
Patient demographic and clinical characteristics were summarized as continuous or categorical variables. Continuous variables were compared between groups using the Mann-Whitney U-test. The frequency between groups were tested using the Chi-squared test or Fisher’s exact test. Models performance metrics in internal validation set were reported as mean ± standard deviation (SD). DFS analyses were performed according to the Kaplan-Meier method, and groups were compared with the log-rank test. A two-sided P value of <0.05 was considered statistically significant. Figures plotting and statistical analyses were performed using R packages, version 4.3.2 and GraphPad Prism 8.0.2.
Results
Patient characteristics
In total, 72 patients were included in this study (Figure 1). In the development cohort, 36 patients had definitively MPLC tumors: tumor pairs in one patients had different histology, tumor pairs in two patients had different histology, six patients had lesion(s) with AAH, AIS or MIA, and 27 patients had multiple IAC whose tumors presented different driver mutation patterns (Table S1). Ten patients in development cohort were definitive IPM patients whose tumors shared a matching histological appearance, SNs on radiology, and driver mutation patterns. Three of them were sequenced in primary lung tumor and corresponding visceral pleural metastatic lesions (Table S2). Patients in test cohort were those whose tumors had a matching IAC with similar histological subtypes or SCC histology, and the uninformative driver mutation patterns (Table S3). The study design is illustrated in Figure 2. Mutiple lung tumors and their corresponding normal lung tissues were collected and subjected to WES (Figure 2A). Based on WES data, four moleculer features were analyzed: genetic divergence, Pearson correlation, shared mutations and early mutations (Figure 2B). Patients with definitive MPLC and IPM were included in the ML model development cohort, where three ML algorithms were used to develop predictive models based on these four molecular features (Figure 2C). The trained ML models were subsequently applied to patients in the test cohort with undetermined multiple lung tumors for classification (Figure 2D). Demographics and clinical characteristics are summarized in Table 1.
Table 1
Characteristics | Model development cohort | Model test cohort | |
---|---|---|---|
MPLC | IPM | ||
Number of patients | 36 | 10 | 26 |
Age (years) | 63 (42–74) | 54.5 (43–71) | 61.5 (44–79) |
Female | 17 [47] | 5 [50] | 10 [38] |
Smoking history | |||
Never | 25 [70] | 6 [60] | 13 [50] |
Ever | 11 [30] | 4 [40] | 13 [50] |
Familial history of cancer | |||
Yes | 18 [50] | 1 [10] | 7 [27] |
No | 18 [50] | 9 [90] | 19 [73] |
Dual tumor history | |||
Yes | 3 [8] | 0 [0] | 2 [8] |
No | 33 [82] | 10 [100] | 24 [82] |
No. of lesions | |||
2 | 31 [86] | 5 [50] | 24 [92] |
3 | 5 [14] | 4 [40] | 2 [8] |
≥4 | 0 [0] | 1 [10] | 0 [0] |
Time course | |||
Synchronous | 34 [95] | 10 [100] | 23 [88] |
Metachronous | 2 [5] | 0 [0] | 3 [12] |
Histologic features | |||
ADC + ADC | 35 [97] | 10 [100] | 23 [89] |
ADC + SCC | 1 [3] | 0 [0] | 0 [0] |
SCC + SCC | 0 [0] | 0 [0] | 3 [11] |
Lymphatic metastasis | |||
Yes | 0 [0] | 5 [50] | 0 [0] |
No | 36 [100] | 5 [50] | 26 [100] |
Data are presented as n [%] or median (range). ADC, adenocarcinoma; IPM, intrapulmonary metastasis; MPLC, multiple primary lung cancer; SCC, squamous cell carcinoma.
Among the 36 MPLC patients, 18 patients had a family history of cancer, with lung cancer being the most common cancer type (11 patients). None of these patients had nodal and distinct metastasis. All IPM patients in developing cohort underwent curative-intent resection at the time of surgery due to the absence of extra thoracic metastasis evidence, with seven of them had pathologically pleural metastasis, while five patients had lymph node metastasis, and three patients were diagnosed with brain metastasis shortly after surgery.
Molecular features of MPLC and IPM in the development cohort
To characterize inter-tumor genetic divergence, we applied the diversity measures from ecology and evolution science to our somatic mutation data obtained through WES. We calculated the minimum pairwise genetic divergence index (ΔSI) for each patient. As shown in Figure 3A, IPM patients exhibited lower inter-tumor genetic divergence, with a median of 0.39 (ranged from 0.08 to 0.48), compared to MPLC patients (median 0.67, range from 0.55 to 0.78, P<0.001).
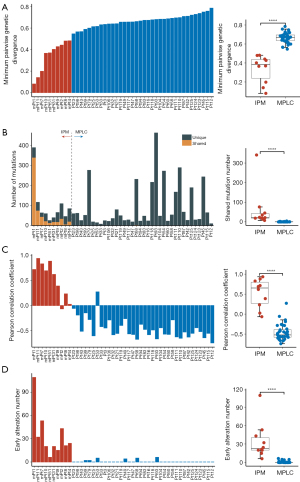
Next, we analyzed the somatic mutations and structural variants to determine the clonal relationships between tumor pairs. Genetic alterations were classified as either shared (present in all tumors) or unique (present in only one tumor) for each patient. We observed a significantly lower shared mutations counts in MPLC patients compared to IPM patients (P<0.001; Figure 3B). In the MPLC group, 25 tumor pairs (69%) exhibited no overlapping somatic mutations, 5 tumor pairs shared only one somatic mutation, and 6 tumor pairs shared multiple (≥2) somatic mutations (median 2, up to 4). In contrast, all tumor pairs of IPM shared multiple somatic alterations (median 22, range from 7 to 341). When comparing the number of unique mutations, the MPLC group (median 47, range from 7 to 463) and the IPM group (median 39, range from 11 to 74) showed comparable numbers (P=0.40).
