Integrative radiomics analysis of peri-tumoral and habitat zones for predicting major pathological response to neoadjuvant immunotherapy and chemotherapy in non-small cell lung cancer
Highlight box
Key findings
• An integrated nomogram including histology, Peri6mm and habitat signatures outperformed individual models with an area under the curve (AUC) of 0.894 in the training cohort, 0.831 in validation and 0.799 in testing.
What is known and what is new?
• It is crucial to identify non-small cell lung cancer (NSCLC) patients who are likely to achieve major pathological response (MPR) following neoadjuvant immunotherapy and chemotherapy (NICT).
• We analyzed the tumor area, developing an algorithm for delineating tumor habitats. The AUC of 0.894 in the training cohort, 0.831 in validation, and 0.799 in testing.
What is the implication, and what should change now?
• Our study’s development of a predictive model using a nomogram integrating clinical and radiomic features significantly improved MPR prediction in NSCLC patients undergoing NICT, enhancing clinical decision-making.
Introduction
Background
The combined use of neoadjuvant immunotherapy and chemotherapy (NICT) has emerged as a promising strategy for the treatment of resectable non-small cell lung cancer (NSCLC). Numerous studies have investigated its feasibility and effectiveness, demonstrating that this approach can improve the rate of pathological response and complete tumor resection, as well as controlling microscopically undetectable metastases, thereby improving the prognosis (1-6).
Major pathological response (MPR) serves as a surrogate endpoint for overall survival (OS) and disease-free survival (DFS) in most trials involving neoadjuvant immunotherapy for NSCLC, the observed MPR rates in current clinical studies on NICT vary significantly, with a range of 18% to 83% (1,2,4,5,7-14). Not every patient benefits from NICT, ineffective therapy can lead to surgery delays and heightened risk of immune-related adverse events. Therefore, the development of a reliable model that can predict MPR response to NICT in patients with resectable NSCLC is of paramount importance.
Computed tomography (CT) is commonly used in clinical settings for assessing post-therapy responses in NSCLC. However, numerous studies have pointed out that the tumor size measurements based on CT scans do not reliably predict pathological responses following neoadjuvant therapy in resectable NSCLC (4,5,15-17). To enhance the precision in predicting which patients will achieve MPR post-surgery in NSCLC after NICT, the incorporation of multidimensional and high-throughput imaging features is crucial. An extended aspect of radiomics involves automated segmentation of informative subregions, often referred to as habitats, which provides a comprehensive understanding of tumor behavior and its environment (18). These habitats are associated with tumor heterogeneity, which can lead to resistance to therapy, promote tumor growth, and result in a poorer prognosis (19,20).
Rationale and knowledge gap
Recent interest has grown in exploring the peritumoral region, the area immediately adjacent to the tumor mass, due to its potential in providing disease-specific prognostic indicators within the tumor microenvironment (21,22). A study indicates that radiomics texture features from CT scans, encompassing both the tumor and its peritumoral region, can serve as predictors of chemotherapy response and are associated with the prognosis of NSCLC patients (23). Zhang et al. also found a correlation between an increase in peritumoral lymphatic microvessel density and poorer NSCLC prognosis (24). Tumor-infiltrating lymphocytes and tumor-associated macrophages, vital to the tumor microenvironment, drive immune therapy responses, which may be reflected in peritumoral textural features (25,26). Additionally, radiomics features might reflect patterns associated with hypoxic tumors, which are recognized for their resistance to therapy (27).
Objective
To better predict MPR in NSCLC patients undergoing NICT, this study conducted a thorough analysis of the regions surrounding and within resectable NSCLC tumors, creating an integrative tumor microenvironment model that encompasses features of both the peri-tumoral areas and habitat-based subregions, aiming at enhancing accurate predictions and supporting clinical decision-making processes. We present this article in accordance with the TRIPOD reporting checklist (available at https://tlcr.amegroups.com/article/view/10.21037/tlcr-2024-1131/rc).
Methods
Patients and CT images acquisition
A total of 243 patients with NSCLC were retrospectively enrolled in this study from three different institutions. The patient distribution among these institutions was as follows: Shandong Cancer Hospital and Institute (Database 1) included 207 patients, Shanxi Provincial Tumor Hospital (Database 2) included 23 patients and Jining No. 1 People’s Hospital (Database 3) had 13 patients. All patients underwent NICT and surgery between November 24, 2020 and September 10, 2023. Patients in Database 1 were randomly assigned to two groups: a training dataset comprising 146 patients and a validation dataset consisting of 61 patients, adhering to a 0.7:0.3 ratio. Due to sample size limitations, patients from Databases 2 and 3 were combined to serve as test datasets. Figure S1 illustrates the selection process for patients and CT images. Comprehensive details on patients and CT image acquisition, preprocessing, treatment protocols, and follow-up strategies can be found in Appendix 1. MPR was defined as the presence of less than 10% viable tumor cells in the pathological analysis of the surgical specimen (28).
The study was conducted in accordance with the Declaration of Helsinki and its subsequent amendments. The study was approved by the ethics committee of the Shandong Cancer Hospital and Institute (No. SDTHEC2023012020), the ethics committee of Shanxi Provincial Tumor Hospital (Medical Ethics Review 2024 No. 39), and the ethics committee of Jining No. 1 People’s Hospital (No. JNRM-2024-KY-037). This study, is retrospectively registered with ResearchRegistry (researchregistry9855). Since it was a retrospective study with no risk to patients, informed consent was waived. Figure 1 illustrates the overall workflow of this study.
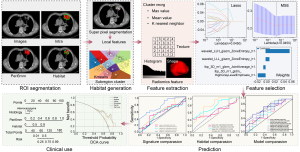
Habitat-based radiomics signature
Peri-tumoral region dilation
In our study, we methodically expanded the region of interest (ROI) masks incrementally by 2, 4, and 6 mm by using the dilation tool. Figure 2A in our paper illustrates these expanded peri-tumoral regions, showcasing the gradual enlargement process and its implications on the imaging analysis.
