A narrative review of preoperative CT for predicting spread through air spaces of lung cancer
Introduction
Lung cancer is the leading cause of cancer-related deaths worldwide (1), with a generally poor prognosis (2). Tumor aggressiveness significantly impacts outcomes in lung cancer. Traditionally, lung cancer invasion is classified into three patterns: (I) a non-lepidic histologic growth pattern, (II) myofibroblastic proliferation with desmoplasia, and (III) vascular or pleural invasion. In 2015, the World Health Organization recognized “spread through air spaces” (STAS) as a fourth type of invasion, which is defined by the presence of micropapillary clusters, solid nests or single tumor cells spreading into air spaces beyond the primary tumor (3).
While postoperative pathology remains the gold standard for diagnosing STAS, it is not timely enough to guide surgical decisions. The sensitivity of frozen section analysis for detecting STAS is relatively low, with reported rates ranging from 44% to 71% (4-6). Other diagnostic methods, such as bronchial cytology (7) and intraoperative frozen sections (8), also lack sufficient accuracy to meet clinical needs. Therefore, developing accurate noninvasive prediction methods using preoperative computed tomography (CT) imaging is crucial for improving clinical decision-making.
Local resection has become a major focus on the surgical management of early-stage lung cancer. Lobectomy has long been the standard treatment for early non-small cell lung cancer (NSCLC). In recent years, advances in imaging technology have led to an increase in the detection rate of small peripheral tumors. Therefore, sublobar resection is now performed more frequently not only for low-risk patients but also for high-risk patients with clinical stage IA NSCLC (9-12). A multi-center clinical trial, JCOG0802/WJOG4607L, demonstrated that sublobar resection provided better overall survival (OS) compared to lobectomy in early-stage lung cancer (13).
However, sublobar resection requires a thorough evaluation of the lesion and lymph node status. A study by Kadota et al. (14) found that patients with STAS(+) lung adenocarcinoma who underwent sublobar resection had a higher five-year cumulative recurrence (CIR) compared to those who had a lobectomy. This is consistent with other studies showing that STAS is an independent predictor of recurrence and is associated with significantly lower overall and disease-free survival (14-16). Given this evidence, sublobar resection should generally be avoided in patients with STAS(+) tumors (17-20). Therefore, accurate preoperative prediction of STAS is crucial for selecting the appropriate clinical treatment.
Currently, most reviews of STAS focus on clinical prognosis and pathologic diagnosis, with limited attention given to imaging studies. In this paper, we review and summarize recent studies on using CT imaging to predict STAS in lung cancer. We organize the existing literature into three main areas: traditional imaging features, radiomics, and deep learning methods, providing a comprehensive overview of the advancements in CT imaging for STAS prediction in recent years. We present this article in accordance with the Narrative Review reporting checklist (available at https://tlcr.amegroups.com/article/view/10.21037/tlcr-24-952/rc).
Methods
We conducted a literature search in Web of Science, PubMed, Cochrane Library, and EMBASE, selecting English-language articles published between July 2017 and June 2024 that focused on research related to STAS and CT. Search terms included “Spread Through Air Spaces”, “STAS”, and their synonyms, combined with “CT” or “Computed Tomography”. Where available, Medical Subject Headings (MeSH) were applied. A total of 133 papers were initially retrieved, and 32 articles were selected based on study design quality and relevance to a broader medical audience (Table 1).
Table 1
Items | Specification |
---|---|
Date of search | May 2024 to June 2024 |
Databases and other sources searched | Science, PubMed, Cochrane Library, and EMBASE |
Search terms used | Spread through air spaces, STAS, CT, computed tomography |
Timeframe | July 2017 and June 2024 |
Inclusion and exclusion criteria | Inclusion criteria: English-language articles; randomized controlled trials, prospective or retrospective cohort studies, case reports or series, case-control studies and translational preclinical studies |
Exclusion criteria: Non-English language articles; editorial comments, abstracts, conference materials, guidelines, consensus statements, and study protocols | |
Selection process | Study selection and full-text articles were assessed by Q.J. and X.W. |
CT, computed tomography; STAS, spread through air space.
The literature is divided into three sections based on different approaches: traditional imaging features of STAS, imaging radiomics models, and deep learning models. In each part, the literature is concatenated according to the research objects and findings.
Findings
Traditional imaging features of STAS
STAS is a pathologic concept that cannot be directly visualized, even with high-resolution CT images. However, certain CT features of lung cancer have been found to correlate with the presence of STAS. In this part, the relationship between quantitative (e.g., tumor size and solid tumor components on CT) and qualitative (e.g., tumor and peritumor imaging features) traditional imaging features and STAS was explored, primarily through the use of univariate and multivariate regression methods.