We then performed Pearson correlation analysis to depict the mutational similarity among multiple tumors in each patient. The result indicated that lesions in MPLC patients had limited relatedness, with a median Pearson correlation coefficient of −0.52 (range from −0.74 to 0.27), compared to IPM patients (median 0.65, range from −0.06 to 0.93, P<0.001; Figure 3C). Detailed Pearson correlation information for MPLC and IPM tumors is provided in Figures S1,S2, respectively.
Moreover, we constructed phylogenetic trees to estimate the ancestral relationship among multiple tumors. We classified the somatic mutations as early mutations (trunk) and late mutations (branch). The presence of more early mutations suggests a higher probability that tumors arose from a common origin. We quantified the number of early mutations for each individual and found that all IPM tumor pairs exhibited significantly higher levels than the MPLC population (P<0.001; Figure 3D). Specifically, IPM patients had at least 6 early mutations (median 22.5, up to 109). In contrast, as much as 30 MPLC tumor pairs (83%) showed no early mutations, and only 6 patients had multiple early mutations (≥2, up to 6).
ML models development
We developed machine ML classification models to differentiate between MPLC and IPM using four molecular characteristics: genetic divergence, shared mutation number, Pearson correlation coefficient, and early alteration number. The development cohort was subjected to five-fold cross-validation to ensure robust training and validation. As illustrated in Table 2, performance metrics such as the AUC, accuracy, F1 score, recall, and precision were calculated to evaluate the models. The DT model achieved a mean AUC of 0.94 (SD 0.09) (Figure 4A), mean accuracy of 0.9556 (SD 0.0544), mean F1 score of 0.9712 (SD 0.0353), mean recall of 0.9714 (SD 0.0571), and mean precision of 0.9750 (SD 0.0500). The RF model demonstrated a mean AUC of 1.00 (SD 0.00) (Figure 4B), mean accuracy of 0.9778 (SD 0.0444), mean F1 score of 0.9846 (SD 0.0308), mean recall of 0.9714 (SD 0.0571), and mean precision of 1.0000 (SD 0.0000). The GBDT model showed a mean AUC of 1.0000 (SD 0.0000) (Figure 4C), mean accuracy of 0.9800 (SD 0.0400), mean F1 score of 0.9882 (SD 0.0235), mean recall of 1.0000 (SD 0.0000), and mean precision of 0.9778 (SD 0.0445). Schematic diagrams of the ML models and the corresponding model weight files are included as Appendix 1 to facilitate testing on independent datasets.
Table 2
Method | Accuracy | F1 | Recall | Precision |
---|---|---|---|---|
DT | 0.9556±0.0544 | 0.9712±0.0353 | 0.9714±0.0571 | 0.9750±0.0500 |
RF | 0.9778±0.0444 | 0.9846±0.0308 | 0.9714±0.0571 | 1.0000±0.0000 |
GBDT | 0.9800±0.0400 | 0.9882±0.0235 | 1.0000±0.0000 | 0.9778±0.0445 |
Data are presented as mean ± standard deviation. DT, decision trees; GBDT, gradient boosting decision trees; ML, machine learning; RF, random forests.
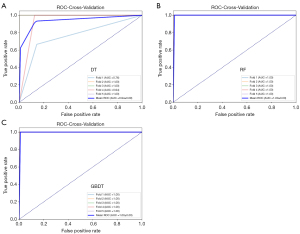
ML models validation
In the test cohort, RF, DT, and GBDT models, which were applied using the models constructed in the previous phase, yielded the same classification results. Fifteen patients were identified as MPLC, and 11 patients were considered as IPM. MPLC in this set still exhibited a higher inter-tumor genetic divergence (median 0.67 vs. 0.39, P<0.001; Figure 5A), less shared mutations (median 1 vs. 26, P<0.001; Figure 5B), lower inter-tumor correlation (median −0.44 vs. 0.57, P<0.001; Figure 5C, Figure S3) and fewer early alterations (median 0 vs. 35, P<0.001; Figure 5D) than IPM patients.
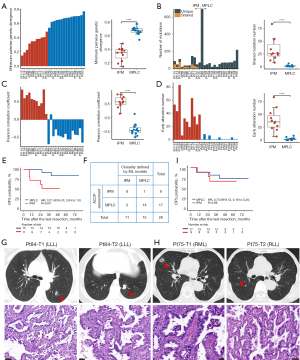
Since MPLC and IPM exhibit distinct clinical outcomes because of the nature of disease, we conducted the comparison of DFS between them. Indeed, MPLC patients identified by ML models in this cohort showed a significant DFS benefit compared to IPM patients [hazard ratio (HR) =0.21; 95% confidence interval (CI): 0.04–1.0; P=0.04; Figure 5E]. We also conducted the assessment for the same population according to ACCP criteria (10). The ACCP assessment classified 17 tumor pairs as MPLC and 9 tumor pairs as IPM. This assessment was consistent with the identification by ML models in 85% of the cases (Figure 5F). One patient (Pt84) was identified as having MPLC by ML models but classified as having IPM by ACCP criteria, whose synchronous tumors located in the same lung lobe showed an overlapping EGFR L858R driver mutation, histologic morphologic feature characterized by acinar patterns, and radiologic appearance of SN and PSN lesions (Figure 5G). Three patients (Pt75, Pt109 and Pt120) were identified as having IPM by ML models but classified as having MPLC by ACCP criteria. As the representative case shown in Figure 5H, tumors from Pt75 located in different lung lobes showed a sharing driver EGFR 20insert alteration, histologic appearance of acinar and papillary patterns, and PSN lesions in CT images. However, there was no significant difference in DFS between groups stratificated by ACCP criteria assessment (P=0.68; Figure 5I).