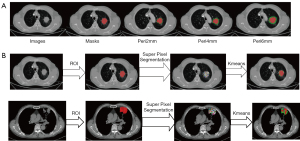
Habitat generation
In our study, we developed an algorithm to define habitat regions within tumors, a process articulated in four key stages as depicted in Figure 2B. Our methodology for delineating tumor habitat regions was multi-faceted and involved several intricate steps:
- Advanced superpixel segmentation: utilizing the simple linear iterative clustering (SLIC) algorithm within the scikit-learn framework, we initially segmented each tumor’s ROI into 100 subregions. The segmentation was fine-tuned with a compactness parameter set at 10.0, balancing color similarity and spatial proximity. The SLIC algorithm is a method for superpixel segmentation. It works by clustering pixels in the image based on their color similarity and proximity in the image space. The key formula for SLIC is the distance measure, which combines color and spatial proximity: where D is the combined distance measure, dlab is the Euclidean distance in color space (Lab color space), ds is the Euclidean distance in the image plane, and S is the grid interval or the size of the superpixel.
- Comprehensive radiomics feature extraction: for each of these subregions, a detailed extraction of 107 radiomics features was performed. This included an array of shape descriptors, textural features and first-order statistical attributes, offering a multidimensional characterization of each subregion.
- In-depth clustering analysis: the K-means algorithm was employed to analyze the multidimensional feature space. We experimented with varying numbers of cluster centers (ranging from 3 to 9) to identify distinct habitat regions within the tumor. The clustering performance was rigorously evaluated using the Calinski-Harabasz score, allowing us to select the most statistically significant clustering arrangement. The K-means algorithm is a method for clustering data into K distinct clusters. The algorithm iteratively updates the centroids of each cluster to minimize the within-cluster sum of squares. The key formula for K-means is the objective function to be minimized: Where J is the objective function, N is the number of data points, K is the number of clusters, wik is a binary indicator (1 if data point i is in cluster k, 0 otherwise), xi is the ith data point, μk is the centroid of cluster k. and is the squared Euclidean distance between data point i and centroid k.
- Habitat region synthesis: following the clustering analysis, subregions with identical cluster IDs were amalgamated. This synthesis resulted in the formation of comprehensive habitat regions, each representing a unique microenvironmental characteristic within the tumor.
Feature extraction
In our research, we classified handcrafted features into three distinct categories: (I) geometry, delineating the tumor’s three-dimensional shape; (II) intensity, focusing on the first-order analysis of voxel intensity distributions within the tumor; and (III) texture, analyzing patterns and spatial distributions of intensities through advanced second and higher order analyses. Techniques such as the gray-level co-occurrence matrix (GLCM) were employed for texture analysis. Feature extraction for each subregion was conducted using the pyradiomics tool (version 3.0.1), in compliance with the Imaging Biomarker Standardization Initiative (IBSI) guidelines. Features were independently extracted from specific habitat regions of each modality. Due to the variable number and distribution of subregions across patients, some regions exhibited insufficient voxel counts in certain habitats. Consequently, to amalgamate these diverse subregions, we evaluated three distinct methods. Firstly, we considered all subregions possessing identical features, extracting both their mean and maximum values. Additionally, we employed a k-nearest neighbors approach to impute missing data. Our experimental results indicated that the method based on maximum values yielded the most optimal outcomes. After feature extraction is completed, but before model training, the dataset is processed using the synthetic minority over-sampling technique (SMOTE) to mitigate the effects of imbalance on the final model’s performance.
Feature selection
Initially, we standardized all features using Z-scores, which normalize the data by centering around the mean and scaling based on standard deviation. This was followed by conducting t-tests to calculate P values for each feature. We retained features with P values less than 0.05, indicating statistically significant differences from the null hypothesis. To further refine our feature set, we examined repeatability using Pearson’s correlation coefficient. When pairs of features showed a high correlation (greater than 0.9), we retained only one feature from each pair. This was achieved through a greedy recursive deletion strategy, effectively reducing redundancy in our dataset. We then employed the minimum redundancy maximum relevance (mRMR) algorithm to select the top 8 features for each modality. For the final selection of features in our radiomics signature, we used least absolute shrinkage and selection operator (LASSO) regression. The optimal regularization weight (λ) for LASSO was determined through 10-fold cross-validation, ensuring a robust selection process.
Radiomics signature
In this study, we developed the intra-tumoral radiomics signature (Rad) employing a post-Lasso feature selection technique. The selected features were then applied to machine learning models such as support vector machine (SVM), Extra-Trees, Random Forest, XGBoost, LightGB, logistic regression (LR), AdaBoost, Naive Bayes, and Gradient Boosting models to build a risk model. For the peri-tumoral radiomics signature (PeriXmm), where ‘X’ signifies the peri-tumoral region, we combined intra-tumor and peri-tumoral features using the same selection method as the Rad. The final model utilized the same machine learning algorithms for analysis. In developing the habitat signature, we calculated mean feature values due to the unsupervised nature of tumor habitat clustering, excluding intraclass correlation coefficient (ICC) from the feature selection. The other selection configurations were similar to the intra-tumoral and peri-tumoral models. Across these signatures, we conducted a comprehensive evaluation of various tumor region analyses, including Rad, Peri2mm, Peri4mm, Peri6mm and habitat, to enhance the predictive modeling in tumor analysis.
Clinical use
In the clinical aspect of our study, we incorporated clinical features into our analysis, employing both univariable and multivariable methods to construct the clinical model. To improve clinical applicability, we integrated the peri-tumoral and habitat signatures with key clinical features into a nomogram. This nomogram’s diagnostic effectiveness was rigorously tested in a test cohort using receiver operating characteristic (ROC) curves for accuracy assessment and calibration curves for reliability. Additionally, the Hosmer-Lemeshow (HL) test confirmed its statistical robustness in outcome prediction. Decision curve analysis (DCA) was also performed to evaluate the nomogram’s practicality in clinical application.
Statistical analysis
Statistical tests, including the independent sample t-test and the χ² test, were conducted to compare patients’ clinical characteristics. The χ² test was used for discrete variables and the t-test for continuous variables. All data analyses were performed using Python 3.7.12. Statistical analyses employed statsmodels v0.13.2, pyradiomics v3.0.1 for radiomics feature extraction and scikit-learn v1.0.2 for machine learning algorithms. We established two classification thresholds: a median score threshold with a cutoff point of 0.5, and the cutoff point that maximizes the Youden index. We evaluated DFS and OS through the Kaplan-Meier method, employing the Log rank test for comparative analysis.
Results
Patients data and clinical features
The baseline characteristics are summarized in Table 1. The overall MPR rate in the cohort was 68% (166/243). The majority of patients were male (220/243, 91%), with a significant proportion aged 60 years or older (158/243, 65%). The predominant histological type was squamous cell carcinoma, accounting for 79% of the cases (193/243) and most patients were in clinical TNM stage III (186/243, 77%).