Tumor size
Several studies have demonstrated a relationship between tumor size and STAS (21-23). For instance, Li et al. (22) analyzed 578 patients with pathologically confirmed lung adenocarcinoma from two centers, using univariate and multivariate logistic regression. Their findings revealed that both the maximum diameter and the maximum area of the tumors (mm2) were significantly associated with STAS. Similarly, studies by Zhang et al. (21) and Qin et al. (23) identified the tumor’s maximum diameter as an independent predictor of STAS.
Additionally, most studies showed that a larger diameter corresponded to a higher incidence of STAS. Toyokawa et al. (24) found that STAS positivity was linked to both larger pathological tumor size and greater radiological tumor diameter. Some studies have offered recommendations regarding tumor size. For example, de Margerie-Mellon et al. (25) analyzed 40 STAS(+) and 40 STAS(−) sub-solid nodules, revealing a significant difference between these groups at a threshold of 20 mm in mean diameter. But no recommended threshold is given to distinguish the two groups. Uruga et al. (26) added to this deficiency by proposing that tumor diameters of ≥10 mm were significantly associated with STAS(+) in 208 cases of small (≤2 cm) stage I lung adenocarcinoma.
In a study by Dai et al. (27), it was concluded that STAS, when combined with tumor size, could more accurately predict patient prognosis. Specifically, in patients with 2-3 cm lung adenocarcinoma, STAS effectively stratified prognosis, with stage IA patients exhibiting STAS having a poorer outcome.
Solid tumor components on preoperative CT
Numerous studies have shown that both the size and percentage of the solid component of a tumor are significantly associated with the presence of STAS in patients with lung adenocarcinoma. Zeng et al. (28) conducted a retrospective study of 170 lung adenocarcinoma patients from December 2017 to November 2018 and found that only one STAS(+) patient presented with a pure ground-glass nodule. Multivariate logistic regression analysis revealed a significant correlation between solid nodules and STAS. This finding is supported by Gu et al. (29), who also reported a strong association between solid nodules and STAS in lung adenocarcinoma patients. Follow-up studies indicated that not only the nodule type (solid, part-solid, pure ground-glass) but also the size of the solid component had a significant correlation with STAS (21,22,25,30). In addition, Chen et al. (31), in a study of 434 patients with pathologically confirmed stage IA lung adenocarcinoma across two centers, confirmed that the percentage of the solid component was an independent risk factor for STAS.
Some studies have proposed recommendations regarding the threshold for the percentage of solid components. Gong et al. (32) found that 95% (152/160) of patients with STAS(+) had a consolidation tumor ratio (CTR) greater than 0.5 in 537 lung cancer patients, a finding consistent with the study of Ding et al. (33). Yin et al. (34) observed that solid nodules were more likely to be associated with STAS, and a CTR greater than 50% was identified as a significant independent predictor of STAS in diagnostic lung adenocarcinomas. Koezuka et al. (35) retrospectively analyzed 62 patients who underwent lobectomy for small (≤2 cm) lung adenocarcinomas and found that patients with STAS(+) tumors or a CT value of ≥−140.6 HU had a poor prognosis. The study concluded that incorporating STAS with GGO ratios and CT values may improve the accuracy of lung cancer recurrence prediction. However, the study’s limited sample size calls for further validation with the larger dataset.
Most studies mentioned above concluded that the size and proportion of the solid component are significantly related to STAS, and some of them gave suggested thresholds, but fewer studies have further analysed the efficacy of this threshold for predicting STAS. Kim et al. (36) analyzed 276 patients with lung adenocarcinoma and demonstrated that a cutoff value of 90% for the percentage of solid components could effectively discriminate STAS, achieving a sensitivity of 89.2% and a specificity of 60.3%. Qi (37) found in 190 (47+) small-sized lung adenocarcinomas (≤2 cm) that a CTR at the 83% threshold provided a sensitivity of 91.5% and specificity of 62.9% for distinguishing STAS, and the performance of the prediction model based on CTR [area under curve (AUC) =0.760] was superior to that of the model based on tumor diameter (AUC =0.640). It can be seen that using the percentage of solid components is able to predict STAS with good sensitivity, but the specificity needs to be improved.
Tumor and peritumor imaging features
In CT imaging, STAS is linked to the characteristics of the nodule. Toyokawa et al. (38) performed univariate and multivariate logistic regression of 327 cases of lung adenocarcinoma [191 STAS(+)]. Their results indicated that the presence of notching was independently associated with STAS. Chen et al. (31) identified lobulation as an independent risk factor for STAS.
STAS is linked not only to CT features related to the tumor itself but also to features of airways, blood vessels, pleura, and surrounding lung parenchyma. Zhang et al. (21) found that STAS was associated with cavitation, lobulation, air bronchogram, and vascular convergence. A retrospective study by Qi et al. (39) showed that among the conventional radiological signs, the satellite sign was significantly associated with STAS, achieving an AUC of 0.606. Additionally, Kim et al. (36) identified associations between STAS and radiological features such as central low attenuation, ill-defined opacity, and air bronchogram. Li et al. (22) included 578 patients with pathologically confirmed adenocarcinoma of the lung from two centers. They evaluated 21 radiomics features and found that eleven CT features, including pleural thickening, pleural retraction, and vascular cluster, were significantly correlated with STAS.