Clinical comparation of MPLC and IPM patients
We next merged the model development and test cohort and compared the clinical characteristics between ML models defined MPLC and IPM patients of the whole populations in this study. As shown in Table 3, seven out of 51 MPLC patients had dual cancers. Twenty-two (43%) with MPLC reported a family history of cancer among their first-degree relatives, with 55% (12 out of 22) reporting a family history of lung cancer. Family history of cancer and relevant clinical information are listed in Table S4. Most IPM patients received the adjuvant therapy (18 out of 21 patients, 85.7%), while less MPLC patients were treated with adjuvant therapy (33.3%) (P<0.001). Considering that the lymph node metastasis, pleural metastases and distant metastasis always lead to worse survival, we compared DFS between MPLC (n=51) and IPM (n=7) whose tumors limited intrapulmonary. The result showed that MPLC patients still exhibited a significant prolonged DFS compared to IPM patients (HR =0.30; 95% CI: 0.07–1.35; P=0.02; Figure S4).
Table 3
Variables | MPLC | IPM | P value |
---|---|---|---|
No. of patients | 51 | 21 | |
Age at diagnosis (years) | 63 [42–80] | 59 [41–79] | 0.053 |
Sex | >0.99 | ||
Female | 23 (45.1) | 9 (42.9) | |
Male | 28 (54.9) | 12 (57.1) | |
Smoking status | 0.18 | ||
Ever | 17 (33.3) | 11 (52.4) | |
Never | 34 (66.7) | 10 (47.6) | |
Family history of tumor | 0.06 | ||
Yes | 22 (43.1) | 4 (19.0) | |
No | 29 (56.9) | 17 (81.0) | |
Dual tumor | 0.10 | ||
Yes | 7 (13.7) | 0 (0.0) | |
No | 44 (86.3) | 21 (100.0) | |
Adjuvant therapy | <0.001 | ||
Yes | 17 (33.3) | 18 (85.7) | |
None | 34 (66.7) | 3 (14.3) |
Data are presented as n (%) or median [range]. IPM, intrapulmonary metastasis; MPLC, multiple primary lung cancer.
Characterization of somatic mutations and germline mutations
Next, we analyzed the somatic and germline mutations characteristics among MPLC and IPM patients in this study. In total, 10,522 potentially functional somatic mutations in 6,470 genes for 157 tumor samples were identified, with mean number of 1.6 somatic mutations per gene. The median number of somatic mutations carried by tumor sample was 53, ranging from 17 to 393. The highest mutated genes in 10 oncogenic pathways (29) included EGFR (87 out of 157 tumor samples, 55%), TP53 (31%) and KRAS (11%). For the gene EGFR, the somatic mutations tended to occur in L858R (53 out of 157 tumor samples, 34%) and Del19 (15%) (Figure 6A). Among the MPLC patients, it is noteworthy that 45% (23 out of 51 patients) harbored EGFR mutations in all lesions, with 65% (15 out of 23 patients) displaying distinct alterations between the lesions, and the remaining 35% (8 out of 23 patients) presenting the same alterations (Figure 6B). Most somatic subtype mutations among the MPLC samples were L858R (37 out of 63 samples, 59%), followed by exon 19 deletions (25%) (Figure 6C). MPLC had lower tumor mutation burden (TMB; median 0.73, range, 0.03–9.67) than IPM (median 1.21, range, 0.58–3.03) (P=0.005, Figure 6D). To characterize the TMB change between paired tumors, we depict the TMB varible among tumor pairs (Figure 6E) and calculated the TMB fold-change (FC; the ratio of maximum to minimum) for each participant. MPLC displayed comparable TMB FC with IPM (median 1.72 vs. 1.22, P=0.07, not shown).
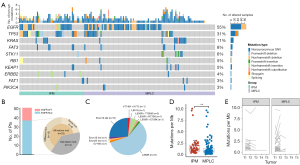
We next explored the germline mutations characterize for these multiple NSCLCs patients. In the 72 patients, a total of 85 P/LP germline mutations in 74 genes carried by 49 patients (68%) were identified, with a median number of 1 (ranging from 0 to 6). Seven patients had dysfunctional germline mutations in FLG, including 2 mutation types, among which one was frameshift deletion and another was stopgain, suggesting the potential harmful effects of the mutations in this gene. Additionally, 4 germline mutations carried by 4 patients were identified in SERPINA1, whose defects were reported associating with chronic obstructive pulmonary disease and emphysema (Figure 7A). We compared the prevalence of P/LP germline alterations in MPLC patients versus IPM patients and found that there was no significant difference (37/51 vs. 9/21, P=0.27, Figure 7B). To investigate whether MPLC patients with P/LP germline mutations had distinct clinical features compared to those without P/LP germline mutations, the prevalence of family cancer history and age at diagnosis were analyzed and found to be comparable between the two groups (Figure 7C,7D). A trend was observed that P/LP germline mutations were more common in females than in males under 50 years of age (Figure 7E) for MPLC patients. Logistic regression analysis indicated that a higher germline P/LP mutation rate tended to be associated with family cancer history but was not correlated with sex, age at diagnosis, smoking status, or dual tumors (Table S5).