Table 1
Characteristics | Training cohort (n=146) | Validation cohort (n=61) | Test cohort (n=36) | ||||||||
---|---|---|---|---|---|---|---|---|---|---|---|
Non-MPR (n=46) | MPR (n=100) | P value | Non-MPR (n=18) | MPR (n=43) | P value | Non-MPR (n=13) | MPR (n=23) | P value | |||
Age | 0.60 | 0.62 | >0.99 | ||||||||
≥60 years | 30 (65.22) | 59 (59.00) | 12 (66.67) | 33 (76.74) | 9 (69.23) | 15 (65.22) | |||||
<60 years | 16 (34.78) | 41 (41.00) | 6 (33.33) | 10 (23.26) | 4 (30.77) | 8 (34.78) | |||||
Gender | 0.51 | >0.99 | >0.99 | ||||||||
Female | 6 (13.04) | 8 (8.00) | 2 (11.11) | 5 (11.63) | 1 (7.69) | 1 (4.35) | |||||
Male | 40 (86.96) | 92 (92.00) | 16 (88.89) | 38 (88.37) | 12 (92.31) | 22 (95.65) | |||||
Smoking status | 0.25 | 0.76 | 0.61 | ||||||||
No | 19 (41.30) | 30 (30.00) | 6 (33.33) | 11 (25.58) | 4 (30.77) | 4 (17.39) | |||||
Yes | 27 (58.70) | 70 (70.00) | 12 (66.67) | 32 (74.42) | 9 (69.23) | 19 (82.61) | |||||
Alcohol status | 0.70 | 0.62 | 0.55 | ||||||||
No | 33 (71.74) | 67 (67.00) | 14 (77.78) | 29 (67.44) | 11 (84.62) | 16 (69.57) | |||||
Yes | 13 (28.26) | 33 (33.00) | 4 (22.22) | 14 (32.56) | 2 (15.38) | 7 (30.43) | |||||
Histology | 0.004 | 0.65 | >0.99 | ||||||||
Adenocarcinoma | 18 (39.13) | 16 (16.00) | 5 (27.78) | 8 (18.60) | 1 (7.69) | 2 (8.70) | |||||
SCC | 28 (60.87) | 84 (84.00) | 13 (72.22) | 35 (81.40) | 12 (92.31) | 21 (91.30) | |||||
Surgica approach | 0.60 | 0.67 | 0.49 | ||||||||
Thoracotomy | 21 (45.65) | 52 (52.00) | 11 (61.11) | 22 (51.16) | 3 (23.08) | 2 (8.70) | |||||
VATS | 25 (54.35) | 48 (48.00) | 7 (38.89) | 21 (48.84) | 10 (76.92) | 21 (91.30) | |||||
Neoadjuvant therapy cycle | 0.07 | 0.19 | 0.44 | ||||||||
1 | 3 (6.52) | 0 | 1 (5.56) | 0 | 0 | 0 | |||||
2 | 24 (52.17) | 54 (54.00) | 13 (72.22) | 27 (62.79) | 9 (69.23) | 16 (69.57) | |||||
3 | 15 (32.61) | 39 (39.00) | 2 (11.11) | 13 (30.23) | 2 (15.38) | 6 (26.09) | |||||
4 | 4 (8.70) | 7 (7.00) | 2 (11.11) | 3 (6.98) | 2 (15.38) | 1 (4.35) | |||||
Clinical T stage | 0.70 | 0.16 | 0.52 | ||||||||
1 | 3 (6.52) | 6 (6.00) | 2 (11.11) | 2 (4.65) | 0 | 3 (13.04) | |||||
2 | 24 (52.17) | 44 (44.00) | 7 (38.89) | 30 (69.77) | 8 (61.54) | 13 (56.52) | |||||
3 | 7 (15.22) | 23 (23.00) | 6 (33.33) | 7 (16.28) | 2 (15.38) | 4 (17.39) | |||||
4 | 12 (26.09) | 27 (27.00) | 3 (16.67) | 4 (9.30) | 3 (23.08) | 3 (13.04) | |||||
Clinical N stage | 0.29 | 0.004 | 0.46 | ||||||||
0 | 9 (19.57) | 27 (27.00) | 8 (44.44) | 4 (9.30) | 3 (23.08) | 2 (8.70) | |||||
1 | 8 (17.39) | 24 (24.00) | 4 (22.22) | 8 (18.60) | 3 (23.08) | 5 (21.74) | |||||
2 | 29 (63.04) | 49 (49.00) | 6 (33.33) | 31 (72.09) | 7 (53.85) | 16 (69.57) | |||||
Clinical TNM stage | 0.41 | 0.22 | >0.99 | ||||||||
I | 1 (2.17) | 2 (2.00) | 1 (5.56) | 3 (6.98) | 0 | 0 | |||||
II | 7 (15.22) | 25 (25.00) | 6 (33.33) | 6 (13.95) | 2 (15.38) | 4 (17.39) | |||||
III | 38 (82.61) | 73 (73.00) | 11 (61.11) | 34 (79.07) | 11 (84.62) | 19 (82.61) | |||||
Surgical procedure | 0.74 | 0.29 | 0.52 | ||||||||
Bilobectomy | 5 (10.87) | 9 (9.00) | 1 (5.56) | 2 (4.65) | 1 (7.69) | 4 (17.39) | |||||
Lobectomy | 38 (82.61) | 87 (87.00) | 14 (77.78) | 39 (90.70) | 12 (92.31) | 18 (78.26) | |||||
Pneumonectomy | 3 (6.52) | 4 (4.00) | 3 (16.67) | 2 (4.65) | 0 | 1 (4.35) |
Data are presented as n (%). MPR, major pathologic response; SCC, squamous cell carcinoma; TNM, tumor node metastasis; VATS, video-assisted thoracic surgery.
We performed a comprehensive univariate and multivariable analysis on all clinical features. As shown in Table 2, histology emerged as an independent predictor for MPR, with a P value under 0.05. Tumor volume did not emerge as a significant predictor of MPR.