Qi et al. (37) retrospectively analyzed 190 (47+) cases of small-sized lung adenocarcinomas (≤2 cm) and identified the ground glass ribbon as a newly discovered indicator with the potential to predict STAS. They defined the ground glass ribbon as a CT finding characterized by a band-shaped ground glass opacity with a blurred edge extending from the nodule into the adjacent lung, possibly linked to reduced distal alveolar airspaces caused by terminal bronchial obstruction. The ground glass ribbon was confirmed as an independent risk predictor in a study by Wang et al. (30).
In summary, several CT imaging features have been found to correlate with STAS, with tumor size, solid component, and lobulation consistently showing significant associations across multiple studies. Other features, such as air bronchogram, pleural thickening, and vascular clustering, have also been linked to STAS in some studies. The most recently identified indicator, the ground glass ribbon, has been explored in two studies. However, as this feature was newly introduced by Lin et al., further validation is required to confirm its reliability as a diagnostic criterion (Table 2).
Table 2
Year | Author | Patient/STAS(+) | Independent predictor |
---|---|---|---|
2017 | Uruga (26) | Stage I lung adenocarcinomas (208/99) | Tumor size (≥10 mm) |
2018 | Kim (36) | Lung adenocarcinomas (276/92) | Solid component |
2018 | De Margerie-Mellon (25) | Lung adenocarcinomas (80/40) | Tumor size, solid component |
2018 | Toyokawa (38) | Lung adenocarcinomas (327/191) | Solid component, CT features (the presence of notch) |
2020 | Zhang (21) | Stage IA lung adenocarcinoma (762/83) | Tumor size, solid component, CT features (air bronchogram) |
2020 | Zeng (28) | Lung adenocarcinomas (340/170) | Solid component |
2020 | Li (22) | Lung adenocarcinomas (578/118) | Tumor size, solid component, CT features (pleural thickening, pleural retraction, mediastinal lymph node enlargement, vascular cluster feature, and lobulation, specula) |
2021 | Qi (37) | Small-sized lung adenocarcinoma (≤2 cm, 190/47) | Solid component, CT features (the ground glass ribbon) |
2022 | Chen (31) | Stage IA lung adenocarcinoma (434/53) | Solid component, CT features (lobulation) |
2023 | Wang (30) | Stage IA non-small cell lung cancer (405/118) | Solid component, CT features (distal ribbon feature) |
2022 | Qin (23) | Stage IA non-small cell lung cancer (503/247) | Solid component, CT features (vacuole, spiculation) |
CT, computed tomography; STAS, spread through air space.
Imaging radiomics models
While recognition of tumor imaging features with the naked eye can be subjective and limited by image resolution, radiomics allows for the rapid extraction of internal quantitative features, transforming them into vast amounts of analyzable data (40). This capability provides much more detailed information, contributing to the rapid worldwide popularization of radiomics in medical imaging, including in STAS studies of lung cancer.
Tumor
Several studies have demonstrated the efficacy of CT imaging radiomics in predicting STAS. Chen et al. (41) analyzed 233 lung adenocarcinoma lesions as regions of interest (ROIs) for outline segmentation and extracted radiomic features to build a prediction model, achieving an AUC of 0.63. This result indicated that a CT-based radiomics model could predict STAS. Similarly, Jiang et al. (42) conducted a retrospective study involving 462 patients who underwent lung adenocarcinoma surgery, extracting 12 radiomics features to develop a random forest model, which yielded an AUC of 0.754. Gong et al. (32) obtained an AUC of 0.802. Bassi et al. (43) prospectively validated a radiologic-radiomics model, achieving an AUC of 0.79 in the internal validation set, further supporting the potential of radiomics-based models in predicting STAS. However, these studies focused solely on the extraction and analysis of intratumoral radiomic features, without utilizing peri-tumor information.
Peritumor information
Since STAS is a pathological finding present at the tumor margins, incorporating radiomics information from the peritumoral area in preoperative CT images may enhance the accuracy of STAS prediction. Kadota et al. (14) showed that the median distance of STAS from the tumor margin was 1.5 mm, and covering 5 mm beyond the tumor captured approximately 90% of cases with STAS. The maximum distance observed between STAS and the tumor margin was 1.35 cm (27).
Peritumoral features are closely associated with STAS. Liao et al. (44) constructed a radiomics model based on features extracted from the tumor and the surrounding peritumoral regions (5, 10, 15, and 20 mm) in 256 patients with stage I lung adenocarcinoma. The model performed best with features from the 15 mm peritumoral region (AUC =0.845). Combining the radiomics features of the tumor and peritumor models, the resulting fusion model achieved the highest AUC across all cohorts (AUC =0.854).