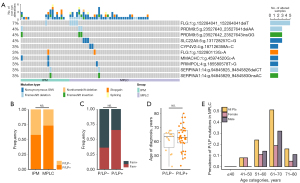
Discussion
The current study applied the novel ML models to determine tumor relatedness in patients with multiple NSCLCs. The highlighted findings of this study are as follows. First, this is the first study to score the genetic divergence among NSCLCs, providing each patient with a precise molecular information. Second, the ML models demonstrated robust performance in discriminating multiple lung lesions. The DFS advantage observed in MPLC patients identified by ML models further supports this classification. Furthermore, our data reveal the clinical and genetic features of the MPLC and IPM population, which may aid in clinical management.
Determining whether multiple NSCLCs represent MPLC or IPM has been a long-standing clinicopathologic dilemma. Traditional clinicopathological method is definitive when tumors have distinct histology, harbor distinct driver mutations, and develop lymph nodule or systemic metastases. When multiple NSCLCs share an overlapping in histology, driver mutations and free of extrapulmonary metastasis, clinicopathologic method is not sufficient to solve these challenging cases. Recent advances in genomic testing in clinical practice allow the genetic methods to be reliable and powerful tool to distinguish tumor relatedness among multiple NSCLCs (30). Unlike previous panels of NGS data, WES allows for a more in-depth understanding of genetic characteristics at the whole exome level. We first applied the ecological and evolutionary index SI to evaluate the multiple lung cancer relatedness in this study. SI is a robust indicator of diversity in a population that accounts for both the number and the relative abundance of clones. Several tumor-related studies have adopted SI to evaluate clonality or sample heterogeneity (31-33). A high SI indicates high mutation diversity in the tumor regions, while a low SI indicates the opposite. This method utilizes the depth and coverage of WES data and comprehensively measures the genetic divergence between tumors using information theory ΔSI, without relying on specific molecular markers. Chang et al. have explored the utility of shared mutation based on the broad-panel NGS for classifying multiple NSCLCs (12). Pearson correlation analysis and phylogeny relationship have also been used to characterize the clonal relationship between tumors (17,19,34). In this study, these four indices exhibited significant difference between MPLC and IPM, which illustrated their reliability in classifying multiple NSCLCs.
Recent advancement in ML algorithms and models are significantly enhancing the diagnosis, treatment, and prognosis of lung cancer (35-40). ML models, particularly those combining radiomic and epidemiological features, show great promise in predicting malignancy risk in indeterminate pulmonary nodules and aiding early-stage detection (41). Multimodal approaches, such as using imaging biomarkers and deep learning techniques on histological images, are being explored to predict gene mutation, invasiveness, and treatment responses, especially in NSCLC patients treated with immunotherapy (42-49). Additionally, artificial intelligence-driven methods, including deep learning for mutation prediction and survival, are poised to improve clinical decision-making (50-52). Several studies have also demonstrated the application of ML in classifying multiple NSCLCs (53,54). For example, Pei et al. reported an RF model based on a broad panel with 808 cancer-related genes to classify multiple NSCLCs, the AUC of this model is 0.947 (53). However, it used ACCP guideline as the golden criteria to differentiate MPLC and IPM which could make mistakes in challenging cases. In current study, we used clearly separate tumors and related tumors, and introduced the DT, GBDT and RF algorithm into diagnostic research in patients with multiple NSCLCs using four selected molecular features to develop ML models, and AUC of these models are 0.94, 1.00 and 1.00, respectively. Except for IPM patients in development cohort, the major population of this study is patients with surgically resected multi-NSCLC without lymph nodules or distant metastases, and each tumor had a T stage no further than T2a. Most of IPM patients in this study received adjuvant therapy, while less than half MPLC patients received adjuvant treatment. With a long follow-up time, MPLC patients identified by ML models still showed a prolonged IPM patients, which in some extent supported this classification.
We also conducted parallel assessment according to ACCP guideline in the same population, but the results failed to show a DFS difference. Limitations of traditional clinicopathologic criteria have been previously reported and also observed in this study, such as interobserver disagreement when the paired tumors present the same histology, time interval and anatomic location of tumors (4,12,55). Clinicpathologic characteristics reported previously are always suggestive rather than decisive. Suh et al. reported that at least one lesion presenting with pure GGN or GGN-predominant PSN among multiple NSCLCs suggested MPLC (56). Imaging presentation of a disease is not determined by the tumor growth pattern alone; it is also influenced by complex microenvironment factors (57,58). IPM patients in current study also showed a GGN lesion among the paired tumors. The surgical procedure may be a important variable for evaluating the possibility of metastasis for metachronous tumors, as different resection techniques have varying impacts on tumor clearance and potential for residual disease (59,60). However, only 5 patients was confirmd to have metachronous tumors in our study. All 5 patients underwent lobectomy as their first surgical procedure. Due to this small sample size and the uniformity in surgical approach, our study was unable to provide additional insights into the impact of the type of surgical resection on evaluating tumor metastasis. Further analysis or stratification by surgical technique with a larger cohort may be considered for future studies.