Table 2
Variables | Univariate analysis | Multivariate analysis | |||
---|---|---|---|---|---|
OR (95% CI) | P value | OR (95% CI) | P value | ||
Age (≥60 years) | 0.945 (0.509, 1.755) | 0.86 | |||
Smoking status (yes) | 1.595 (0.858, 2.962) | 0.14 | |||
Alcohol status (yes) | 1.354 (0.703, 2.607) | 0.37 | |||
Gender (male) | 1.429 (0.561, 3.638) | 0.46 | |||
Histology (SCC) | 2.782 (1.419, 5.453) | 0.003 | 2.782 (1.419,5.453) | 0.003 | |
Neoadjuvant therapy cycle (≤2) | 0.733 (0.399, 1.347) | 0.32 | |||
Surgical approach (VATS) | 0.932 (0.517, 1.682) | 0.82 | |||
Clinical T stage | |||||
1 | Reference | ||||
2 | 1.492 (0.452, 4.921) | 0.51 | |||
3 | 1.442 (0.396, 5.256) | 0.58 | |||
4 | 1.292 (0.361, 4.628) | 0.70 | |||
Clinical N stage | |||||
0 | Reference | ||||
1 | 1.462 (0.601, 3.557) | 0.40 | |||
2 | 1.253 (0.615, 2.556) | 0.53 | |||
Clinical TNM stage | |||||
I | Reference | ||||
II | 0.954 (0.164, 5.561) | 0.96 | |||
III | 0.873 (0.164, 4.660) | 0.87 | |||
Surgical procedure | |||||
Bilobectomy | Reference | ||||
Lobectomy | 1.322 (0.464, 3.761) | 0.60 | |||
Pneumonectomy | 0.545 (0.121, 2.461) | 0.43 | |||
Tumor size (cm3) | 0.998 (0.984, 1.012) | 0.76 |
CI, confidence interval; OR, odds ratio; SCC, squamous cell carcinoma; TNM, tumor node metastasis; VATS, video-assisted thoracic surgery.
Radiomics features and models development
In our research, we successfully extracted 1,834 handcrafted radiomics features, systematically classifying them into shape, first-order and texture categories. Among these, 360 were first-order features and 14 were shape features, complemented by a diverse array texture features. The distribution of these feature groups is graphically depicted in Figure 3A, offering a visual overview of their relative proportions within our dataset. Figure 3B-3D depicts the selection of habitat radiomics features employing the LASSO algorithm, from which four habitat radiomics features have been identified and chosen for subsequent analysis. For detailed results regarding peri-tumoral 2, 4, 6 mm and intra-tumoral radiomics features, please see Figure S2.
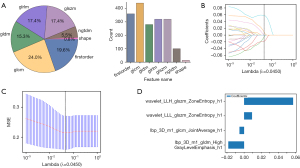
Further, we conducted an in-depth evaluation of shape-related radiomics features for their potential to predict MPR following NICT in patients with NSCLC. The results showed that all shape features possessed P values exceeding 0.05, signifying their insignificance in the prediction of MPR (Table S1).
Radiomics models performance
Figure 4 depicts the ROC curves of various machine learning algorithms in the habitat model across the training, testing and validation sets. XGBoost demonstrated superior performance in the validation cohort, achieving the highest area under the curve (AUC) score of 0.822, thereby outperforming other models in controlled settings. In contrast, models such as Random Forest, Extra Trees, SVM and LightGBM, while showing robust training performances, exhibited decreased effectiveness in the test cohort, suggesting challenges in their generalization capabilities relative to XGBoost. Given these insights, we chose XGBoost for the habitat model in subsequent signature comparisons due to its standout validation performance, indicating superior predictive ability in controlled environments. Tables S2-S6 showcase predictive performance for intra-tumoral, peritumoral (2, 4, 6 mm), and habitat signatures in predicting MPR using various machine learning algorithms across across training, testing, and validation datasets. Similarly, for other models, we selected the best-performing machine learning classifier for comparative analysis: the ExtraTrees model for the intra-tumoral signature, LightGBM for the Peri2mm signature, RandomForest for the Peri4mm signature, and ExtraTrees for the Peri6mm signature. We ultimately selected Peri6mm and habitat signatures for further analysis. Detailed performance results comparing radiomics signatures in predicting MPR are presented in Figure S3.
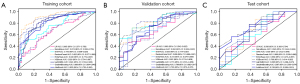
Signature comparison and clinical use
We combined insights from the peri-tumoral and habitat signatures with clinically significant features, identified via multivariate analysis, into an integrated model. This model was effectively visualized using a nomogram (Figure 5), which facilitates clinical utilization. Table 3 compares the performance metrics of clinical characteristics, Peri6mm, habitat, and the combined predictive power of three models within a nomogram, using the cutoff point that maximizes the Youden index. Table 4 illustrates the comparative performance metrics of various models utilizing a median score threshold (cutoff point of 0.5). In applying model prospectively to clinical scenarios, it is possible to adjust the cutoff value according to specific requirements to achieve desired clinical outcomes.
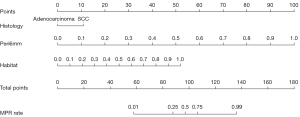
Table 3
Cohort | Signature | Accuracy | AUC (95% CI) | Sensitivity | Specificity | PPV | NPV |
---|---|---|---|---|---|---|---|
Training | Clinical | 0.863 | 0.917 (0.8692–0.9643) | 0.880 | 0.826 | 0.917 | 0.760 |
Peri6mm | 0.747 | 0.730 (0.6500–0.8096) | 0.850 | 0.522 | 0.794 | 0.615 | |
Habitat | 0.712 | 0.826 (0.7540–0.8970) | 0.660 | 0.826 | 0.892 | 0.528 | |
Nomogram | 0.890 | 0.894 (0.8313–0.9567) | 0.950 | 0.761 | 0.896 | 0.875 | |
Validation | Clinical | 0.639 | 0.545 (0.3861–0.7044) | 0.744 | 0.389 | 0.744 | 0.389 |
Peri6mm | 0.738 | 0.554 (0.4210–0.6876) | 0.977 | 0.167 | 0.737 | 0.750 | |
Habitat | 0.574 | 0.822 (0.7160–0.9287) | 0.395 | 1.000 | 1.000 | 0.409 | |
Nomogram | 0.721 | 0.831 (0.7255–0.9360) | 0.674 | 0.833 | 0.906 | 0.517 | |
Test | Clinical | 0.583 | 0.622 (0.4376–0.8066) | 0.348 | 1.000 | 1.000 | 0.464 |
Peri6mm | 0.583 | 0.609 (0.4240–0.7934) | 0.783 | 0.231 | 0.643 | 0.375 | |
Habitat | 0.639 | 0.769 (0.6138–0.9247) | 0.522 | 0.846 | 0.857 | 0.500 | |
Nomogram | 0.722 | 0.799 (0.6412–0.9575) | 0.696 | 0.769 | 0.842 | 0.588 |
AUC, area under the curve; CI, confidence interval; NPV, negative predictive value; PPV, positive predictive value.