In developing the peritumoral STAS prediction model, the methods used for tumor segmentation and the expansion of the peritumoral region can significantly impact the model’s performance. Zhuo et al. (45) constructed prediction models by extending the segmentation of lung nodules by 5, 10 and 15 mm. While this approach achieved high prediction efficacy, the calibration curve showed a poor fit. In this study, the radiomics model was developed by expanding 5, 10 and 15 mm from the tumor surface using point localization and regional growth methods. However, for tumors near the chest wall and mediastinum, this method was unable to avoid areas other than lung tissue. Qi et al. (39) addressed this by designing a program to capture the peritumor region while excluding extrapulmonary areas. Radiomics features were extracted from the tumor and its surrounding margins at distances of 2, 4, 6, 8, 10, and 20 mm. The tumor-peritumor fusion model yielded good performance (AUC 0.907, 0.897).
The STAS prediction model based on imaging radiomics can also help assess patient prognosis and select appropriate candidates for sublobectomy. Takehana et al. (46) defined ROIs by extending 5 mm inward and 5 mm outward from the tumor surface to construct a STAS radiomics prediction model, achieving an AUC of 0.76. Based on the model’s predictions, patients were categorized into a high-risk and low-risk group of STAS. In the sublobectomy group, the risk of recurrence was significantly higher in the STAS high-risk group than in the STAS low-risk group. In contrast, in the lobectomy group, there was no significant difference in the risk of recurrence between the two groups. This finding is further supported by Onozato et al. (47). For patients undergoing sublobectomy, the STAS high-risk group showed a higher incidence of CIR and poorer OS.
Most of the prediction models mentioned above rely on CT imaging features. However, it has been reported that clinical factors, such as age and gender, are also significant predictors of STAS. Liao et al. demonstrated that a combined model incorporating age and CEA status outperformed the tumor-peritumoral image model, achieving an AUC of 0.871 compared to 0.854 (44). Similarly, Han et al. found that a combined model including age, gender, and smoking history was more effective than a radiomics-only model (48). Additionally, many studies have demonstrated that combining radiomics features with clinical data can result in more accurate STAS prediction models (44,48-50).
In conclusion, radiomics models have proven effective in predicting STAS, and integrating peritumoral and clinical information further enhances the predictive accuracy of the model. However, several key considerations should be noted when constructing the peritumoral radiomics model. First, when expanding the tumor margin outward using algorithms, it is crucial to exclude normal tissue structures such as the pleura and mediastinal soft tissues. This can be accomplished through additional programming or manual judging to remove those not meeting the criteria. Second, the construction of a fusion model combining tumor and peritumor is currently implemented in several ways, which can be achieved by fusing ROI regions. On the other hand, some studies construct a prediction model by extracting tumor features and peritumoral characteristics separately and integrating the radiomics features of the two. There is no consensus yet on which method yields the best results. Third, there is no universal threshold for defining the optimal range of tumor-peritumor ROI. For example, as shown in Figure 1, most studies on tumor-peritumor fusion models have taken the entire tumor extent and its outward extension. However, Takehana et al. (46) used a narrower range, extending 5 mm inward. Despite the differences, all the above studies have achieved strong predictive efficacy (Table 3).
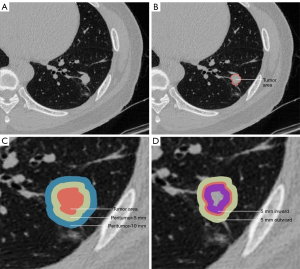
Table 3
Year | Author | Patient/STAS(+) | Method | ROI | AUC |
---|---|---|---|---|---|
2020 | Zhuo (45) | Lung adenocarcinoma (212/107) | Radiomics + clinical signatures | Tumor, peritumor-5, 10, 15, 20 mm | 0.99 |
2020 | Jiang (42) | Lung adenocarcinoma (462/90) | Radiomics + clinical signatures | Tumor area | 0.754 |
2020 | Chen (41) | Stage I lung adenocarcinoma (233/69) | Radiomics | Tumor area | 0.63 |
2021 | Onozato (47) | Non-small cell lung cancer (≤2 cm, 226/35) | Radiomics | Tumor area | 0.77 |
2021 | Qi (39) | Lung adenocarcinoma (216/56) | Tumor + peritumoral radiomics signature | Tumor, peritumor-5, 10, 15, 20 mm | 0.907 |
2022 | Takehana (46) | Lung adenocarcinoma (339/95) | Tumor + peritumoral radiomics signature | 5 mm inward and 5 mm outward from the tumor surface | 0.76 |
2022 | Bassi (43) | Retrospective lung adenocarcinoma (99/65), prospective study (50/33) | Radiomics + radiological signature | Tumor area | 0.79 |
2022 | Liao (44) | Stage I lung adenocarcinoma (256/85) | Tumor + peritumoral radiomics signature + clinical signatures | Tumor, peritumor-5, 10, 15, 20 mm | 0.869 |
2023 | Gong (32) | Lung cancer (537/205) | Radiomics | Tumor area | 0.802 |
2024 | Suh (50) | Early stage lung adenocarcinoma (550/174) | Radiomics + clinical signatures | Tumor area | 0.815 |
AUC, area under curve; ROI, regions of interest; STAS, spread through air space.