One important observation in our study is the high frequency of family cancer history, particularly lung cancer, in MPLC population. This finding suggests the possibility of inherited susceptibility or a familial component in the development of MPLC. EGFR is the most common driver in the current study. We also found a high occurrence of multiple lesions in MPLC patients harboring the same EGFR driver (45%), let alone the consistency of specific EGFR mutations among lesions. Hu et al. recently reported that MPLC is driven by different molecular events and often exhibit a low TMB (61). Our results are consistent with this finding, as we observed a lower TMB in MPLC, possibly due to the lower prevalence of smoking among this population. Environmental risk factors, such as tobacco exposure, second-hand smoke, dust, asbestos are well-established risk factors for lung cancer (62). However, due to the retrospective nature of our data collection, we could not obtain detailed information on these factors. Our study also observed TP53 alteration differences in lesions of IPM, although TP53 aberrations have long been recognized to occur in the early stage of lung cancer (63). In fact, we reported previously a LUAD patient whose primary lesion showed wide-type TP53, while its corresponding pleural lesions presented TP53 p.G245S mutation (64). Previous study has reported an increased frequency of germline alterations in patients with lung cancer with a family cancer history, early age at onset, or carrying a diagnosis of multiple cancers (65). Our study identified a wide spectrum of P/LP germline variants exclusively in MPLC or in IPM, but we did not find some shared germline P/LP alterations for MPLC population or IPM population. As proposed previously, tumor development depends on the complex interaction between germline variants, somatic mutations and environmental factors (66). Further investigation is needed to understand the role of these germline variants in the mechanism of multiple NSCLCs carcinogenesis. In addition to histologic evaluation and molecular alterations, biomarker expression at the protein level, assessed through immunohistochemistry (IHC), plays a crucial role in evaluating tumor relatedness and predicting treatment response (61,67,68). Consequently, the multimodal integration of radiology, pathology (including both histology and IHC), and genomics, combined with ML algorithms, holds great promise for enhancing the prediction of tumor behavior and optimizing adjuvant treatment strategies in patients with multiple lung tumors.
The limitations of the current study are as follows. First, potential bias may exist due to the limited sample size and the two-center retrospective study design. To ensure consistent surgical resection quality and tumor samples quality, we chose to include the radical resection samples in the two institutions. The promising results signify that a prospective study with a large sample size is imperative. Second, our models were tested using data from a single center. For an ML model, the test data from other centers are essential for examining generalization capacity. A multicenter study is warranted to further confirm our findings. Third, our model cannot be used in the clinical situations where patients with multiple lung cancer only undergo a biopsy rather than surgical resection. Fourth, our model should neither be used in patients who have received neoadjuvant treatment. Since molecular features calculated for each patients reflect the naive tumor heterogeneity and its component diversity, which could be affected by the pressure of neoadjuvant therapy.
Conclusions
We developed and evaluated ML algorithms to differentiate multiple NSCLC as either MPLC or IPM using molecular characteristics based on the WES. The strong performance of these ML models may assist in improving the accuracy of multiple NSCLCs diagnosis and clinical decision-making, particularly for challenging cases in clinical practice.
Acknowledgments
None.
Footnote
Reporting Checklist: The authors have completed the TRIPOD reporting checklist. Available at https://tlcr.amegroups.com/article/view/10.21037/tlcr-24-875/rc
Data Sharing Statement: Available at https://tlcr.amegroups.com/article/view/10.21037/tlcr-24-875/dss
Peer Review File: Available at https://tlcr.amegroups.com/article/view/10.21037/tlcr-24-875/prf
Funding: This work was supported by
Conflicts of Interest: All authors have completed the ICMJE uniform disclosure form (available at https://tlcr.amegroups.com/article/view/10.21037/tlcr-24-875/coif). F.X. and W.C. are currently employees of Genecast Biotechnology Co., Ltd. The other authors have no conflicts of interest to declare.
Ethical Statement: The authors are accountable for all aspects of the work in ensuring that questions related to the accuracy or integrity of any part of the work are appropriately investigated and resolved. The study was conducted in accordance with the Declaration of Helsinki and its subsequent amendments. The study was approved by the Ethics Committee of West China Hospital of Sichuan University (No. 2017-SHEN-399), the leading center. As all data were de-identified and no additional interventions were involved, our collaborating institution, Sichuan Cancer Hospital, accepted this ethical review, and separate Institutional Review Board approval was deemed unnecessary. Individual consent for this retrospective analysis was waived.
Open Access Statement: This is an Open Access article distributed in accordance with the Creative Commons Attribution-NonCommercial-NoDerivs 4.0 International License (CC BY-NC-ND 4.0), which permits the non-commercial replication and distribution of the article with the strict proviso that no changes or edits are made and the original work is properly cited (including links to both the formal publication through the relevant DOI and the license). See: https://creativecommons.org/licenses/by-nc-nd/4.0/.