Table 4
Cohort | Signature | Accuracy | AUC (95% CI) | Sensitivity | Specificity | PPV | NPV |
---|---|---|---|---|---|---|---|
Training | Clinical | 0.801 | 0.917 (0.8692–0.9643) | 0.960 | 0.457 | 0.793 | 0.840 |
Peri6mm | 0.774 | 0.730 (0.6500–0.8096) | 0.980 | 0.326 | 0.760 | 0.882 | |
Habitat | 0.740 | 0.826 (0.7540–0.8970) | 0.760 | 0.696 | 0.844 | 0.571 | |
Nomogram | 0.897 | 0.894 (0.8313–0.9567) | 0.970 | 0.739 | 0.890 | 0.919 | |
Validation | Clinical | 0.656 | 0.545 (0.3861–0.7044) | 0.860 | 0.167 | 0.712 | 0.333 |
Peri6mm | 0.738 | 0.554 (0.4210–0.6876) | 0.977 | 0.167 | 0.737 | 0.750 | |
Habitat | 0.705 | 0.822 (0.7160–0.9287) | 0.698 | 0.722 | 0.857 | 0.500 | |
Nomogram | 0.787 | 0.831 (0.7255–0.9360) | 0.907 | 0.500 | 0.812 | 0.692 | |
Test | Clinical | 0.583 | 0.622 (0.4376–0.8066) | 0.870 | 0.077 | 0.625 | 0.250 |
Peri6mm | 0.583 | 0.609 (0.4240–0.7934) | 0.783 | 0.231 | 0.643 | 0.375 | |
Habitat | 0.639 | 0.769 (0.6138–0.9247) | 0.609 | 0.692 | 0.778 | 0.500 | |
Nomogram | 0.778 | 0.799 (0.6412–0.9575) | 0.913 | 0.538 | 0.778 | 0.778 |
AUC, area under the curve; CI, confidence interval; NPV, negative predictive value; PPV, positive predictive value.
The nomogram, integrating features from the habitat, Peri6mm and histology, significantly enhances predictive performance. It achieves the highest accuracy (0.890) and a high AUC (0.894) in the training cohort, surpassing individual models. The model maintains its superior performance in the validation cohort with an AUC of 0.831, outperforming the Peri6mm and clinical model while matching the efficacy of the habitat model. In the test cohort, the nomogram maintains superior performance with an AUC of 0.799, demonstrating its effectiveness even when compared to the strong results of the habitat model. Figure 6A shows the ROC curves for clinical characteristic, Peri6mm, habitat and the combined predictive efficacy of three models in a nomogram. In our analysis, the nomogram model exhibited superior calibration performance across all cohorts, as evidenced by its HL test statistics of 0.319 in the training cohort, 0.194 in the validation cohort, and 0.106 in the test cohort. Figure 6B shows the calibration curves for different models in the training, validation and test cohort. Figure 6C displays the DeLong test results for different models. It can be observed that the Nomogram, which incorporates both clinical and Peri6mm results, exhibits a certain improvement in performance. Figure 6D shows the DCA curves for the training, validation and test sets. From the results, it can be observed that our fusion model (nomogram) yields noticeable benefits based on the predicted probabilities.
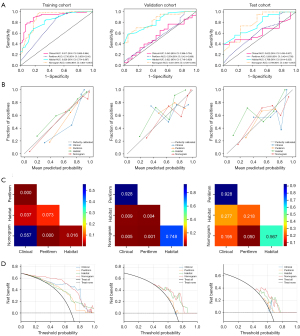
Figure 7A,7B illustrate the DFS and OS curves, respectively, for NICT patients in the MPR group versus the non-MPR group at our center. The MPR group shows better DFS and OS, with significant statistical differences between the two groups.
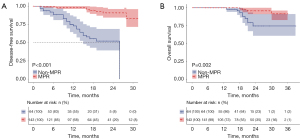
Discussion
Key findings
Our study employed a methodology integrating SLIC algorithm-based superpixel segmentation, comprehensive radiomics feature extraction, K-means clustering analysis and synthesis of habitat regions. This approach provided detailed insights into the tumor microenvironment and enhanced the predictive accuracy for MPR in NSCLC.
Comparison with similar researches
MPR is increasingly recognized as a critical prognostic marker in resectable NSCLC, particularly in the context of NICT. The ability of MPR to accurately mirror the tumor’s response to these treatments is essential for prognosticating patient outcomes. Research has shown that MPR is linked to long-term OS in patients with NSCLC who undergo neoadjuvant chemotherapy (29). This underscores the utility of MPR as a surrogate endpoint for survival and as an evaluative tool for neoadjuvant therapy in clinical trials. Furthermore, numerous studies have delved into the prognostic significance of MPR in NSCLC patients receiving NICT. Findings indicate that MPR correlates with improved DFS and OS, thereby positioning MPR could be effectively used as a potential surrogate marker for survival outcomes in evaluating the efficacy of NICT (3,5,6,30,31). Our study and related findings highlight the pivotal role of MPR and related markers in determining the effectiveness of neoadjuvant treatment strategies in NSCLC, which is also the reason why we chose MPR as the predictor of the effectiveness of NICT in NSCLC.
Intra-tumor spatial heterogeneity, stemming from varied tumor clonality and microenvironmental factors, is a known driver of tumor adaptation and resistance during cancer treatment (32). The application of noninvasive medical imaging for longitudinal tissue property analysis positions radiomics as an indispensable tool in characterizing cancer cell behavior and the tumor microenvironment, as well as in monitoring therapeutic responses. Given the heterogeneous nature of tumors, which display varied phenotypes in the peri-tumor and intra-tumor areas, it’s crucial to evolve conventional radiomics analysis into a three-dimensional approach. Fine characterization of spatial phenotypic distribution is possible by computing voxel features and categorizing tumor subregions into imaging habitats based on radiographic similarities (33,34). Employing imaging habitats derived from CT images has unveiled hidden tumor heterogeneity, significantly enhancing the accuracy of diagnostic and predictive models in lung cancer, thereby improving patient management and treatment outcomes (35). Based on these perspectives our research involved developing an innovative, multifaceted radiomics model to enhance the prediction of MPR in NSCLC, which entailed a detailed analysis of various tumor regions including Rad, Peri2mm, Peri4mm, Peri6mm and habitat.