Deep learning models
Deep learning methods have been widely used in the medical field due to their ability to handle complex tasks, and they have shown excellent efficacy in predicting STAS. Tao et al. (51) analyzed 203 patients with non-small cell lung cancer and compared the predictive performance of a clinicopathological/CT model, a conventional radiomics model, a computer vision model, and a three-dimensional (3D) convolutional neural network (CNN) model. Their results indicated that the 3D-CNN model had the best predictive efficacy. Besides, Lin et al. (52) demonstrated that prediction models using the deep learning method outperformed others, achieving the best performance with an AUC of 0.82.
Deep learning can also be applied flexibly for feature extraction. Jin et al. (53) extracted traditional radiomics features, deep learning features, delta-radiomics, and delta-DL features, respectively, and combined these to develop a prediction model named the double delta model. This model demonstrated satisfactory predictive performance for STAS (AUC, 0.94) and showed reliable performance in a multi-center cohort validation. This study used deep learning based on image registration and subtraction, demonstrating that combining radiomics-based quantitative analysis with CNN-based machine vision effectively improves model performance. These advancements offer promising methods for future clinical applications, including patient follow-up.
Wang et al. (54) illustrated that the integrating deep learning method with an attention mechanism could further enhance the prediction efficacy of STAS models. In a study of 602 lung adenocarcinoma patients, the use of deep learning combined with the attention mechanism yielded substantial improvements in performance (AUC =0.933) compared to the conventional deep learning model (AUC =0.728).
Limitations and direction of development
The above studies have shown that CT-based STAS prediction models can achieve good performance and effectively assist in clinical surgical decision-making and patient prognosis. To promote CT-based STAS prediction, it is crucial to refine and standardize the definition and grading criteria of STAS. The detection of tumor cells in areas beyond the tumor margins through postoperative pathology remains the gold standard for diagnosing STAS. However, there is no consensus on the minimum number of tumor cells (or clusters) required or the necessary distance from the main tumor border (15,55,56). Uruga and Toyokawa classified STAS into three categories: no STAS, low STAS (1–4 single cells or clusters of STAS), and high STAS (≥5 single cells or clusters of STAS) using a 20× objective and a 10× ocular lens (24,26). Although STAS has long been recognized as a crucial concept in thoracic pathology with established clinical relevance, its definition and grading criteria remain insufficiently precise, which may contribute to misunderstandings and inconsistencies in interpretation.
Besides, different tumors may show different tumor and peritumor features, and the imaging features included in different studies can also vary. Despite this, some common features, such as CTR and lobulation, have shown their value in several studies and have been preliminarily validated. Further research is needed to confirm these findings and broaden the evidence base. In addition, although deep learning technology has shown great potential in medical applications, the number of studies applying it to STAS prediction model development remains limited. Further research could lead to more robust predictive models and improved clinical outcomes.
Conclusions
The STAS prediction models based on preoperative CT imaging have demonstrated good efficacy using traditional imaging, radiomics models, and deep learning models. These findings support more informed clinical decisions regarding surgical interventions, promoting the clinical integration of precision medicine. However, a more precise definition of STAS and further validation through large-sample, prospective, and multi-center studies are necessary for the future.
Acknowledgments
None.
Footnote
Reporting Checklist: The authors have completed the Narrative Review reporting checklist. Available at https://tlcr.amegroups.com/article/view/10.21037/tlcr-24-952/rc
Peer Review File: Available at https://tlcr.amegroups.com/article/view/10.21037/tlcr-24-952/prf
Funding: This work has received funding from
Conflicts of Interest: All authors have completed the ICMJE uniform disclosure form (available at https://tlcr.amegroups.com/article/view/10.21037/tlcr-24-952/coif). The authors have no conflicts of interest to declare.
Ethical Statement: The authors are accountable for all aspects of the work in ensuring that questions related to the accuracy or integrity of any part of the work are appropriately investigated and resolved.
Open Access Statement: This is an Open Access article distributed in accordance with the Creative Commons Attribution-NonCommercial-NoDerivs 4.0 International License (CC BY-NC-ND 4.0), which permits the non-commercial replication and distribution of the article with the strict proviso that no changes or edits are made and the original work is properly cited (including links to both the formal publication through the relevant DOI and the license). See: https://creativecommons.org/licenses/by-nc-nd/4.0/.