References
- Sung H, Ferlay J, Siegel RL, et al. Global Cancer Statistics 2020: GLOBOCAN Estimates of Incidence and Mortality Worldwide for 36 Cancers in 185 Countries. CA Cancer J Clin 2021;71:209-49. [Crossref] [PubMed]
- Han J, Liu Y, Yang S, et al. MEK inhibitors for the treatment of non-small cell lung cancer. J Hematol Oncol 2021;14:1. [Crossref] [PubMed]
- Mascalchi M, Comin CE, Bertelli E, et al. Screen-detected multiple primary lung cancers in the ITALUNG trial. J Thorac Dis 2018;10:1058-66. [Crossref] [PubMed]
- Chang JC, Rekhtman N. Pathologic Assessment and Staging of Multiple Non-Small Cell Lung Carcinomas: A Paradigm Shift with the Emerging Role of Molecular Methods. Mod Pathol 2024;37:100453. [Crossref] [PubMed]
- Jensen SØ, Moore DA, Surani AA, et al. Second Primary Lung Cancer - An Emerging Issue in Lung Cancer Survivors. J Thorac Oncol 2024;19:1415-26. [Crossref] [PubMed]
- Chou TY, Dacic S, Wistuba I, et al. Differentiating Separate Primary Lung Adenocarcinomas From Intrapulmonary Metastases With Emphasis on Pathological and Molecular Considerations: Recommendations From the International Association for the Study of Lung Cancer Pathology Committee. J Thorac Oncol 2025;20:311-30. [Crossref] [PubMed]
- Martini N, Melamed MR. Multiple primary lung cancers. J Thorac Cardiovasc Surg 1975;70:606-12. [Crossref] [PubMed]
- Detterbeck FC, Jones DR, Kernstine KH, et al. Lung cancer. Special treatment issues. Chest 2003;123:244S-58S. [Crossref] [PubMed]
- Shen KR, Meyers BF, Larner JM, et al. Special treatment issues in lung cancer: ACCP evidence-based clinical practice guidelines (2nd edition). Chest 2007;132:290S-305S.
- Kozower BD, Larner JM, Detterbeck FC, et al. Special treatment issues in non-small cell lung cancer: Diagnosis and management of lung cancer, 3rd ed: American College of Chest Physicians evidence-based clinical practice guidelines. Chest 2013;143:e369S-99S.
- Mansuet-Lupo A, Barritault M, Alifano M, et al. Proposal for a Combined Histomolecular Algorithm to Distinguish Multiple Primary Adenocarcinomas from Intrapulmonary Metastasis in Patients with Multiple Lung Tumors. J Thorac Oncol 2019;14:844-56. [Crossref] [PubMed]
- Chang JC, Alex D, Bott M, et al. Comprehensive Next-Generation Sequencing Unambiguously Distinguishes Separate Primary Lung Carcinomas From Intrapulmonary Metastases: Comparison with Standard Histopathologic Approach. Clin Cancer Res 2019;25:7113-25. [Crossref] [PubMed]
- Detterbeck FC, Franklin WA, Nicholson AG, et al. The IASLC Lung Cancer Staging Project: Background Data and Proposed Criteria to Distinguish Separate Primary Lung Cancers from Metastatic Foci in Patients with Two Lung Tumors in the Forthcoming Eighth Edition of the TNM Classification for Lung Cancer. J Thorac Oncol 2016;11:651-65.
- Alexandrov LB, Nik-Zainal S, Wedge DC, et al. Signatures of mutational processes in human cancer. Nature 2013;500:415-21. [Crossref] [PubMed]
- Campbell JD, Alexandrov A, Kim J, et al. Distinct patterns of somatic genome alterations in lung adenocarcinomas and squamous cell carcinomas. Nat Genet 2016;48:607-16. [Crossref] [PubMed]
- Comprehensive molecular profiling of lung adenocarcinoma. Nature 2014;511:543-50. [Crossref] [PubMed]
- Liu Y, Zhang J, Li L, et al. Genomic heterogeneity of multiple synchronous lung cancer. Nat Commun 2016;7:13200. [Crossref] [PubMed]
- Furuta M, Ueno M, Fujimoto A, et al. Whole genome sequencing discriminates hepatocellular carcinoma with intrahepatic metastasis from multi-centric tumors. J Hepatol 2017;66:363-73. [Crossref] [PubMed]
- Ma P, Fu Y, Cai MC, et al. Simultaneous evolutionary expansion and constraint of genomic heterogeneity in multifocal lung cancer. Nat Commun 2017;8:823. [Crossref] [PubMed]
- Li Y, Wu X, Yang P, et al. Machine Learning for Lung Cancer Diagnosis, Treatment, and Prognosis. Genomics Proteomics Bioinformatics 2022;20:850-66. [Crossref] [PubMed]
- Tian D, Yan HJ, Huang H, et al. Machine Learning-Based Prognostic Model for Patients After Lung Transplantation. JAMA Netw Open 2023;6:e2312022. [Crossref] [PubMed]
- Moon I, LoPiccolo J, Baca SC, et al. Machine learning for genetics-based classification and treatment response prediction in cancer of unknown primary. Nat Med 2023;29:2057-67. [Crossref] [PubMed]
- Travis WD, Brambilla E, Nicholson AG, et al. The 2015 World Health Organization Classification of Lung Tumors: Impact of Genetic, Clinical and Radiologic Advances Since the 2004 Classification. J Thorac Oncol 2015;10:1243-60. [Crossref] [PubMed]
- Van der Auwera GA, Carneiro MO, Hartl C, et al. From FastQ data to high confidence variant calls: the Genome Analysis Toolkit best practices pipeline. Curr Protoc Bioinformatics 2013;43:11.10.1-11.10.33.