Our study aims to define peritumoral regions at various distances from the tumor boundary to identify the optimal area that most accurately reflects the tumor microenvironment relevant to our research question. It has been shown that to cover 95% of micrometastases, the peripheral extension should be adjusted to 8 mm for lung adenocarcinoma and 6 mm for lung squamous carcinoma (36). Additionally, other research has suggested that an area ranging from 3 to 9 mm around the tumor can provide biological insights into the heterogeneity of lung adenocarcinoma (37). Our analysis assessed peritumoral expansions of 2, 4, and 6 mm, drawing on a breast cancer study on radiomics that used thicknesses of 2, 4, 6, and 8 mm to pinpoint the optimal size for predicting treatment outcomes effectively (38). We intentionally limited the expansion to no more than 6 mm to prevent encroachment into extrapulmonary areas, which could potentially reduce the specificity of our radiomics features. Our research findings indicate the significance of optimizing the size of the peritumoral region in future radiomics studies, with the aim of enhancing the accuracy of predictive models and boosting their efficacy.
Habitat model’s consistent and reliable performance across various datasets underscores its significance as a vital tool in medical imaging predictive analysis. This is supported by numerous studies and findings. A study on 18F-fluorodeoxyglucose positron emission tomography/CT radiomics, which incorporated habitat imaging to distinguish between NSCLC and benign inflammatory diseases, demonstrated the efficacy of the habitat model (39). The model showed improved discrimination performance, highlighting its potential as a biomarker for differentiating between these conditions. Another study involving the survival risk stratification of clinical and pathologic stage IA pure-solid NSCLC through radiomics analysis further reinforced the utility of the habitat model (40). By extracting features from different tumor regions and combining them into a comprehensive radiomics signature, the study showcased the model’s predictive accuracy, particularly in survival risk stratification. Another research focusing on the prognostication of cancer recurrence in early stage NSCLC used a habitat model that integrated features based on stability and discriminability criteria (41). This model demonstrated higher discriminability in validation datasets, suggesting its effectiveness in predicting cancer recurrence following surgery. These studies provide a robust foundation for the habitat model’s application in predictive analysis in medical imaging, particularly in the context of NSCLC.
Strengths and limitations
In our study, we recognized the significance of both the peri-tumoral and habitat regions as representations of the tumor microenvironment, as well as the vital role of patient clinical information. To improve its clinical relevance, we combined insights from the peri-tumoral and habitat signatures with clinically significant features, identified via multivariate analysis, into an integrated model. Our study recognizes the advantages of deep learning approaches (42), yet distinguishes itself by employing an integrative radiomics strategy to delve into the tumor heterogeneity of NSCLC. Our method offers a complementary perspective to deep learning models, significantly enhancing interpretability and clinical relevance. Notably, our research introduces a novel approach by exploring, for the first time to our knowledge, the predictive importance of tumor habitat in NSCLC. Moreover, our detailed analysis of the tumor microenvironment, including the peri-tumoral, intra-tumoral, and habitat subregions, captures a comprehensive radiomics profile of the tumor landscape, offering a holistic view of its complexity. The limitations of deep learning are notably evident in its “black box” nature (43). While techniques like Grad-CAM offer some insights through heatmaps, the interpretability of these models can be significantly compromised, especially for more complex samples. A major reason we opted not to use deep learning in this study stems from the characteristics of habitat clustering outcomes. Specifically, the segmentation results often include numerous non-contiguous regions, whereas deep learning generally relies on rectangular image inputs. This mismatch could result in training data that span multiple habitat zones, complicating the analysis. Additionally, a key advantage of habitat analysis is the interpretability of its subregions. Integrating deep learning into this analysis could potentially diminish these insights, detracting from the intrinsic value of habitat-based analysis.
There are several notable limitations in the current study that should be taken into account. The retrospective nature of the study and the necessity for prospective validation are areas that need to be addressed by future research. Additionally, the limited sample size in this study represents a constraint, highlighting the need for research with larger datasets to confirm these findings. Furthermore, we have not explored the biological connections of the radiomics features. Another limitation involves the determination of cutoff thresholds for predictive values. The positive predictive value (PPV) and negative predictive value (NPV) are significantly influenced by the chosen cutoff threshold, as different thresholds can alter levels of sensitivity and specificity, thereby affecting the resulting PPV and NPV values. Initially, we utilized the Youden index to determine the optimal classification threshold, which may have led to variability in PPV and NPV across various datasets. We discovered that adopting a fixed threshold of 0.5 for the cutoff value resulted in a more consistent distribution of PPV, NPV, sensitivity, and specificity for the habitat signature. When prospectively applying this model in clinical settings, it is essential to adjust the cutoff value to meet specific clinical requirements and optimize outcomes. Future research should focus on improving the model and exploring its relevance to underlying biological processes.
Implications and actions needed
Future research should focus on improving the model and exploring its relevance to underlying biological processes. Multi-omics approaches are evaluated to have superior predictive performance compared to stand-alone radiomics or pathomics. Given the continuously evolving nature of AI models, future model iterations will aim to integrate more dimensions of patient data to further improve predictive performance. Subsequent studies should focus on combining pathomics, single-cell sequencing, and proteomics to build multimodal predictive models to further improve predictive performance.
Conclusions
The nomogram’s integration of diverse features from habitat, Peri6mm and clinical model results in a marked improvement in accuracy and AUC across all cohorts. This is particularly significant for predicting the MPR after NICT in NSCLC patients. This combined approach highlights the benefit of merging different but complementary data sources. Notably, the enhanced performance of the nomogram in validation and test cohorts highlights its potential as a holistic and accurate tool for clinical applications.
Acknowledgments
A portion of this study has been presented in the form of a poster at the “World Conference on Lung Cancer”.
Footnote
Reporting Checklist: The authors have completed the TRIPOD reporting checklist. Available at https://tlcr.amegroups.com/article/view/10.21037/tlcr-2024-1131/rc
Data Sharing Statement: Available at https://tlcr.amegroups.com/article/view/10.21037/tlcr-2024-1131/dss
Peer Review File: Available at https://tlcr.amegroups.com/article/view/10.21037/tlcr-2024-1131/prf
Funding: This research was supported by various grants, including
Conflicts of Interest: All authors have completed the ICMJE uniform disclosure form (available at https://tlcr.amegroups.com/article/view/10.21037/tlcr-2024-1131/coif). The authors have no conflicts of interest to declare.