References
- Sung H, Ferlay J, Siegel RL, et al. Global Cancer Statistics 2020: GLOBOCAN Estimates of Incidence and Mortality Worldwide for 36 Cancers in 185 Countries. CA Cancer J Clin 2021;71:209-49. [Crossref] [PubMed]
- Zhang Y, Luo G, Etxeberria J, et al. Global Patterns and Trends in Lung Cancer Incidence: A Population-Based Study. J Thorac Oncol 2021;16:933-44. [Crossref] [PubMed]
- Travis WD, Brambilla E, Nicholson AG, et al. The 2015 World Health Organization Classification of Lung Tumors: Impact of Genetic, Clinical and Radiologic Advances Since the 2004 Classification. J Thorac Oncol 2015;10:1243-60. [Crossref] [PubMed]
- Villalba JA, Shih AR, Sayo TMS, et al. Accuracy and Reproducibility of Intraoperative Assessment on Tumor Spread Through Air Spaces in Stage 1 Lung Adenocarcinomas. J Thorac Oncol 2021;16:619-29. [Crossref] [PubMed]
- Zhou F, Villalba JA, Sayo TMS, et al. Assessment of the feasibility of frozen sections for the detection of spread through air spaces (STAS) in pulmonary adenocarcinoma. Mod Pathol 2022;35:210-7. [Crossref] [PubMed]
- Walts AE, Marchevsky AM. Current Evidence Does Not Warrant Frozen Section Evaluation for the Presence of Tumor Spread Through Alveolar Spaces. Arch Pathol Lab Med 2018;142:59-63. [Crossref] [PubMed]
- Isaka T, Yokose T, Miyagi Y, et al. Detection of tumor spread through airspaces by airway secretion cytology from resected lung cancer specimens. Pathol Int 2017;67:487-94. [Crossref] [PubMed]
- Kameda K, Lu S, Eguchi T, et al. MA12.05 Can Tumor Spread through Air Spaces (STAS) in Lung Adenocarcinomas Be Predicted Pre- and Intraoperatively? J Thorac Oncol 2017;12:S411-2.
- Brown LM, Louie BE, Jackson N, et al. Recurrence and Survival After Segmentectomy in Patients With Prior Lung Resection for Early-Stage Non-Small Cell Lung Cancer. Ann Thorac Surg 2016;102:1110-8. [Crossref] [PubMed]
- Takahashi Y, Kuroda H, Oya Y, et al. Challenges for real-time intraoperative diagnosis of high risk histology in lung adenocarcinoma: A necessity for sublobar resection. Thorac Cancer 2019;10:1663-8. [Crossref] [PubMed]
- Madariaga ML, Lennes IT, Best T, et al. Multidisciplinary selection of pulmonary nodules for surgical resection: Diagnostic results and long-term outcomes. J Thorac Cardiovasc Surg 2020;159:1558-1566.e3. [Crossref] [PubMed]
- Masai K, Sakurai H, Sukeda A, et al. Prognostic Impact of Margin Distance and Tumor Spread Through Air Spaces in Limited Resection for Primary Lung Cancer. J Thorac Oncol 2017;12:1788-97. [Crossref] [PubMed]
- Saji H, Okada M, Tsuboi M, et al. Segmentectomy versus lobectomy in small-sized peripheral non-small-cell lung cancer (JCOG0802/WJOG4607L): a multicentre, open-label, phase 3, randomised, controlled, non-inferiority trial. Lancet 2022;399:1607-17. [Crossref] [PubMed]
- Kadota K, Nitadori JI, Sima CS, et al. Tumor Spread through Air Spaces is an Important Pattern of Invasion and Impacts the Frequency and Location of Recurrences after Limited Resection for Small Stage I Lung Adenocarcinomas. J Thorac Oncol 2015;10:806-14. [Crossref] [PubMed]
- Morimoto J, Nakajima T, Suzuki H, et al. Impact of free tumor clusters on prognosis after resection of pulmonary adenocarcinoma. J Thorac Cardiovasc Surg 2016;152:64-72.e1. [Crossref] [PubMed]
- Warth A, Muley T, Kossakowski CA, et al. Prognostic Impact of Intra-alveolar Tumor Spread in Pulmonary Adenocarcinoma. Am J Surg Pathol 2015;39:793-801. [Crossref] [PubMed]
- Liu H, Yin Q, Yang G, et al. Prognostic Impact of Tumor Spread Through Air Spaces in Non-small Cell Lung Cancers: a Meta-Analysis Including 3564 Patients. Pathol Oncol Res 2019;25:1303-10. [Crossref] [PubMed]
- Li J, Wang Y, Li J, et al. Meta-analysis of Lobectomy and Sublobar Resection for Stage I Non-small Cell Lung Cancer With Spread Through Air Spaces. Clin Lung Cancer 2022;23:208-13. [Crossref] [PubMed]