- Wang K, Li M, Hakonarson H. ANNOVAR: functional annotation of genetic variants from high-throughput sequencing data. Nucleic Acids Res 2010;38:e164. [Crossref] [PubMed]
- Scott AD, Huang KL, Weerasinghe A, et al. CharGer: clinical Characterization of Germline variants. Bioinformatics 2019;35:865-7. [Crossref] [PubMed]
- Magurran AE. Measuring biological diversity. Curr Biol 2021;31:R1174-7. [Crossref] [PubMed]
- Popic V, Salari R, Hajirasouliha I, et al. Fast and scalable inference of multi-sample cancer lineages. Genome Biol 2015;16:91. [Crossref] [PubMed]
- Sanchez-Vega F, Mina M, Armenia J, et al. Oncogenic Signaling Pathways in The Cancer Genome Atlas. Cell 2018;173:321-337.e10. [Crossref] [PubMed]
- Zhang X, Fan X, Sun C, et al. A novel NGS-based diagnostic algorithm for classifying multifocal lung adenocarcinomas in pN0M0 patients. J Pathol Clin Res 2023;9:108-20. [Crossref] [PubMed]
- Maley CC, Galipeau PC, Finley JC, et al. Genetic clonal diversity predicts progression to esophageal adenocarcinoma. Nat Genet 2006;38:468-73. [Crossref] [PubMed]
- Chung YR, Kim HJ, Kim YA, et al. Diversity index as a novel prognostic factor in breast cancer. Oncotarget 2017;8:97114-26. [Crossref] [PubMed]
- Paschalis A, Sheehan B, Riisnaes R, et al. Prostate-specific Membrane Antigen Heterogeneity and DNA Repair Defects in Prostate Cancer. Eur Urol 2019;76:469-78. [Crossref] [PubMed]
- Tang WF, Wu M, Bao H, et al. Timing and Origins of Local and Distant Metastases in Lung Cancer. J Thorac Oncol 2021;16:1136-48. [Crossref] [PubMed]
- Liu Y, Cai C, Xu W, et al. Interpretable Machine Learning-Aided Optical Deciphering of Serum Exosomes for Early Detection, Staging, and Subtyping of Lung Cancer. Anal Chem 2024;96:16227-35. [Crossref] [PubMed]
- Fiste O, Gkiozos I, Charpidou A, et al. Artificial Intelligence-Based Treatment Decisions: A New Era for NSCLC. Cancers (Basel) 2024;16:831. [Crossref] [PubMed]
- Gurcan F, Soylu A. Learning from Imbalanced Data: Integration of Advanced Resampling Techniques and Machine Learning Models for Enhanced Cancer Diagnosis and Prognosis. Cancers (Basel) 2024;16:3417. [Crossref] [PubMed]
- Pu L, Dhupar R, Meng X. Predicting Postoperative Lung Cancer Recurrence and Survival Using Cox Proportional Hazards Regression and Machine Learning. Cancers (Basel) 2024;17:33. [Crossref] [PubMed]
- Chi J, Xue Y, Zhou Y, et al. Perovskite Probe-Based Machine Learning Imaging Model for Rapid Pathologic Diagnosis of Cancers. ACS Nano 2024;18:24295-305. [Crossref] [PubMed]
- Wang L, Yin Y, Glampson B, et al. Transformer-based deep learning model for the diagnosis of suspected lung cancer in primary care based on electronic health record data. EBioMedicine 2024;110:105442. [Crossref] [PubMed]
- Warkentin MT, Al-Sawaihey H, Lam S, et al. Radiomics analysis to predict pulmonary nodule malignancy using machine learning approaches. Thorax 2024;79:307-15. [Crossref] [PubMed]
- Zhao Y, Xiong S, Ren Q, et al. Deep learning using histological images for gene mutation prediction in lung cancer: a multicentre retrospective study. Lancet Oncol 2025;26:136-46. [Crossref] [PubMed]
- Mahajan A, Kania V, Agarwal U, et al. Deep-Learning-Based Predictive Imaging Biomarker Model for EGFR Mutation Status in Non-Small Cell Lung Cancer from CT Imaging. Cancers (Basel) 2024;16:1130. [Crossref] [PubMed]
- Pan Z, Hu G, Zhu Z, et al. Predicting Invasiveness of Lung Adenocarcinoma at Chest CT with Deep Learning Ternary Classification Models. Radiology 2024;311:e232057. [Crossref] [PubMed]
- Janzen I, Ho C, Melosky B, et al. Machine Learning and Computed Tomography Radiomics to Predict Disease Progression to Upfront Pembrolizumab Monotherapy in Advanced Non-Small-Cell Lung Cancer: A Pilot Study. Cancers (Basel) 2024;17:58. [Crossref] [PubMed]
- Shibaki R, Fujimoto D, Nozawa T, et al. Machine learning analysis of pathological images to predict 1-year progression-free survival of immunotherapy in patients with small-cell lung cancer. J Immunother Cancer 2024;12:e007987. [Crossref] [PubMed]
- Captier N, Lerousseau M, Orlhac F, et al. Integration of clinical, pathological, radiological, and transcriptomic data improves prediction for first-line immunotherapy outcome in metastatic non-small cell lung cancer. Nat Commun 2025;16:614. [Crossref] [PubMed]