Ethical Statement: The authors are accountable for all aspects of the work in ensuring that questions related to the accuracy or integrity of any part of the work are appropriately investigated and resolved. The study was conducted in accordance with the Declaration of Helsinki and its subsequent amendments. The study was approved by the ethics committee of the Shandong Cancer Hospital and Institute (No. SDTHEC2023012020), the ethics committee of Shanxi Provincial Tumor Hospital (Medical Ethics Review 2024 No. 39), and the ethics committee of Jining No. 1 People’s Hospital (No. JNRM-2024-KY-037). Since it was a retrospective study with no risk to patients, informed consent was waived.
Open Access Statement: This is an Open Access article distributed in accordance with the Creative Commons Attribution-NonCommercial-NoDerivs 4.0 International License (CC BY-NC-ND 4.0), which permits the non-commercial replication and distribution of the article with the strict proviso that no changes or edits are made and the original work is properly cited (including links to both the formal publication through the relevant DOI and the license). See: https://creativecommons.org/licenses/by-nc-nd/4.0/.
References
- Shu CA, Gainor JF, Awad MM, et al. Neoadjuvant atezolizumab and chemotherapy in patients with resectable non-small-cell lung cancer: an open-label, multicentre, single-arm, phase 2 trial. Lancet Oncol 2020;21:786-95. [Crossref] [PubMed]
- Cascone T, Leung CH, Weissferdt A, et al. Neoadjuvant chemotherapy plus nivolumab with or without ipilimumab in operable non-small cell lung cancer: the phase 2 platform NEOSTAR trial. Nat Med 2023;29:593-604. [Crossref] [PubMed]
- Chen Y, Qin J, Wu Y, et al. Does major pathological response after neoadjuvant Immunotherapy in resectable nonsmall-cell lung cancers predict prognosis? A systematic review and meta-analysis. Int J Surg 2023;109:2794-807. [Crossref] [PubMed]
- Forde PM, Spicer J, Lu S, et al. Neoadjuvant Nivolumab plus Chemotherapy in Resectable Lung Cancer. N Engl J Med 2022;386:1973-85. [Crossref] [PubMed]
- Provencio M, Nadal E, Insa A, et al. Neoadjuvant chemotherapy and nivolumab in resectable non-small-cell lung cancer (NADIM): an open-label, multicentre, single-arm, phase 2 trial. Lancet Oncol 2020;21:1413-22. [Crossref] [PubMed]
- Zhao J, Hao S, Li Y, et al. Comparative Efficacy and Safety of Neoadjuvant Immunotherapy with Chemotherapy versus Chemotherapy Alone in Non-Small Cell Lung Cancer: A Propensity Score and Inverse Probability Treatment Weighting Analysis. Immunotargets Ther 2023;12:113-33. [Crossref] [PubMed]
- Heymach JV, Mitsudomi T, Harpole D, et al. Design and Rationale for a Phase III, Double-Blind, Placebo-Controlled Study of Neoadjuvant Durvalumab + Chemotherapy Followed by Adjuvant Durvalumab for the Treatment of Patients With Resectable Stages II and III non-small-cell Lung Cancer: The AEGEAN Trial. Clin Lung Cancer 2022;23:e247-51. [Crossref] [PubMed]
- Lu S, Wu L, Zhang W, et al. Perioperative toripalimab + platinum-doublet chemotherapy vs chemotherapy in resectable stage II/III non-small cell lung cancer (NSCLC): Interim event-free survival (EFS) analysis of the phase III Neotorch study. J Clin Oncol 2023;41:8501.
- Qiu F, Fan J, Shao M, et al. Two cycles versus three cycles of neoadjuvant sintilimab plus platinum-doublet chemotherapy in patients with resectable non-small-cell lung cancer (neoSCORE): A randomized, single center, two-arm phase II trial. J Clin Oncol 2022;40:8500.
- Wakelee H, Liberman M, Kato T, et al. Perioperative Pembrolizumab for Early-Stage Non-Small-Cell Lung Cancer. N Engl J Med 2023;389:491-503. [Crossref] [PubMed]
- Yan W, Zhong WZ, Liu YH, et al. Adebrelimab (SHR-1316) in Combination With Chemotherapy as Perioperative Treatment in Patients With Resectable Stage II to III NSCLCs: An Open-Label, Multicenter, Phase 1b Trial. J Thorac Oncol 2023;18:194-203. [Crossref] [PubMed]
- Zhao Z, Chen S, Qi H, et al. Phase II trial of toripalimab plus chemotherapy as neoadjuvant treatment in resectable stage III non-small cell lung cancer (NeoTPD01 Study). J Clin Oncol 2021;39:8541.