- Eguchi T, Kameda K, Lu S, et al. Lobectomy Is Associated with Better Outcomes than Sublobar Resection in Spread through Air Spaces (STAS)-Positive T1 Lung Adenocarcinoma: A Propensity Score-Matched Analysis. J Thorac Oncol 2019;14:87-98. [Crossref] [PubMed]
- Shiono S, Endo M, Suzuki K, et al. Spread Through Air Spaces Is a Prognostic Factor in Sublobar Resection of Non-Small Cell Lung Cancer. Ann Thorac Surg 2018;106:354-60. [Crossref] [PubMed]
- Zhang Z, Liu Z, Feng H, et al. Predictive value of radiological features on spread through air space in stage cIA lung adenocarcinoma. J Thorac Dis 2020;12:6494-504. [Crossref] [PubMed]
- Li C, Jiang C, Gong J, et al. A CT-based logistic regression model to predict spread through air space in lung adenocarcinoma. Quant Imaging Med Surg 2020;10:1984-93. [Crossref] [PubMed]
- Qin L, Sun Y, Zhu R, et al. Clinicopathological and CT features of tumor spread through air space in invasive lung adenocarcinoma. Front Oncol 2022;12:959113. [Crossref] [PubMed]
- Toyokawa G, Yamada Y, Tagawa T, et al. Significance of Spread Through Air Spaces in Resected Pathological Stage I Lung Adenocarcinoma. Ann Thorac Surg 2018;105:1655-63. [Crossref] [PubMed]
- de Margerie-Mellon C, Onken A, Heidinger BH, et al. CT Manifestations of Tumor Spread Through Airspaces in Pulmonary Adenocarcinomas Presenting as Subsolid Nodules. J Thorac Imaging 2018;33:402-8. [Crossref] [PubMed]
- Uruga H, Fujii T, Fujimori S, et al. Semiquantitative Assessment of Tumor Spread through Air Spaces (STAS) in Early-Stage Lung Adenocarcinomas. J Thorac Oncol 2017;12:1046-51. [Crossref] [PubMed]
- Dai C, Xie H, Su H, et al. Tumor Spread through Air Spaces Affects the Recurrence and Overall Survival in Patients with Lung Adenocarcinoma >2 to 3 cm. J Thorac Oncol. 2017;12:1052-60. [Crossref] [PubMed]
- Zeng Q, Wang B, Li J, et al. Solid Nodule Appearance as a Predictor of Tumor Spread Through Air Spaces in Patients with Lung Adenocarcinoma: A Propensity Score Matching Study. Cancer Manag Res 2020;12:8197-207. [Crossref] [PubMed]
- Gu Y, Zheng B, Zhao T, et al. Computed Tomography Features and Tumor Spread Through Air Spaces in Lung Adenocarcinoma: A Meta-analysis. J Thorac Imaging 2023;38:W19-29. [Crossref] [PubMed]
- Wang Y, Lyu D, Zhang D, et al. Nomogram based on clinical characteristics and radiological features for the preoperative prediction of spread through air spaces in patients with clinical stage IA non-small cell lung cancer: a multicenter study. Diagn Interv Radiol 2023;29:771-85. [Crossref] [PubMed]
- Chen Y, Jiang C, Kang W, et al. Development and validation of a CT-based nomogram to predict spread through air space (STAS) in peripheral stage IA lung adenocarcinoma. Jpn J Radiol 2022;40:586-94. [Crossref] [PubMed]
- Gong J, Yin R, Sun L, et al. CT-based radiomics model to predict spread through air space in resectable lung cancer. Cancer Med 2023;12:18755-66. [Crossref] [PubMed]
- Ding Y, Chen Y, Wen H, et al. Pretreatment prediction of tumour spread through air spaces in clinical stage I non-small-cell lung cancer. Eur J Cardiothorac Surg 2022;62:ezac248. [Crossref] [PubMed]
- Yin Q, Wang H, Cui H, et al. Meta-analysis of association between CT-based features and tumor spread through air spaces in lung adenocarcinoma. J Cardiothorac Surg 2020;15:243. [Crossref] [PubMed]
- Koezuka S, Mikami T, Tochigi N, et al. Toward improving prognosis prediction in patients undergoing small lung adenocarcinoma resection: Radiological and pathological assessment of diversity and intratumor heterogeneity. Lung Cancer 2019;135:40-6. [Crossref] [PubMed]
- Kim SK, Kim TJ, Chung MJ, et al. Lung Adenocarcinoma: CT Features Associated with Spread through Air Spaces. Radiology 2018;289:831-40. [Crossref] [PubMed]
- Qi L, Xue K, Cai Y, et al. Predictors of CT Morphologic Features to Identify Spread Through Air Spaces Preoperatively in Small-Sized Lung Adenocarcinoma. Front Oncol 2020;10:548430. [Crossref] [PubMed]