- Ye G, Wu G, Qi Y, et al. Non-invasive multimodal CT deep learning biomarker to predict pathological complete response of non-small cell lung cancer following neoadjuvant immunochemotherapy: a multicenter study. J Immunother Cancer 2024;12:e009348. [Crossref] [PubMed]
- Masson-Grehaigne C, Lafon M, Palussière J, et al. Enhancing Immunotherapy Response Prediction in Metastatic Lung Adenocarcinoma: Leveraging Shallow and Deep Learning with CT-Based Radiomics across Single and Multiple Tumor Sites. Cancers (Basel) 2024;16:2491. [Crossref] [PubMed]
- Marcinkiewicz AM, Buchwald M, Shanbhag A, et al. AI for Multistructure Incidental Findings and Mortality Prediction at Chest CT in Lung Cancer Screening. Radiology 2024;312:e240541. [Crossref] [PubMed]
- Karimzadeh M, Momen-Roknabadi A, Cavazos TB, et al. Deep generative AI models analyzing circulating orphan non-coding RNAs enable detection of early-stage lung cancer. Nat Commun 2024;15:10090. [Crossref] [PubMed]
- Dernbach G, Kazdal D, Ruff L, et al. Dissecting AI-based mutation prediction in lung adenocarcinoma: A comprehensive real-world study. Eur J Cancer 2024;211:114292. [Crossref] [PubMed]
- Pei G, Sun K, Yang Y, et al. Classification of multiple primary lung cancer in patients with multifocal lung cancer: assessment of a machine learning approach using multidimensional genomic data. Front Oncol 2024;14:1388575. [Crossref] [PubMed]
- Li X, Hu B, Li H, et al. Application of artificial intelligence in the diagnosis of multiple primary lung cancer. Thorac Cancer 2019;10:2168-74. [Crossref] [PubMed]
- Paech DC, Weston AR, Pavlakis N, et al. A systematic review of the interobserver variability for histology in the differentiation between squamous and nonsquamous non-small cell lung cancer. J Thorac Oncol 2011;6:55-63. [Crossref] [PubMed]
- Suh YJ, Lee HJ, Sung P, et al. A Novel Algorithm to Differentiate Between Multiple Primary Lung Cancers and Intrapulmonary Metastasis in Multiple Lung Cancers With Multiple Pulmonary Sites of Involvement. J Thorac Oncol 2020;15:203-15. [Crossref] [PubMed]
- Park E, Ahn S, Kim H, et al. Targeted Sequencing Analysis of Pulmonary Adenocarcinoma with Multiple Synchronous Ground-Glass/Lepidic Nodules. J Thorac Oncol 2018;13:1776-83. [Crossref] [PubMed]
- Ko JP, Suh J, Ibidapo O, et al. Lung Adenocarcinoma: Correlation of Quantitative CT Findings with Pathologic Findings. Radiology 2016;280:931-9. [Crossref] [PubMed]
- Saji H, Okada M, Tsuboi M, et al. Segmentectomy versus lobectomy in small-sized peripheral non-small-cell lung cancer (JCOG0802/WJOG4607L): a multicentre, open-label, phase 3, randomised, controlled, non-inferiority trial. Lancet 2022;399:1607-17. [Crossref] [PubMed]
- Hattori A, Suzuki K, Takamochi K, et al. Segmentectomy versus lobectomy in small-sized peripheral non-small-cell lung cancer with radiologically pure-solid appearance in Japan (JCOG0802/WJOG4607L): a post-hoc supplemental analysis of a multicentre, open-label, phase 3 trial. Lancet Respir Med 2024;12:105-16. [Crossref] [PubMed]
- Hu C, Zhao L, Liu W, et al. Genomic profiles and their associations with TMB, PD-L1 expression, and immune cell infiltration landscapes in synchronous multiple primary lung cancers. J Immunother Cancer 2021;9:e003773. [Crossref] [PubMed]
- Liao Y, Xu L, Lin X, et al. Temporal Trend in Lung Cancer Burden Attributed to Ambient Fine Particulate Matter in Guangzhou, China. Biomed Environ Sci 2017;30:708-17. [PubMed]
- Zhang T, Joubert P, Ansari-Pour N, et al. Genomic and evolutionary classification of lung cancer in never smokers. Nat Genet 2021;53:1348-59. [Crossref] [PubMed]
- Liu N, Yu M, Yin T, et al. Progression of malignant pleural effusion during the early stage of gefitinib treatment in advanced EGFR-mutant lung adenocarcinoma involving complex driver gene mutations. Signal Transduct Target Ther 2020;5:63. [Crossref] [PubMed]
- Mukherjee S, Zauderer MG, Ravichandran V, et al. Frequency of actionable cancer predisposing germline mutations in patients with lung cancers. J Clin Oncol 2018;36:1504. [Crossref]
- Chanock SJ. How the germline informs the somatic landscape. Nat Genet 2021;53:1523-5. [Crossref] [PubMed]
- La Salvia A, Meyer ML, Hirsch FR, et al. Rediscovering immunohistochemistry in lung cancer. Crit Rev Oncol Hematol 2024;200:104401. [Crossref] [PubMed]
- Yang Z, Zhou B, Guo W, et al. Genomic characteristics and immune landscape of super multiple primary lung cancer. EBioMedicine 2024;101:105019. [Crossref] [PubMed]