- Rothschild SI, Zippelius A, Eboulet EI, et al. SAKK 16/14: Durvalumab in Addition to Neoadjuvant Chemotherapy in Patients With Stage IIIA(N2) Non-Small-Cell Lung Cancer-A Multicenter Single-Arm Phase II Trial. J Clin Oncol 2021;39:2872-80. [Crossref] [PubMed]
- Zhao ZR, Yang CP, Chen S, et al. Phase 2 trial of neoadjuvant toripalimab with chemotherapy for resectable stage III non-small-cell lung cancer. Oncoimmunology 2021;10:1996000. [Crossref] [PubMed]
- William WN Jr, Pataer A, Kalhor N, et al. Computed tomography RECIST assessment of histopathologic response and prediction of survival in patients with resectable non-small-cell lung cancer after neoadjuvant chemotherapy. J Thorac Oncol 2013;8:222-8. [Crossref] [PubMed]
- Forde PM, Chaft JE, Smith KN, et al. Neoadjuvant PD-1 Blockade in Resectable Lung Cancer. N Engl J Med 2018;378:1976-86. [Crossref] [PubMed]
- Provencio M, Serna-Blasco R, Nadal E, et al. Overall Survival and Biomarker Analysis of Neoadjuvant Nivolumab Plus Chemotherapy in Operable Stage IIIA Non-Small-Cell Lung Cancer (NADIM phase II trial). J Clin Oncol 2022;40:2924-33. [Crossref] [PubMed]
- Napel S, Mu W, Jardim-Perassi BV, et al. Quantitative imaging of cancer in the postgenomic era: Radio(geno)mics, deep learning, and habitats. Cancer 2018;124:4633-49. [Crossref] [PubMed]
- Wu J, Cui Y, Sun X, et al. Unsupervised Clustering of Quantitative Image Phenotypes Reveals Breast Cancer Subtypes with Distinct Prognoses and Molecular Pathways. Clin Cancer Res 2017;23:3334-42. [Crossref] [PubMed]
- Dagogo-Jack I, Shaw AT. Tumour heterogeneity and resistance to cancer therapies. Nat Rev Clin Oncol 2018;15:81-94. [Crossref] [PubMed]
- Tunali I, Hall LO, Napel S, et al. Stability and reproducibility of computed tomography radiomic features extracted from peritumoral regions of lung cancer lesions. Med Phys 2019;46:5075-85. [Crossref] [PubMed]
- Pérez-Morales J, Tunali I, Stringfield O, et al. Peritumoral and intratumoral radiomic features predict survival outcomes among patients diagnosed in lung cancer screening. Sci Rep 2020;10:10528. [Crossref] [PubMed]
- Khorrami M, Khunger M, Zagouras A, et al. Combination of Peri- and Intratumoral Radiomic Features on Baseline CT Scans Predicts Response to Chemotherapy in Lung Adenocarcinoma. Radiol Artif Intell 2019;1:e180012. [Crossref] [PubMed]
- Zhang BC, Guan S, Zhang YF, et al. Peritumoral lymphatic microvessel density is related to poor prognosis in lung adenocarcinoma: A retrospective study of 65 cases. Exp Ther Med 2012;3:636-40. [Crossref] [PubMed]
- Geng Y, Shao Y, He W, et al. Prognostic Role of Tumor-Infiltrating Lymphocytes in Lung Cancer: a Meta-Analysis. Cell Physiol Biochem 2015;37:1560-71. [Crossref] [PubMed]
- Parra ER, Behrens C, Rodriguez-Canales J, et al. Image Analysis-based Assessment of PD-L1 and Tumor-Associated Immune Cells Density Supports Distinct Intratumoral Microenvironment Groups in Non-small Cell Lung Carcinoma Patients. Clin Cancer Res 2016;22:6278-89. [Crossref] [PubMed]
- Ganeshan B, Goh V, Mandeville HC, et al. Non-small cell lung cancer: histopathologic correlates for texture parameters at CT. Radiology 2013;266:326-36. [Crossref] [PubMed]
- Dacic S, Travis W, Redman M, et al. International Association for the Study of Lung Cancer Study of Reproducibility in Assessment of Pathologic Response in Resected Lung Cancers After Neoadjuvant Therapy. J Thorac Oncol 2023;18:1290-302. [Crossref] [PubMed]
- Weissferdt A, Pataer A, Vaporciyan AA, et al. Agreement on Major Pathological Response in NSCLC Patients Receiving Neoadjuvant Chemotherapy. Clin Lung Cancer 2020;21:341-8. [Crossref] [PubMed]
- Liu Y, Xiong L, Chen Y, et al. Complete pathological remission and tertiary lymphoid structures are associated with the efficacy of resectable NSCLC receiving neoadjuvant chemoimmunotherapy: A double-center retrospective study. Hum Vaccin Immunother 2023;19:2285902. [Crossref] [PubMed]
- Zhang B, Xiao H, Pu X, et al. A real-world comparison between neoadjuvant chemoimmunotherapy and chemotherapy alone for resectable non-small cell lung cancer. Cancer Med 2023;12:274-86. [Crossref] [PubMed]
- Gerlinger M, Rowan AJ, Horswell S, et al. Intratumor heterogeneity and branched evolution revealed by multiregion sequencing. N Engl J Med 2012;366:883-92. [Crossref] [PubMed]
- Bernatowicz K, Grussu F, Ligero M, et al. Robust imaging habitat computation using voxel-wise radiomics features. Sci Rep 2021;11:20133. [Crossref] [PubMed]
- Xue C, Zhou Q, Xi H, et al. Radiomics: A review of current applications and possibilities in the assessment of tumor microenvironment. Diagn Interv Imaging 2023;104:113-22. [Crossref] [PubMed]
- Cherezov D, Goldgof D, Hall L, et al. Revealing Tumor Habitats from Texture Heterogeneity Analysis for Classification of Lung Cancer Malignancy and Aggressiveness. Sci Rep 2019;9:4500. [Crossref] [PubMed]
- Giraud P, Antoine M, Larrouy A, et al. Evaluation of microscopic tumor extension in non-small-cell lung cancer for three-dimensional conformal radiotherapy planning. Int J Radiat Oncol Biol Phys 2000;48:1015-24. [Crossref] [PubMed]
- Liu K, Li K, Wu T, et al. Improving the accuracy of prognosis for clinical stage I solid lung adenocarcinoma by radiomics models covering tumor per se and peritumoral changes on CT. Eur Radiol 2022;32:1065-77. [Crossref] [PubMed]
- Ding J, Chen S, Serrano Sosa M, et al. Optimizing the Peritumoral Region Size in Radiomics Analysis for Sentinel Lymph Node Status Prediction in Breast Cancer. Acad Radiol 2022;29:S223-8. [Crossref] [PubMed]
- Chen L, Liu K, Zhao X, et al. Habitat Imaging-Based (18)F-FDG PET/CT Radiomics for the Preoperative Discrimination of Non-small Cell Lung Cancer and Benign Inflammatory Diseases. Front Oncol 2021;11:759897. [Crossref] [PubMed]
- Wang T, She Y, Yang Y, et al. Radiomics for Survival Risk Stratification of Clinical and Pathologic Stage IA Pure-Solid Non-Small Cell Lung Cancer. Radiology 2022;302:425-34. [Crossref] [PubMed]
- Khorrami M, Bera K, Leo P, et al. Stable and discriminating radiomic predictor of recurrence in early stage non-small cell lung cancer: Multi-site study. Lung Cancer 2020;142:90-7. [Crossref] [PubMed]
- She Y, He B, Wang F, et al. Deep learning for predicting major pathological response to neoadjuvant chemoimmunotherapy in non-small cell lung cancer: A multicentre study. EBioMedicine 2022;86:104364. [Crossref] [PubMed]
- Castelvecchi D. Can we open the black box of AI? Nature 2016;538:20-3. [Crossref] [PubMed]