- Toyokawa G, Yamada Y, Tagawa T, et al. Computed tomography features of resected lung adenocarcinomas with spread through air spaces. J Thorac Cardiovasc Surg 2018;156:1670-1676.e4. [Crossref] [PubMed]
- Qi L, Li X, He L, et al. Comparison of Diagnostic Performance of Spread Through Airspaces of Lung Adenocarcinoma Based on Morphological Analysis and Perinodular and Intranodular Radiomic Features on Chest CT Images. Front Oncol 2021;11:654413. [Crossref] [PubMed]
- Lambin P, Rios-Velazquez E, Leijenaar R, et al. Radiomics: extracting more information from medical images using advanced feature analysis. Eur J Cancer 2012;48:441-6. [Crossref] [PubMed]
- Chen D, She Y, Wang T, et al. Radiomics-based prediction for tumour spread through air spaces in stage I lung adenocarcinoma using machine learning. Eur J Cardiothorac Surg 2020;58:51-8. [Crossref] [PubMed]
- Jiang C, Luo Y, Yuan J, et al. CT-based radiomics and machine learning to predict spread through air space in lung adenocarcinoma. Eur Radiol 2020;30:4050-7. [Crossref] [PubMed]
- Bassi M, Russomando A, Vannucci J, et al. Role of radiomics in predicting lung cancer spread through air spaces in a heterogeneous dataset. Transl Lung Cancer Res 2022;11:560-71. [Crossref] [PubMed]
- Liao G, Huang L, Wu S, et al. Preoperative CT-based peritumoral and tumoral radiomic features prediction for tumor spread through air spaces in clinical stage I lung adenocarcinoma. Lung Cancer 2022;163:87-95. [Crossref] [PubMed]
- Zhuo Y, Feng M, Yang S, et al. Radiomics nomograms of tumors and peritumoral regions for the preoperative prediction of spread through air spaces in lung adenocarcinoma. Transl Oncol 2020;13:100820. [Crossref] [PubMed]
- Takehana K, Sakamoto R, Fujimoto K, et al. Peritumoral radiomics features on preoperative thin-slice CT images can predict the spread through air spaces of lung adenocarcinoma. Sci Rep 2022;12:10323. [Crossref] [PubMed]
- Onozato Y, Nakajima T, Yokota H, et al. Radiomics is feasible for prediction of spread through air spaces in patients with nonsmall cell lung cancer. Sci Rep 2021;11:13526. [Crossref] [PubMed]
- Han X, Fan J, Zheng Y, et al. The Value of CT-Based Radiomics for Predicting Spread Through Air Spaces in Stage IA Lung Adenocarcinoma. Front Oncol 2022;12:757389. [Crossref] [PubMed]
- Jia M, Yu S, Gao H, et al. Spread Through Air Spaces (STAS) in Lung Cancer: A Multiple-Perspective and Update Review. Cancer Manag Res 2020;12:2743-52. [Crossref] [PubMed]
- Suh YJ, Han K, Kwon Y, et al. Computed Tomography Radiomics for Preoperative Prediction of Spread Through Air Spaces in the Early Stage of Surgically Resected Lung Adenocarcinomas. Yonsei Med J 2024;65:163-73. [Crossref] [PubMed]
- Tao J, Liang C, Yin K, et al. 3D convolutional neural network model from contrast-enhanced CT to predict spread through air spaces in non-small cell lung cancer. Diagn Interv Imaging 2022;103:535-44. [Crossref] [PubMed]
- Lin MW, Chen LW, Yang SM, et al. CT-Based Deep-Learning Model for Spread-Through-Air-Spaces Prediction in Ground Glass-Predominant Lung Adenocarcinoma. Ann Surg Oncol 2024;31:1536-45. [Crossref] [PubMed]
- Jin W, Shen L, Tian Y, et al. Improving the prediction of Spreading Through Air Spaces (STAS) in primary lung cancer with a dynamic dual-delta hybrid machine learning model: a multicenter cohort study. Biomark Res 2023;11:102. [Crossref] [PubMed]
- Wang S, Liu X, Jiang C, et al. CT-Based Super-Resolution Deep Learning Models with Attention Mechanisms for Predicting Spread Through Air Spaces of Solid or Part-Solid Lung Adenocarcinoma. Acad Radiol 2024;31:2601-9. [Crossref] [PubMed]
- Shiono S, Yanagawa N. Spread through air spaces is a predictive factor of recurrence and a prognostic factor in stage I lung adenocarcinoma. Interact Cardiovasc Thorac Surg 2016;23:567-72. [Crossref] [PubMed]
- Lu S, Tan KS, Kadota K, et al. Spread through Air Spaces (STAS) Is an Independent Predictor of Recurrence and Lung Cancer-Specific Death in Squamous Cell Carcinoma. J Thorac Oncol 2017;12:223-34. [Crossref] [PubMed]